Back to Courses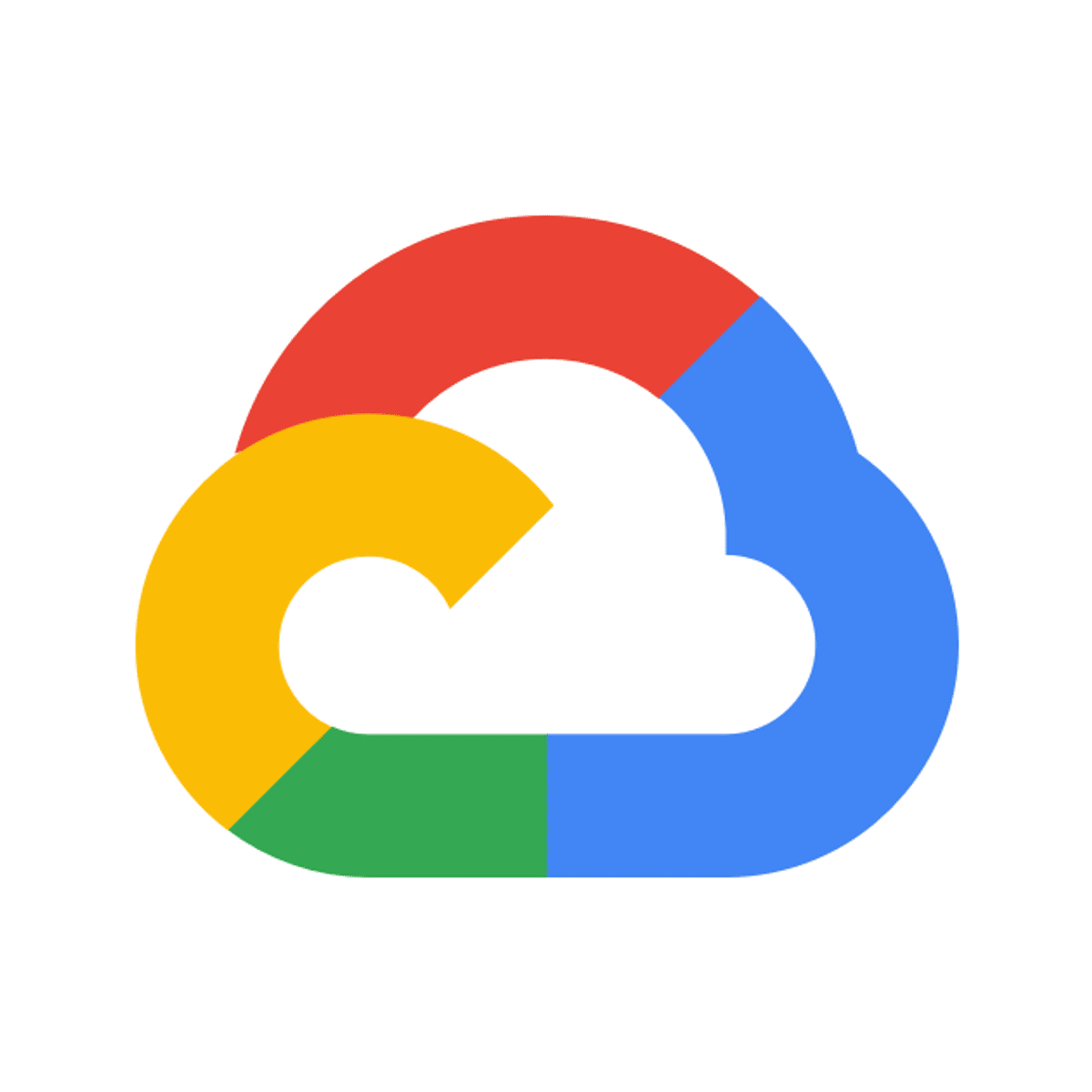
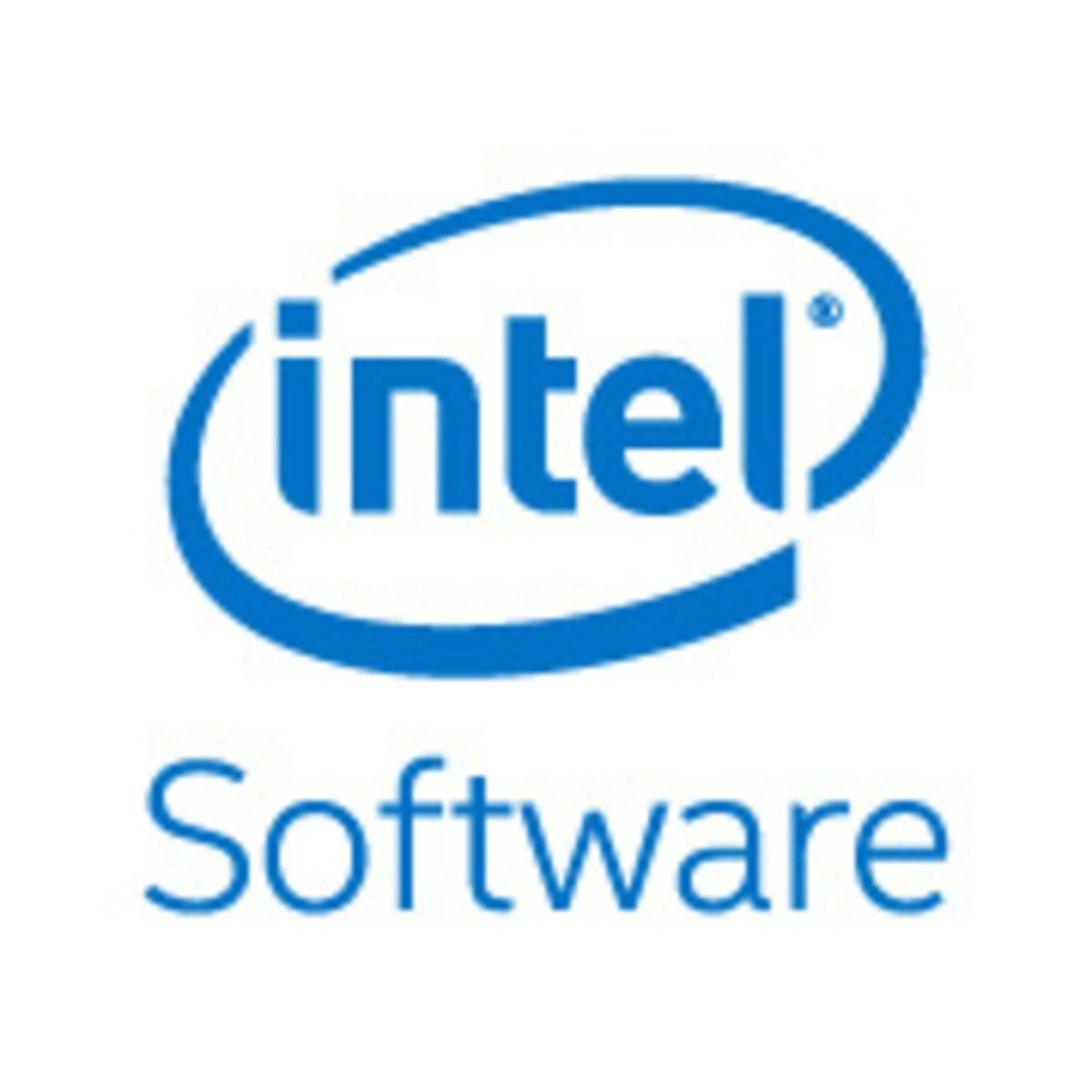
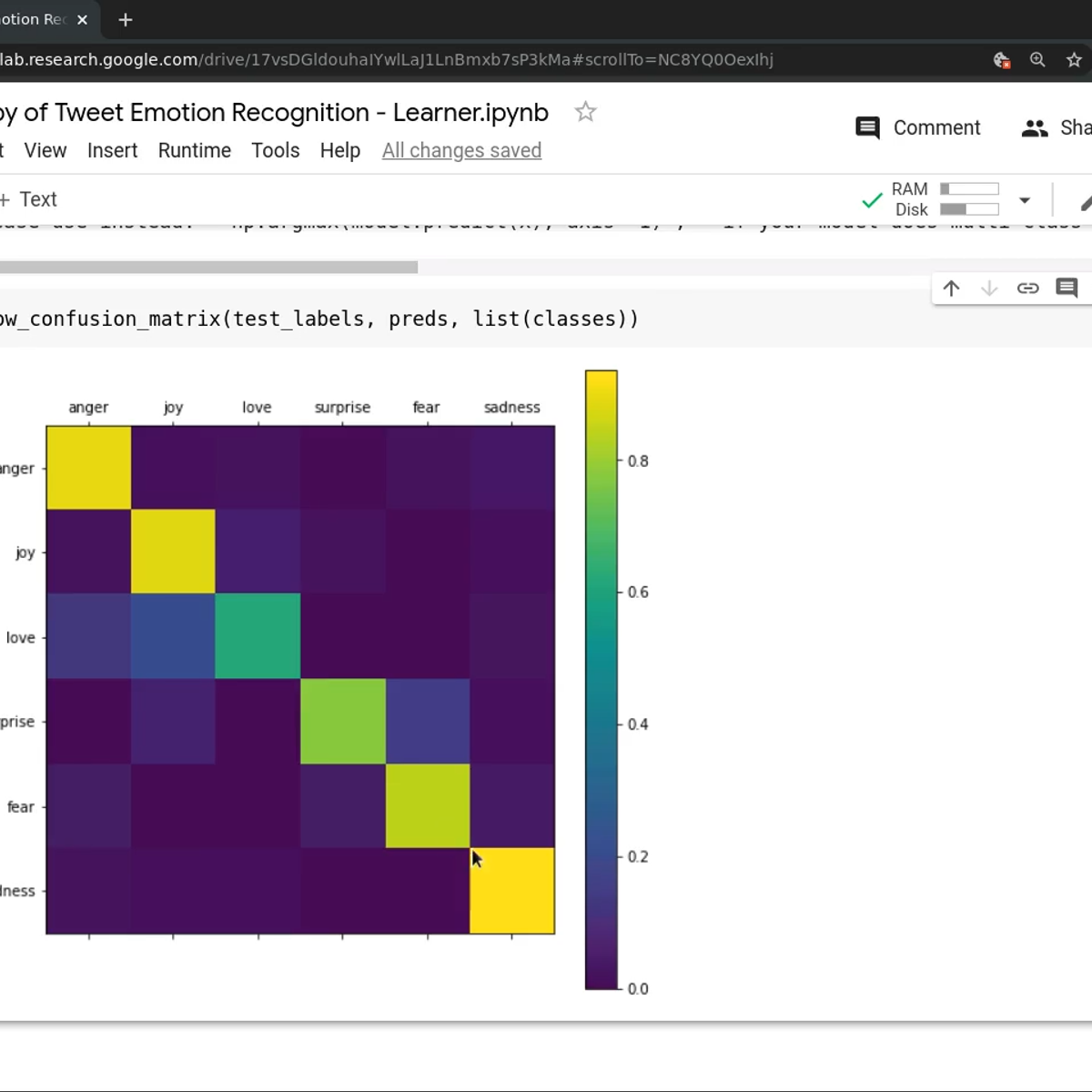
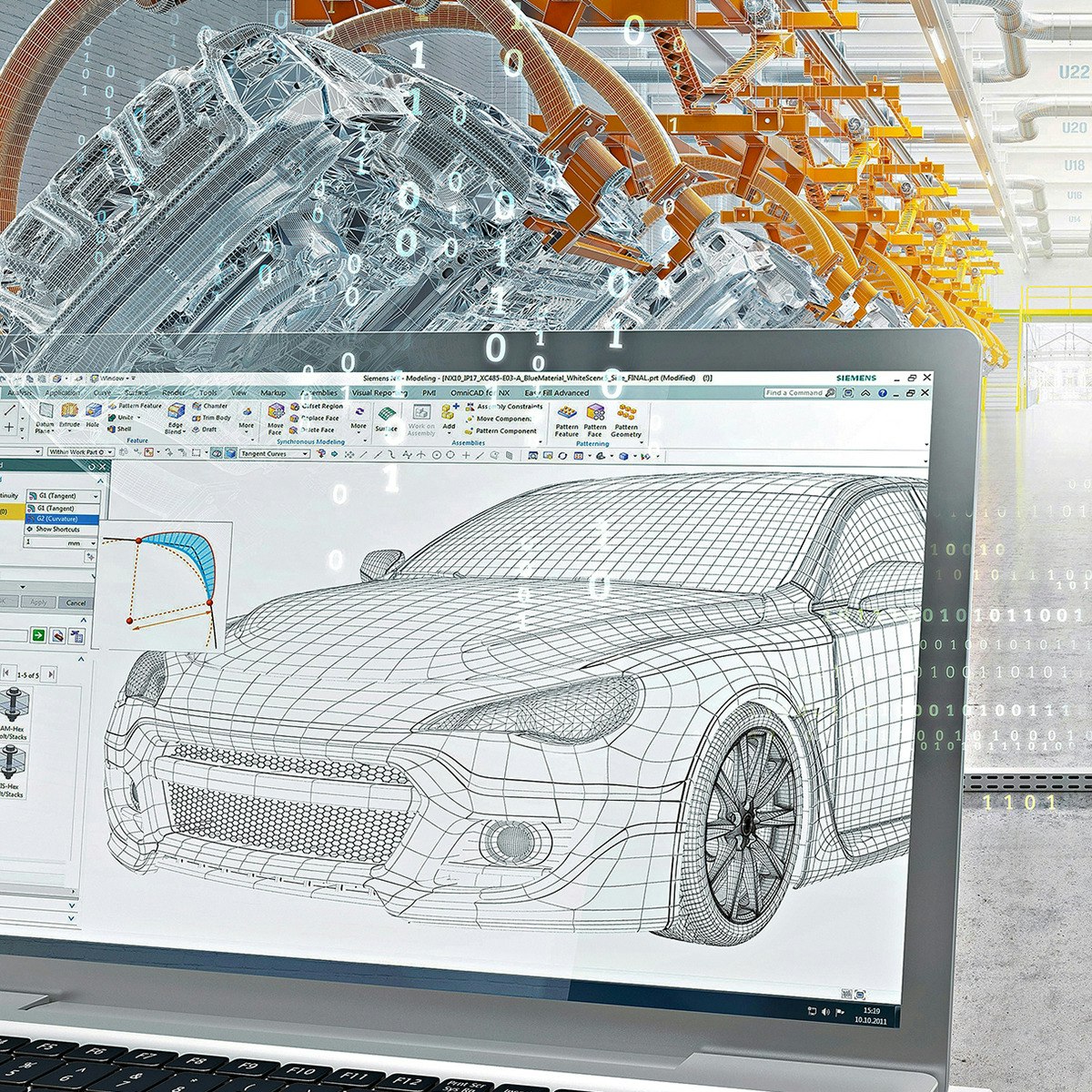
