Back to Courses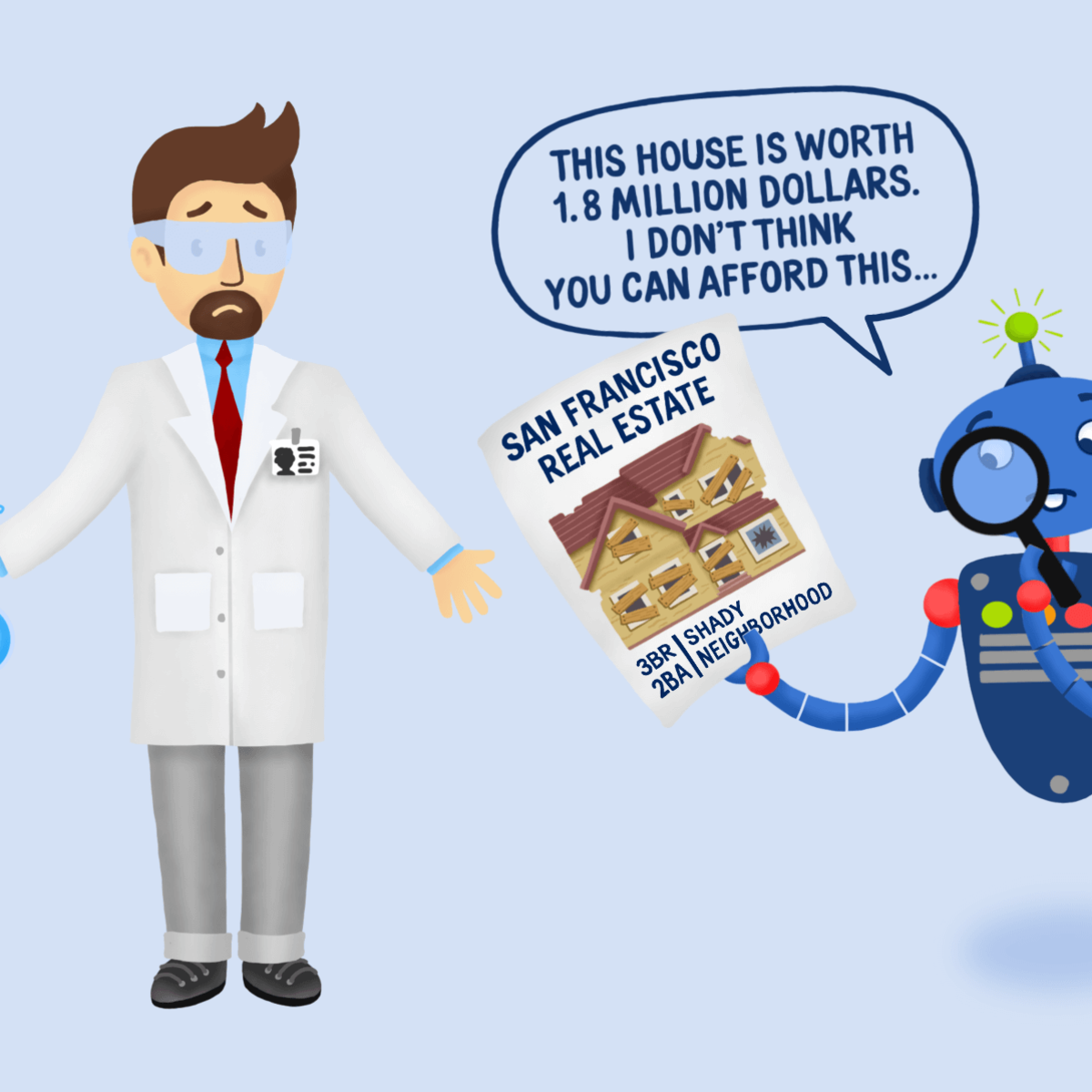
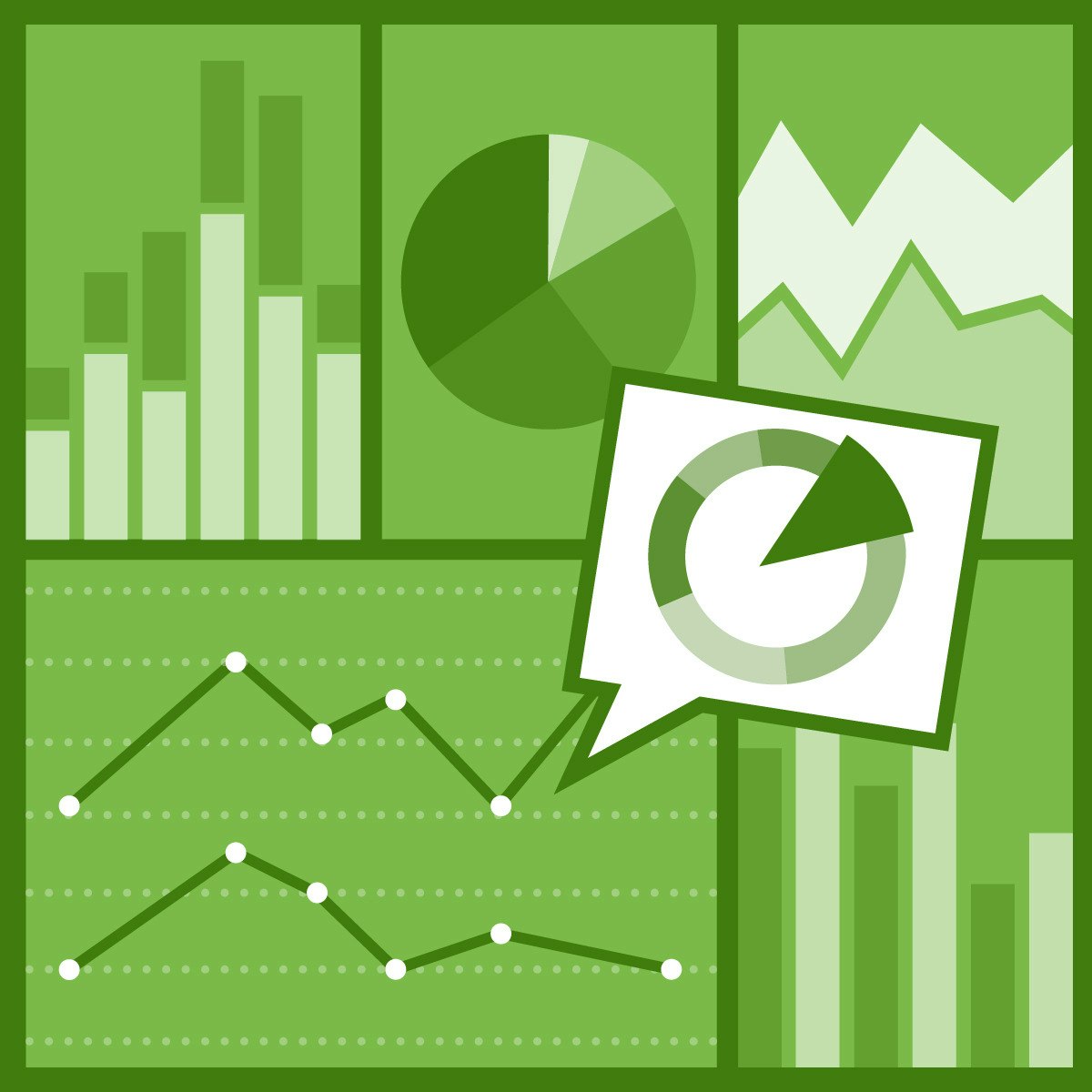
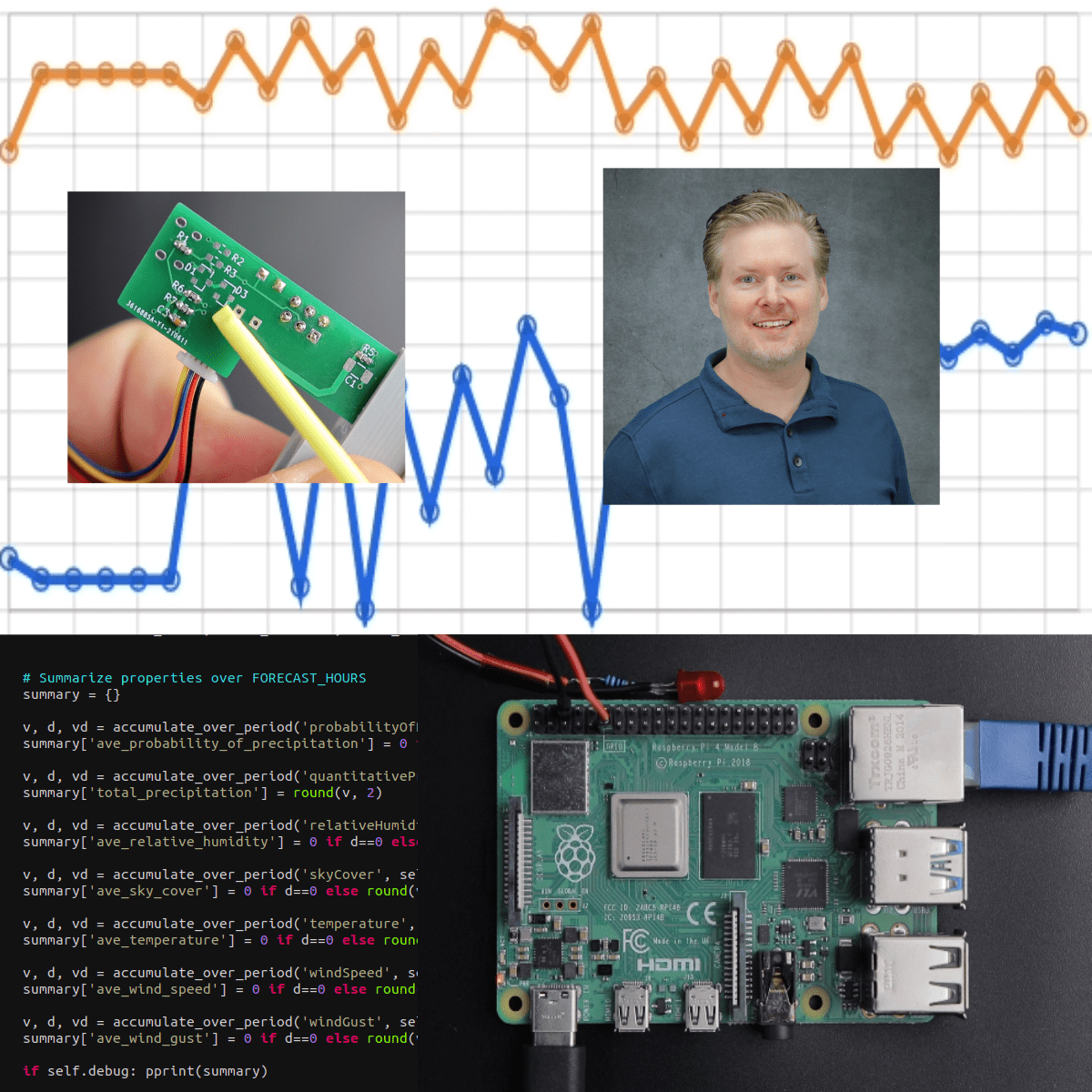
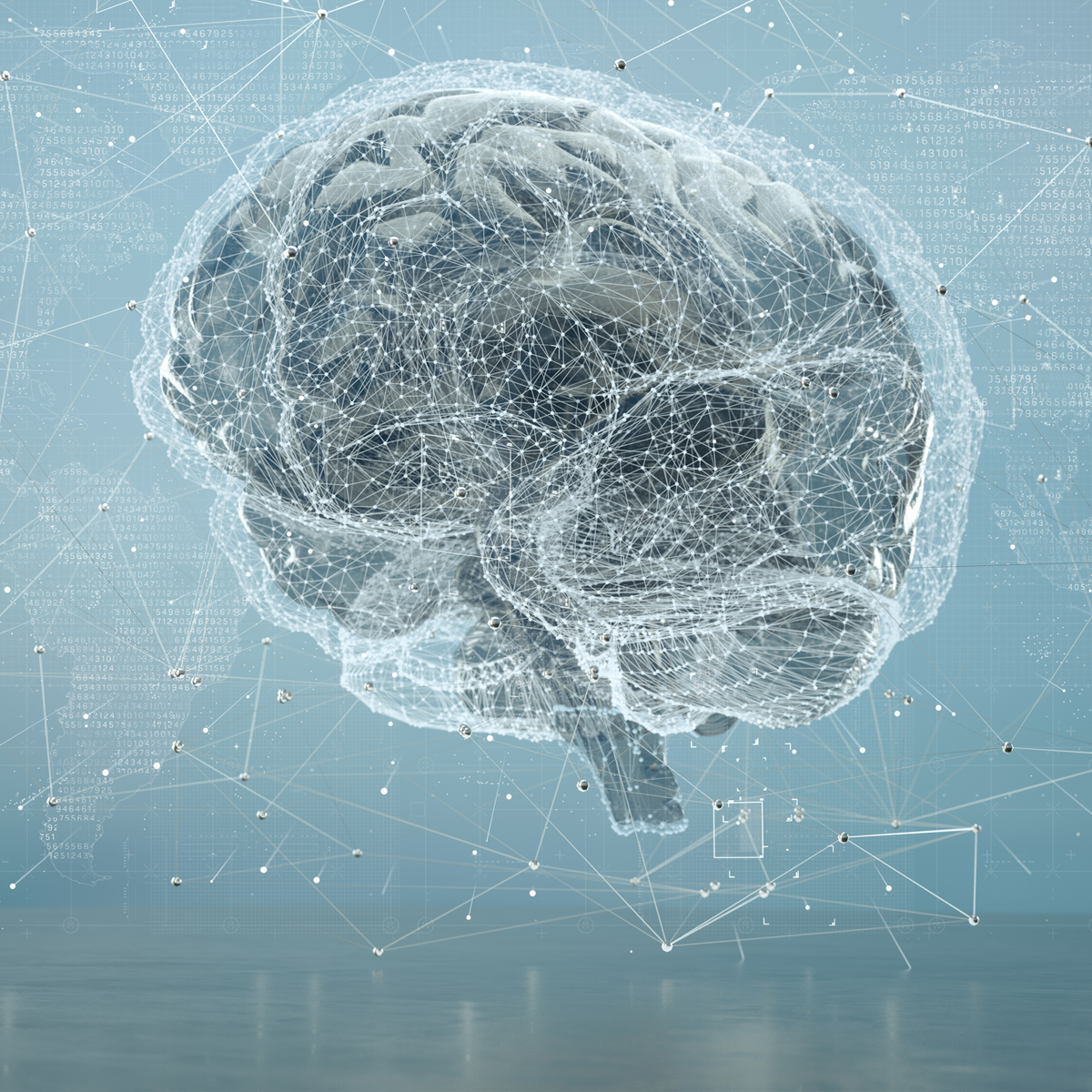
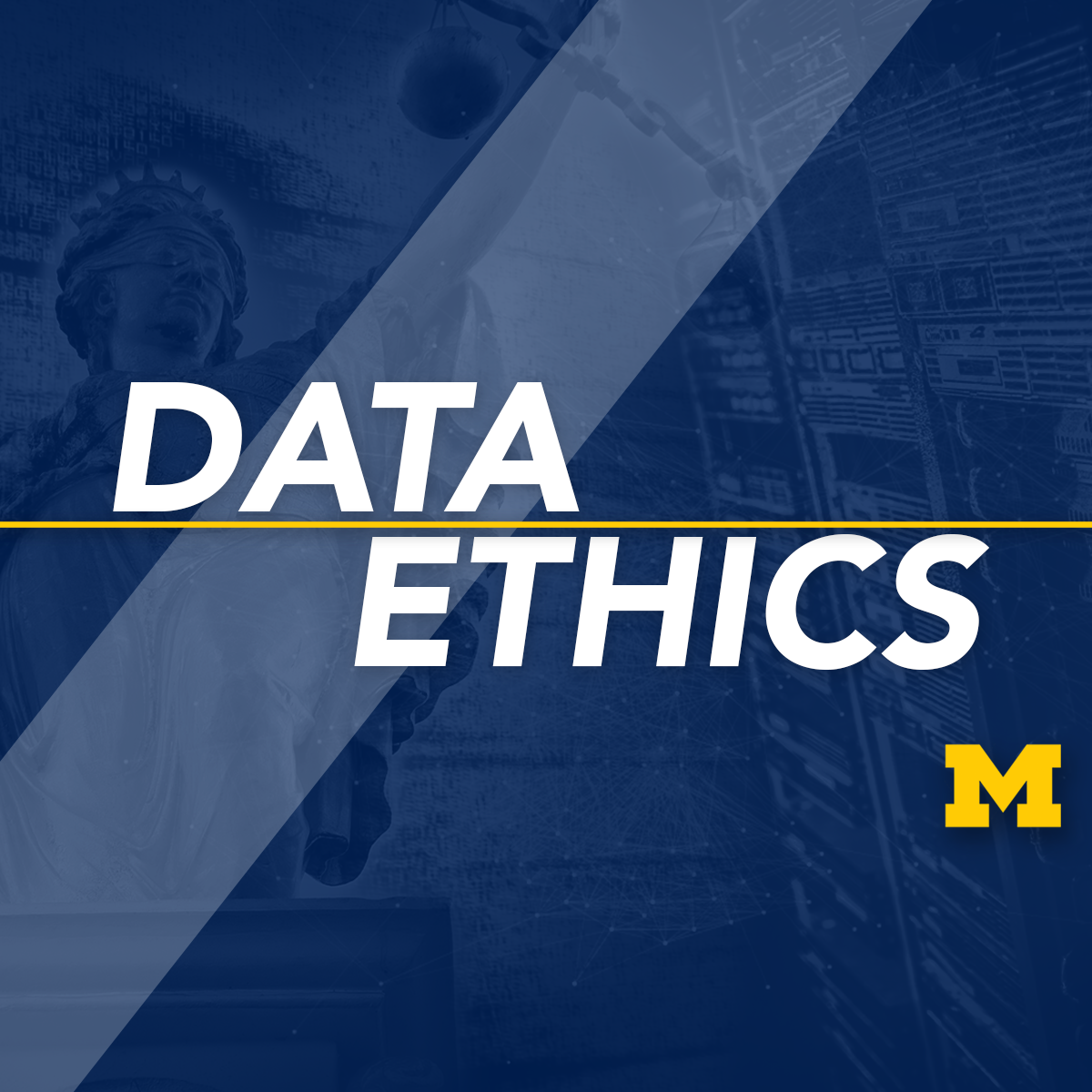
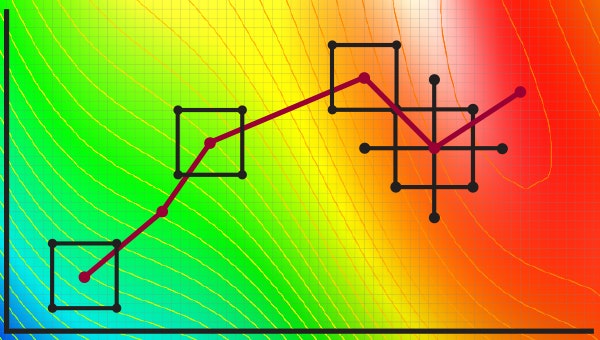
