Back to Courses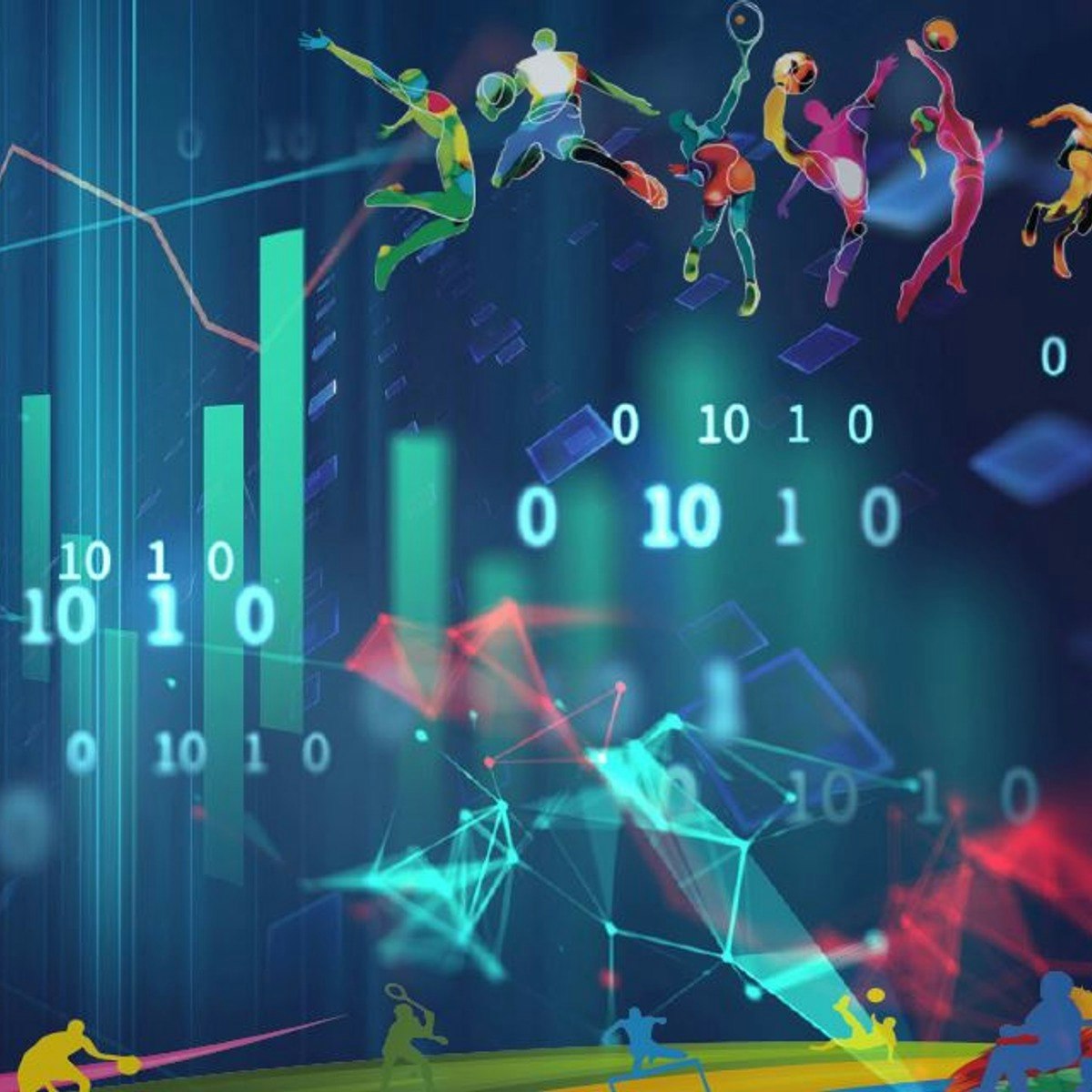
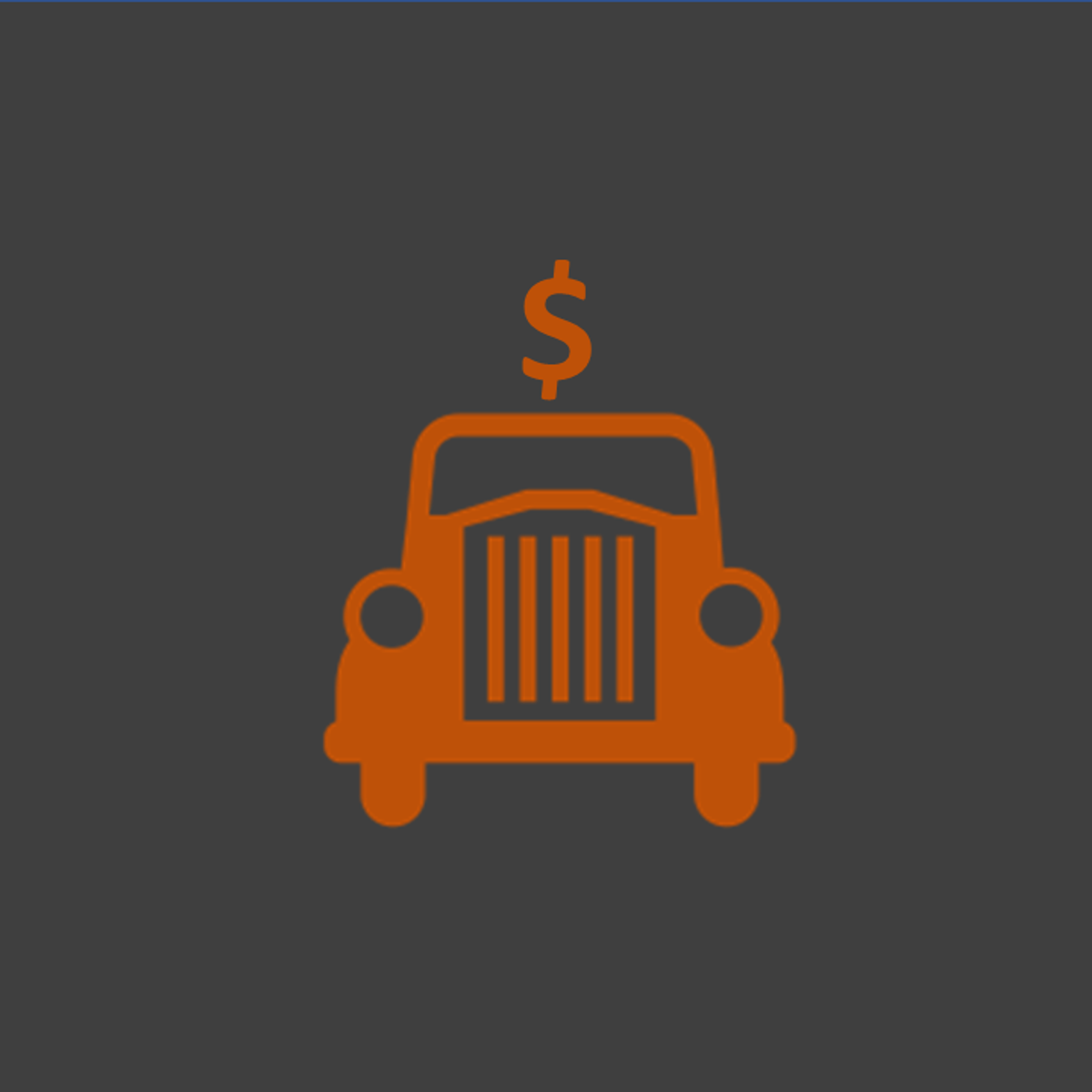
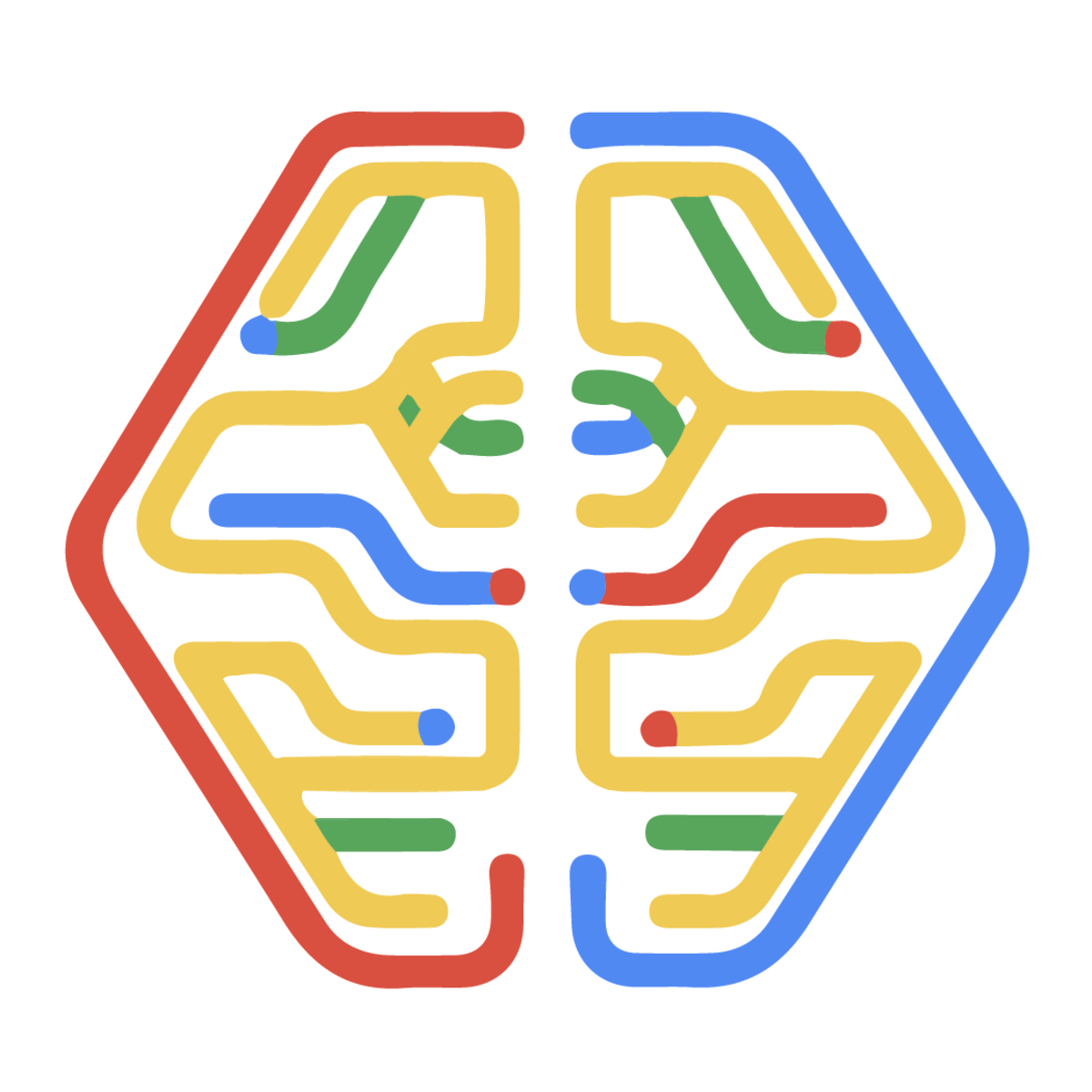
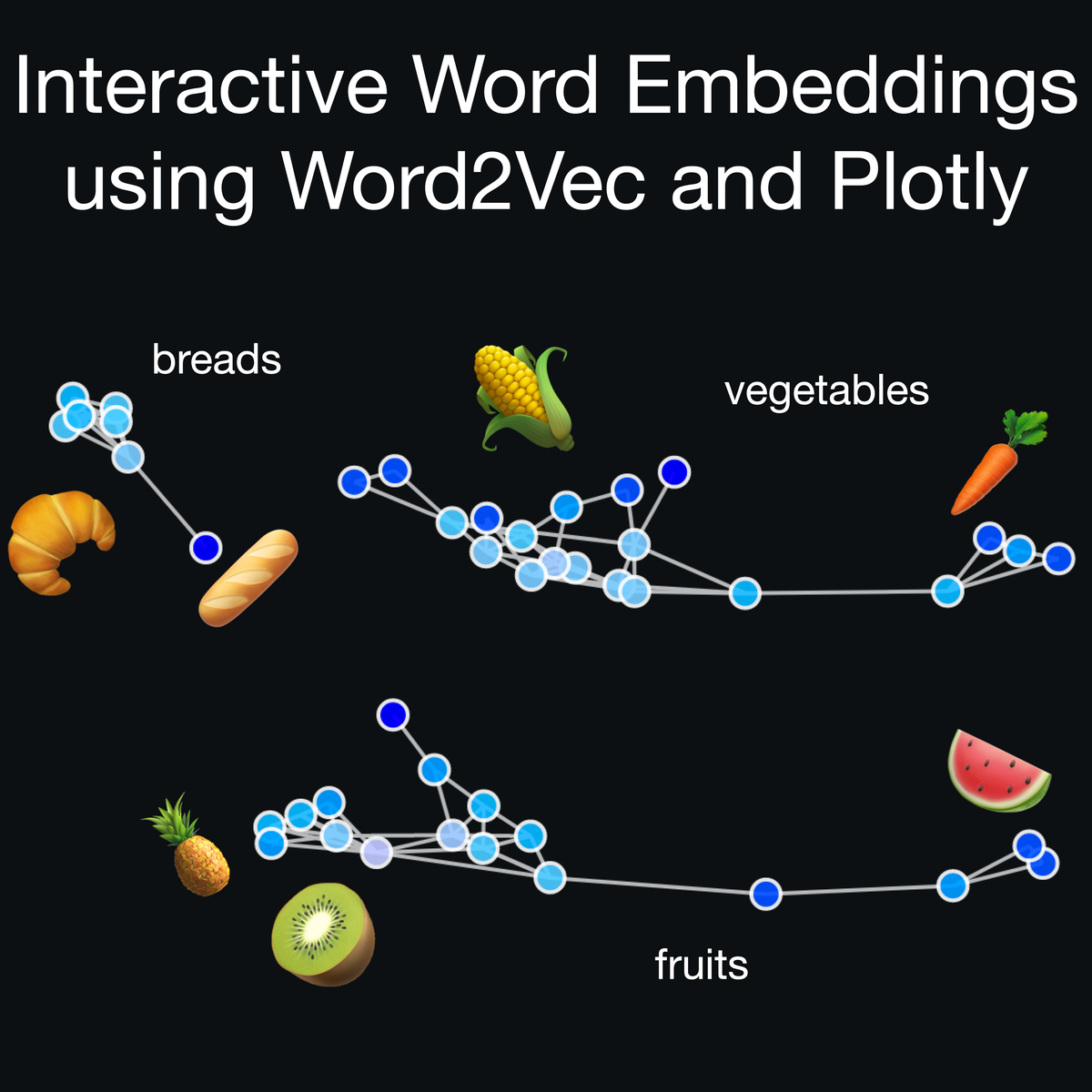
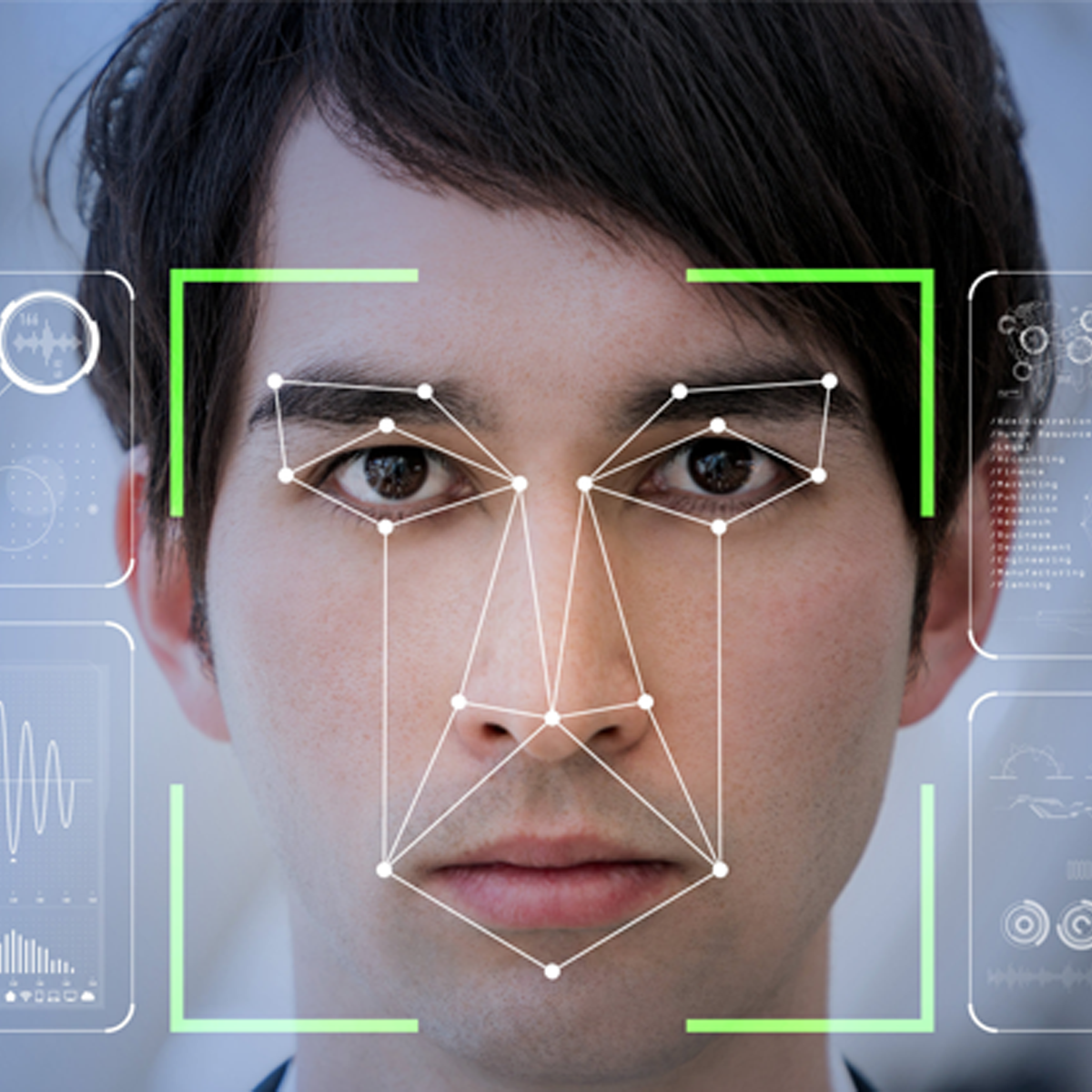
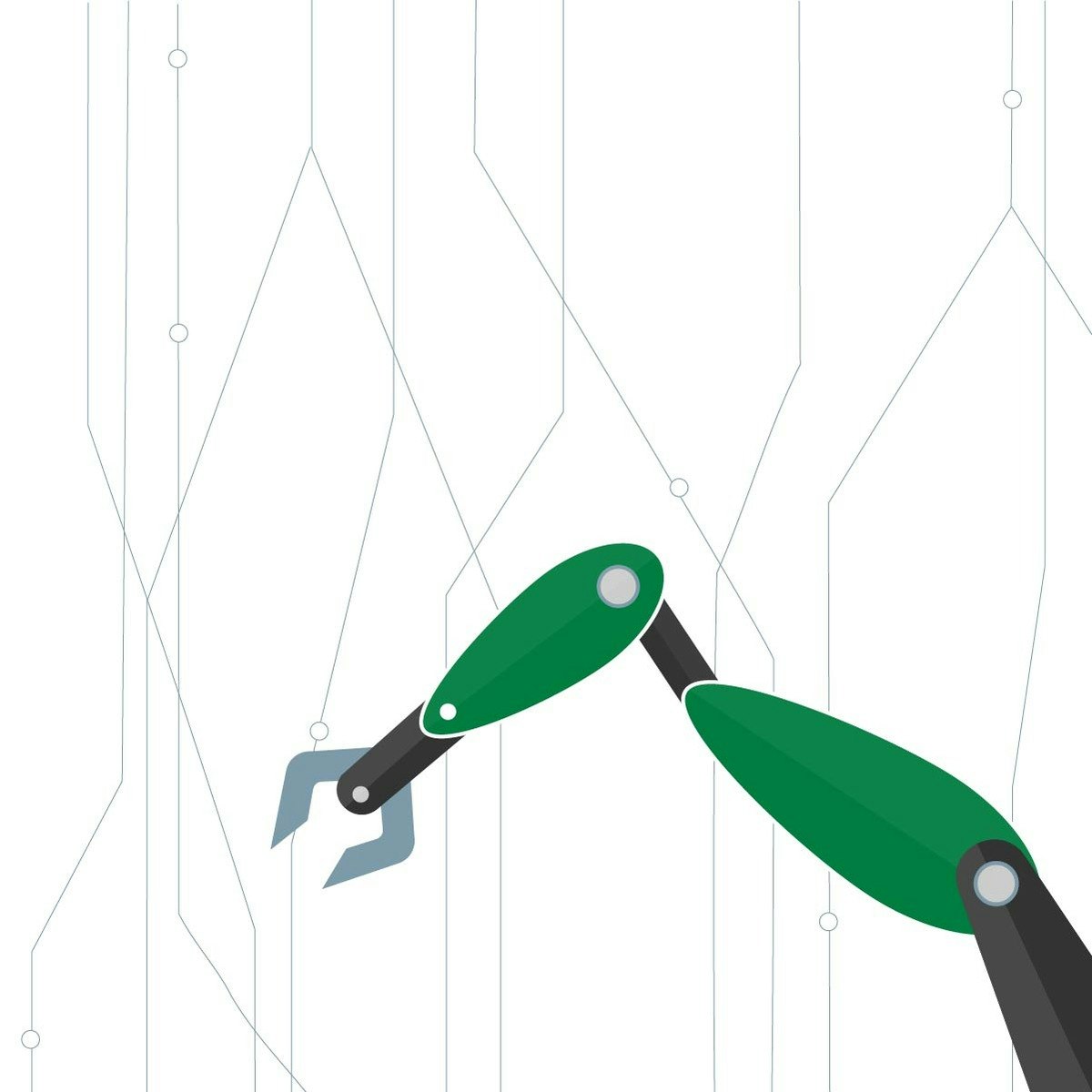
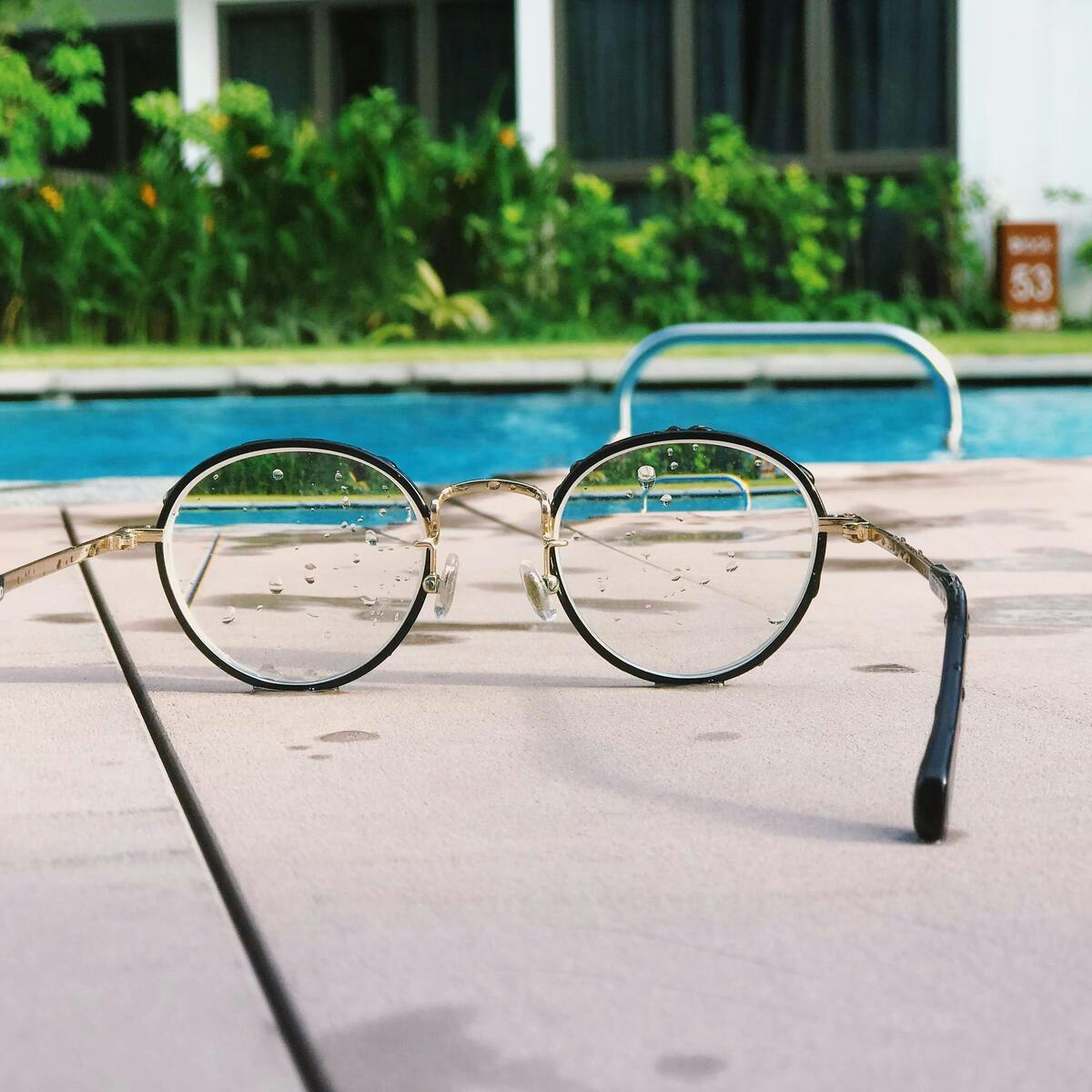
