Back to Courses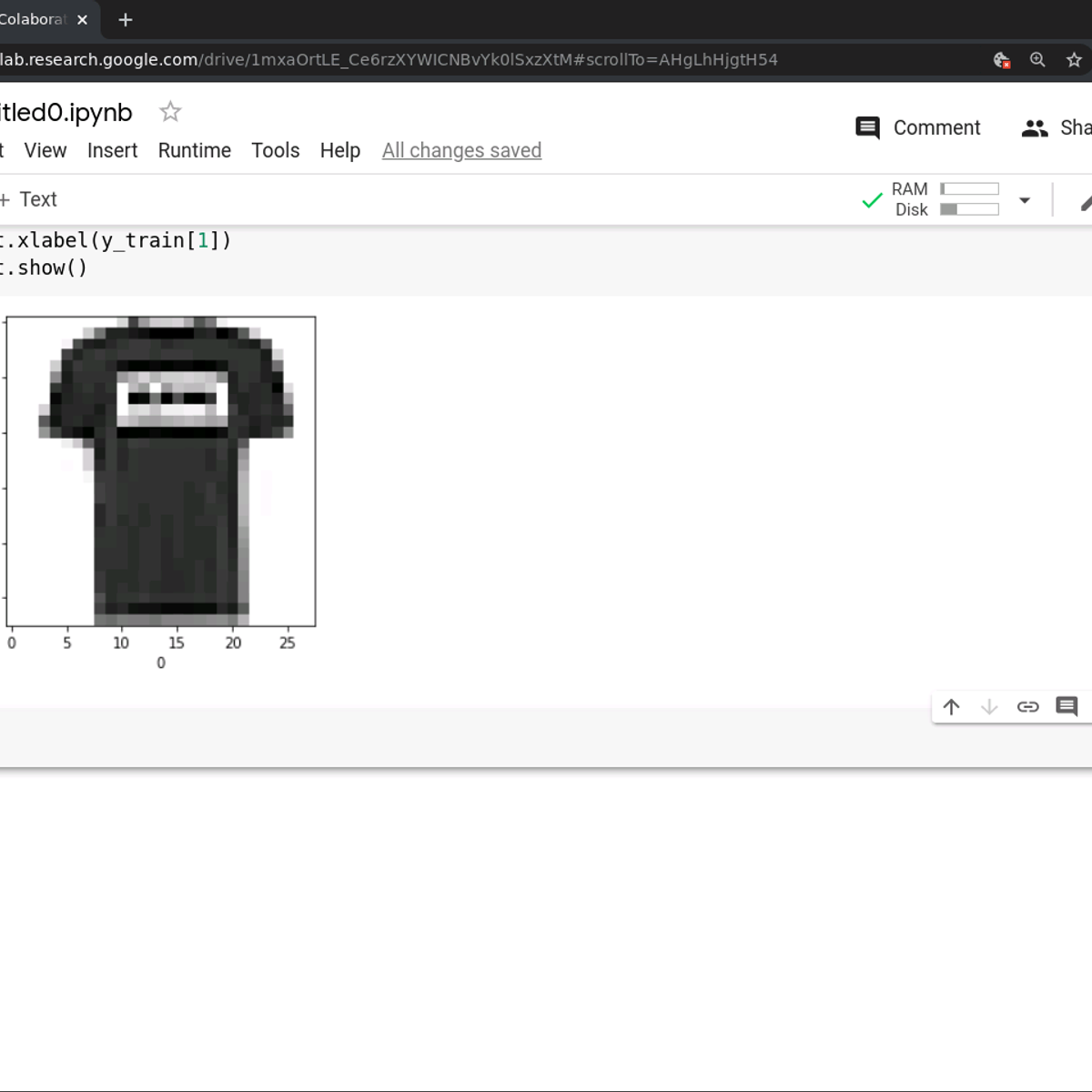
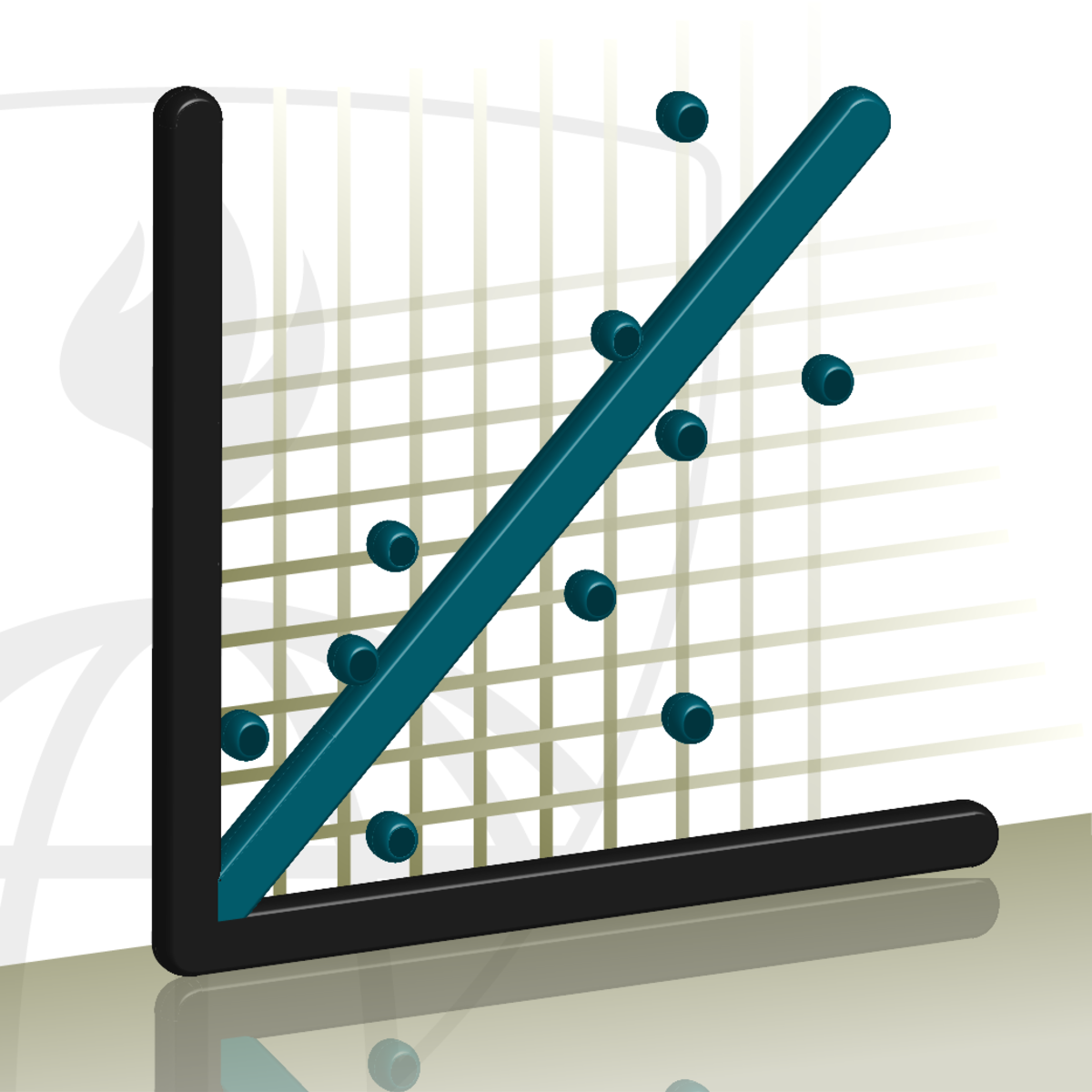
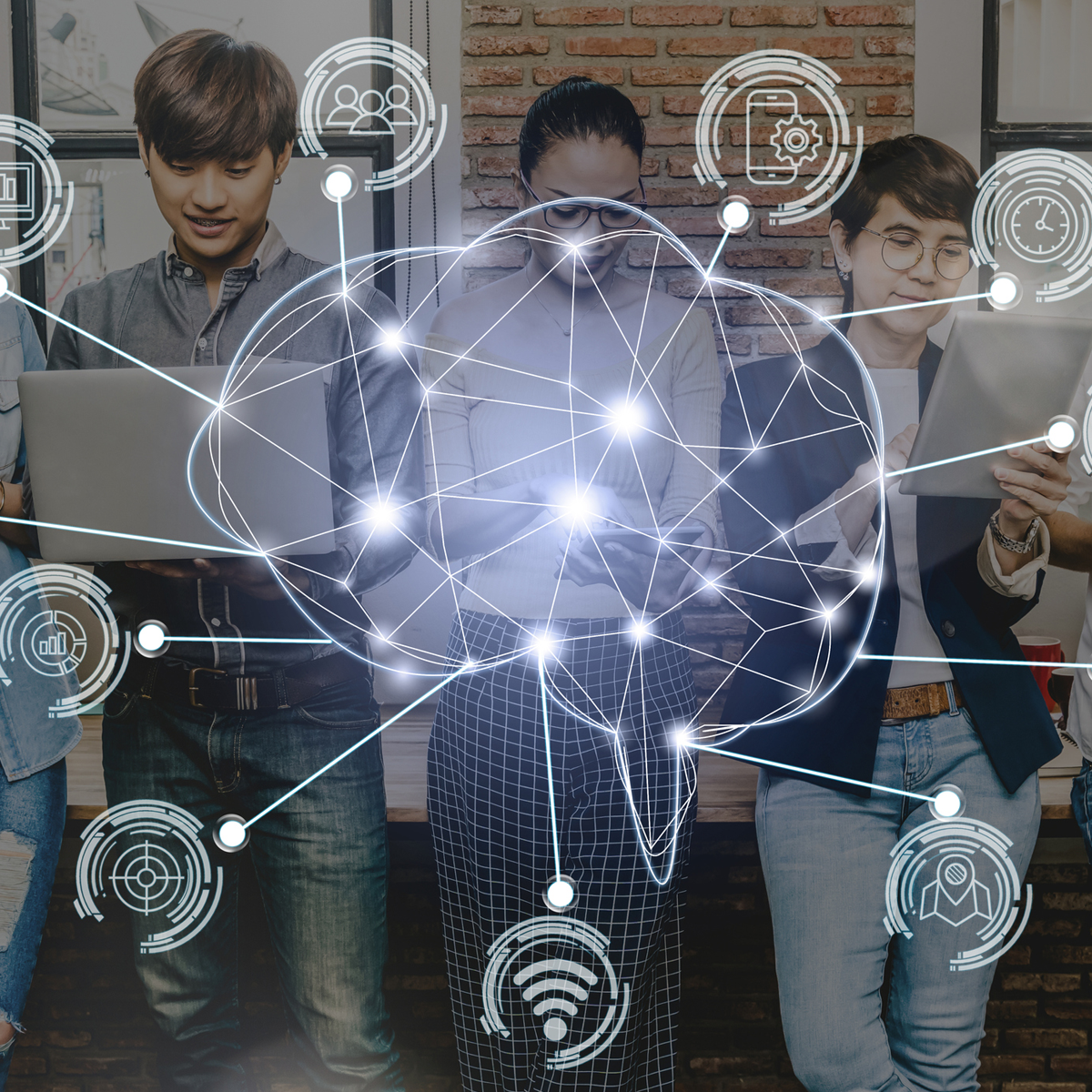
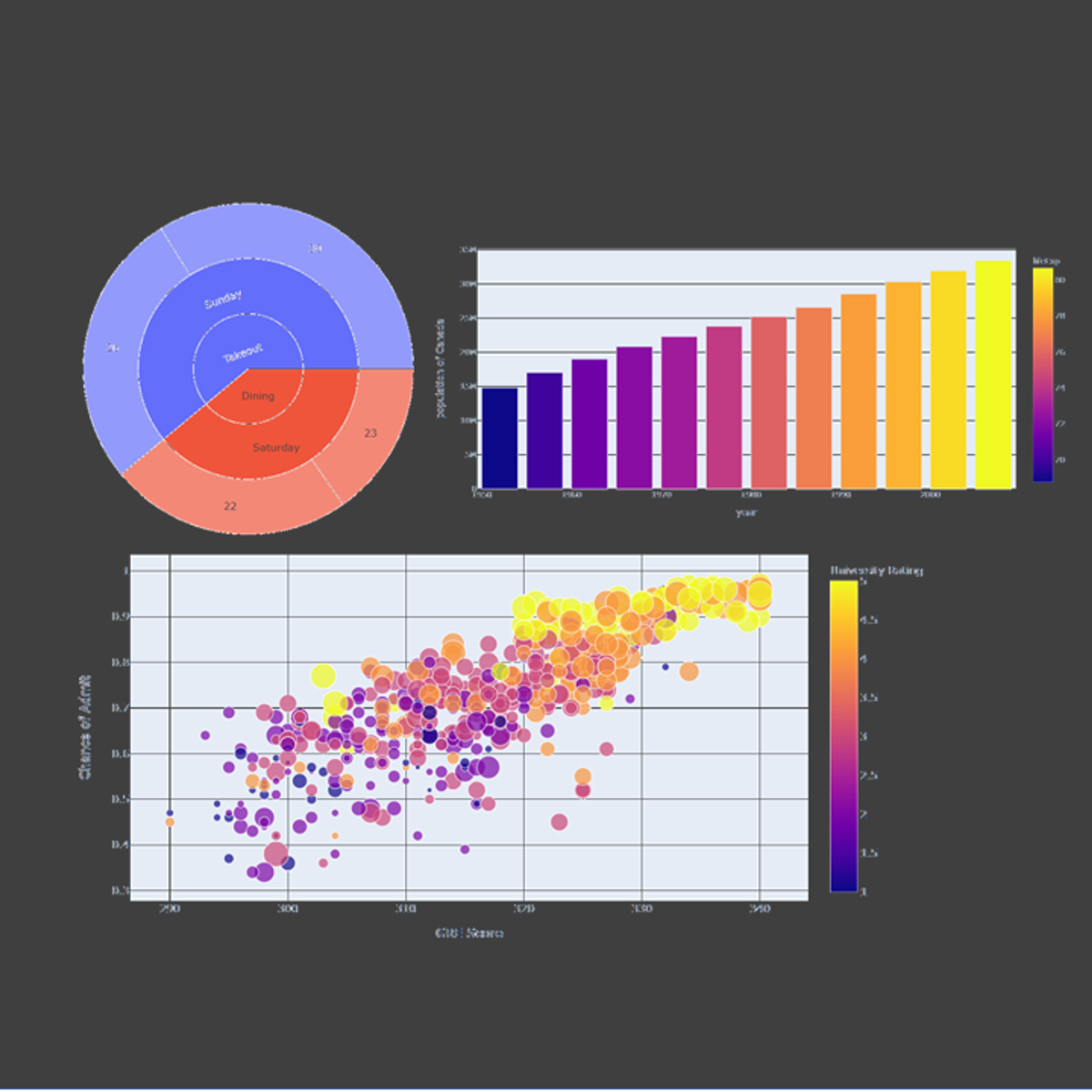
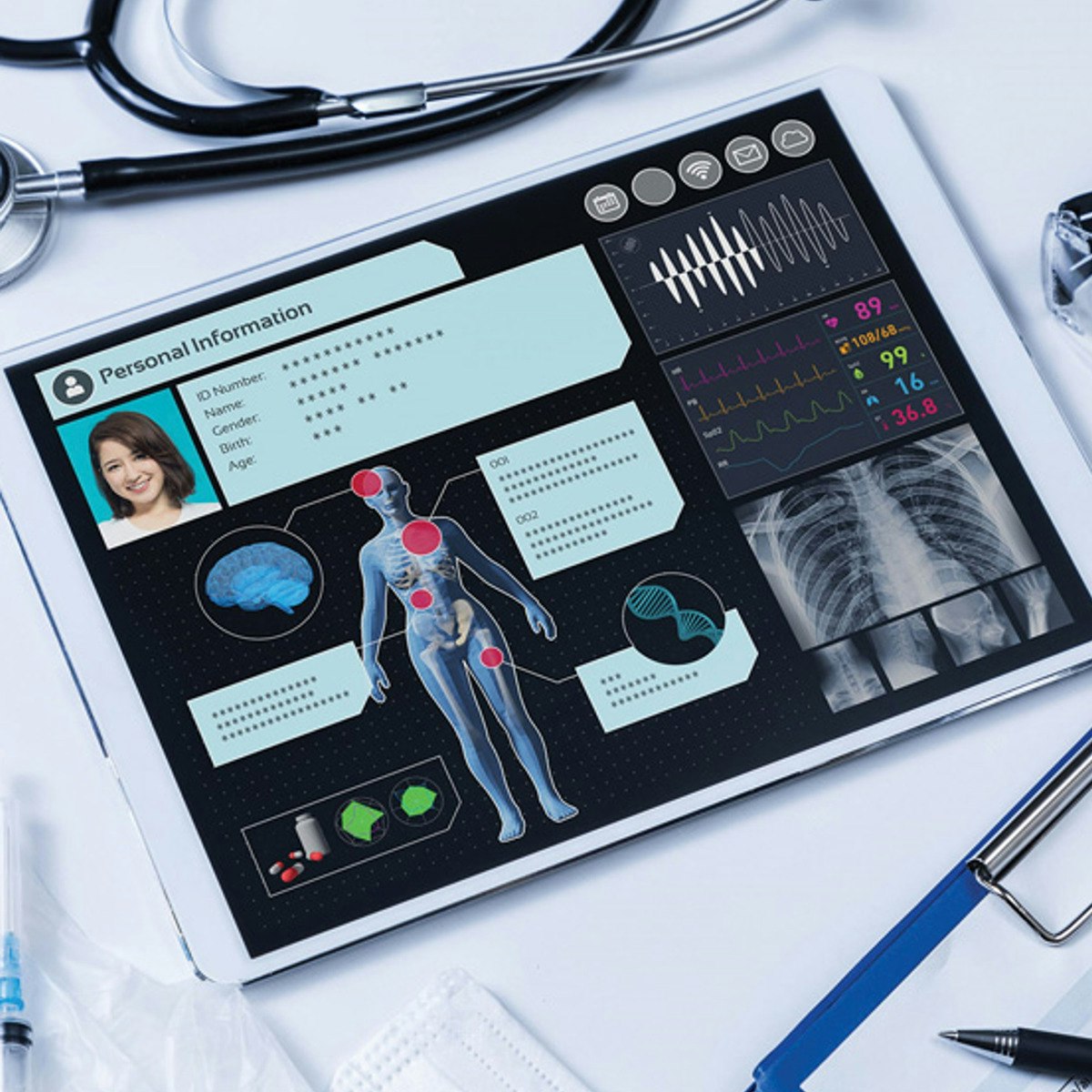
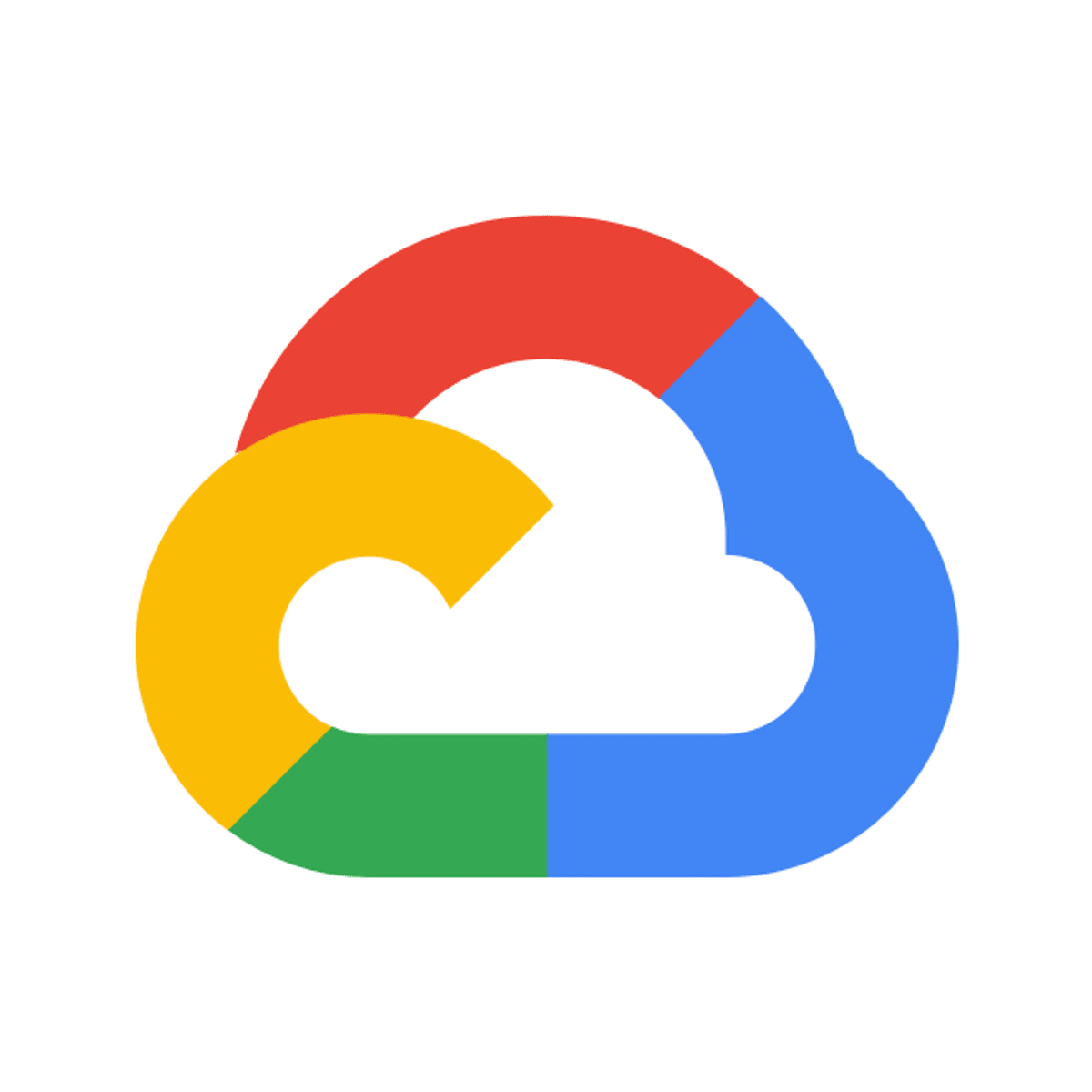
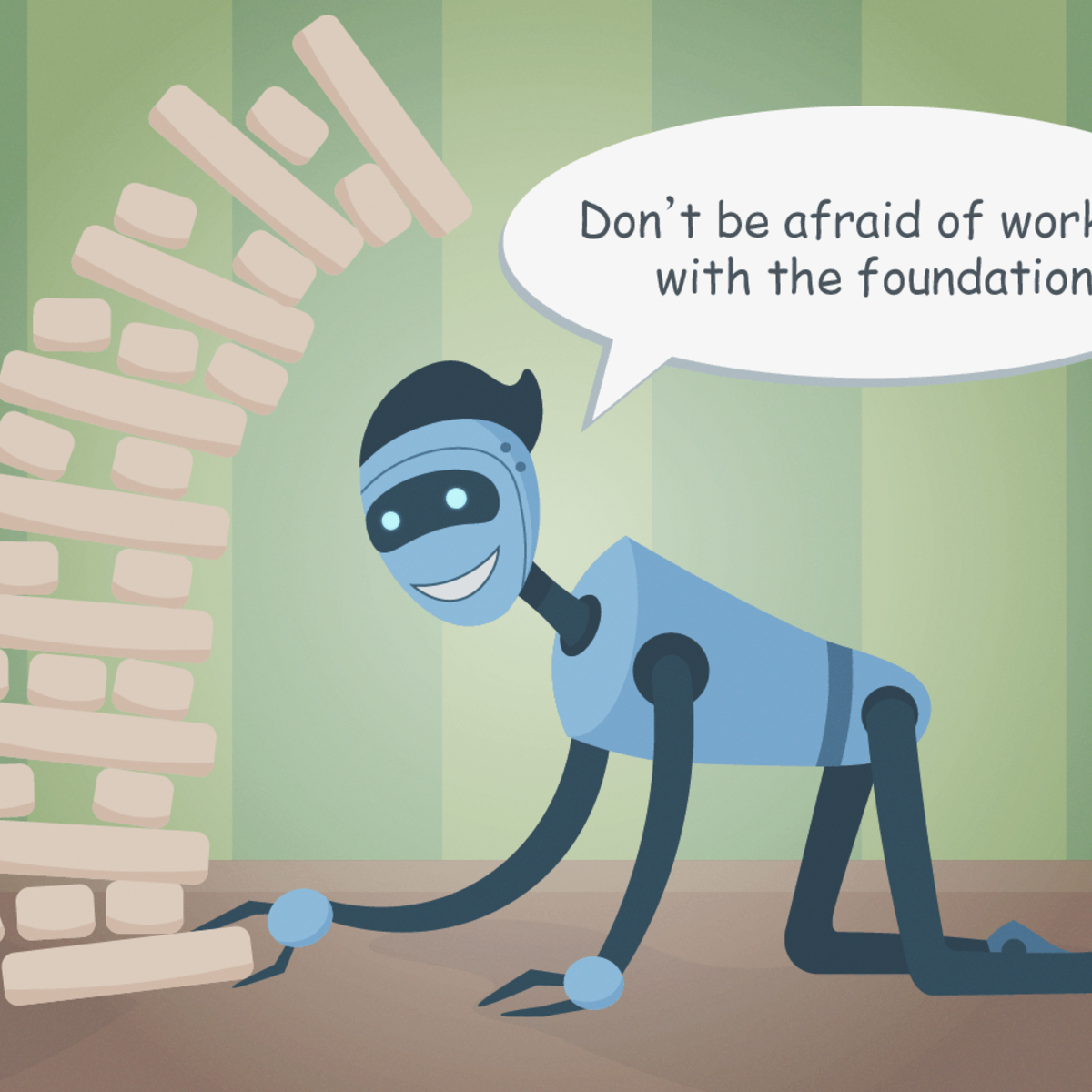
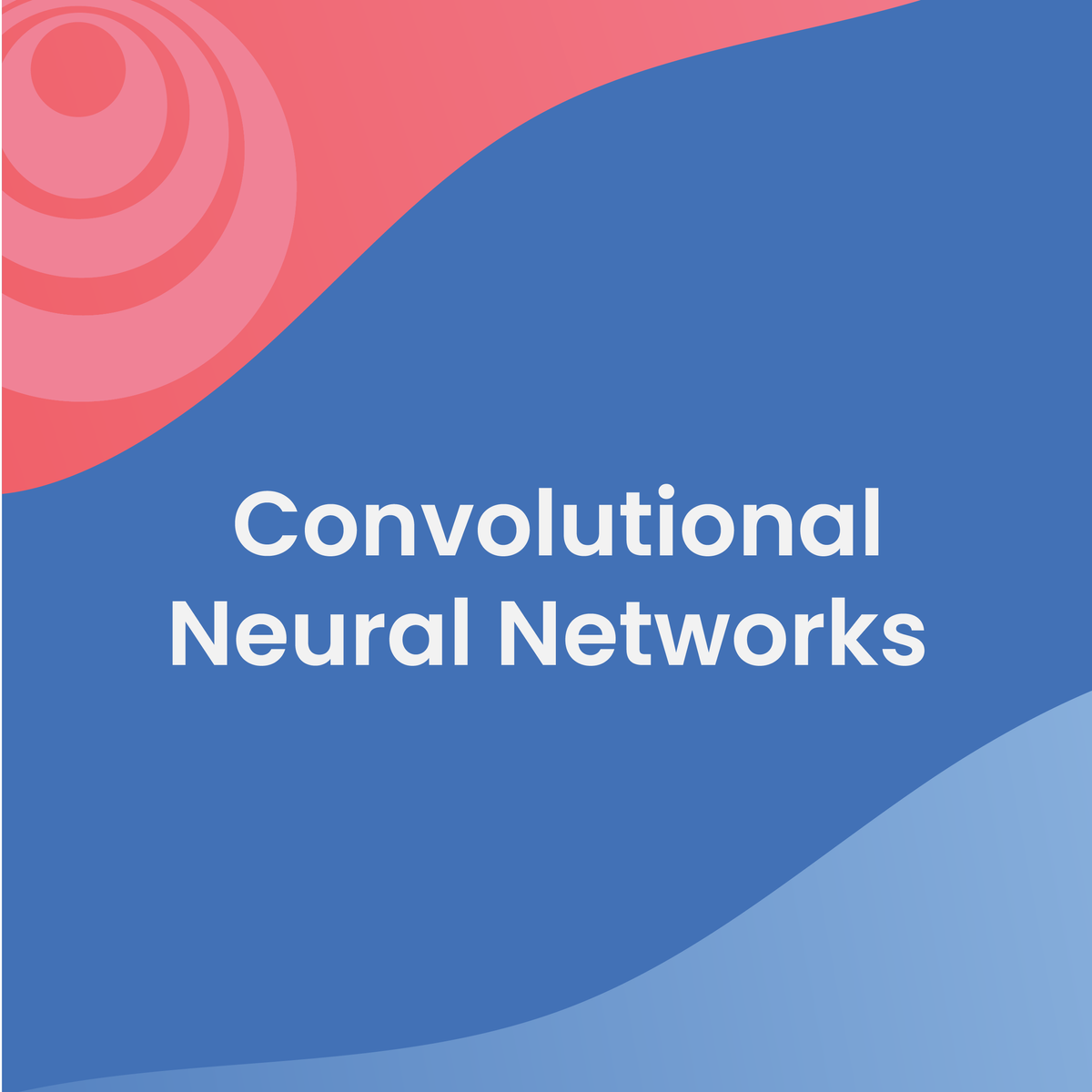
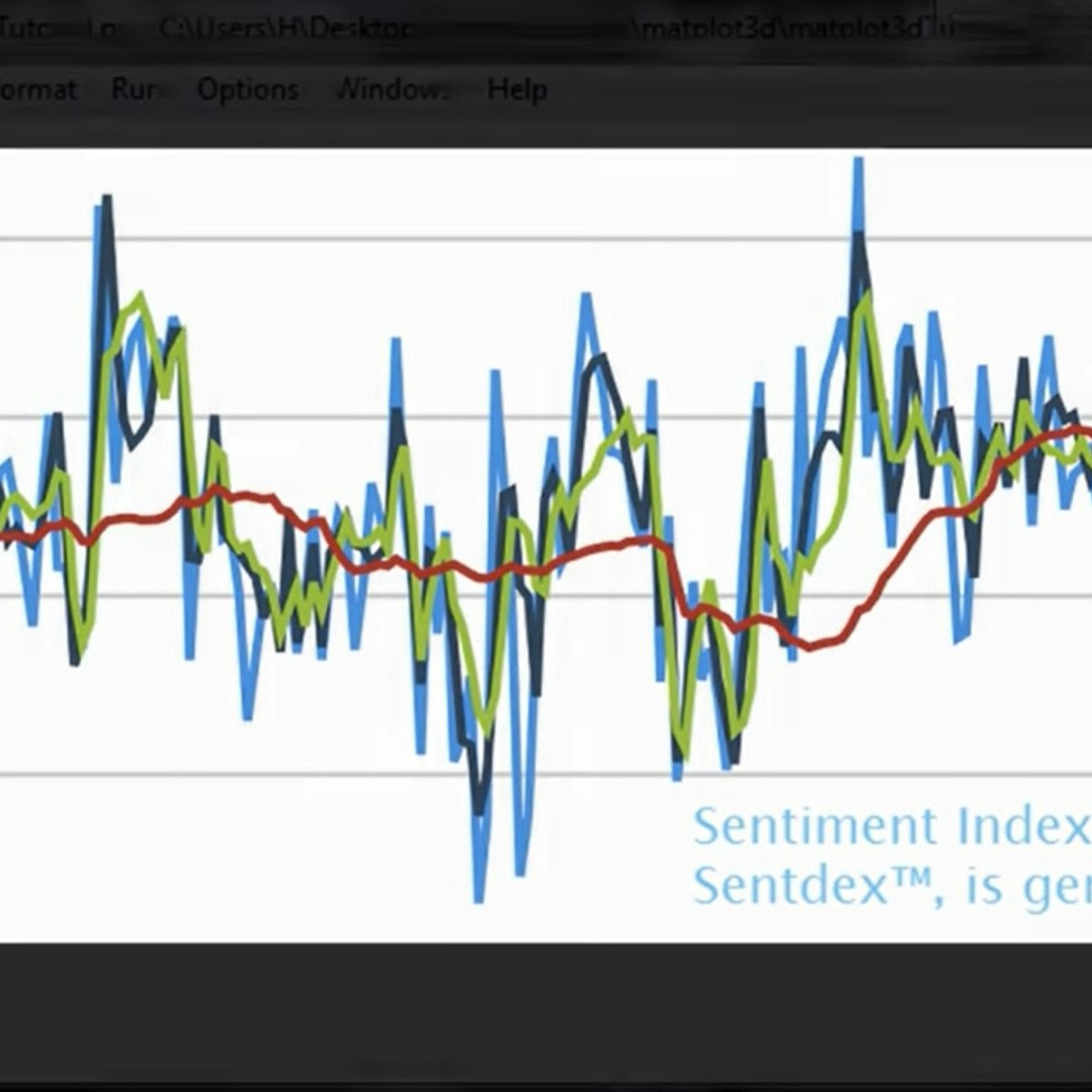