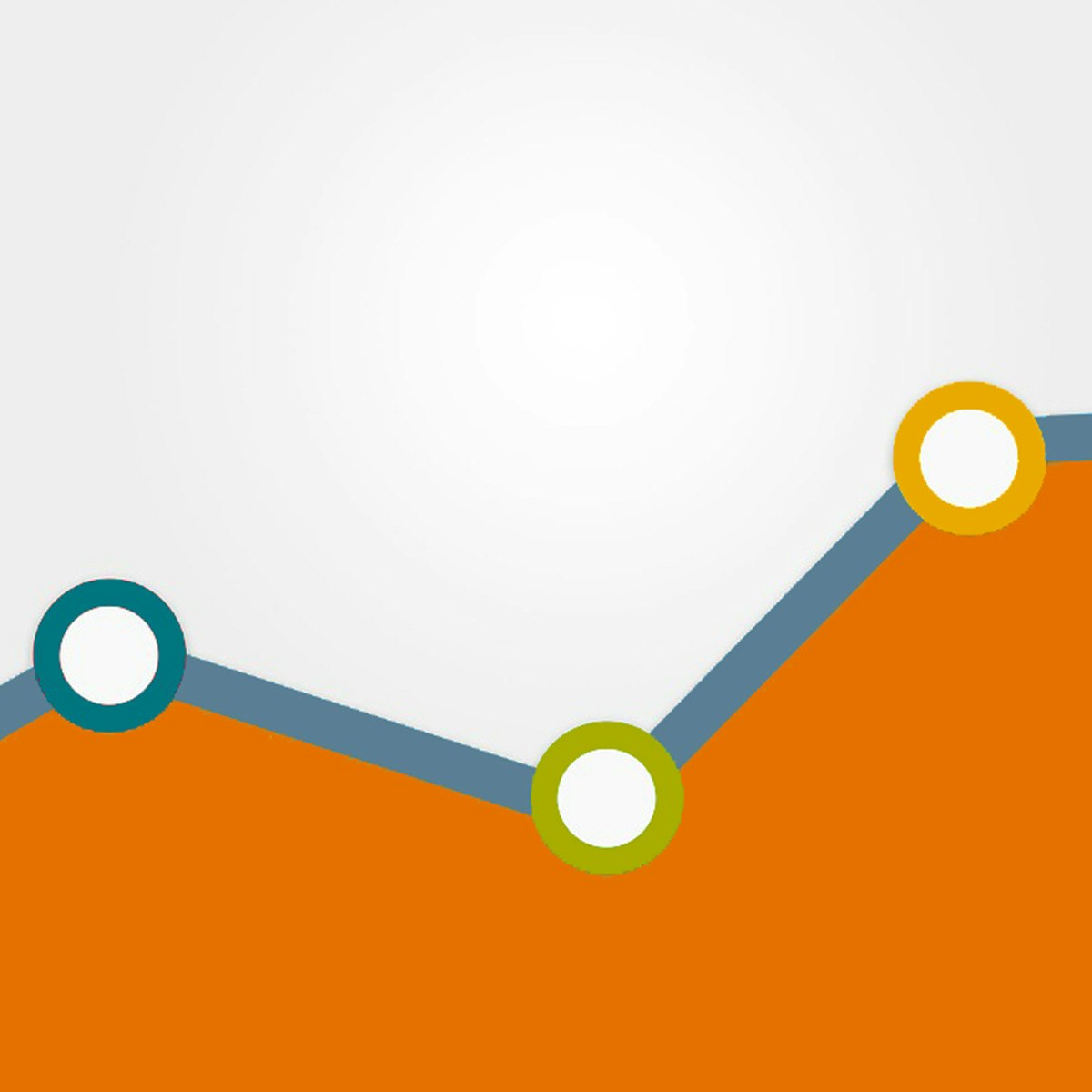
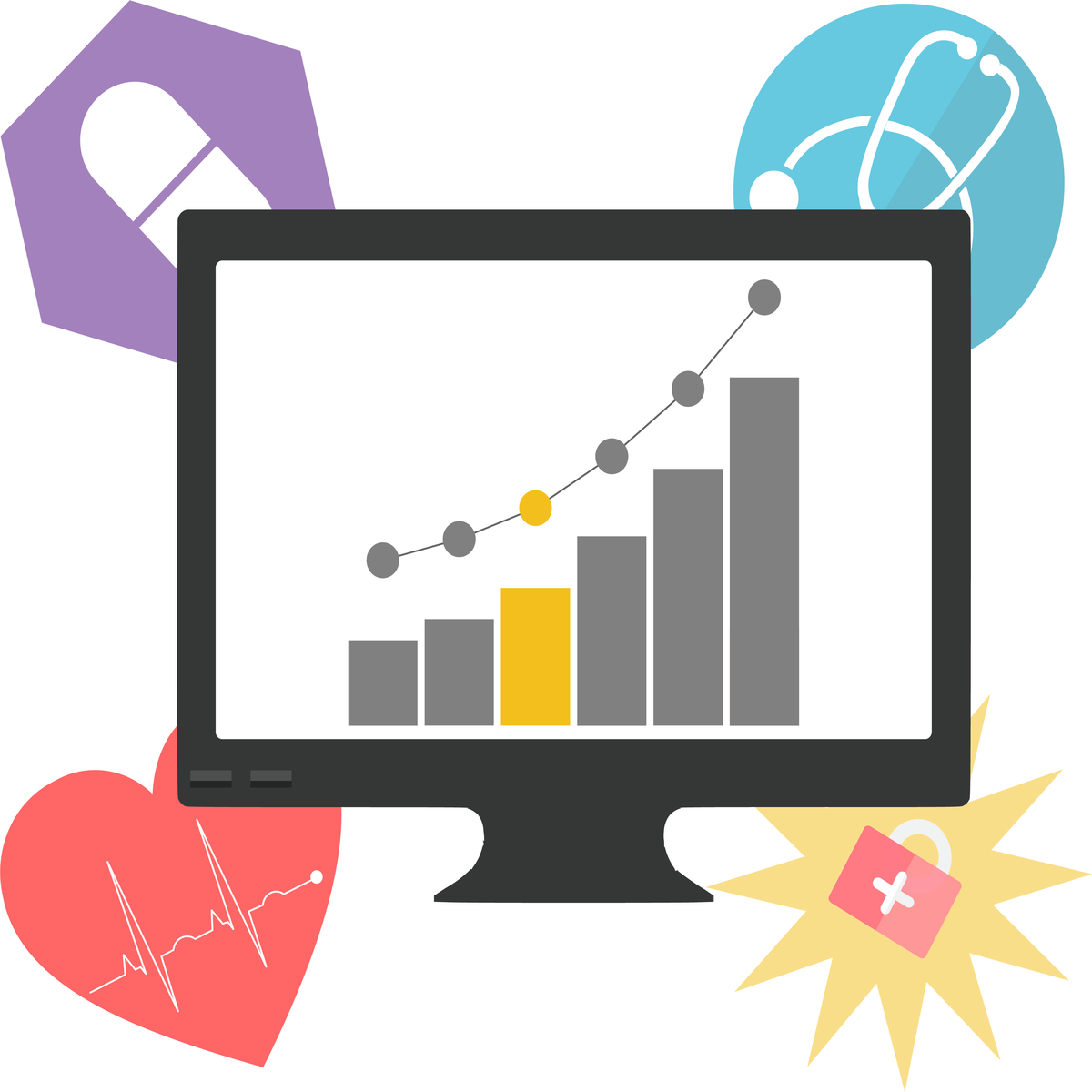
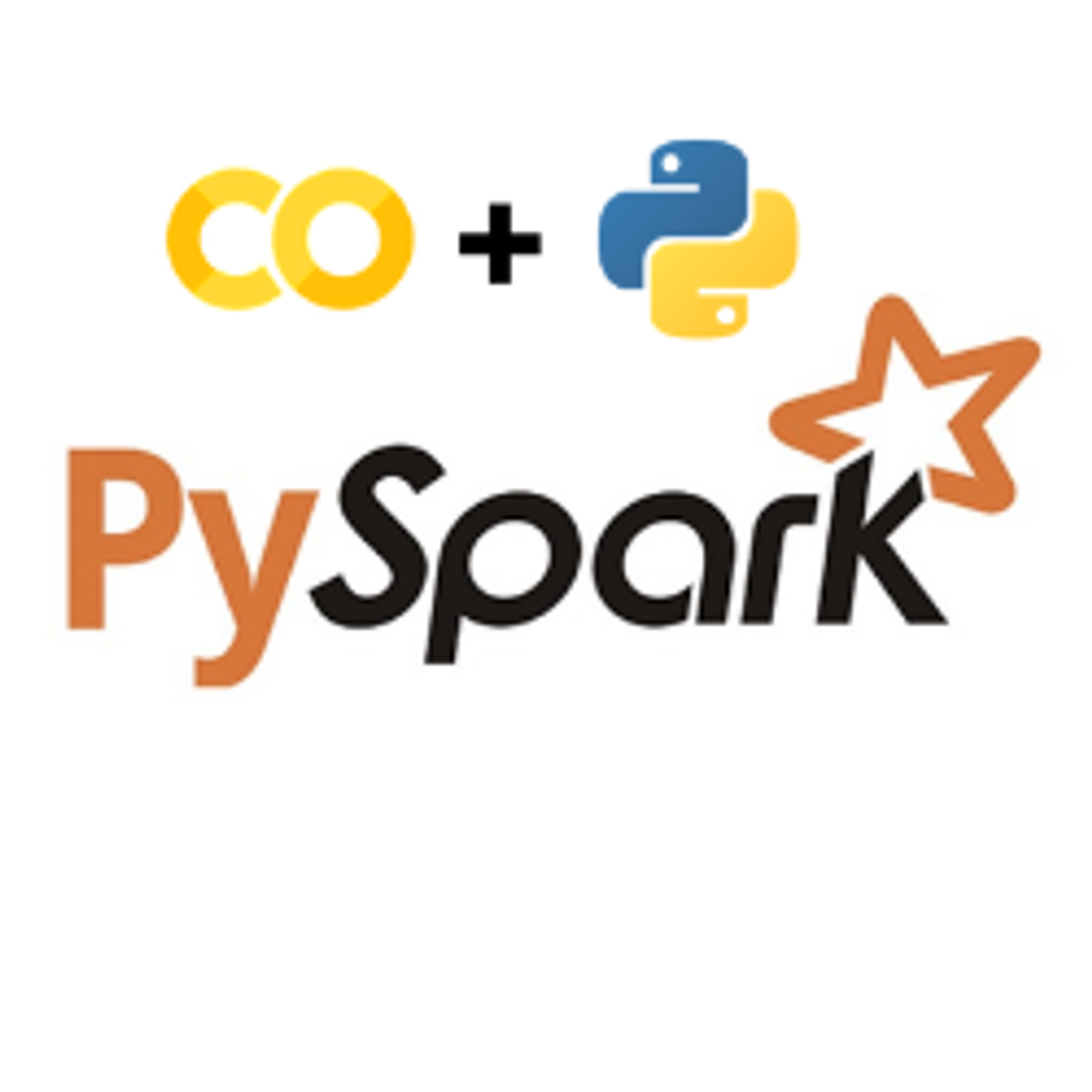
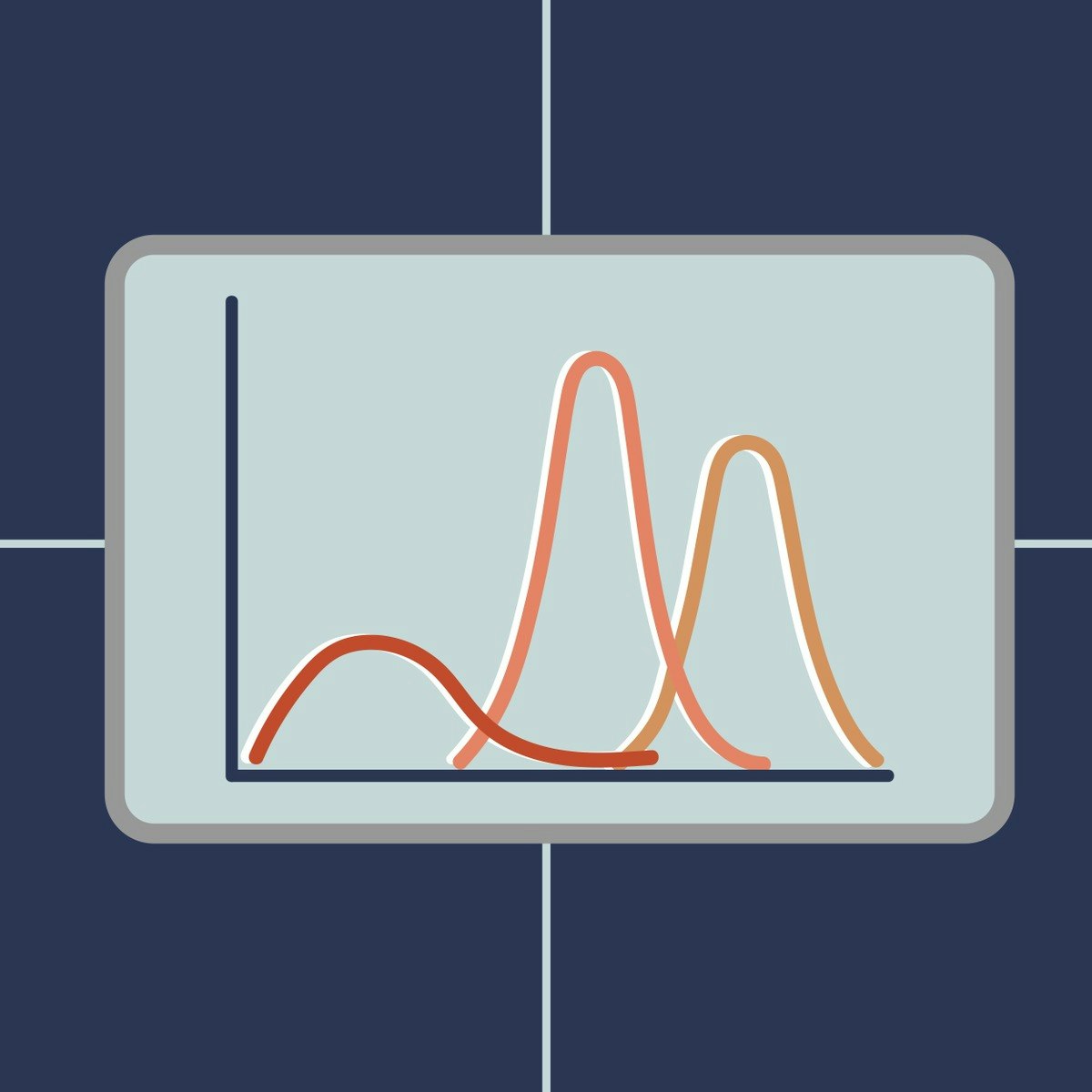
Data Science Courses - Page 83
Showing results 821-830 of 1407
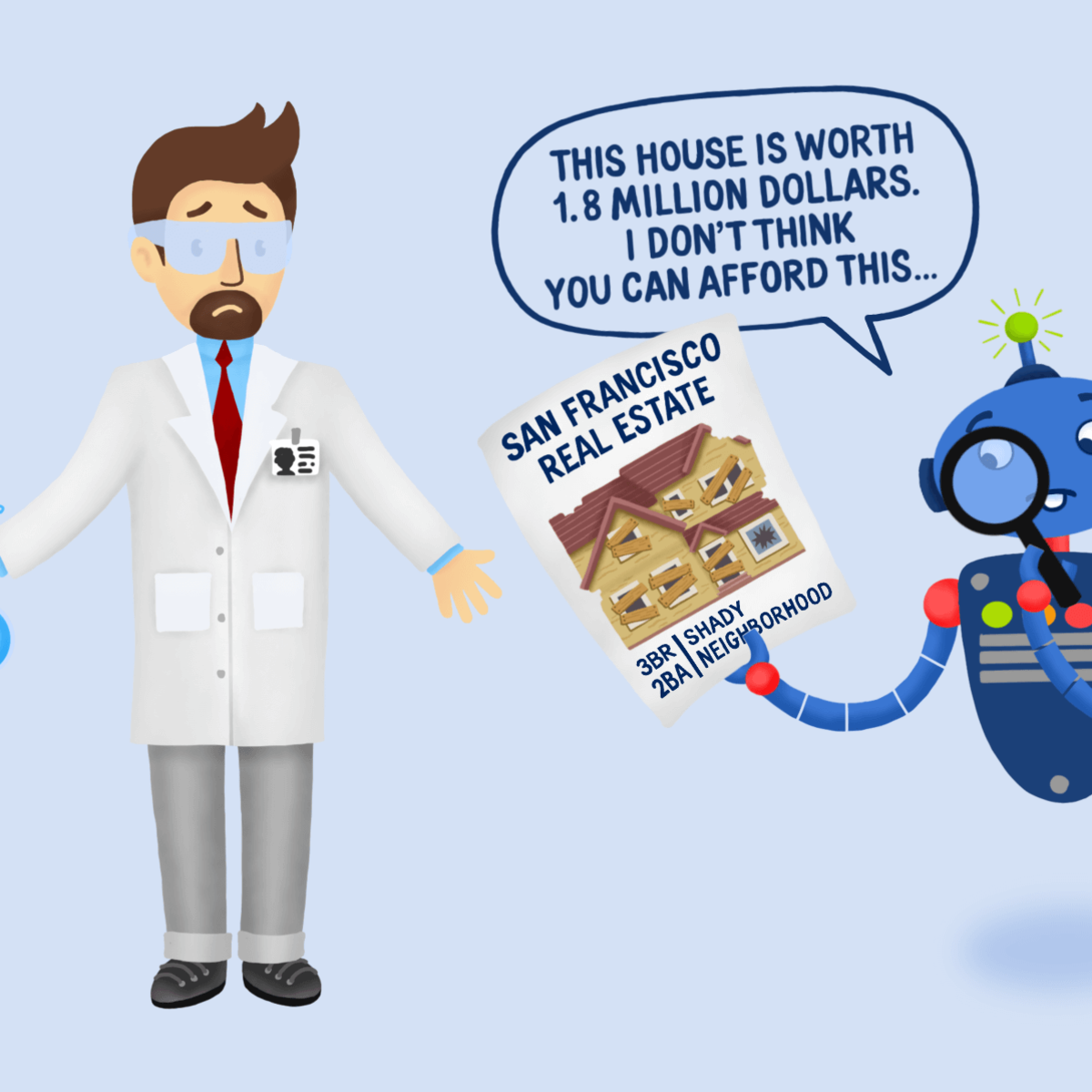
Predicting House Prices with Regression using TensorFlow
In this 2-hour long project-based course, you will learn the basics of using Keras with TensorFlow as its backend and you will learn to use it to solve a basic regression problem. By the end of this project, you will have created, trained, and evaluated a neural network model that, after the training, will be able to predict house prices with a high degree of accuracy.
Notes:
- This course works best for learners who are based in the North America region. We’re currently working on providing the same experience in other regions.