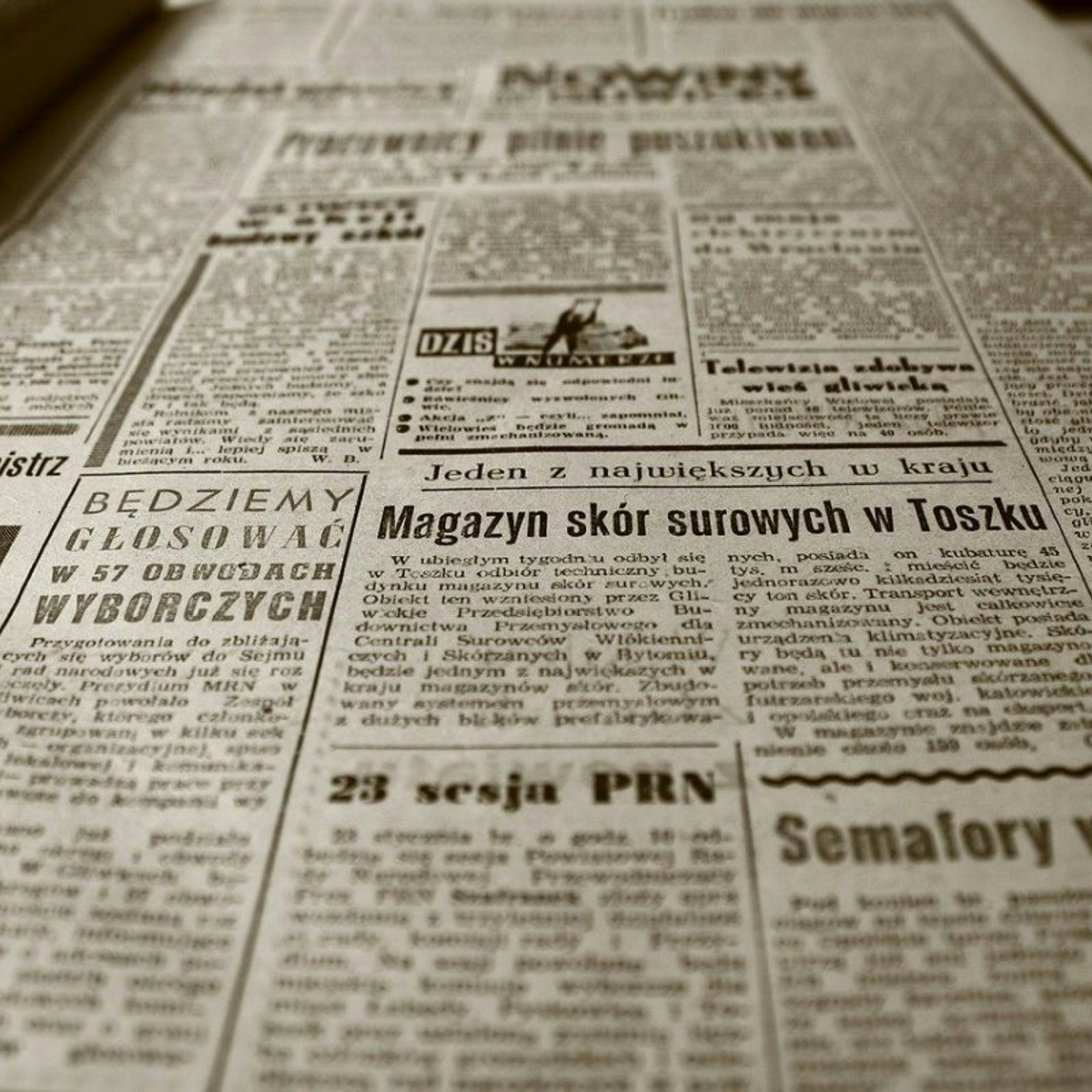
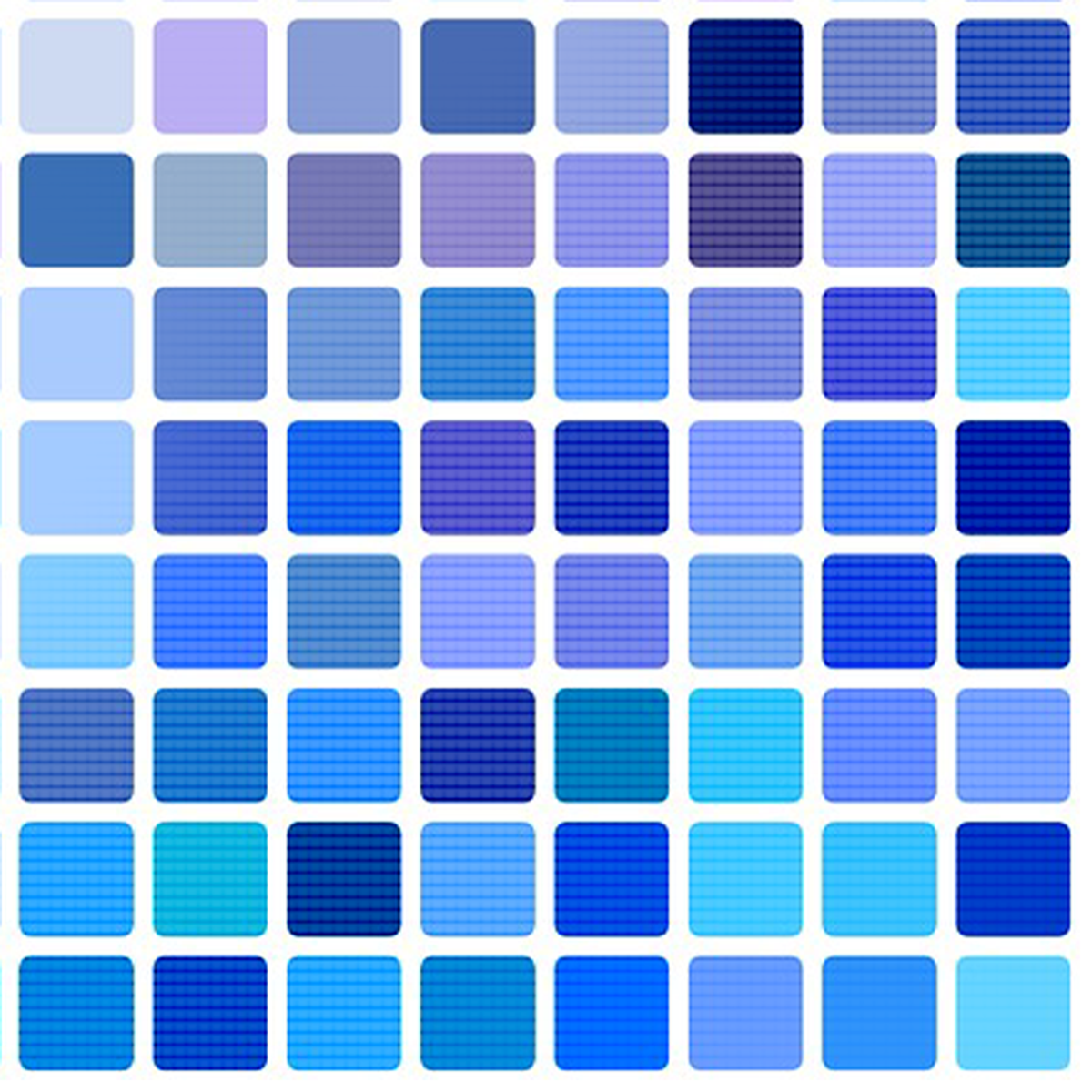
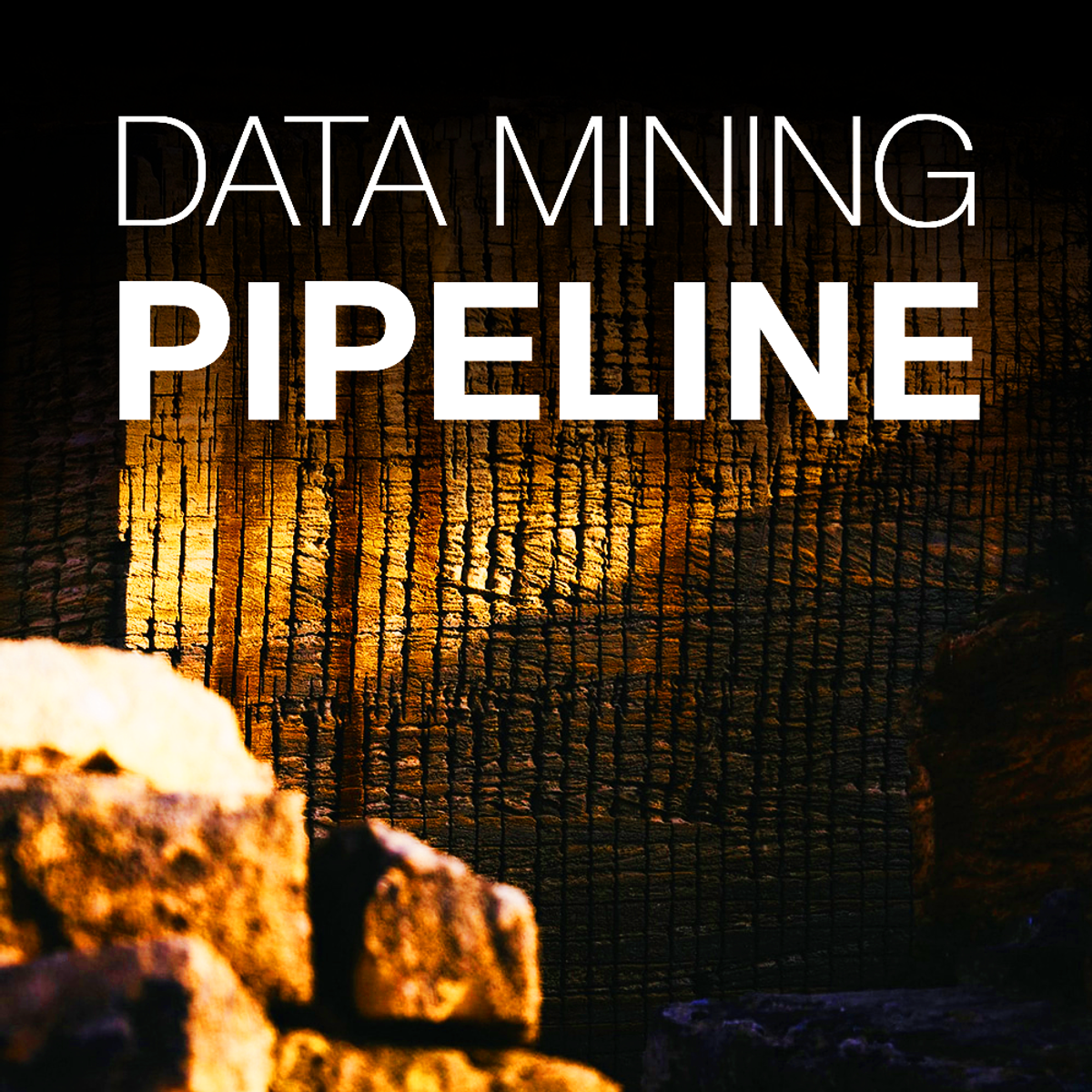
Data Science Courses - Page 22
Showing results 211-220 of 1407
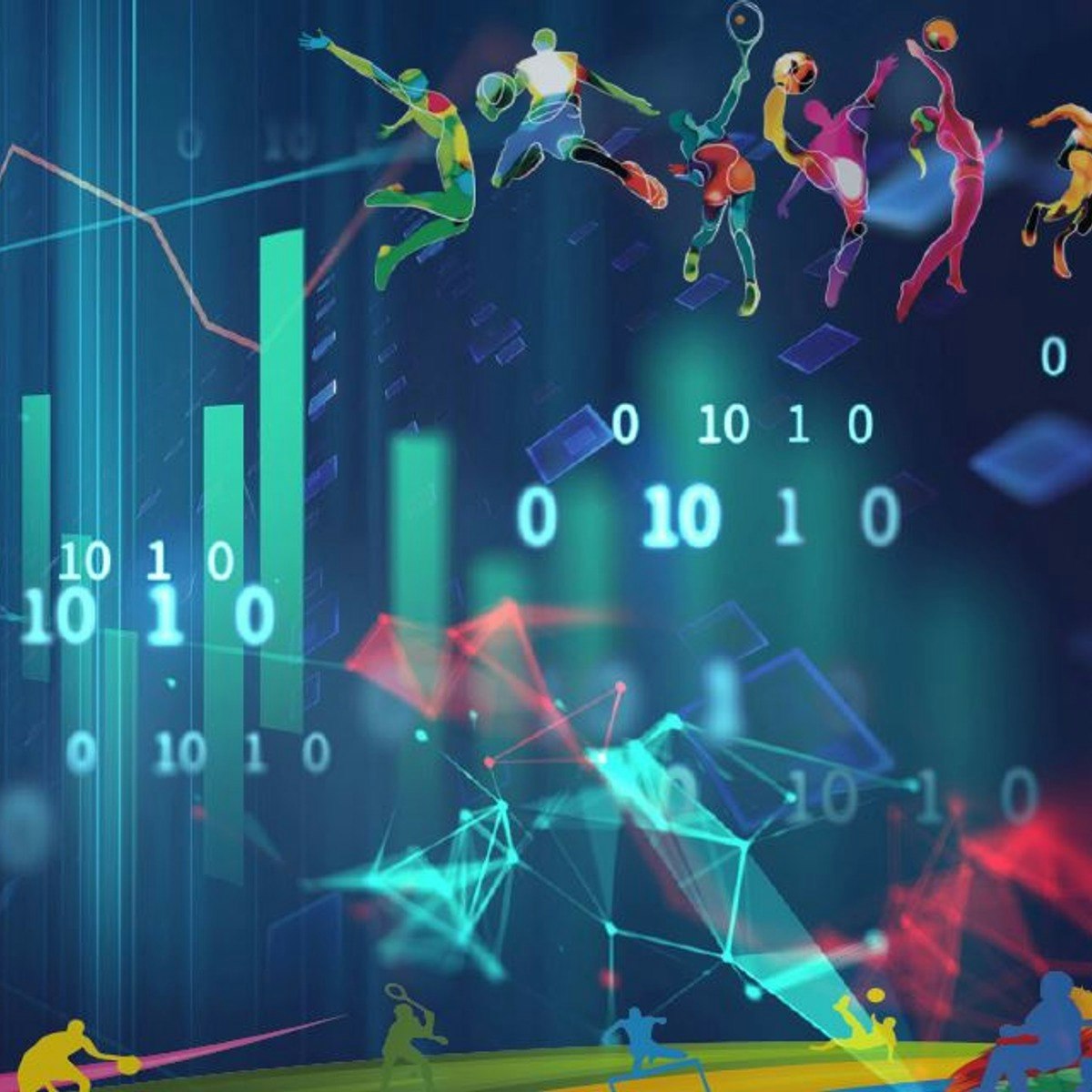
Data Analytics in Sports Law and Team Management
This course provides an introduction to the fundamental ideas in applying data analytics to issues surrounding key regulatory and management functions within the sports industry. Sports as an industry is increasingly relying on data analytics for more effective deployment of resources and assessment of performance in areas ranging from player productivity to fan engagement, talent identification and development, coaching, sponsorship, and marketing. This course and its successors will help you understand this new role of data analysis in the sports business.