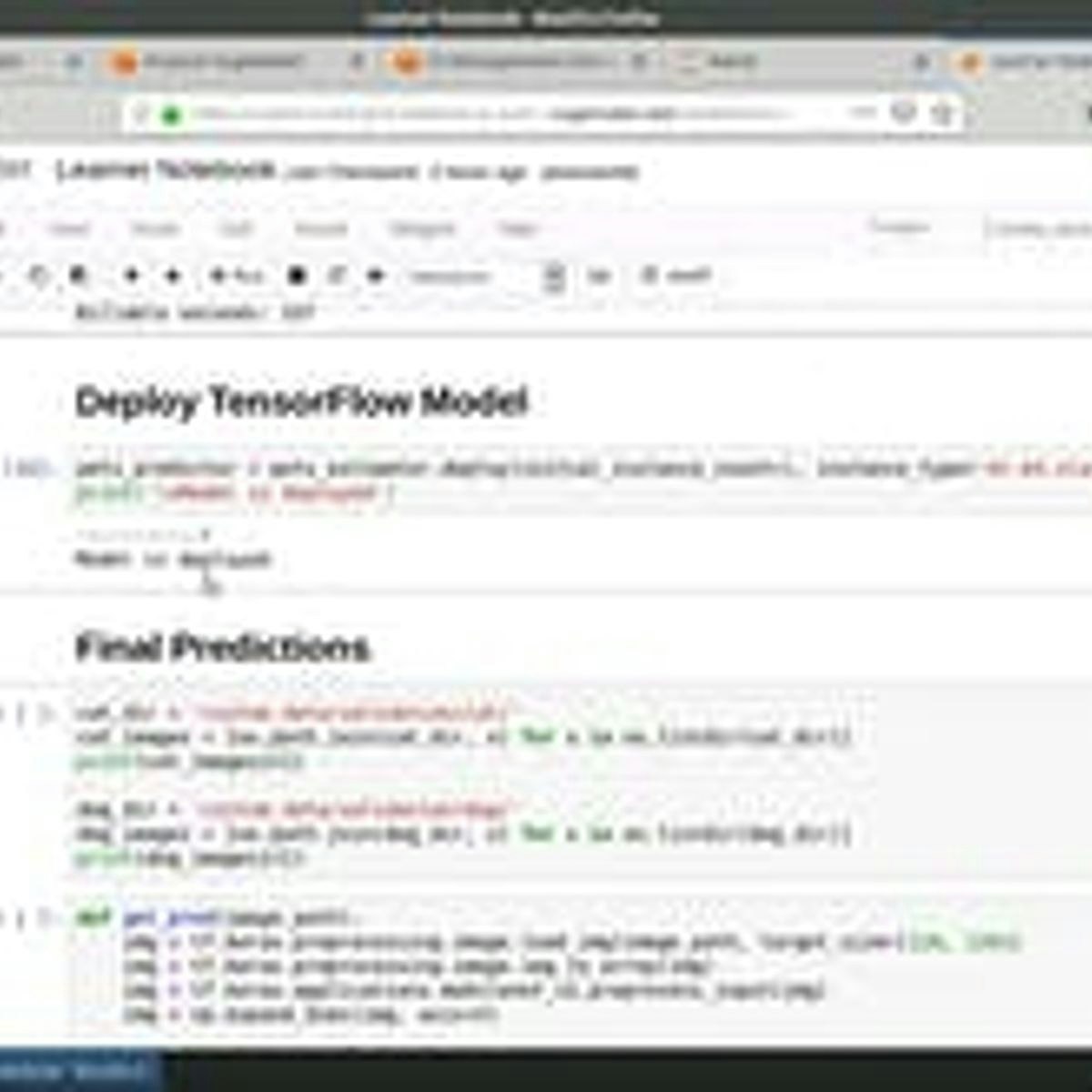
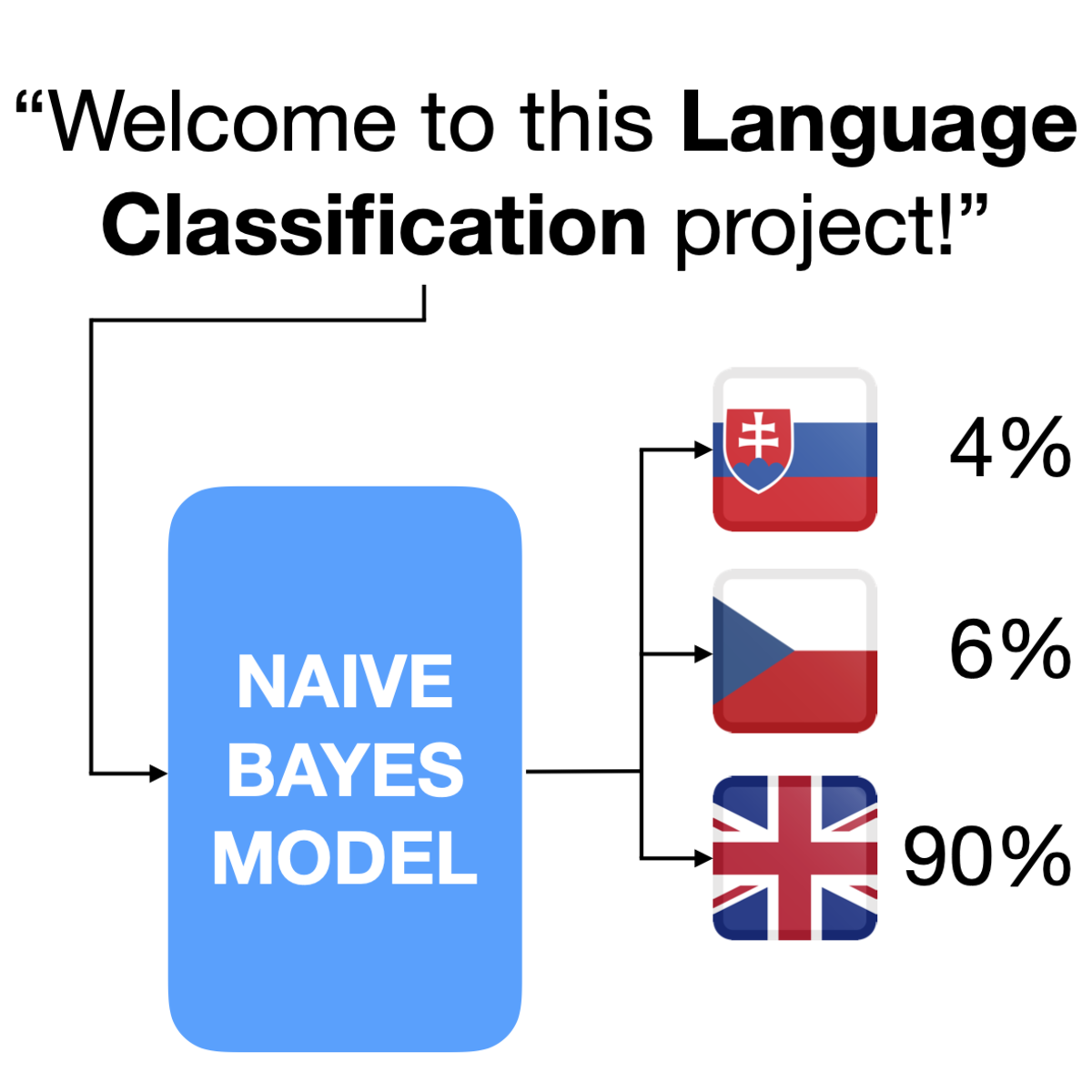
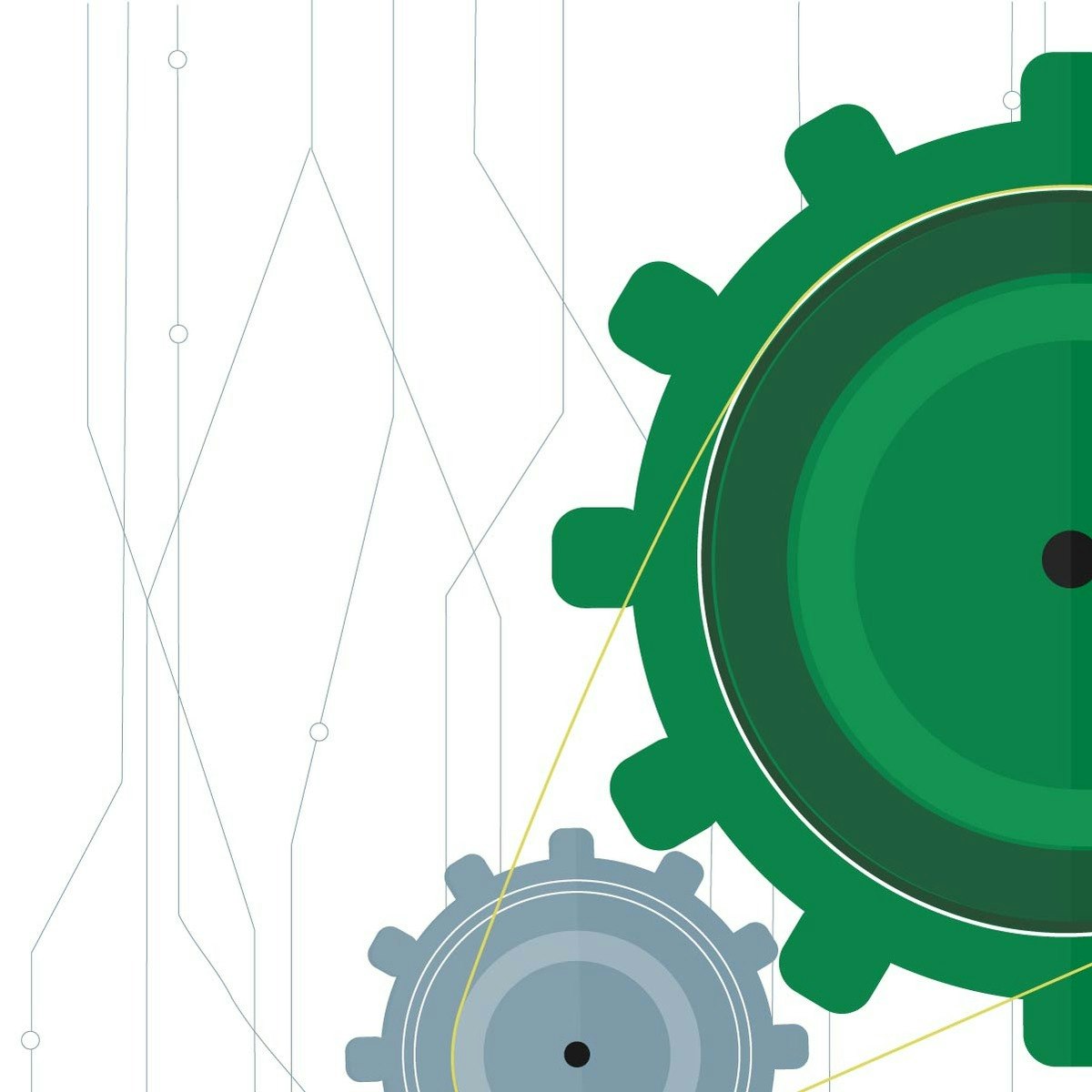
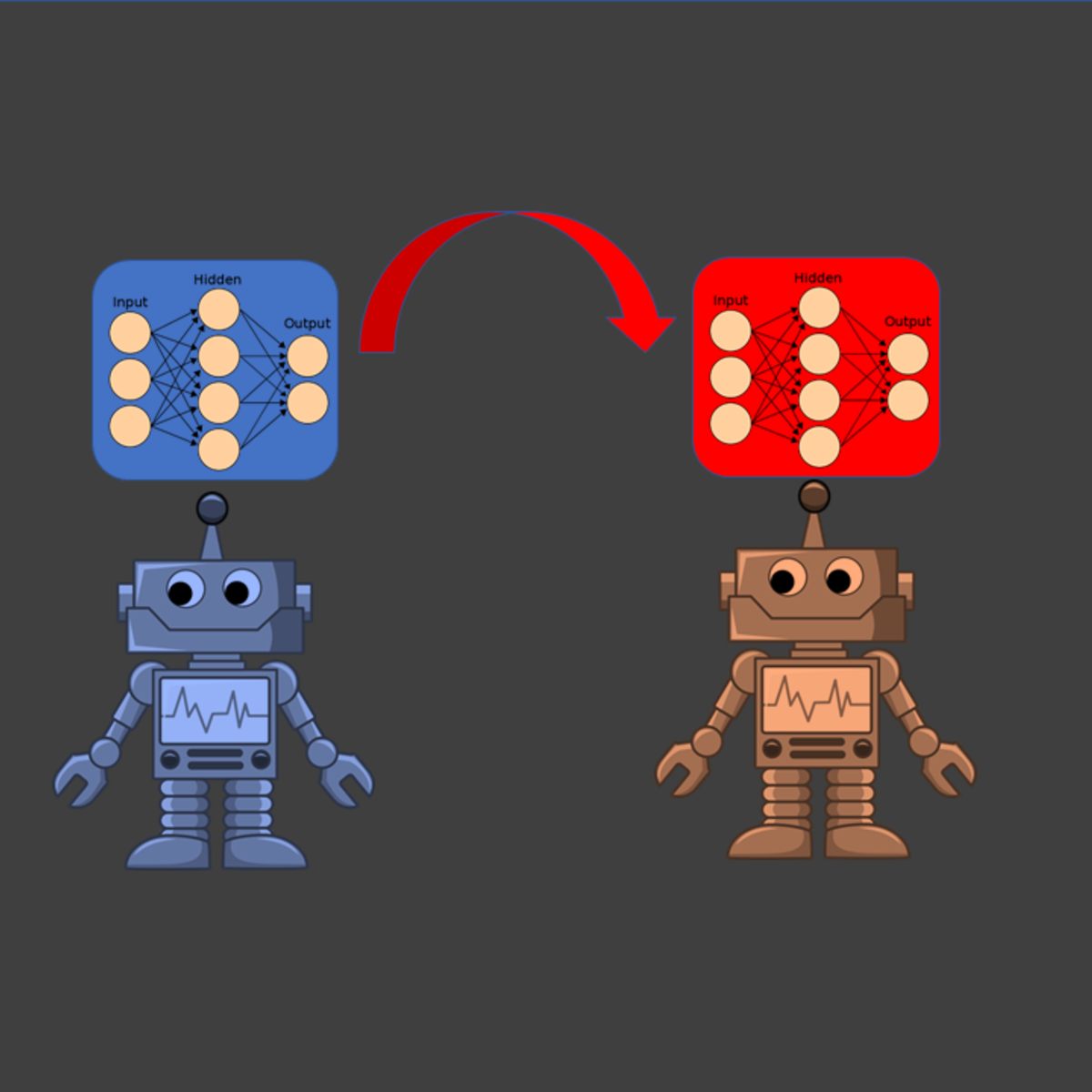