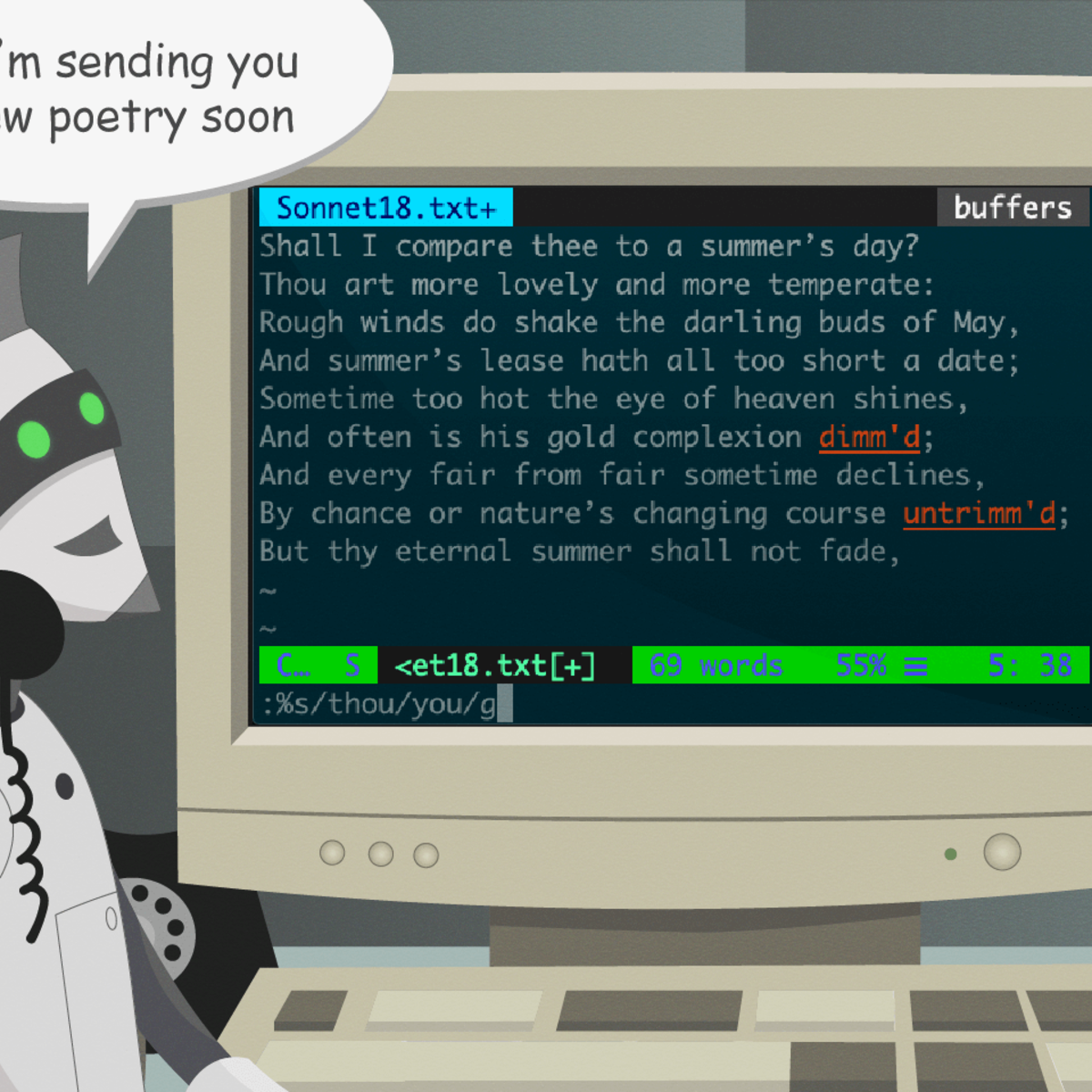
Data Science Courses - Page 81
Showing results 801-810 of 1407
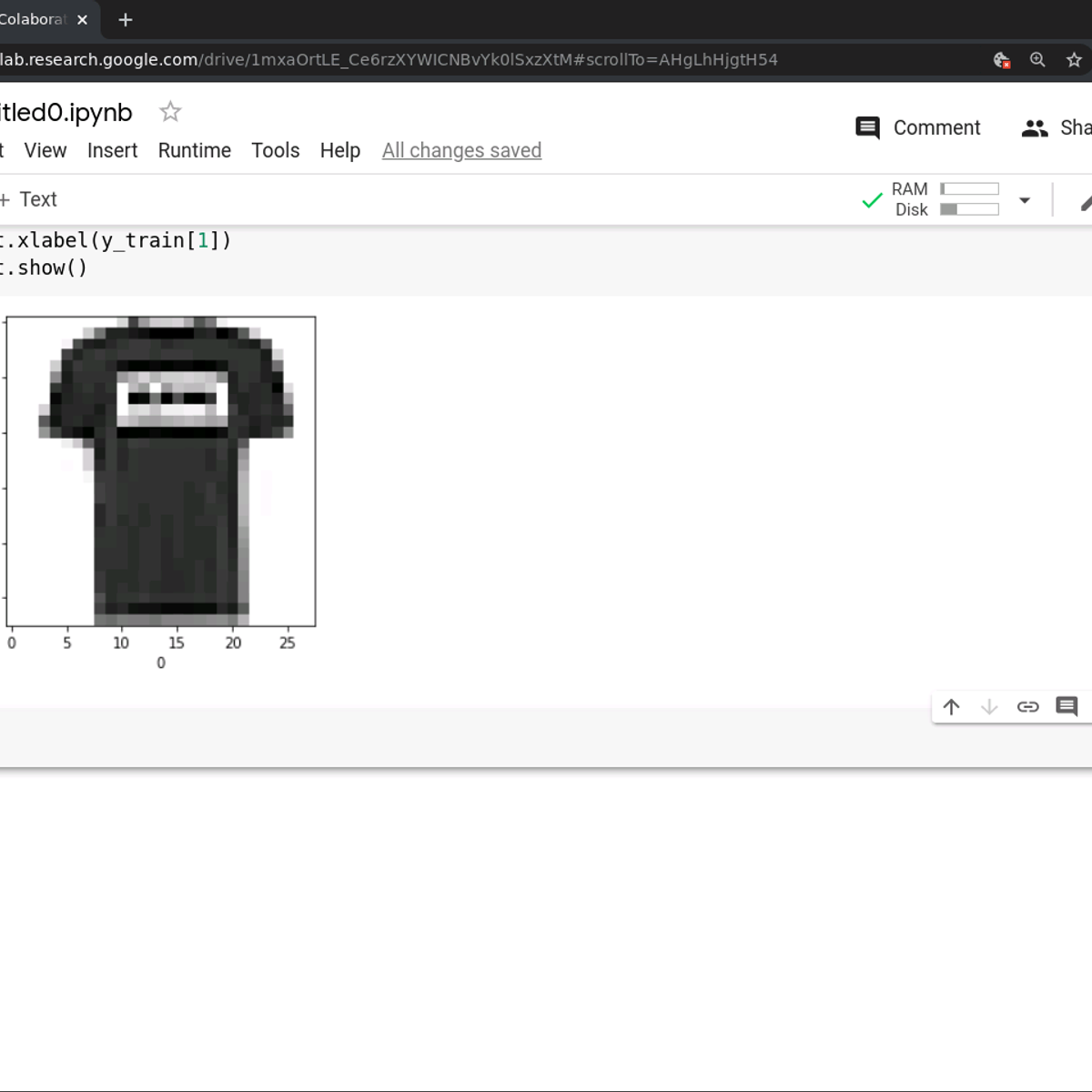
Hyperparameter Tuning with Keras Tuner
In this 2-hour long guided project, we will use Keras Tuner to find optimal hyperparamters for a Keras model. Keras Tuner is an open source package for Keras which can help machine learning practitioners automate Hyperparameter tuning tasks for their Keras models. The concepts learned in this project will apply across a variety of model architectures and problem scenarios. Please note that we are going to learn to use Keras Tuner for hyperparameter tuning, and are not going to implement the tuning algorithms ourselves. At the time of recording this project, Keras Tuner has a few tuning algorithms including Random Search, Bayesian Optimization and HyperBand.
In order to complete this project successfully, you will need prior programming experience in Python. This is a practical, hands on guided project for learners who already have theoretical understanding of Neural Networks, and optimization algorithms like gradient descent but want to understand how to use Keras Tuner to start optimizing hyperparameters for training their Keras models. You should also be familiar with the Keras API.
Note: This course works best for learners who are based in the North America region. We’re currently working on providing the same experience in other regions.