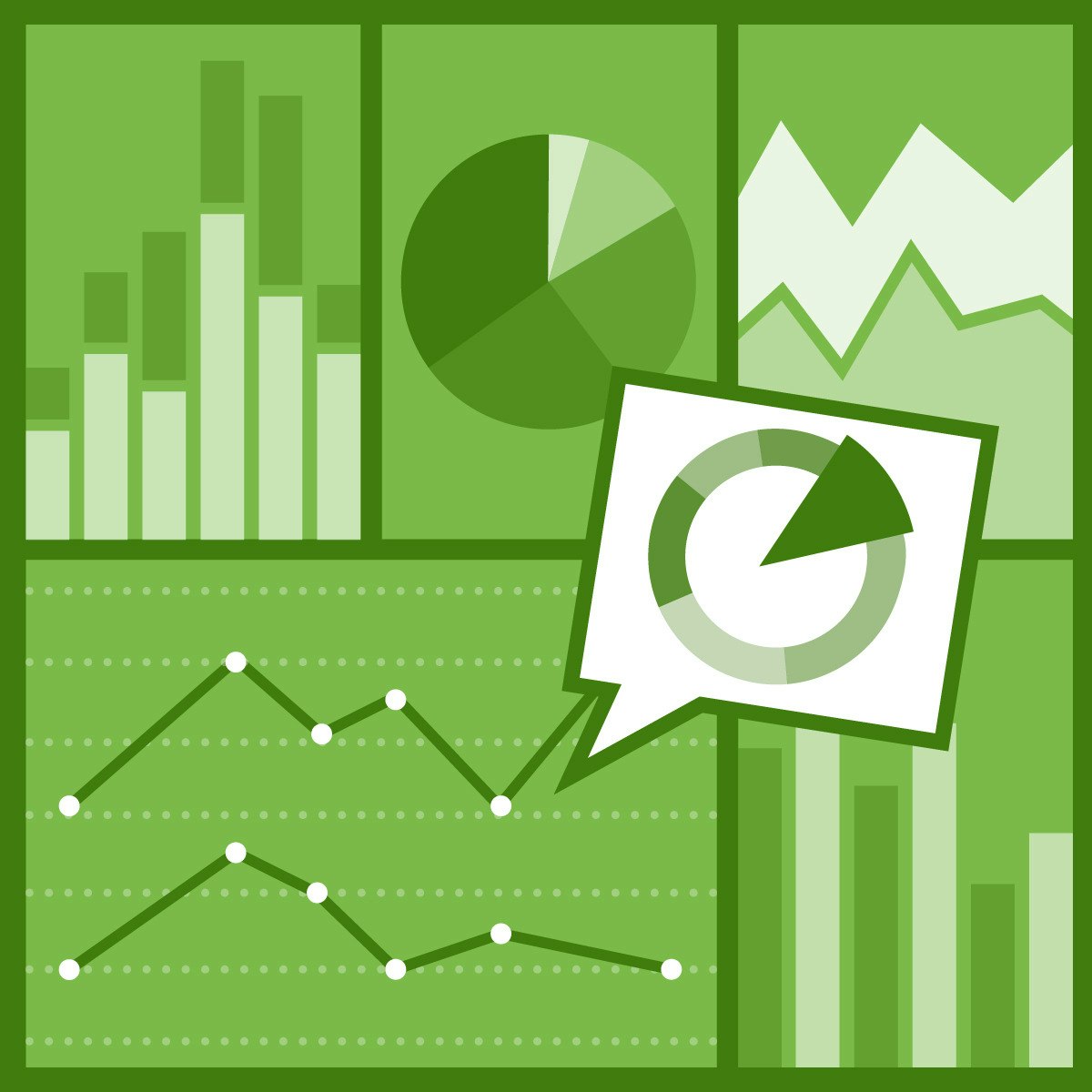
Data Visualization and Communication with Tableau
One of the skills that characterizes great business data analysts is the ability to communicate practical implications of quantitative analyses to any kind of audience member. Even the most sophisticated statistical analyses are not useful to a business if they do not lead to actionable advice, or if the answers to those business questions are not conveyed in a way that non-technical people can understand.
In this course you will learn how to become a master at communicating business-relevant implications of data analyses. By the end, you will know how to structure your data analysis projects to ensure the fruits of your hard labor yield results for your stakeholders. You will also know how to streamline your analyses and highlight their implications efficiently using visualizations in Tableau, the most popular visualization program in the business world. Using other Tableau features, you will be able to make effective visualizations that harness the human brain’s innate perceptual and cognitive tendencies to convey conclusions directly and clearly. Finally, you will be practiced in designing and persuasively presenting business “data stories” that use these visualizations, capitalizing on business-tested methods and design principles.
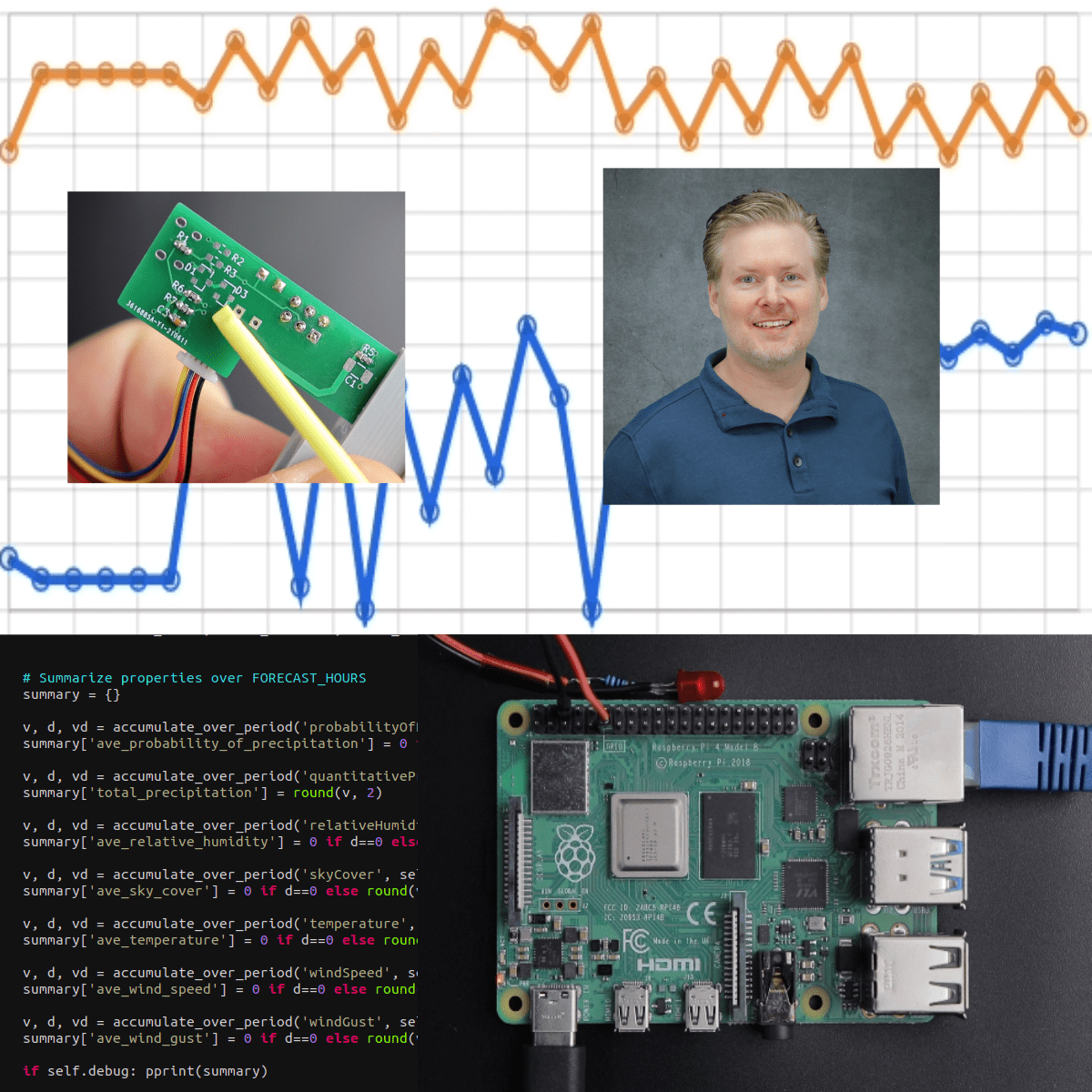
Using Sensors With Your Raspberry Pi
This course on integrating sensors with your Raspberry Pi is course 3 of a Coursera Specialization and can be taken separately or as part of the specialization. Although some material and explanations from the prior two courses are used, this course largely assumes no prior experience with sensors or data processing other than ideas about your own projects and an interest in building projects with sensors.
This course focuses on core concepts and techniques in designing and integrating any sensor, rather than overly specific examples to copy. This method allows you to use these concepts in your projects to build highly customized sensors for your applications.
Some of the ideas covered include calibrating sensors and the trade-offs between different mathematical methods of storing and applying calibration curves to your sensors. We also discuss accuracy, precision, and how to understand uncertainty in your measurements. We study methods of interfacing analog sensors with your Raspberry Pi (or other platform) with amplifiers and the theory and technique involved in reducing noise with spectral filters. Lastly, we borrow from the fields of data science, statistics, and digital signal processing, to post-process our data in Python.