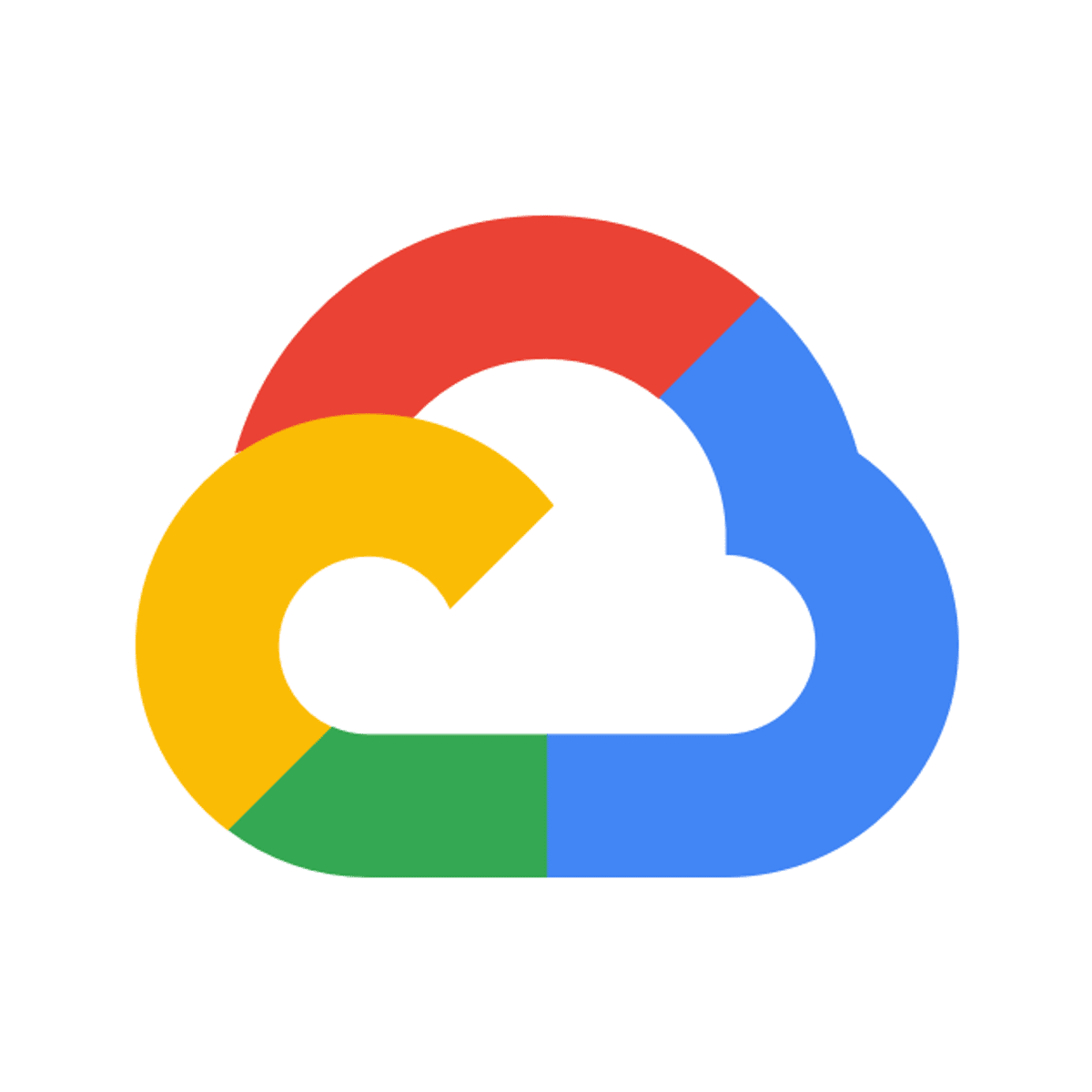
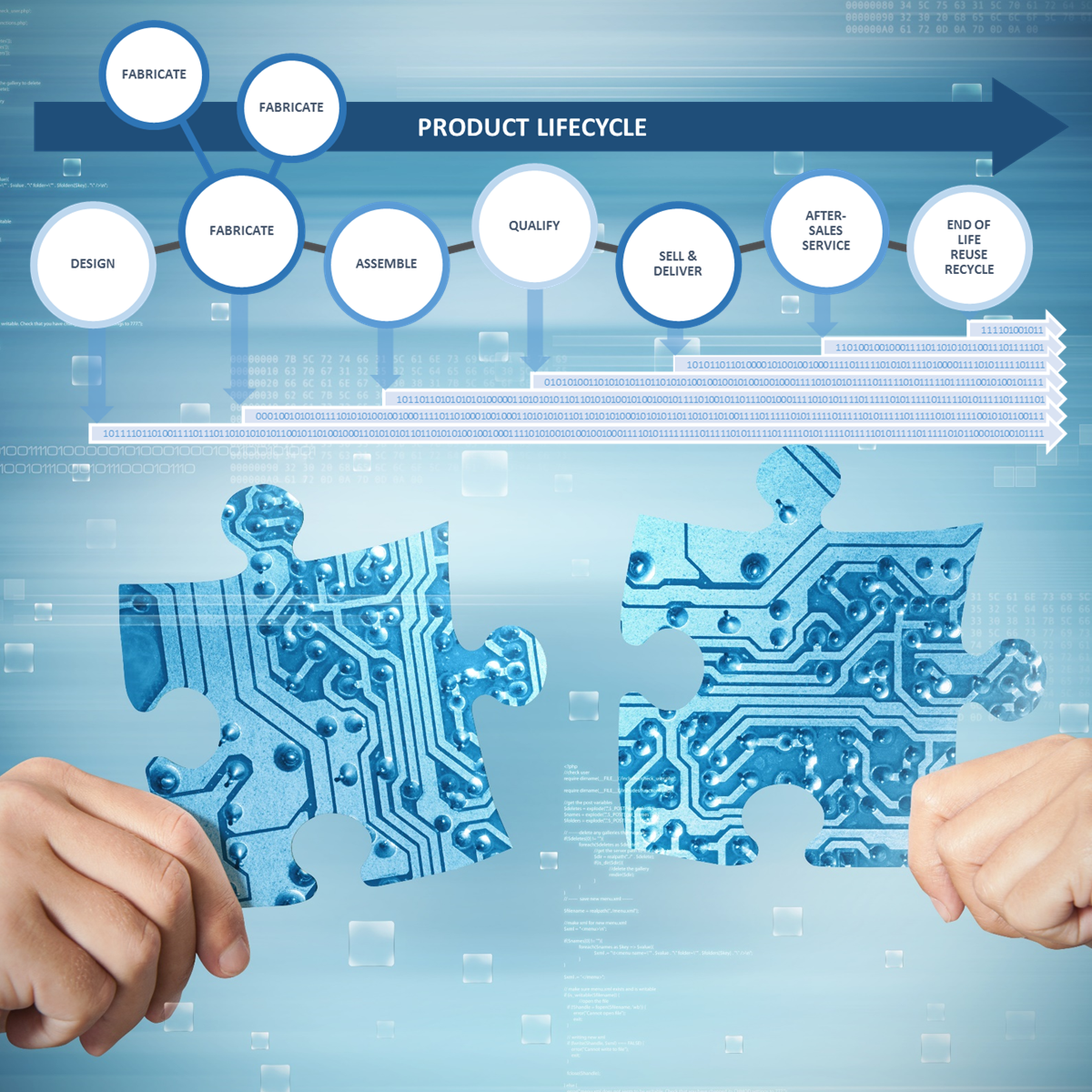
Machine Learning Courses - Page 20
Showing results 191-200 of 485