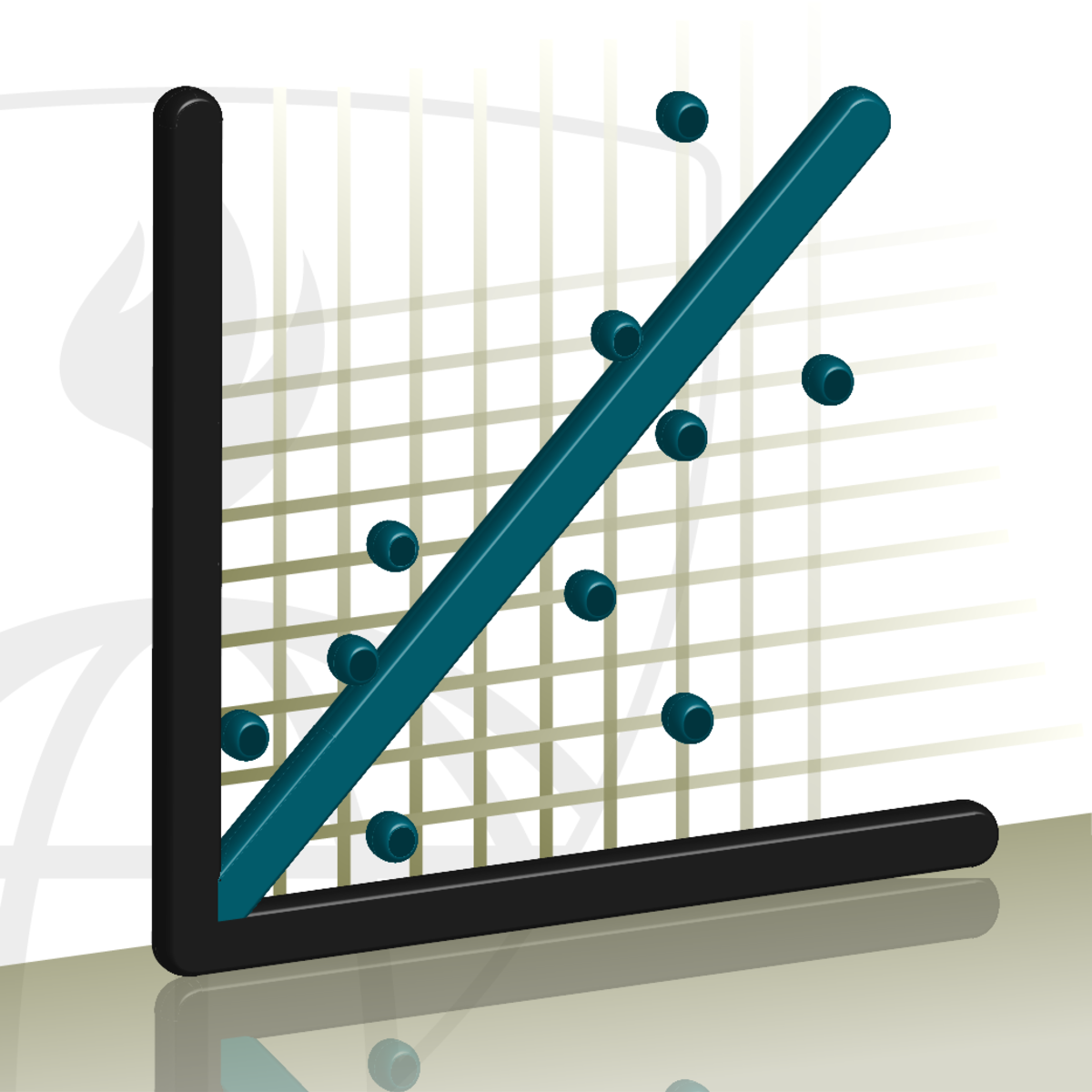
Simple Regression Analysis in Public Health
Biostatistics is the application of statistical reasoning to the life sciences, and it's the key to unlocking the data gathered by researchers and the evidence presented in the scientific public health literature. In this course, we'll focus on the use of simple regression methods to determine the relationship between an outcome of interest and a single predictor via a linear equation. Along the way, you'll be introduced to a variety of methods, and you'll practice interpreting data and performing calculations on real data from published studies. Topics include logistic regression, confidence intervals, p-values, Cox regression, confounding, adjustment, and effect modification.
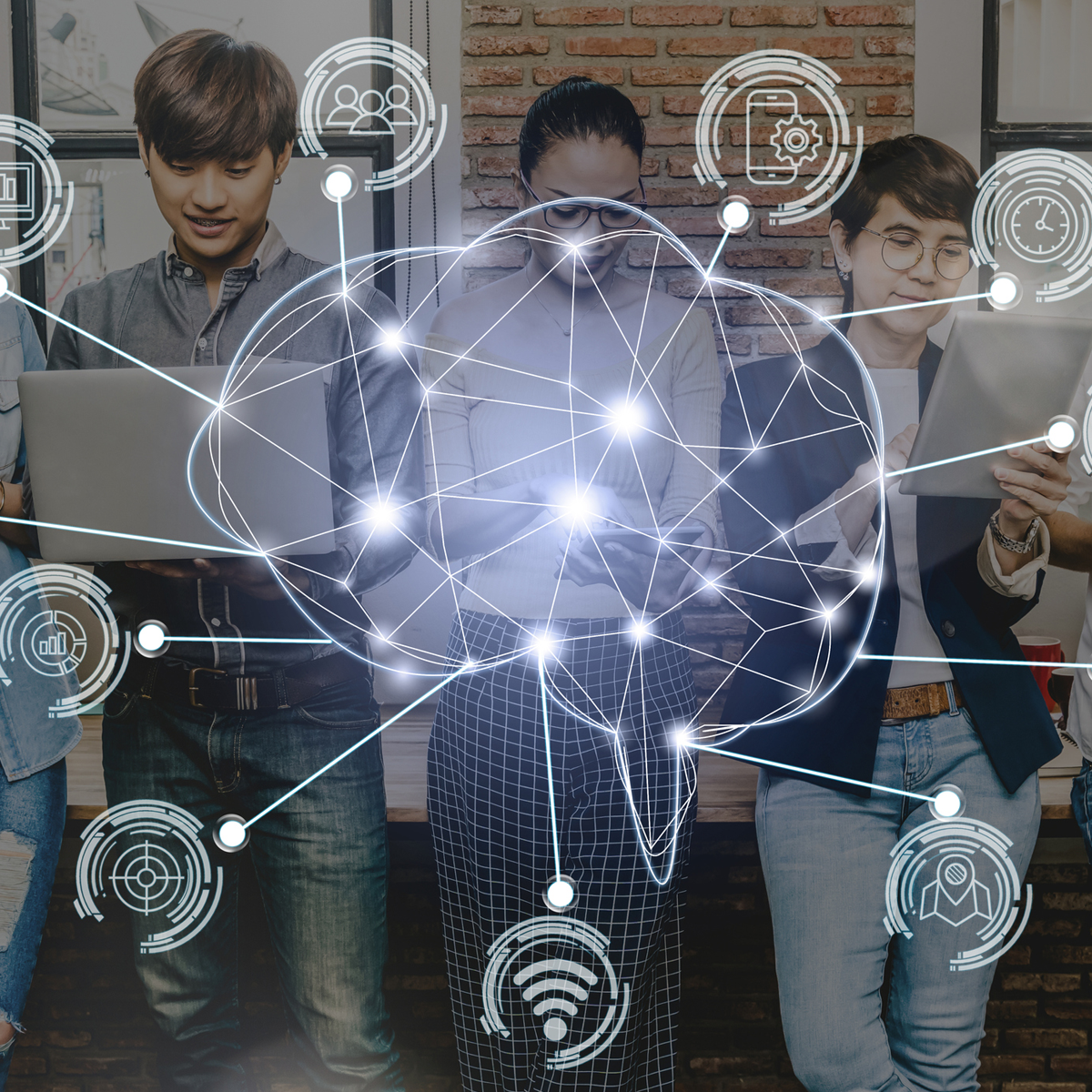
Computational Social Science Methods
This course gives you an overview of the current opportunities and the omnipresent reach of computational social science. The results are all around us, every day, reaching from the services provided by the world’s most valuable companies, over the hidden influence of governmental agencies, to the power of social and political movements. All of them study human behavior in order to shape it. In short, all of them do social science by computational means.
In this course we answer three questions:
I. Why Computational Social Science (CSS) now?
II. What does CSS cover?
III. What are examples of CSS?
In this last part, we take a bird’s-eye view on four main applications of CSS. First, Prof. Blumenstock from UC Berkeley discusses how we can gain insights by studying the massive digital footprint left behind today’s social interactions, especially to foster international development. Second, Prof. Shelton from UC Riverside introduces us to the world of machine learning, including the basic concepts behind this current driver of much of today's computational landscape. Prof. Fowler, from UC San Diego introduces us to the power of social networks, and finally, Prof. Smaldino, from UC Merced, explains how computer simulation help us to untangle some of the mysteries of social emergence.