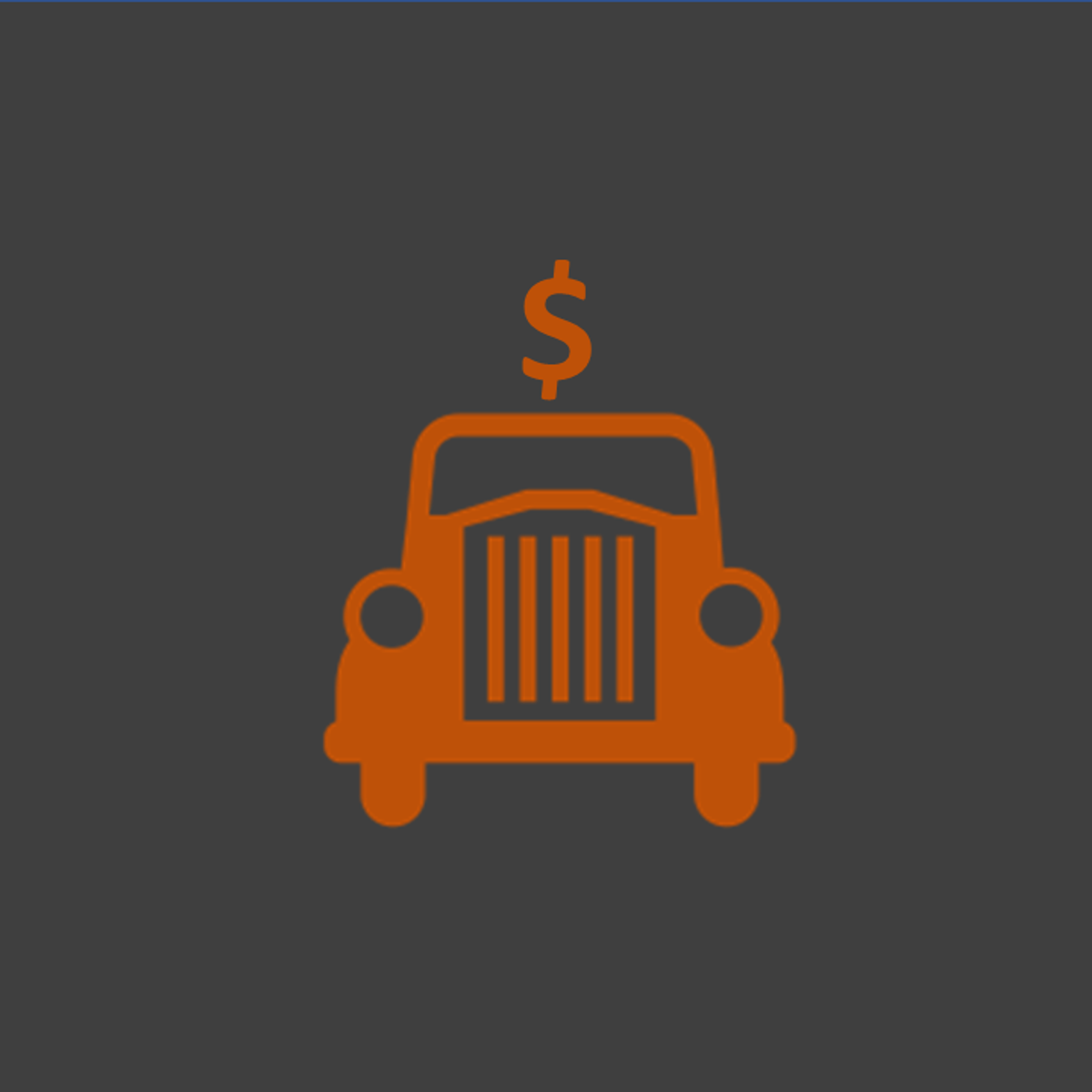
XG-Boost 101: Used Cars Price Prediction
In this hands-on project, we will train 3 Machine Learning algorithms namely Multiple Linear Regression, Random Forest Regression, and XG-Boost to predict used cars prices. This project can be used by car dealerships to predict used car prices and understand the key factors that contribute to used car prices.
By the end of this project, you will be able to:
- Understand the applications of Artificial Intelligence and Machine Learning techniques in the banking industry
- Understand the theory and intuition behind XG-Boost Algorithm
- Import key Python libraries, dataset, and perform Exploratory Data Analysis.
- Perform data visualization using Seaborn, Plotly and Word Cloud.
- Standardize the data and split them into train and test datasets.
- Build, train and evaluate XG-Boost, Random Forest, Decision Tree, and Multiple Linear Regression Models Using Scikit-Learn.
- Assess the performance of regression models using various Key Performance Indicators (KPIs).
Note: This course works best for learners who are based in the North America region. We’re currently working on providing the same experience in other regions.
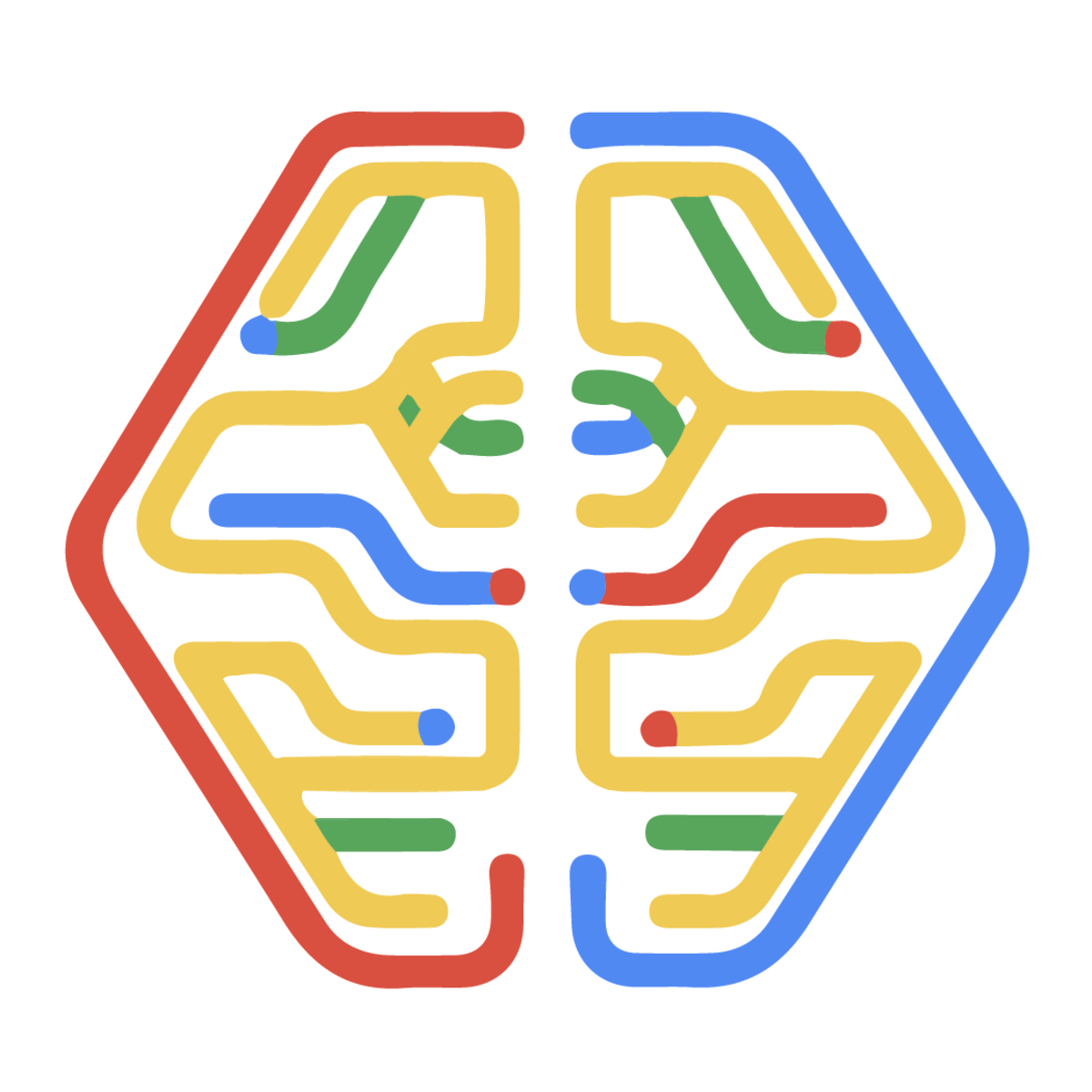
Production Machine Learning Systems
This course covers how to implement the various flavors of production ML systems— static, dynamic, and continuous training; static and dynamic inference; and batch and online processing. You delve into TensorFlow abstraction levels, the various options for doing distributed training, and how to write distributed training models with custom estimators.