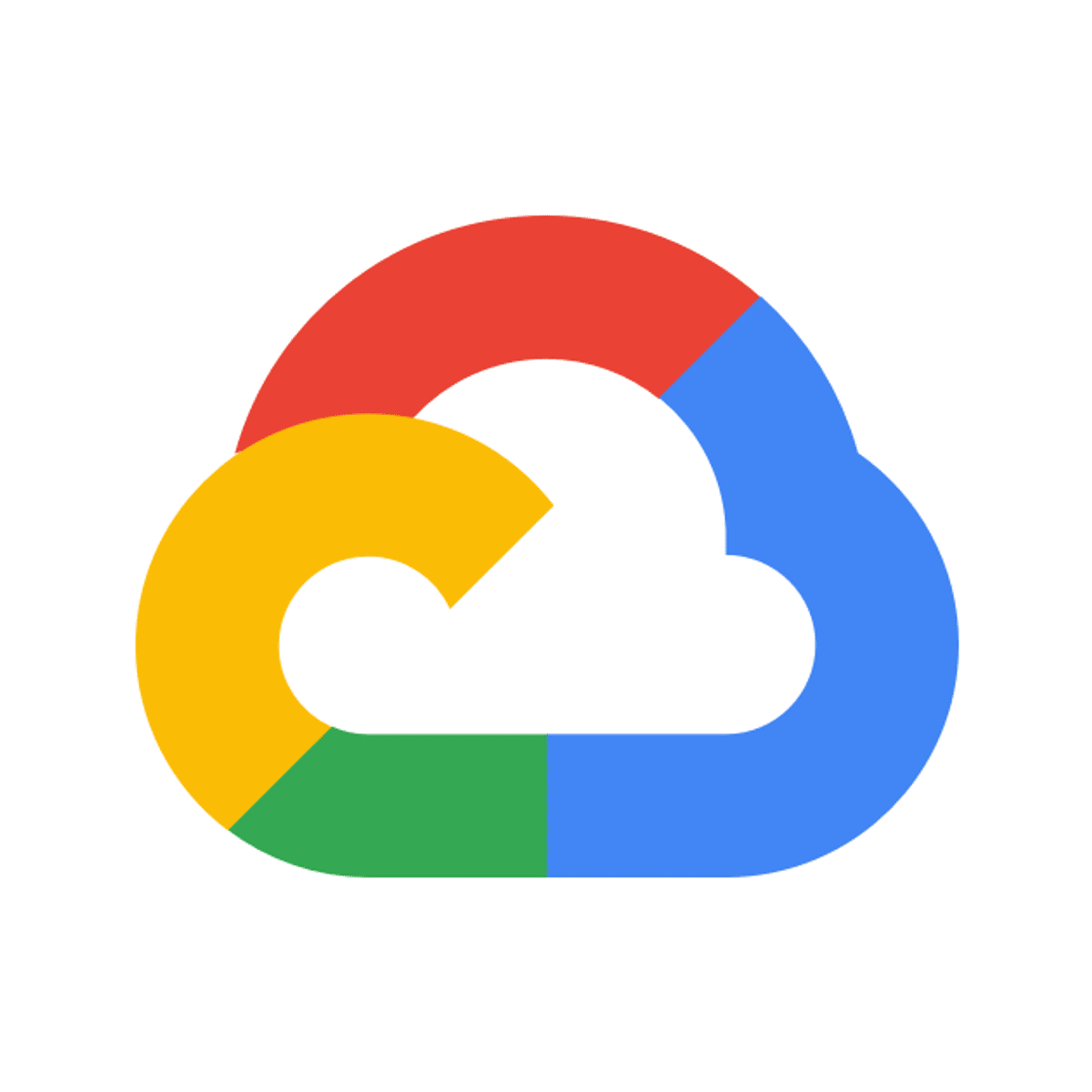
Detect Labels, Faces, and Landmarks in Images with the Cloud Vision API
This is a self-paced lab that takes place in the Google Cloud console.