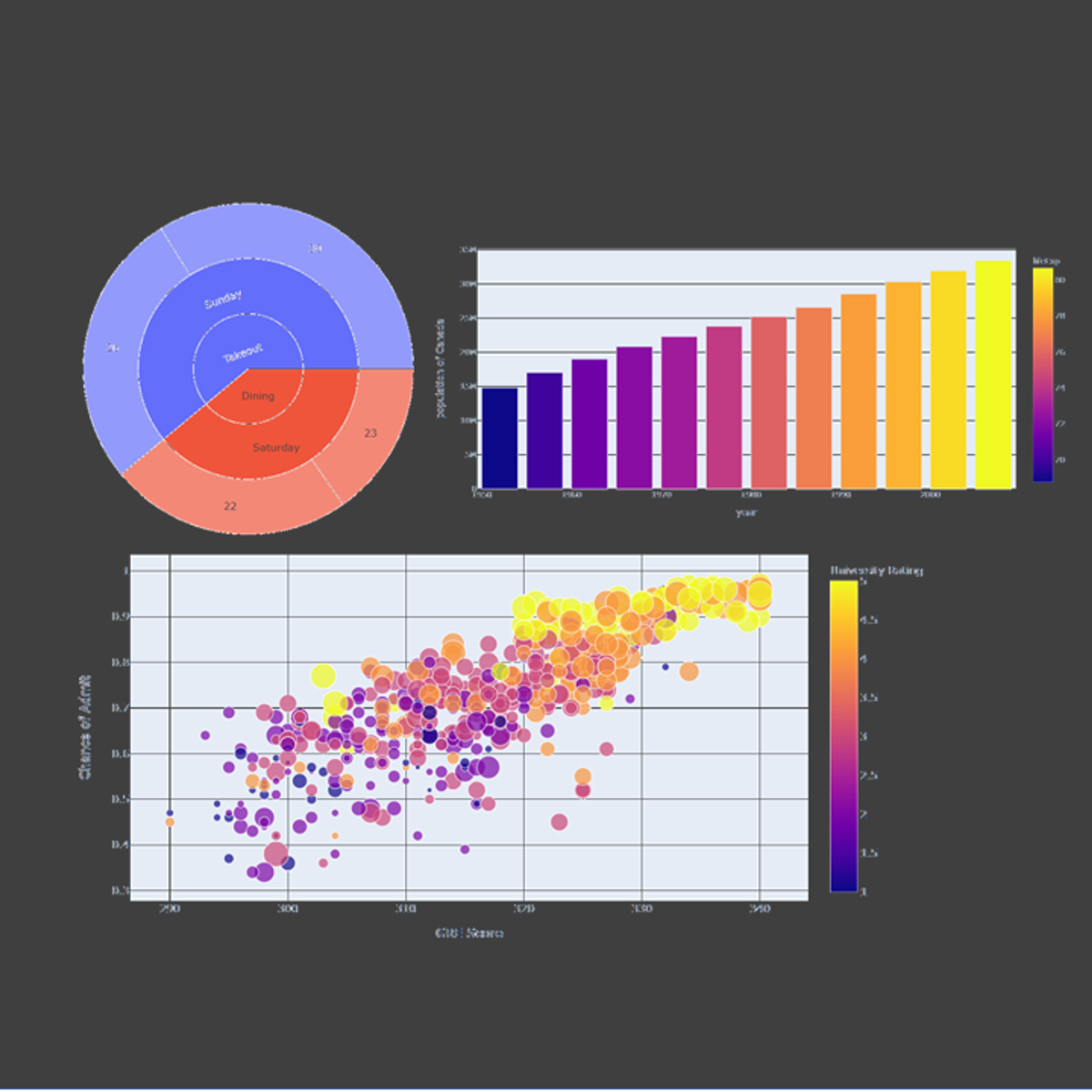
Crash Course on Interactive Data Visualization with Plotly
In this hands-on project, we will understand the fundamentals of interactive data visualization using Plolty Express. Plotly Express is a powerful Python package that empowers anyone to create, manipulate and render graphical figures with very few lines of code. Plotly Express is the recommended entry-point into the plotly package. We will leverage Plotly Express to generate interactive single Line plots, multiple line plots, histograms, pie charts, scatterplots, bubble charts, and bar charts.
Note: This course works best for learners who are based in the North America region. We’re currently working on providing the same experience in other regions.
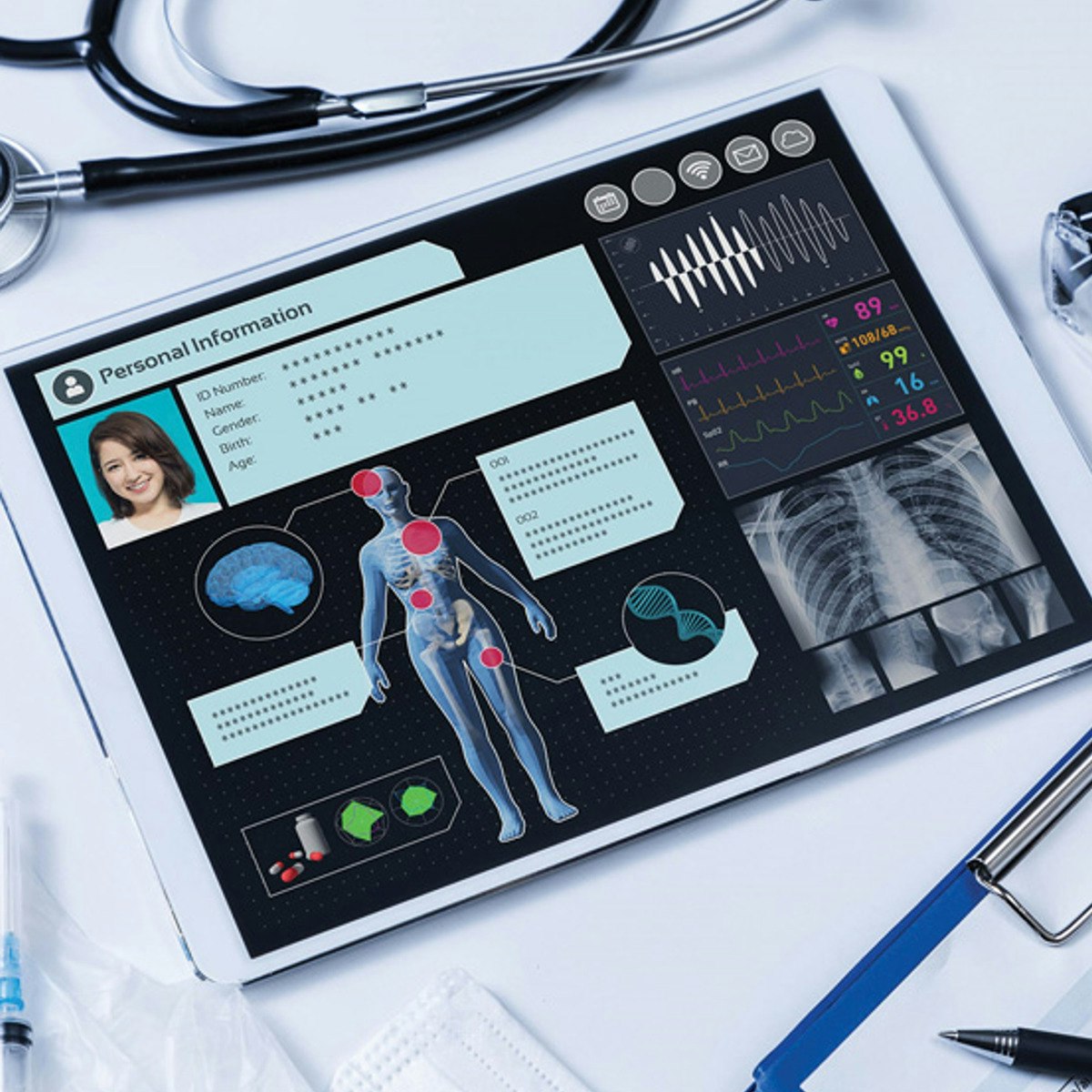
Explainable deep learning models for healthcare - CDSS 3
This course will introduce the concepts of interpretability and explainability in machine learning applications. The learner will understand the difference between global, local, model-agnostic and model-specific explanations. State-of-the-art explainability methods such as Permutation Feature Importance (PFI), Local Interpretable Model-agnostic Explanations (LIME) and SHapley Additive exPlanation (SHAP) are explained and applied in time-series classification. Subsequently, model-specific explanations such as Class-Activation Mapping (CAM) and Gradient-Weighted CAM are explained and implemented. The learners will understand axiomatic attributions and why they are important. Finally, attention mechanisms are going to be incorporated after Recurrent Layers and the attention weights will be visualised to produce local explanations of the model.