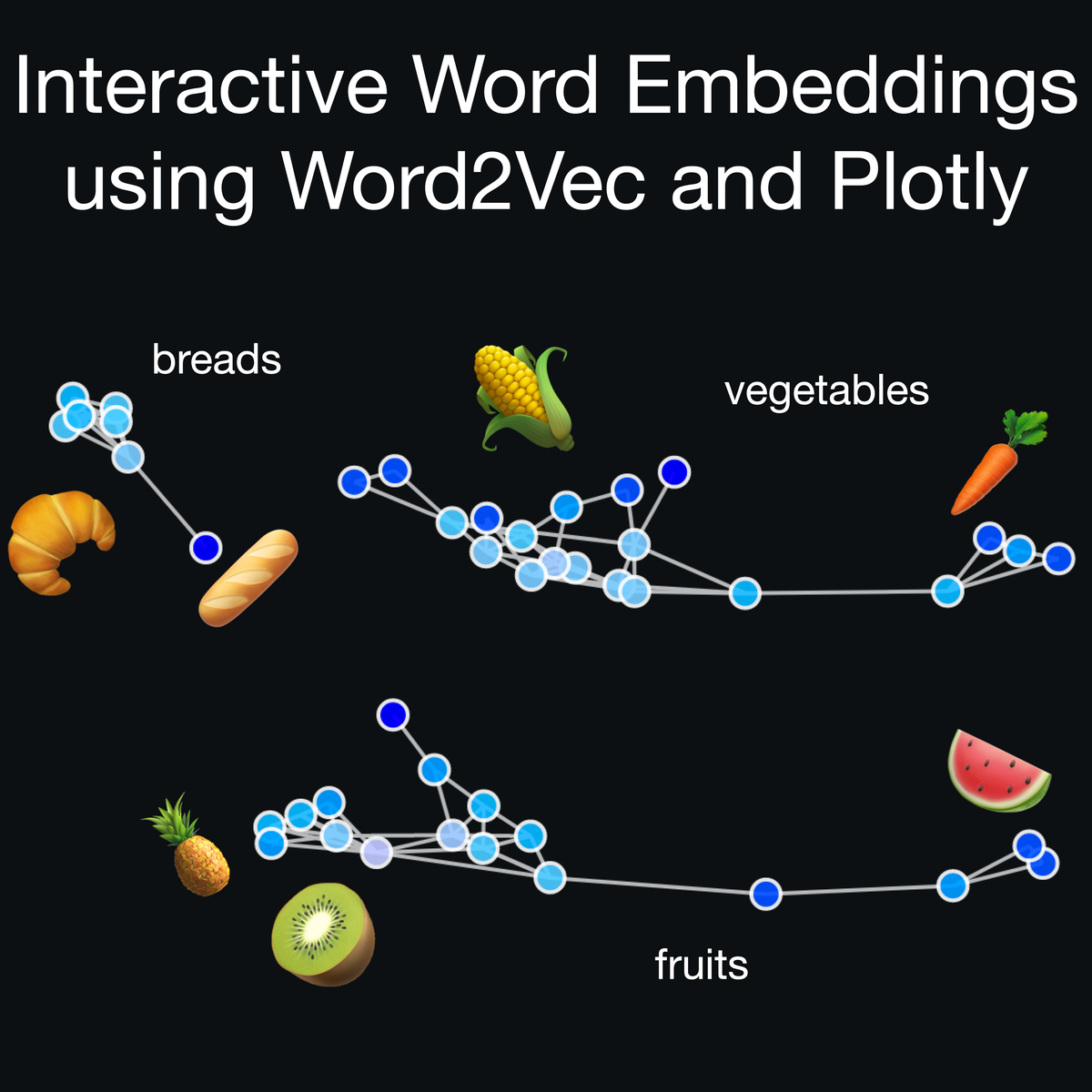
Interactive Word Embeddings using Word2Vec and Plotly
In this 2 hour long project, you will learn how to preprocess a text dataset comprising recipes. You will learn how to use natural language processing techniques to generate word embeddings for these ingredients, using Word2Vec. These word embeddings can be used for recommendations in an online store based on added items in a basket, or to suggest alternative items as replacements when stock is limited. You will build this recommendation/discovery feature in an interactive and aesthetic visualization tool.
Note: This course works best for learners who are based in the North America region. We’re currently working on providing the same experience in other regions.
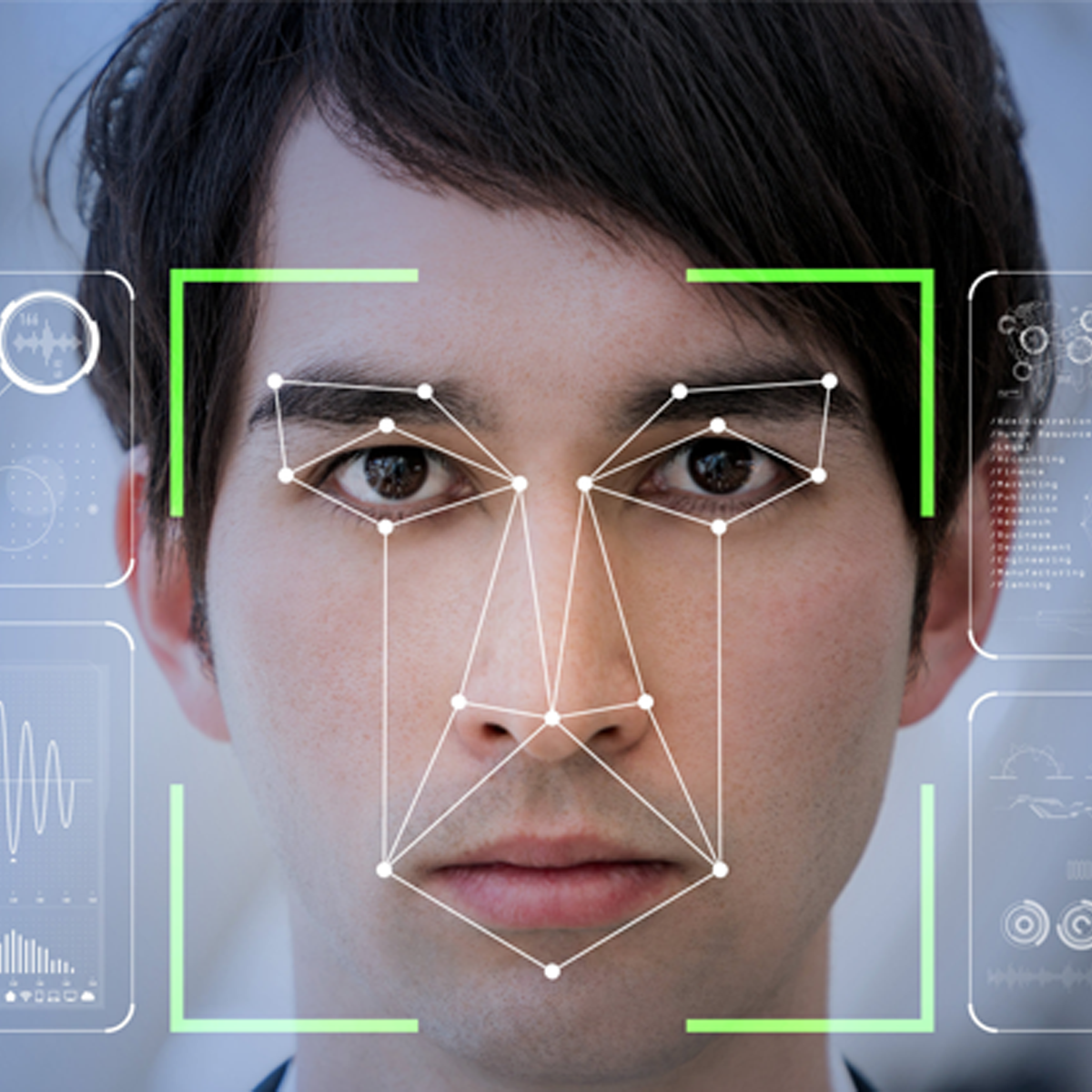
Recognizing Facials and Objects with Amazon Rekognition
In this two hours project, you understand how Amazon Rekognition works and will learn how to use the AWS SDK to Analyze Faces, detect objects and labels in image scenes, moderate images, identify celebrities and recognize and compare faces using Artificial Intelligence.
Amazon Rekognition is one of the most used Artificial Intelligence services in AWS and popular to analyze images with huge confidence and low costs.
Once you're done with this project, you will be able to use Amazon Rekognition to analyze your own images in just a few steps.
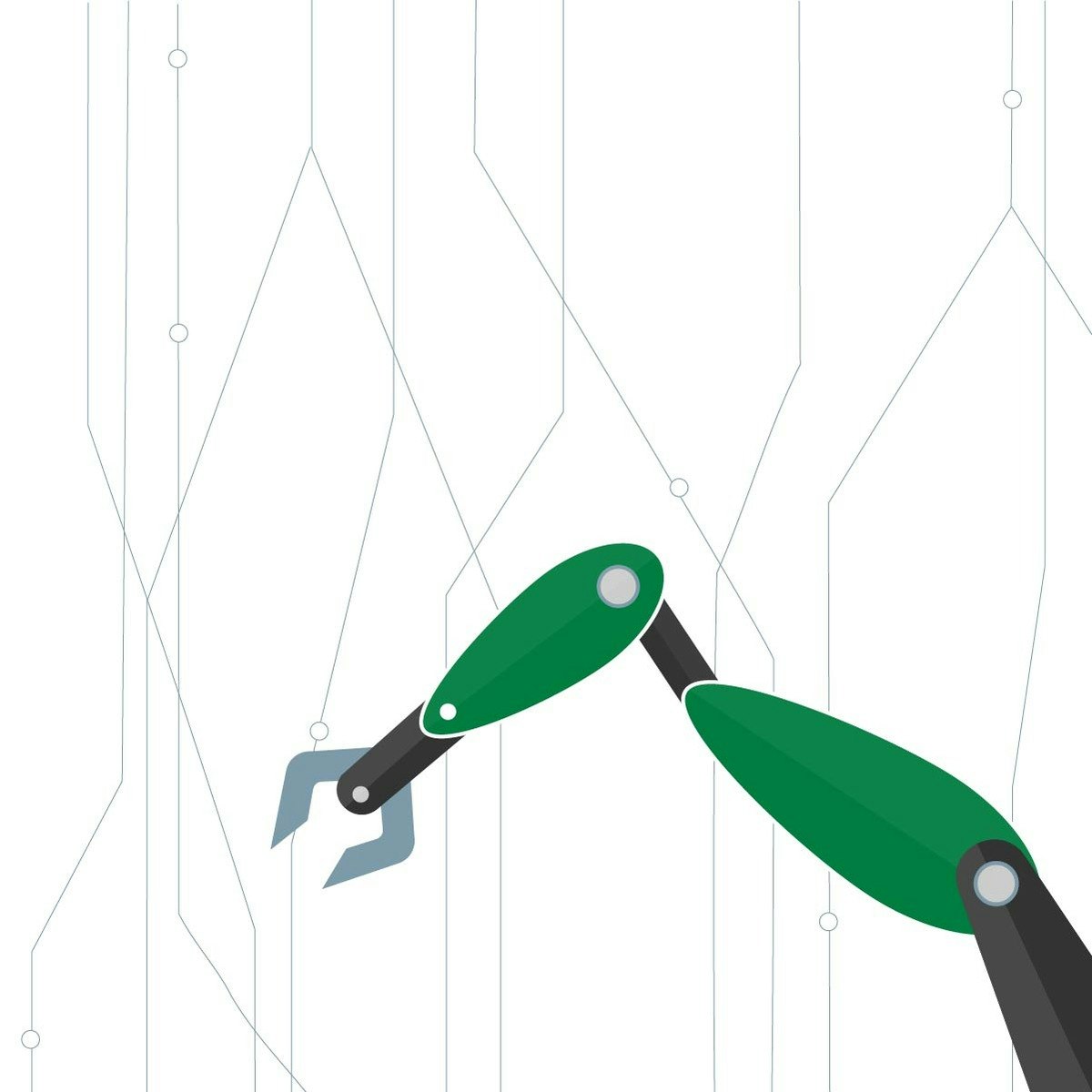
Prediction and Control with Function Approximation
In this course, you will learn how to solve problems with large, high-dimensional, and potentially infinite state spaces. You will see that estimating value functions can be cast as a supervised learning problem---function approximation---allowing you to build agents that carefully balance generalization and discrimination in order to maximize reward. We will begin this journey by investigating how our policy evaluation or prediction methods like Monte Carlo and TD can be extended to the function approximation setting. You will learn about feature construction techniques for RL, and representation learning via neural networks and backprop. We conclude this course with a deep-dive into policy gradient methods; a way to learn policies directly without learning a value function. In this course you will solve two continuous-state control tasks and investigate the benefits of policy gradient methods in a continuous-action environment.