The Cloud Vision API lets you understand the content of an image by encapsulating powerful machine learning models in a simple REST API. In this lab you’ll send an image to the Cloud Vision API and have it identify objects, faces, and landmarks.
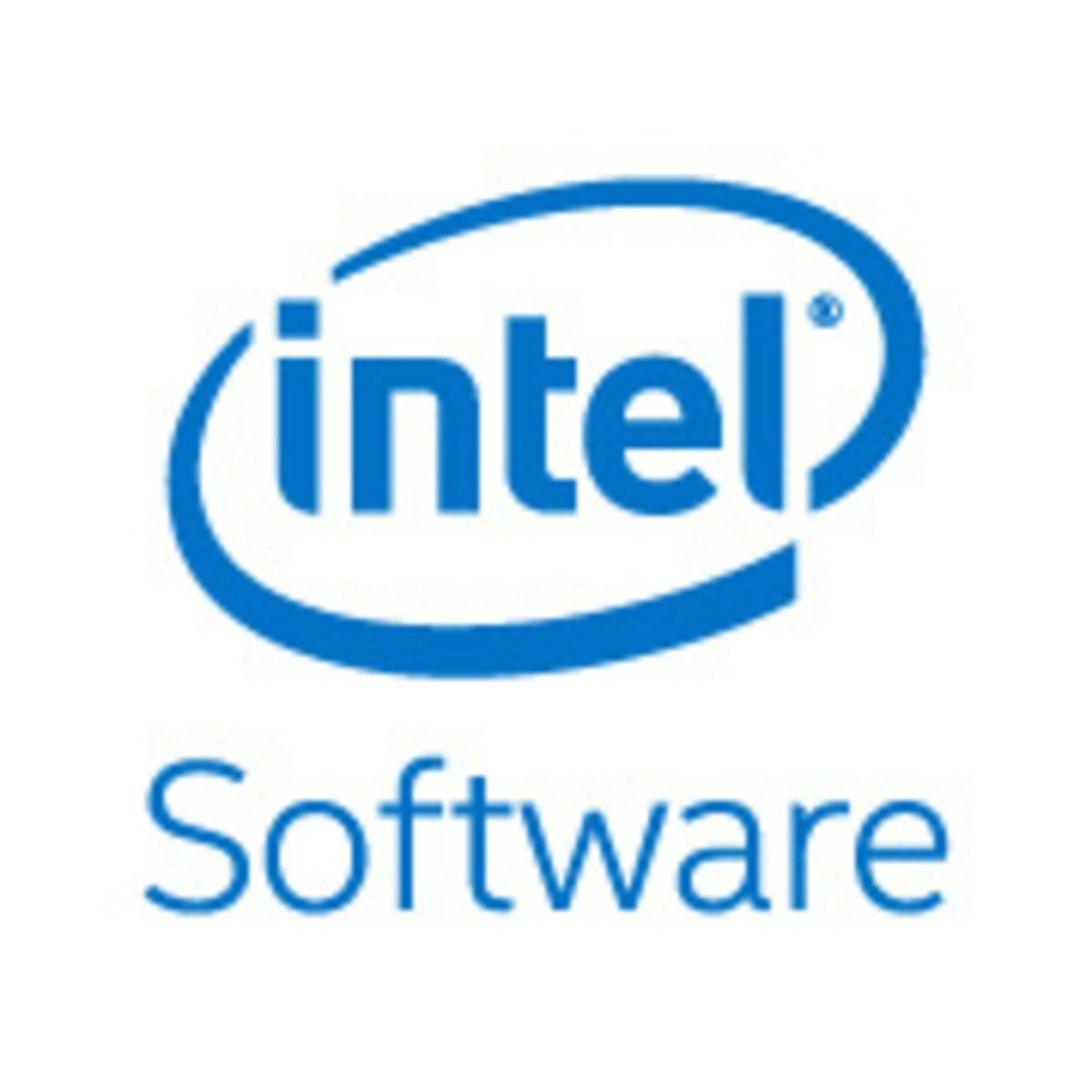
An Introduction to Practical Deep Learning
This course provides an introduction to Deep Learning, a field that aims to harness the enormous amounts of data that we are surrounded by with artificial neural networks, allowing for the development of self-driving cars, speech interfaces, genomic sequence analysis and algorithmic trading.
You will explore important concepts in Deep Learning, train deep networks using Intel Nervana Neon, apply Deep Learning to various applications and explore new and emerging Deep Learning topics.
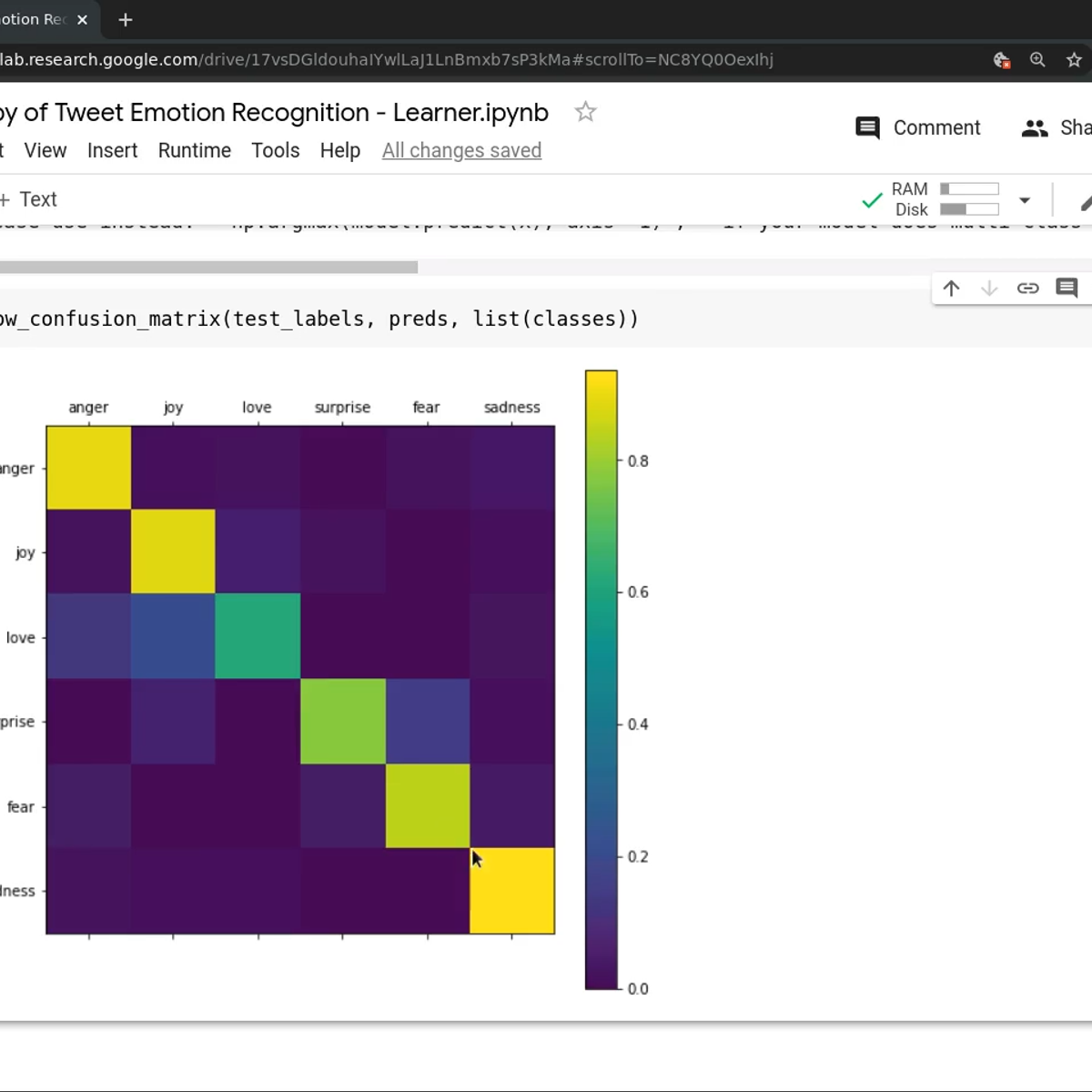
Tweet Emotion Recognition with TensorFlow
In this 2-hour long guided project, we are going to create a recurrent neural network and train it on a tweet emotion dataset to learn to recognize emotions in tweets. The dataset has thousands of tweets each classified in one of 6 emotions. This is a multi class classification problem in the natural language processing domain. We will be using TensorFlow as our machine learning framework.
You will need prior programming experience in Python. This is a practical, hands on guided project for learners who already have theoretical understanding of Neural Networks, recurrent neural networks, and optimization algorithms like gradient descent but want to understand how to use the Tensorflow to start performing natural language processing tasks like text classification. You should also have some basic familiarity with TensorFlow.
Note: This course works best for learners who are based in the North America region. We’re currently working on providing the same experience in other regions.