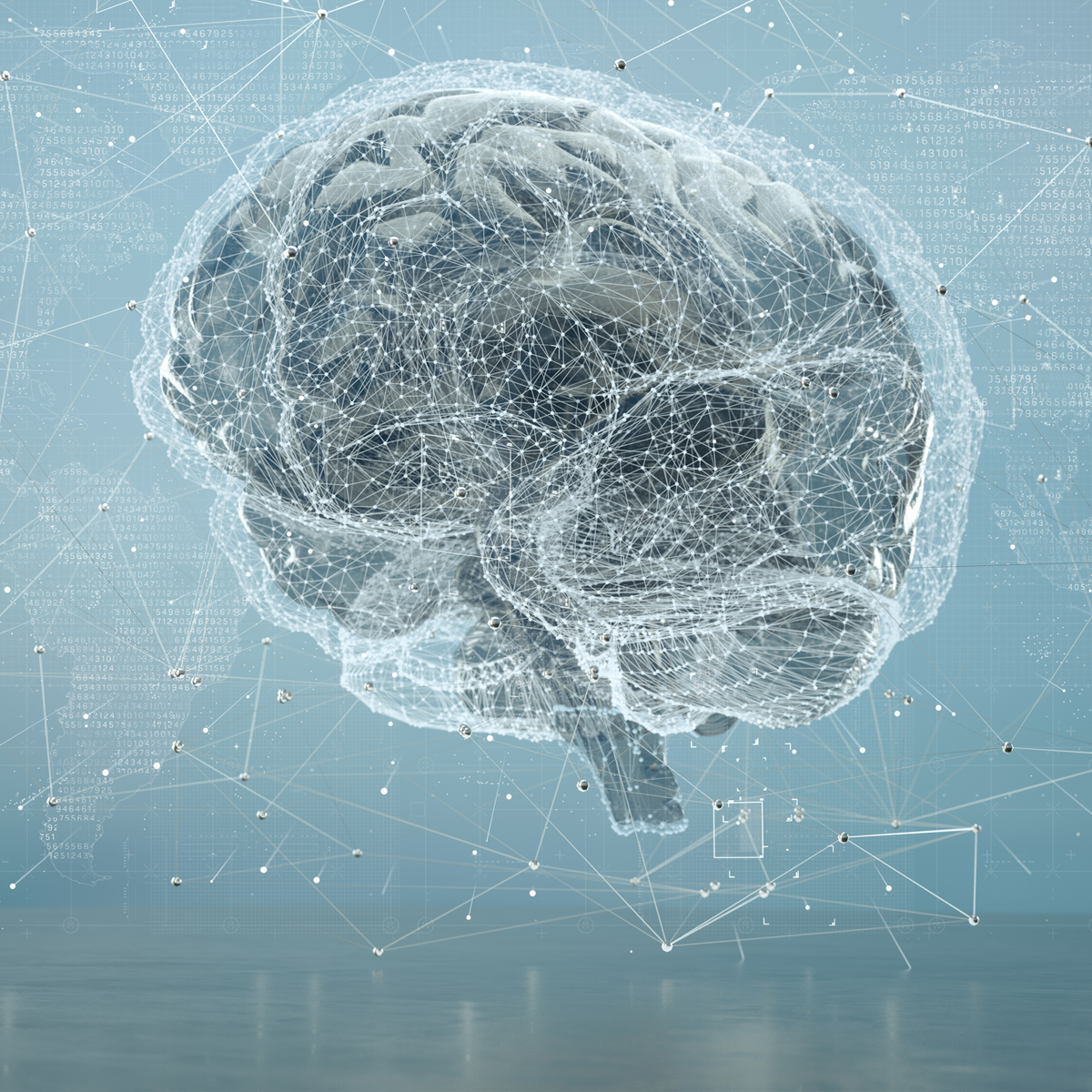
Big Data, Artificial Intelligence, and Ethics
This course gives you context and first-hand experience with the two major catalyzers of the computational science revolution: big data and artificial intelligence. With more than 99% of all mediated information in digital format and with 98% of the world population using digital technology, humanity produces an impressive digital footprint. In theory, this provides unprecedented opportunities to understand and shape society. In practice, the only way this information deluge can be processed is through using the same digital technologies that produced it. Data is the fuel, but machine learning it the motor to extract remarkable new knowledge from vasts amounts of data. Since an important part of this data is about ourselves, using algorithms in order to learn more about ourselves naturally leads to ethical questions. Therefore, we cannot finish this course without also talking about research ethics and about some of the old and new lines computational social scientists have to keep in mind. As hands-on labs, you will use IBM Watson’s artificial intelligence to extract the personality of people from their digital text traces, and you will experience the power and limitations of machine learning by teaching two teachable machines from Google yourself.
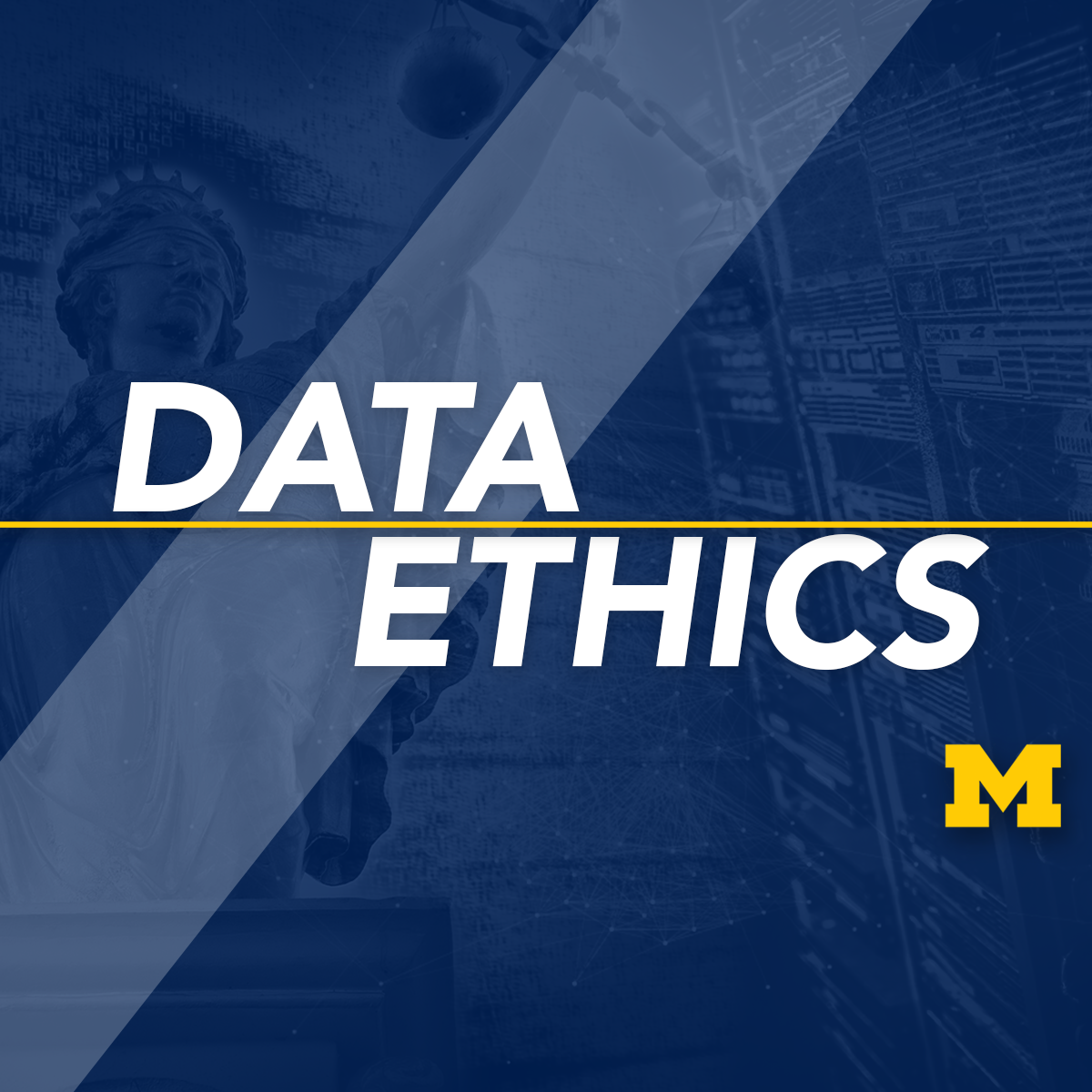
Data Science Ethics
What are the ethical considerations regarding the privacy and control of consumer information and big data, especially in the aftermath of recent large-scale data breaches?
This course provides a framework to analyze these concerns as you examine the ethical and privacy implications of collecting and managing big data. Explore the broader impact of the data science field on modern society and the principles of fairness, accountability and transparency as you gain a deeper understanding of the importance of a shared set of ethical values. You will examine the need for voluntary disclosure when leveraging metadata to inform basic algorithms and/or complex artificial intelligence systems while also learning best practices for responsible data management, understanding the significance of the Fair Information Practices Principles Act and the laws concerning the "right to be forgotten."
This course will help you answer questions such as who owns data, how do we value privacy, how to receive informed consent and what it means to be fair.
Data scientists and anyone beginning to use or expand their use of data will benefit from this course. No particular previous knowledge needed.
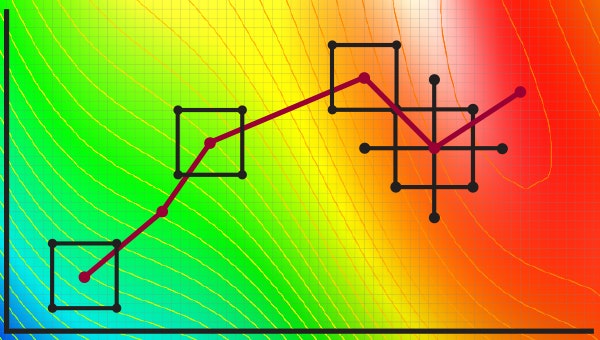
Experimentation for Improvement
We are always using experiments to improve our lives, our community, and our work. Are you doing it efficiently? Or are you (incorrectly) changing one thing at a time and hoping for the best?
In this course, you will learn how to plan efficient experiments - testing with many variables. Our goal is to find the best results using only a few experiments. A key part of the course is how to optimize a system.
We use simple tools: starting with fast calculations by hand, then we show how to use FREE software.
The course comes with slides, transcripts of all lectures, subtitles (English, Spanish and Portuguese; some Chinese and French), videos, audio files, source code, and a free textbook. You get to keep all of it, all freely downloadable.
This course is for anyone working in a company, or wanting to make changes to their life, their community, their neighbourhood. You don't need to be a statistician or scientist! There's something for everyone in here.