Prerequisites: This course strongly builds on the fundamentals of Courses 1 and 2, and learners should have completed these before starting this course. Learners should also be comfortable with probabilities & expectations, basic linear algebra, basic calculus, Python 3.0 (at least 1 year), and implementing algorithms from pseudocode.
By the end of this course, you will be able to:
-Understand how to use supervised learning approaches to approximate value functions
-Understand objectives for prediction (value estimation) under function approximation
-Implement TD with function approximation (state aggregation), on an environment with an infinite state space (continuous state space)
-Understand fixed basis and neural network approaches to feature construction
-Implement TD with neural network function approximation in a continuous state environment
-Understand new difficulties in exploration when moving to function approximation
-Contrast discounted problem formulations for control versus an average reward problem formulation
-Implement expected Sarsa and Q-learning with function approximation on a continuous state control task
-Understand objectives for directly estimating policies (policy gradient objectives)
-Implement a policy gradient method (called Actor-Critic) on a discrete state environment
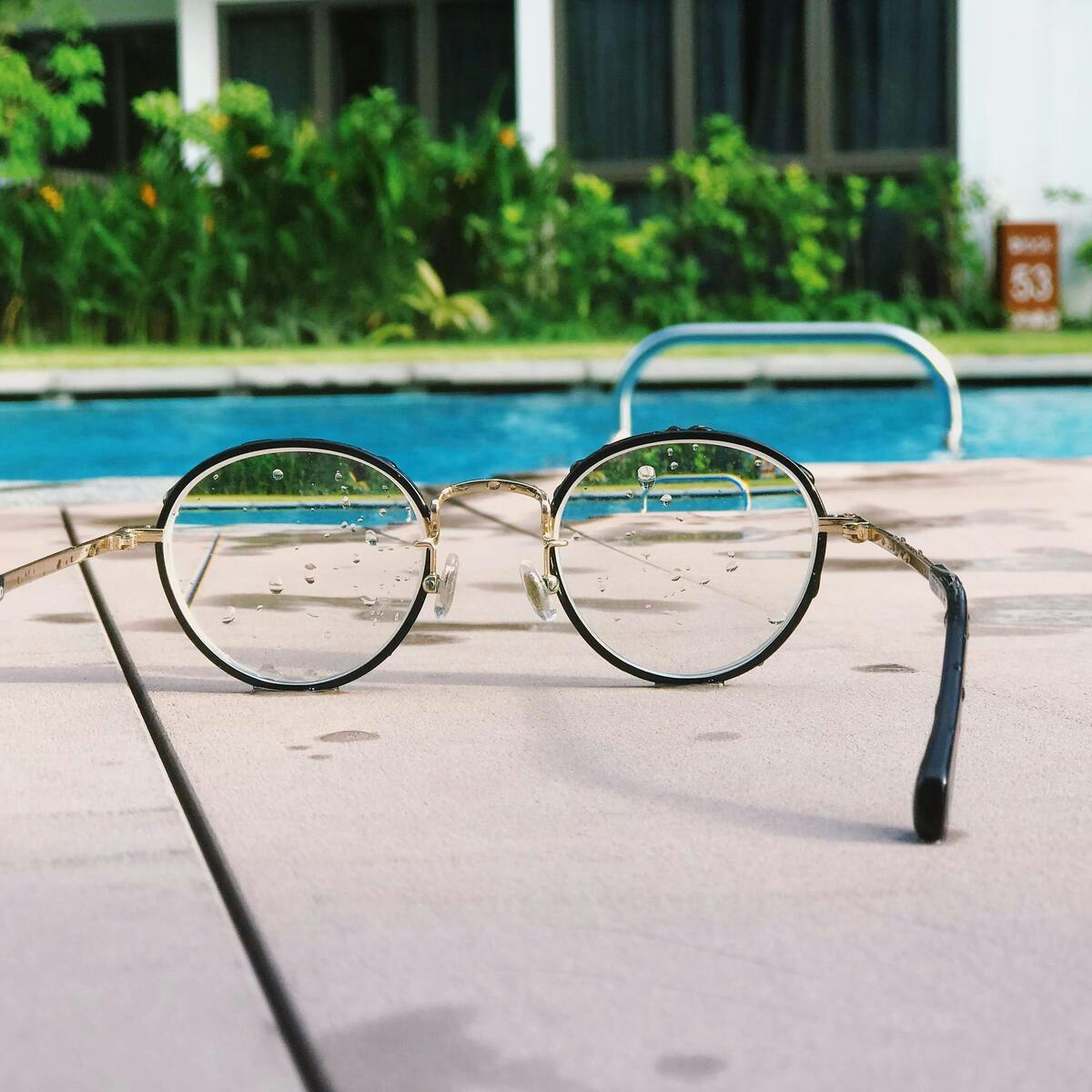
Computer Vision - Image Basics with OpenCV and Python
In this 1-hour long project-based course, you will learn how to do Computer Vision on images with OpenCV and Python using Jupyter Notebook.
This course runs on Coursera's hands-on project platform called Rhyme. On Rhyme, you do projects in a hands-on manner in your browser. You will get instant access to pre-configured cloud desktops containing all of the software and data you need for the project. Everything is already set up directly in your Internet browser so you can just focus on learning. For this project, you’ll get instant access to a cloud desktop with Python, Jupyter, and OpenCV pre-installed.
Prerequisites:
In order to be successful in this project, you should have a basic knowledge of Python.
Notes:
- You will be able to access the cloud desktop 5 times. However, you will be able to access instructions videos as many times as you want.
- This course works best for learners who are based in the North America region. We’re currently working on providing the same experience in other regions.
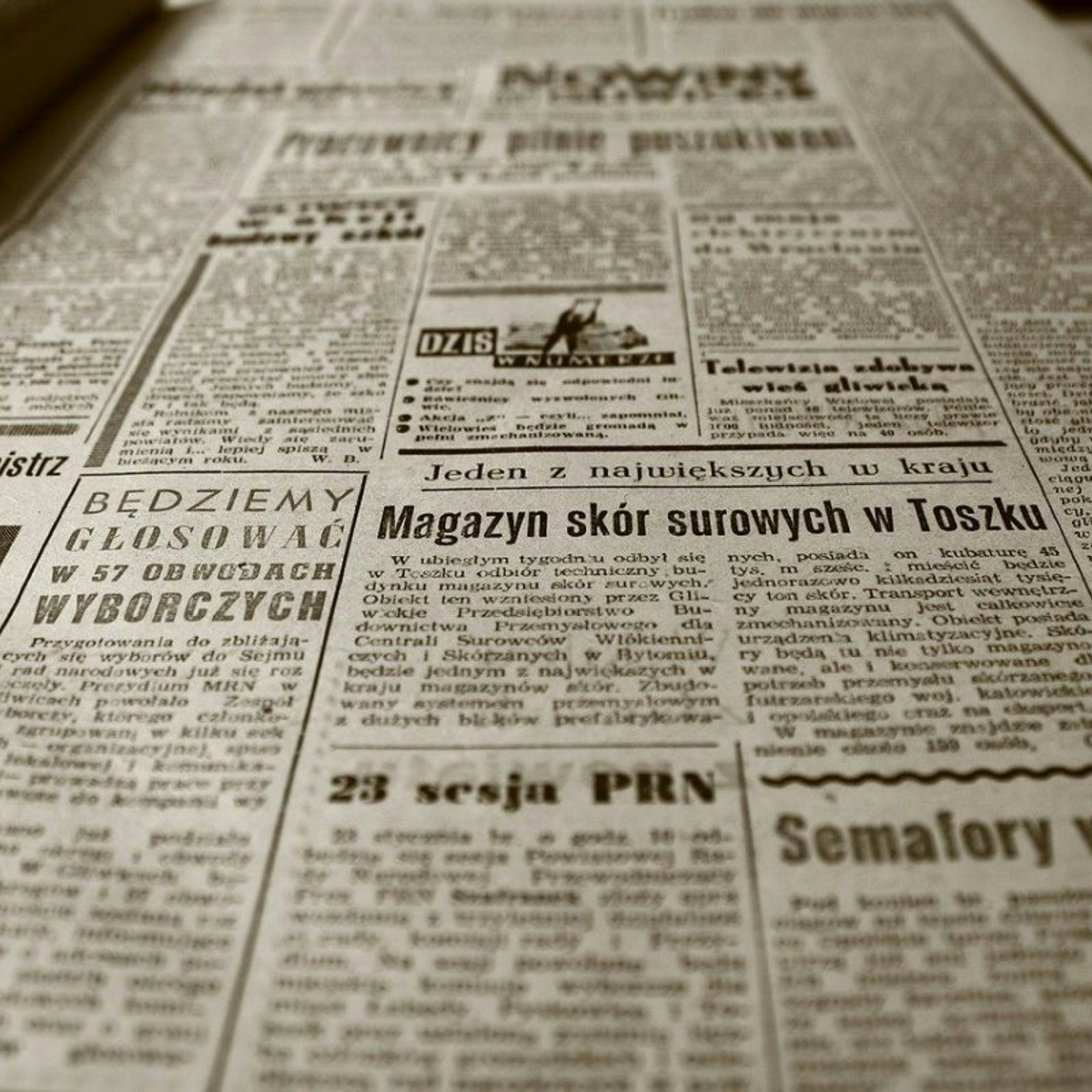
Introduction to Topic Modelling in R
By the end of this project, you will know how to load and pre-process a data set of text documents by converting the data set into a document feature matrix and reducing it’s dimensionality. You will also know how to run an unsupervised machine learning LDA topic model (Latent Dirichlet Allocation). You will know how to plot the change in topics over time as well as explore the distribution of topic probability in each document.