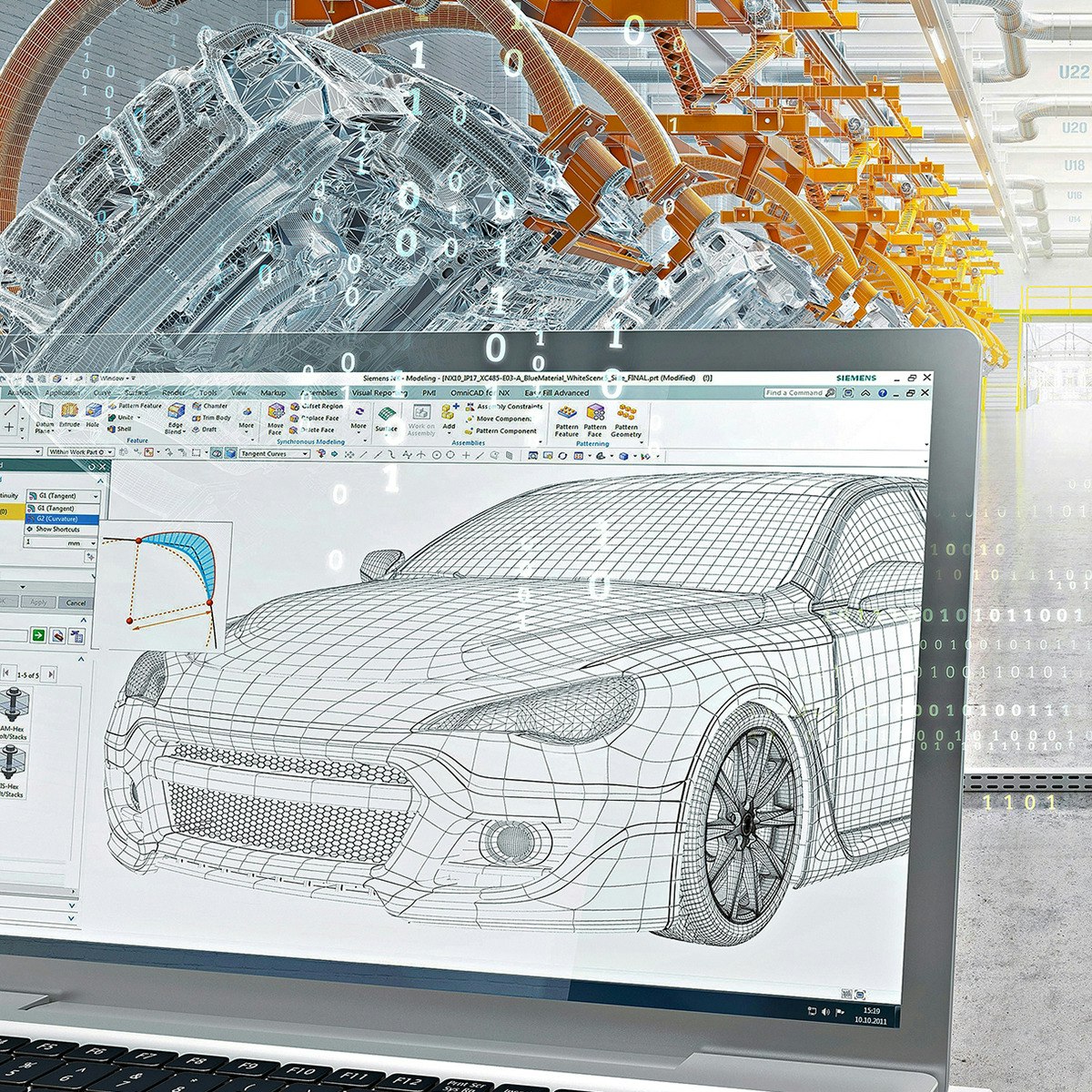
Digital Thread: Implementation
There are opportunities throughout the design process of any product to make significant changes, and ultimately impact the future of manufacturing, by embracing the digital thread. In this course, you will dig into the transformation taking place in how products are designed and manufactured throughout the world. It is the second of two courses that focuses on the "digital thread" – the stream that starts at the creation of a product concept and continues to accumulate information and data throughout the product life cycle.
Hear about the realities of implementing the digital thread, directly from someone responsible for making it happen at a company. Learn how the digital thread can fit into product development processes in an office, on a shop floor, and even across an enterprise. Be prepared to talk about the benefits, and limitations, of enacting it.
Main concepts of this course will be delivered through lectures, readings, discussions and various videos.
This is the third course in the Digital Manufacturing & Design Technology specialization that explores the many facets of manufacturing’s “Fourth Revolution,” aka Industry 4.0, and features a culminating project involving creation of a roadmap to achieve a self-established DMD-related professional goal. To learn more about the Digital Manufacturing and Design Technology specialization, please watch the overview video by copying and pasting the following link into your web browser: https://youtu.be/wETK1O9c-CA
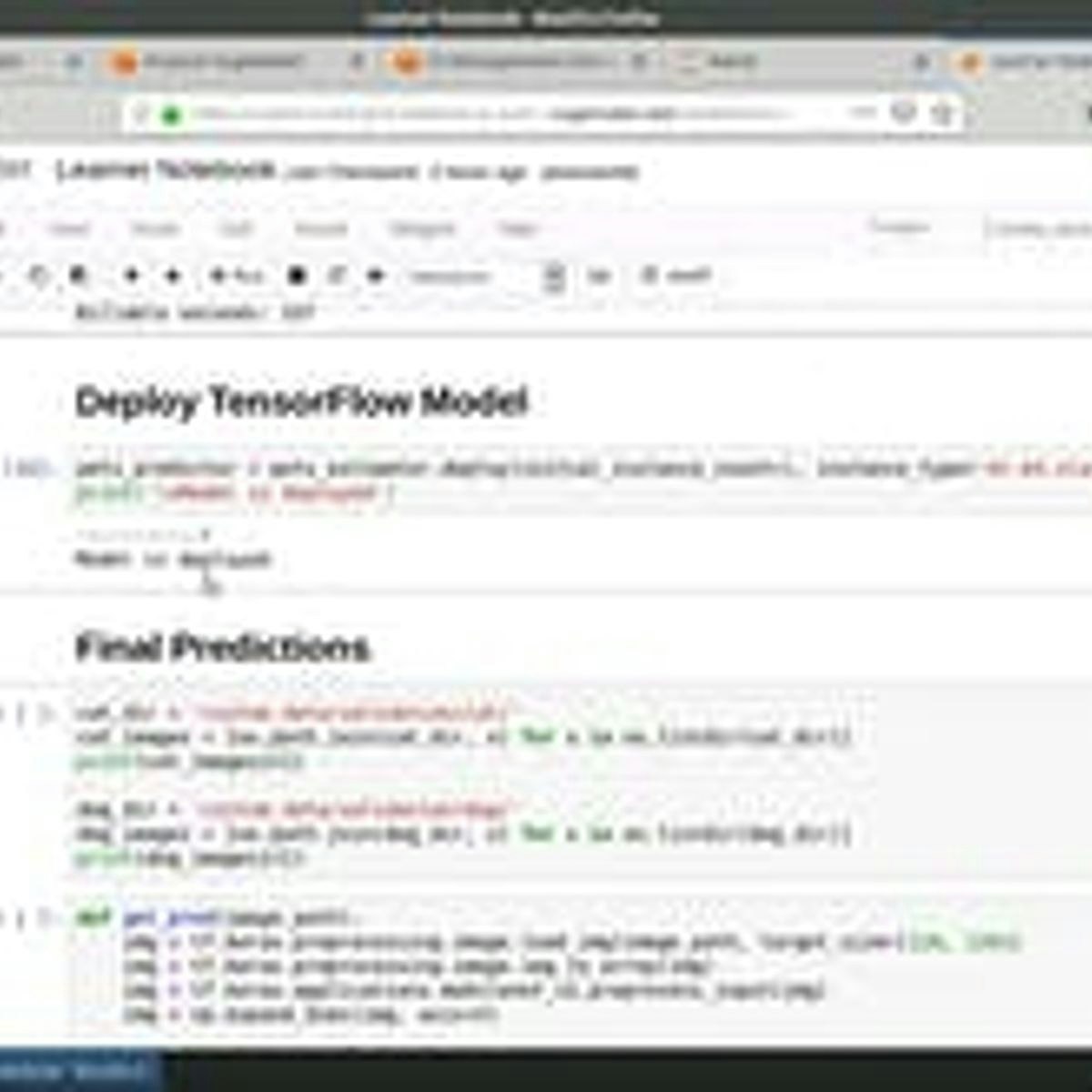
Using TensorFlow with Amazon Sagemaker
Please note: You will need an AWS account to complete this course. Your AWS account will be charged as per your usage. Please make sure that you are able to access Sagemaker within your AWS account. If your AWS account is new, you may need to ask AWS support for access to certain resources. You should be familiar with python programming, and AWS before starting this hands on project. We use a Sagemaker P type instance in this project, and if you don't have access to this instance type, please contact AWS support and request access.
In this 2-hour long project-based course, you will learn how to train and deploy an image classifier created and trained with the TensorFlow framework within the Amazon Sagemaker ecosystem. Sagemaker provides a number of machine learning algorithms ready to be used for solving a number of tasks. However, it is possible to use Sagemaker for custom training scripts as well. We will use TensorFlow and Sagemaker's TensorFlow Estimator to create, train and deploy a model that will be able to classify images of dogs and cats from the popular Oxford IIIT Pet Dataset.
Since this is a practical, project-based course, we will not dive in the theory behind deep learning based image classification, but will focus purely on training and deploying a model with Sagemaker and TensorFlow. You will also need to have some experience with Amazon Web Services (AWS).
Note: This course works best for learners who are based in the North America region. We’re currently working on providing the same experience in other regions.