⎯⎯⎯⎯⎯⎯⎯⎯⎯⎯⎯⎯⎯⎯⎯
Over 1500 people have completed this online course. What have prior students said about this course?
"This definitely is one of the most fruitful courses I have participated at Coursera, considering the takeaways and implementations! And so far I finished 12 [courses]."
"Excelente curso, flexible y con suficiente material didáctico fácilmente digerible y cómodo. No importa si se tiene pocas bases matemáticas o estadísticas, el curso proporciona casi toda explicación necesaria para un entendimiento alto."
"I wish I had enrolled in your course years ago -- it would have saved us a lot of time in optimizing experimental conditions." Jason Eriksen, 3 Jan 2017
"Interesting and developing both analytical and creative thinking. The lecturer took care to bring lots of real live examples which are fun to analyze." 20 February 2016.
"... love your style of presentation, and the examples you took from everyday life to explain things. It is very difficult to make such a mathematical course accessible and comprehensible to this wide a variety of people!"
⎯⎯⎯⎯⎯⎯⎯⎯⎯⎯⎯⎯⎯⎯⎯
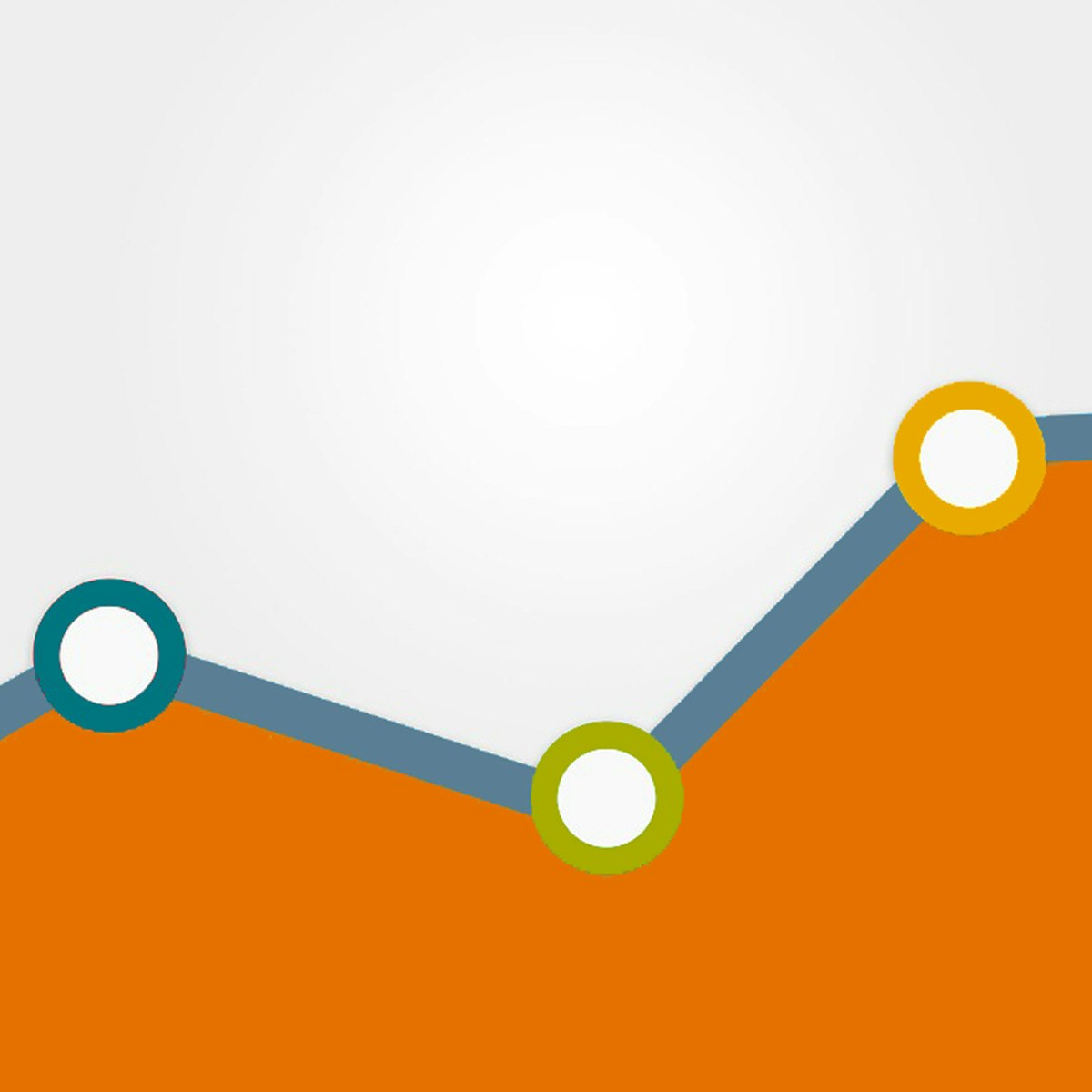
Marketing Analytics
Organizations large and small are inundated with data about consumer choices. But that wealth of information does not always translate into better decisions. Knowing how to interpret data is the challenge -- and marketers in particular are increasingly expected to use analytics to inform and justify their decisions.
Marketing analytics enables marketers to measure, manage and analyze marketing performance to maximize its effectiveness and optimize return on investment (ROI). Beyond the obvious sales and lead generation applications, marketing analytics can offer profound insights into customer preferences and trends, which can be further utilized for future marketing and business decisions.
This course, developed at the Darden School of Business at the University of Virginia, gives you the tools to measure brand and customer assets, understand regression analysis, and design experiments as a way to evaluate and optimize marketing campaigns. You'll leave the course with a solid understanding of how to use marketing analytics to predict outcomes and systematically allocate resources.
You can follow my posts in Twitter, @rajkumarvenk, and on linkedin: https://www.linkedin.com/in/education-marketing.
Thanks,
Raj
Professor of Business Administration at Darden
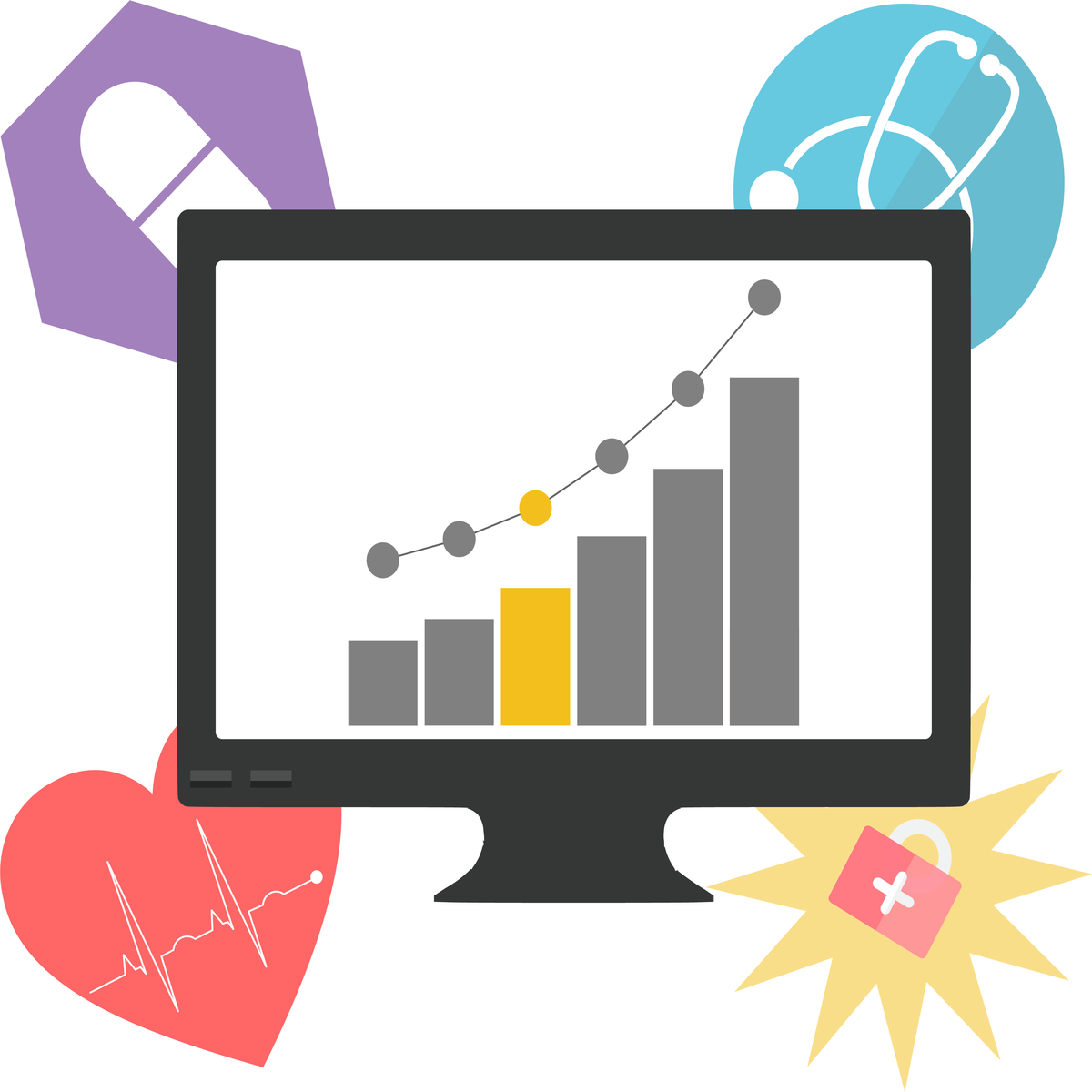
Identifying Patient Populations
This course teaches you the fundamentals of computational phenotyping, a biomedical informatics method for identifying patient populations. In this course you will learn how different clinical data types perform when trying to identify patients with a particular disease or trait. You will also learn how to program different data manipulations and combinations to increase the complexity and improve the performance of your algorithms. Finally, you will have a chance to put your skills to the test with a real-world practical application where you develop a computational phenotyping algorithm to identify patients who have hypertension. You will complete this work using a real clinical data set while using a free, online computational environment for data science hosted by our Industry Partner Google Cloud.