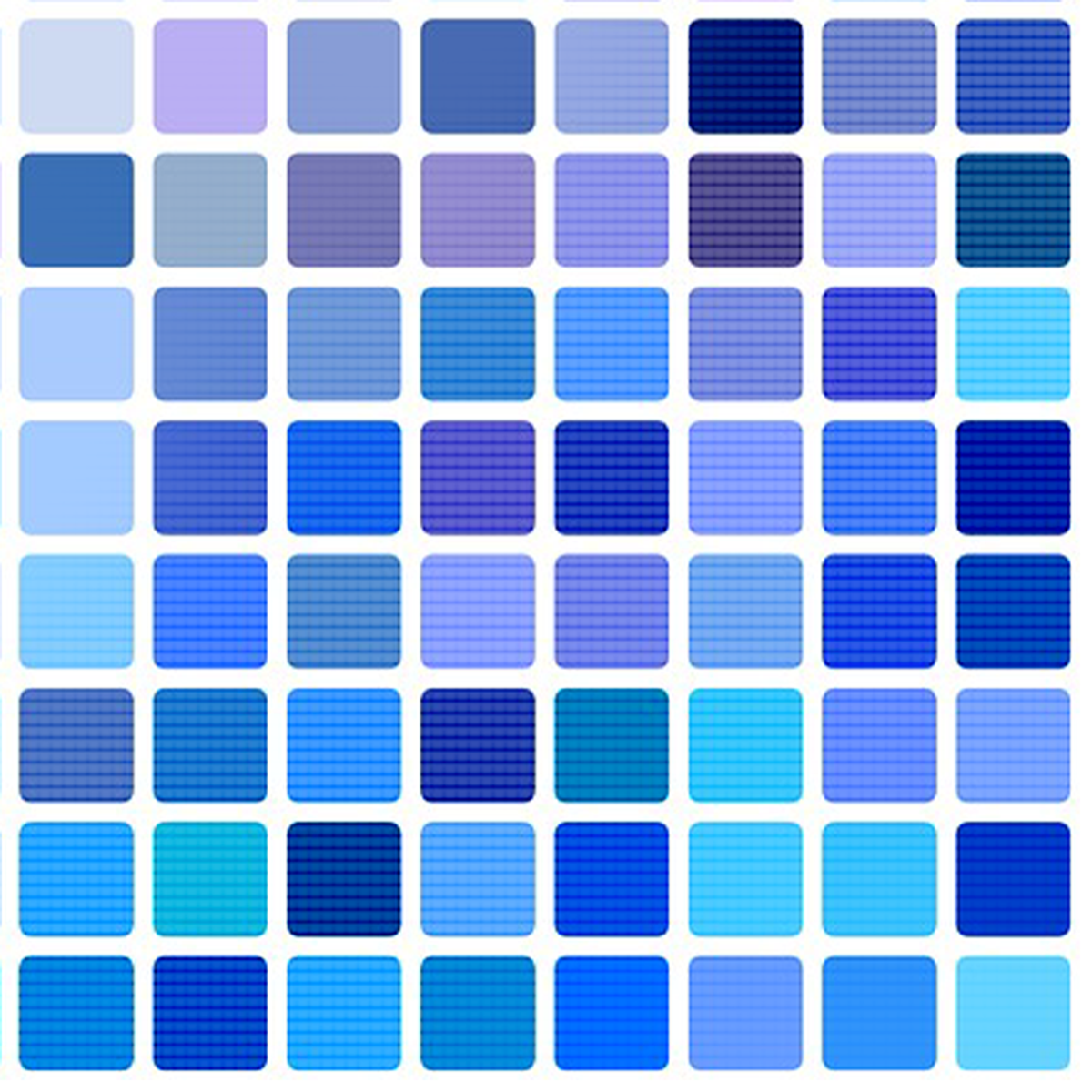
Predicting Credit Card Fraud with R
Welcome to Predicting Credit Card Fraud with R. In this project-based course, you will learn how to use R to identify fraudulent credit card transactions with a variety of classification methods and use R to generate synthetic samples to address the common problem of classification bias for highly imbalanced datasets—the class of interest (fraud) represents less than 1% of the observations.
Class imbalance can make it difficult to detect the effect independent variables have on fraud, ultimately leading to higher misclassification rates. Fixing the imbalance allows the minority class (fraud) to be better learned by the classifier algorithms.
After completing the project, you will be able to apply the methods introduced in the project to a wide range of classification problems that typically confront class imbalance, including predicting loan default, customer churn, cancer diagnosis, early high school dropout risk, and malware detection.
Note: This course works best for learners who are based in the North America region. We’re currently working on providing the same experience in other regions.
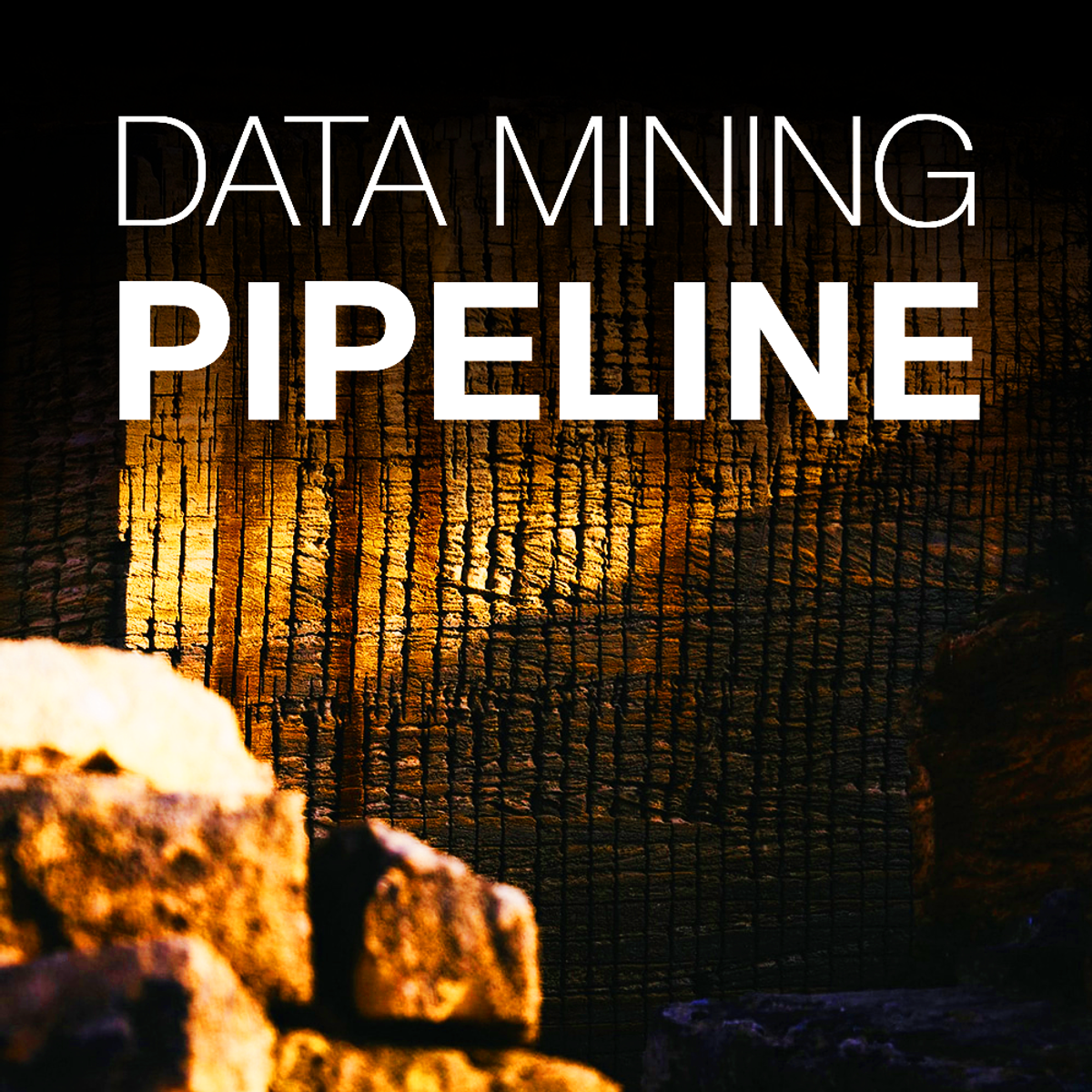
Data Mining Pipeline
This course introduces the key steps involved in the data mining pipeline, including data understanding, data preprocessing, data warehousing, data modeling, interpretation and evaluation, and real-world applications.
Data Mining Pipeline can be taken for academic credit as part of CU Boulder’s Master of Science in Data Science (MS-DS) degree offered on the Coursera platform. The MS-DS is an interdisciplinary degree that brings together faculty from CU Boulder’s departments of Applied Mathematics, Computer Science, Information Science, and others. With performance-based admissions and no application process, the MS-DS is ideal for individuals with a broad range of undergraduate education and/or professional experience in computer science, information science, mathematics, and statistics. Learn more about the MS-DS program at https://www.coursera.org/degrees/master-of-science-data-science-boulder.
Course logo image courtesy of Francesco Ungaro, available here on Unsplash: https://unsplash.com/photos/C89G61oKDDA
Popular Internships and Jobs by Categories
Browse
© 2024 BoostGrad | All rights reserved