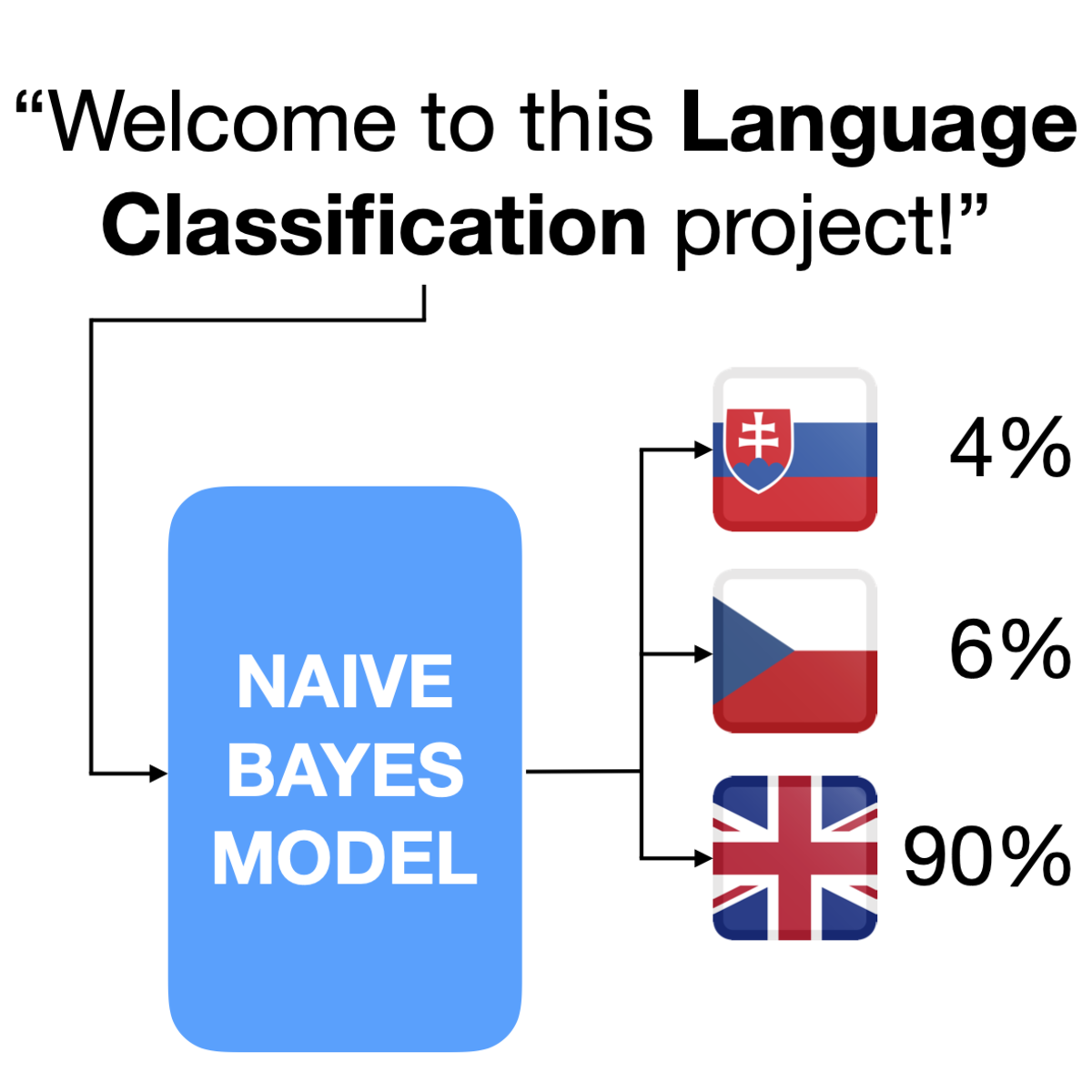
Language Classification with Naive Bayes in Python
In this 1-hour long project, you will learn how to clean and preprocess data for language classification. You will learn some theory behind Naive Bayes Modeling, and the impact that class imbalance of training data has on classification performance. You will learn how to use subword units to further mitigate the negative effects of class imbalance, and build an even better model.
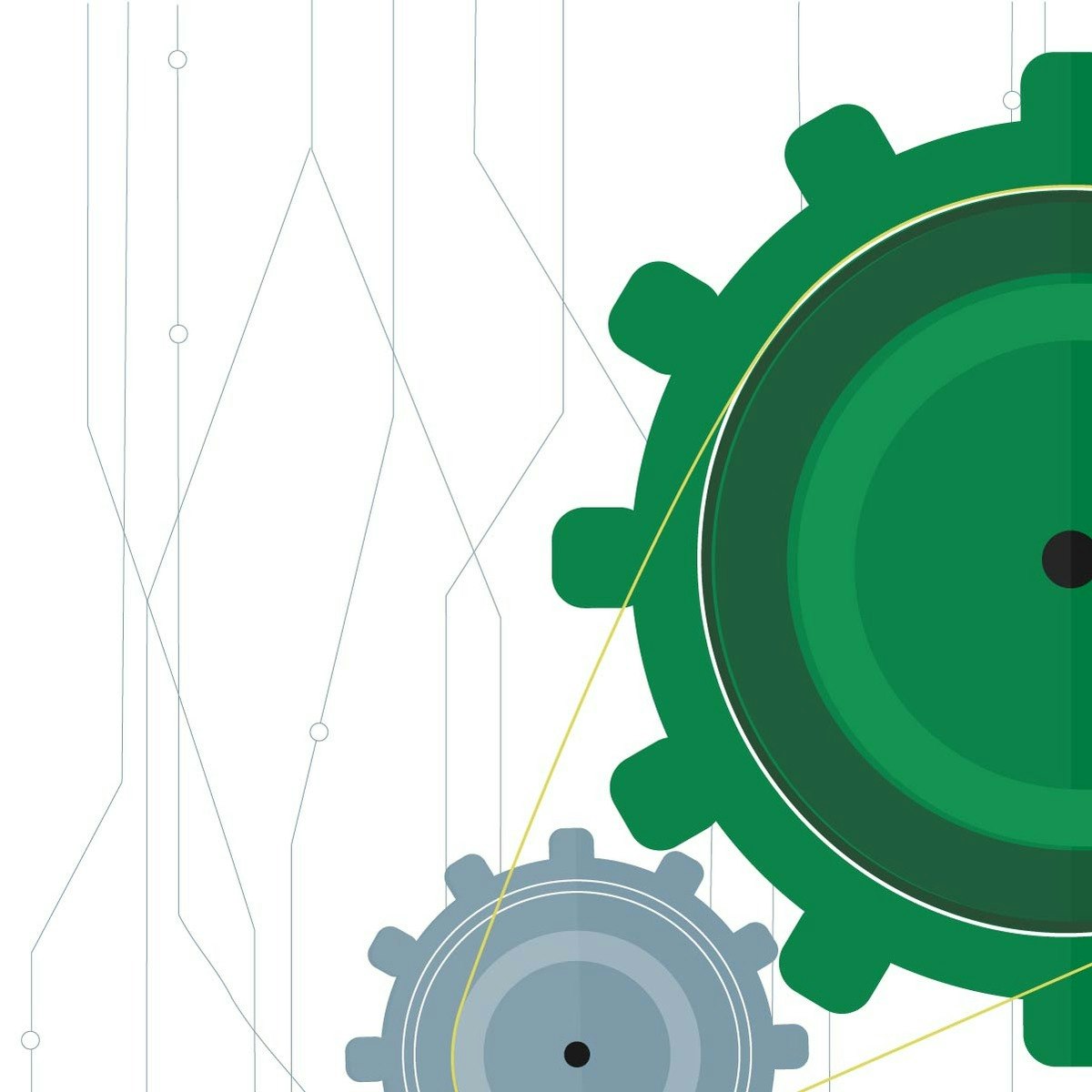
Fundamentals of Reinforcement Learning
Reinforcement Learning is a subfield of Machine Learning, but is also a general purpose formalism for automated decision-making and AI. This course introduces you to statistical learning techniques where an agent explicitly takes actions and interacts with the world. Understanding the importance and challenges of learning agents that make decisions is of vital importance today, with more and more companies interested in interactive agents and intelligent decision-making.
This course introduces you to the fundamentals of Reinforcement Learning. When you finish this course, you will:
- Formalize problems as Markov Decision Processes
- Understand basic exploration methods and the exploration/exploitation tradeoff
- Understand value functions, as a general-purpose tool for optimal decision-making
- Know how to implement dynamic programming as an efficient solution approach to an industrial control problem
This course teaches you the key concepts of Reinforcement Learning, underlying classic and modern algorithms in RL. After completing this course, you will be able to start using RL for real problems, where you have or can specify the MDP.
This is the first course of the Reinforcement Learning Specialization.
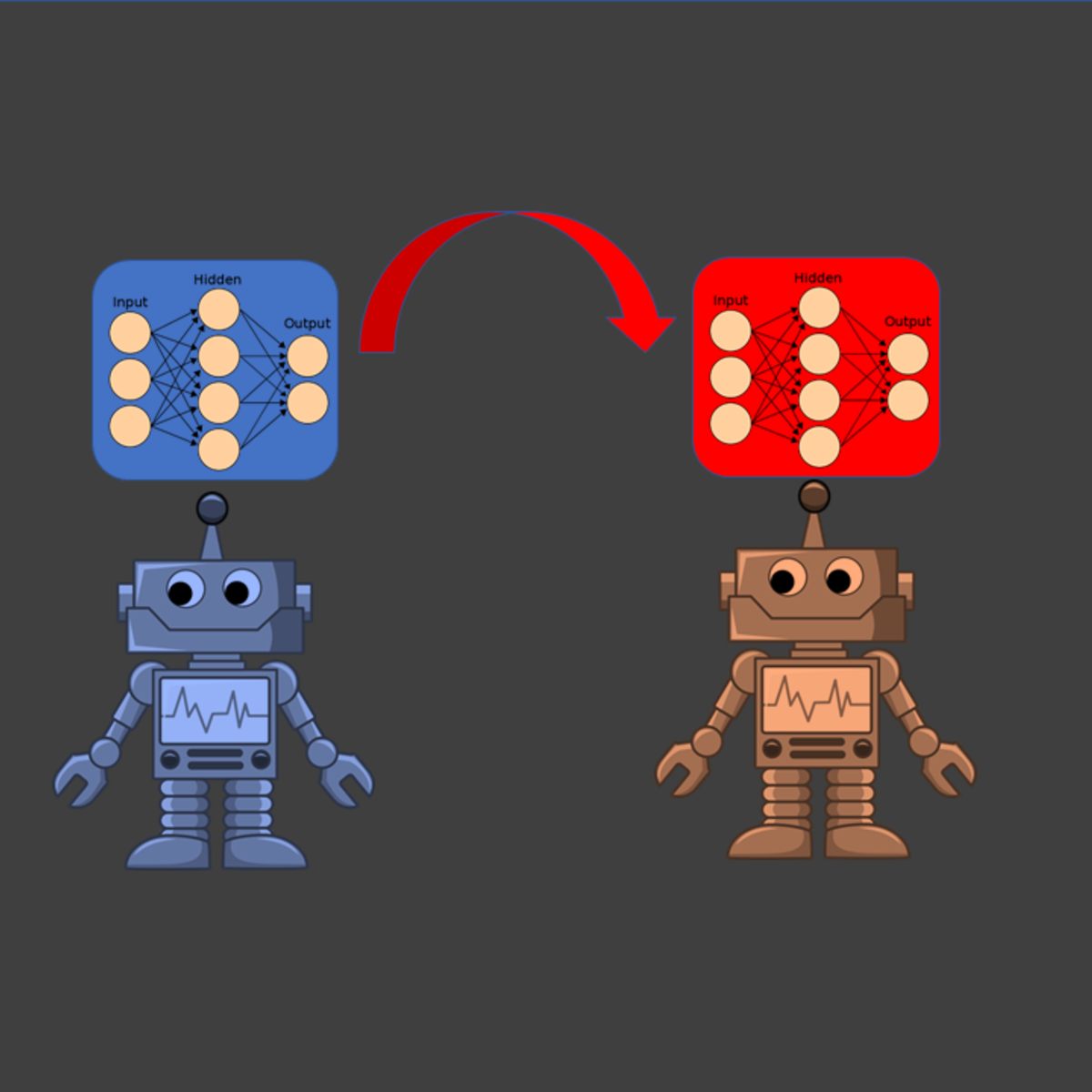
Transfer Learning for Food Classification
In this hands-on project, we will train a deep learning model to predict the type of food and then fine tune the model to improve its performance. This project could be practically applied in food industry to detect the type and quality of food. In this 2-hours long project-based course, you will be able to:
- Understand the theory and intuition behind Convolutional Neural Networks (CNNs).