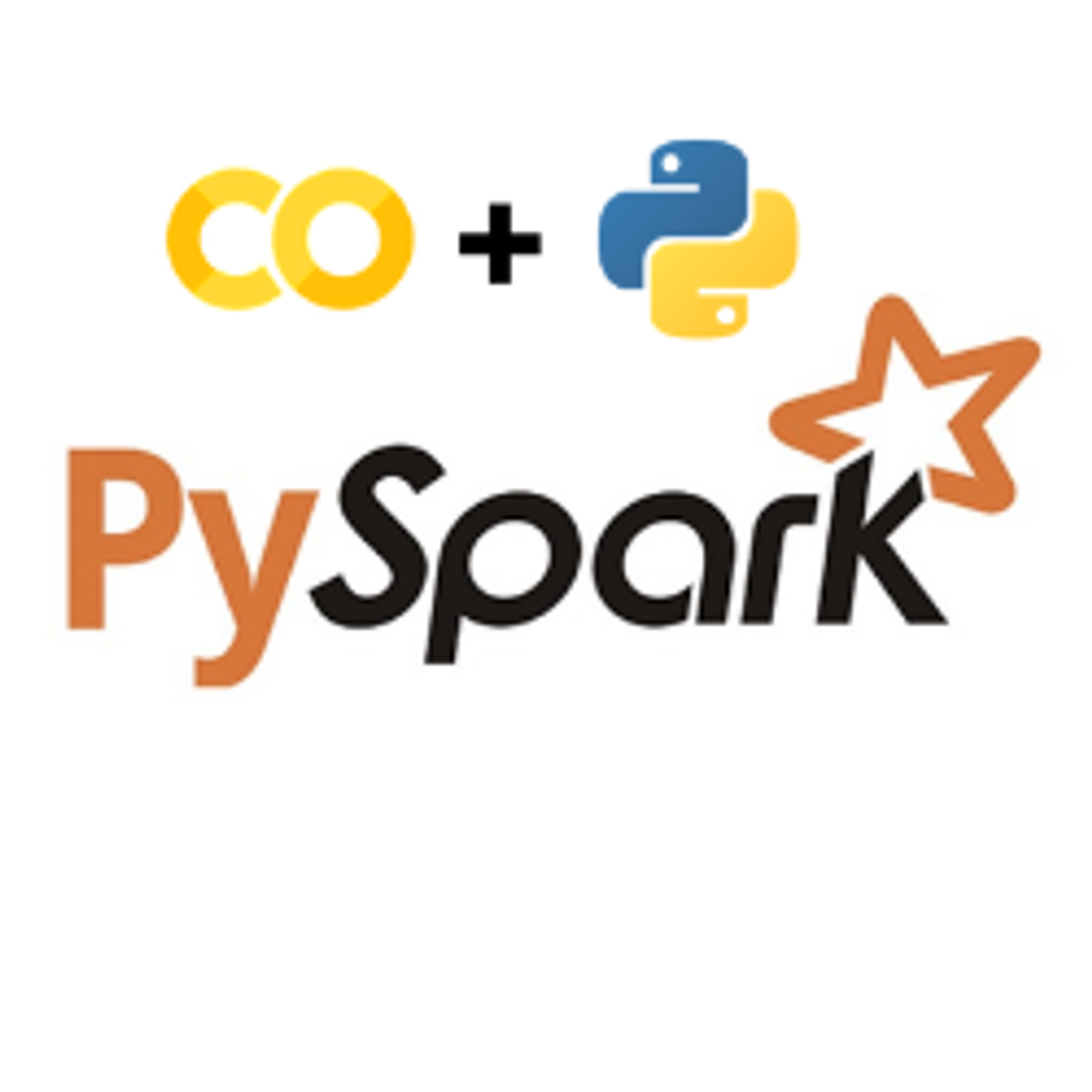
Graduate Admission Prediction with Pyspark ML
In this 1 hour long project-based course, you will learn to build a linear regression model using Pyspark ML to predict students' admission at the university. We will use the graduate admission 2 data set from Kaggle. Our goal is to use a Simple Linear Regression Machine Learning Algorithm from the Pyspark Machine learning library to predict the chances of getting admission. We will be carrying out the entire project on the Google Colab environment with the installation of Pyspark. You will need a free Gmail account to complete this project. Please be aware of the fact that the dataset and the model in this project, can not be used in the real-life. We are only using this data for the learning purposes.
By the end of this project, you will be able to build the linear regression model using Pyspark ML to predict admission chances.You will also be able to setup and work with Pyspark on the Google Colab environment. Additionally, you will also be able to clean and prepare data for analysis.
You should be familiar with the Python Programming language and you should have a theoretical understanding of Linear Regression algorithm.
Note: This course works best for learners who are based in the North America region. We’re currently working on providing the same experience in other regions.
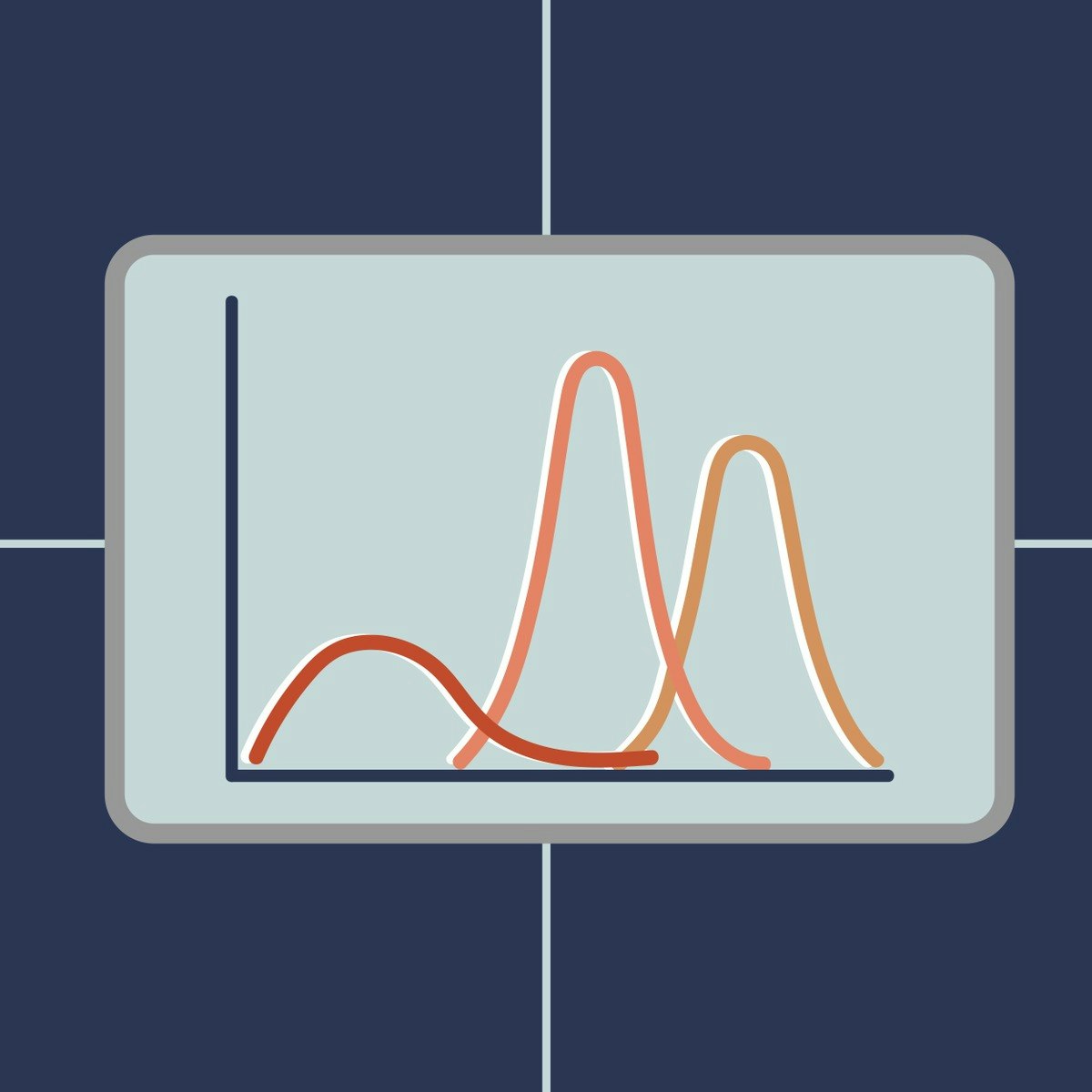
Bayesian Statistics: From Concept to Data Analysis
This course introduces the Bayesian approach to statistics, starting with the concept of probability and moving to the analysis of data. We will learn about the philosophy of the Bayesian approach as well as how to implement it for common types of data. We will compare the Bayesian approach to the more commonly-taught Frequentist approach, and see some of the benefits of the Bayesian approach. In particular, the Bayesian approach allows for better accounting of uncertainty, results that have more intuitive and interpretable meaning, and more explicit statements of assumptions. This course combines lecture videos, computer demonstrations, readings, exercises, and discussion boards to create an active learning experience. For computing, you have the choice of using Microsoft Excel or the open-source, freely available statistical package R, with equivalent content for both options. The lectures provide some of the basic mathematical development as well as explanations of philosophy and interpretation. Completion of this course will give you an understanding of the concepts of the Bayesian approach, understanding the key differences between Bayesian and Frequentist approaches, and the ability to do basic data analyses.
Popular Internships and Jobs by Categories
Find Jobs & Internships
Browse
© 2024 BoostGrad | All rights reserved