- Understand the theory and intuition behind transfer learning.
- Import Key libraries, dataset and visualize images.
- Perform data augmentation.
- Build a Deep Learning Model using Pre-Trained InceptionResnetV2.
- Compile and fit Deep Learning model to training data.
- Assess the performance of trained CNN and ensure its generalization using various KPIs.
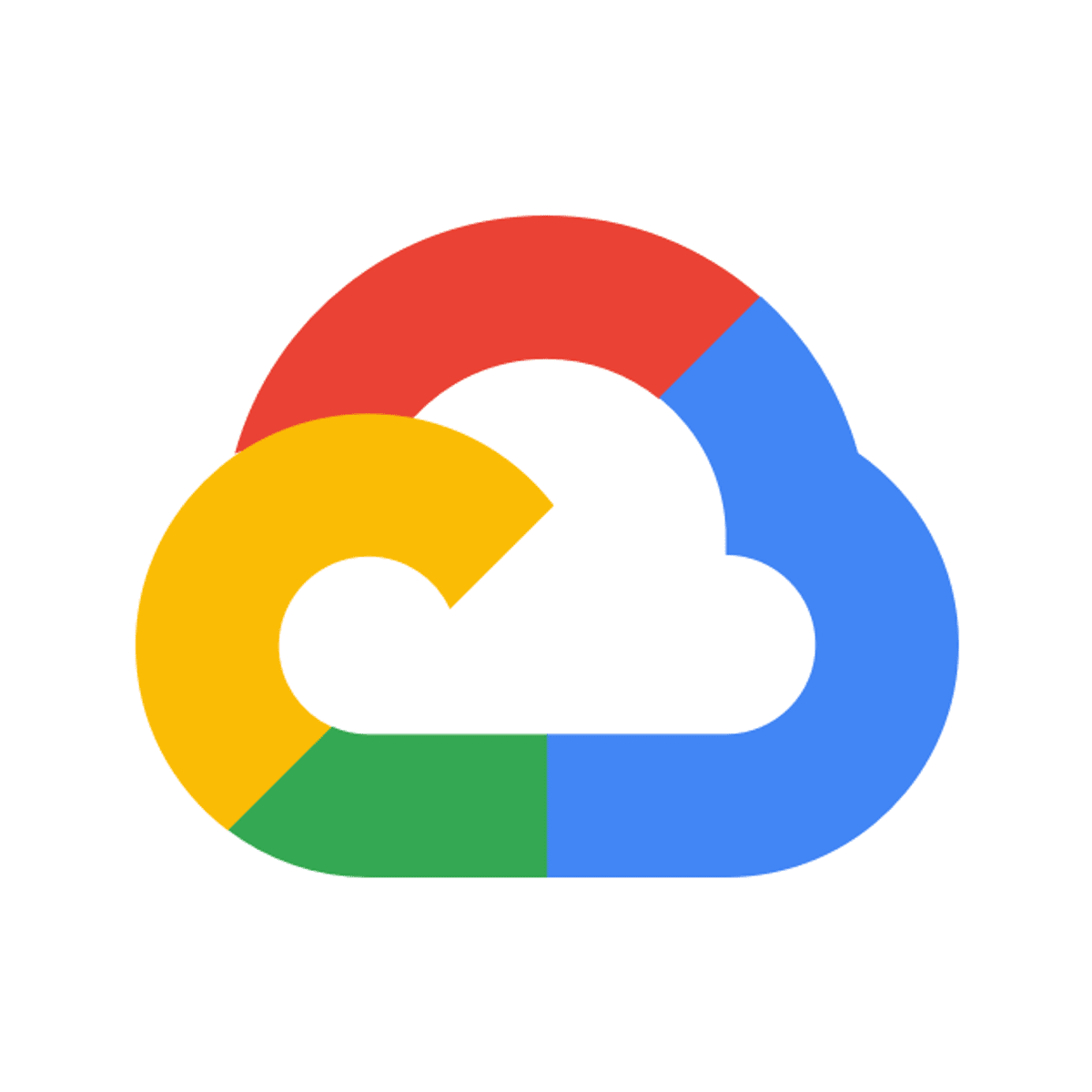
Classify Images of Cats and Dogs using Transfer Learning
This is a self-paced lab that takes place in the Google Cloud console.
TensorFlow is an end-to-end open source platform for machine learning. It has a comprehensive, flexible ecosystem of tools, libraries and community resources that lets researchers push the state-of-the-art in ML and developers easily build and deploy ML powered applications.
This lab uses transfer learning to train your machine. In transfer learning, when you build a new model to classify your original dataset, you reuse the feature extraction part and re-train the classification part with your dataset. This method uses less computational resources and training time. Deep learning from scratch can take days, but transfer learning can be done in short order.
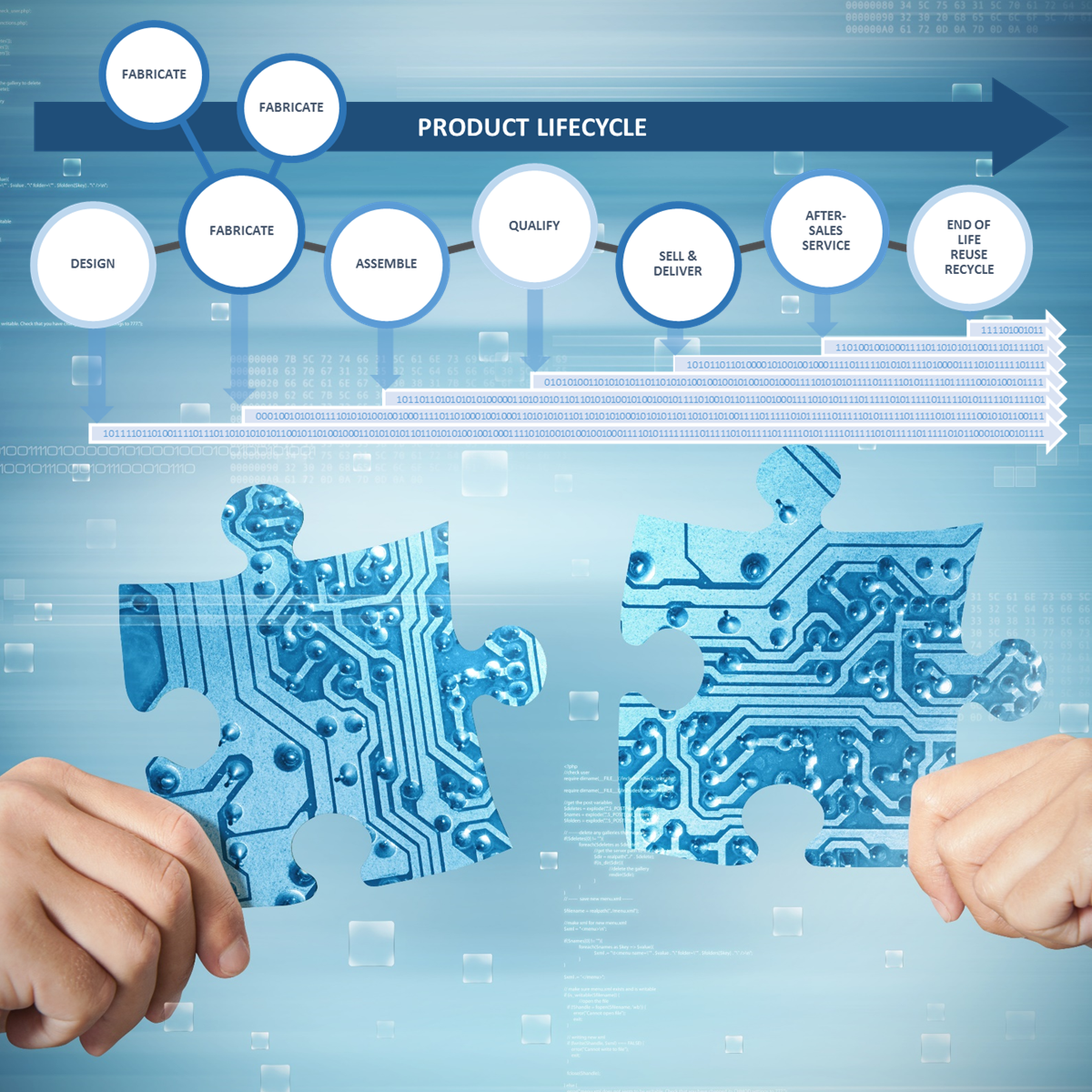
Digital Thread: Components
This course will help you recognize how the "digital thread" is the backbone of the digital manufacturing and design (DM&D) transformation, turning manufacturing processes from paper-based to digital-based. You will have a working understanding of the digital thread – the stream that starts at product concept and continues to accumulate information and data throughout the product’s life cycle – and identify opportunities to leverage it.
Gain an understanding of how "the right information, in the right place, at the right time" should flow. This is one of the keys to unlocking the potential of a digital design process. Acknowledging this will enable you to be more involved in a product’s development cycle, and to help a company become more flexible.
Main concepts of this course will be delivered through lectures, readings, discussions and various videos.
This is the second course in the Digital Manufacturing & Design Technology specialization that explores the many facets of manufacturing’s “Fourth Revolution,” aka Industry 4.0, and features a culminating project involving creation of a roadmap to achieve a self-established DMD-related professional goal. To learn more about the Digital Manufacturing and Design Technology specialization, please watch the overview video by copying and pasting the following link into your web browser: https://youtu.be/wETK1O9c-CA
Popular Internships and Jobs by Categories
Browse
© 2024 BoostGrad | All rights reserved