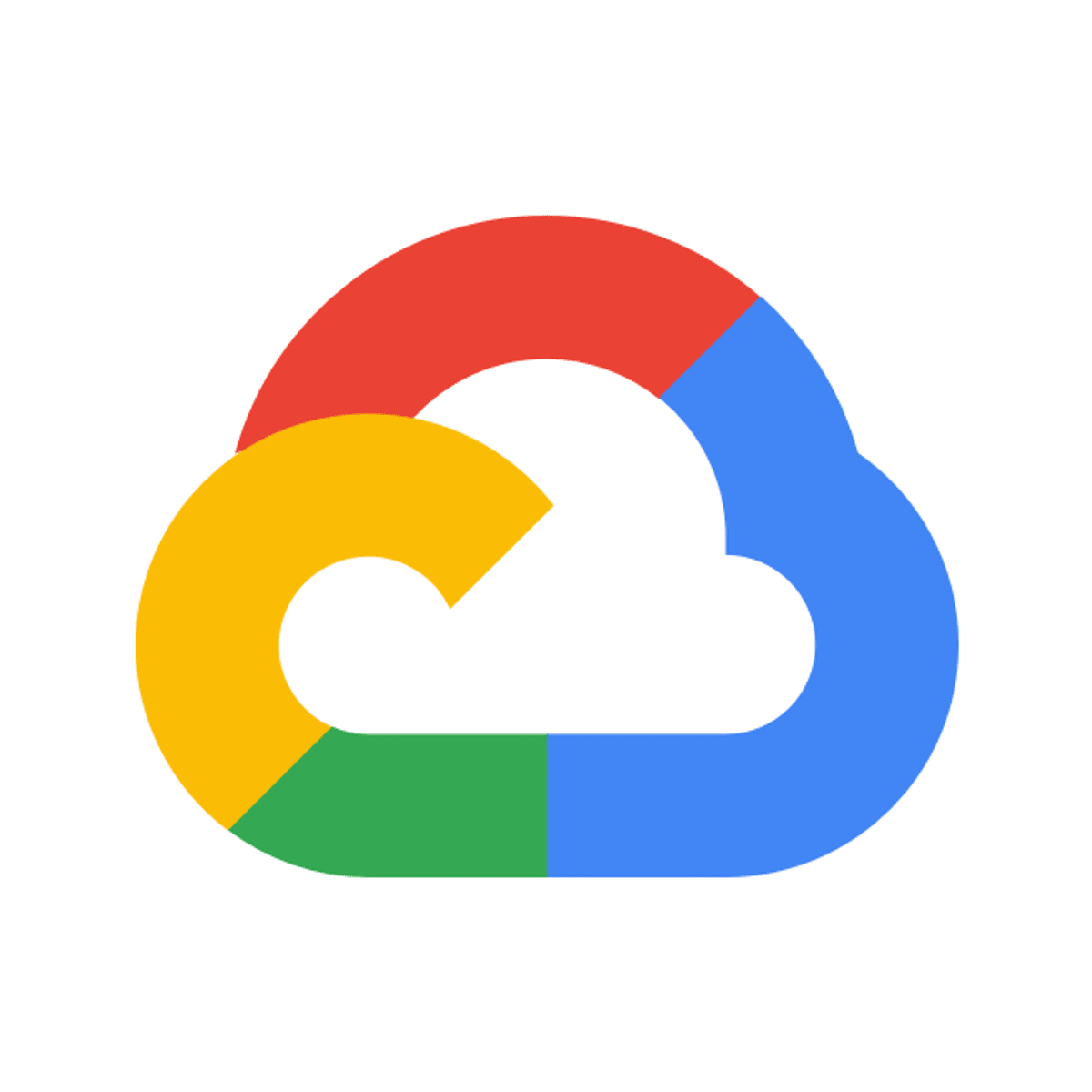
Cloud Operations for GKE
This is a self-paced lab that takes place in the Google Cloud console. In this lab you will set up Monitoring and visualizing metrics from a Kubernetes Engine cluster
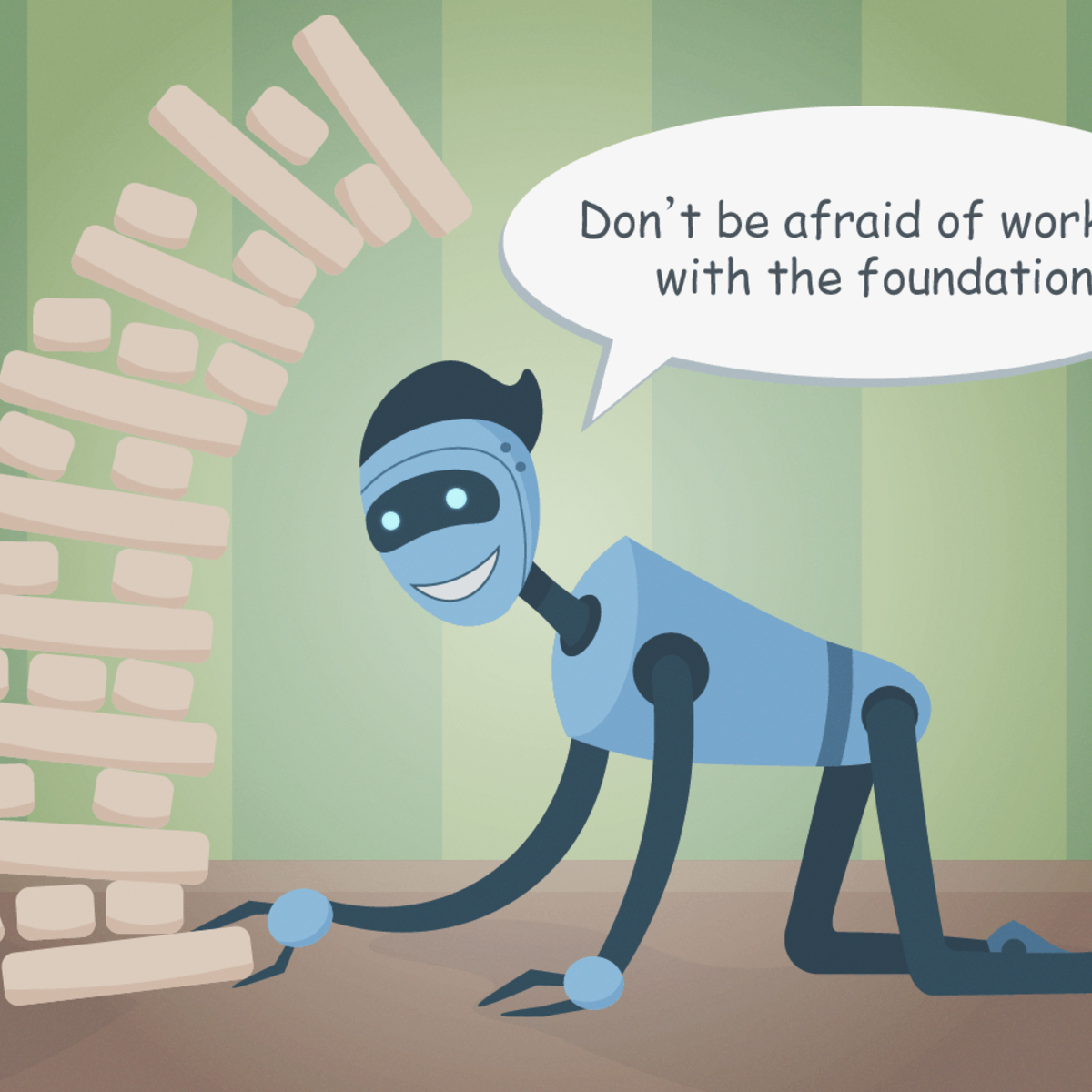
Neural Network from Scratch in TensorFlow
In this 2-hours long project-based course, you will learn how to implement a Neural Network model in TensorFlow using its core functionality (i.e. without the help of a high level API like Keras). You will also implement the gradient descent algorithm with the help of TensorFlow's automatic differentiation. While it’s easier to get started with TensorFlow with the Keras API, it’s still worth understanding how a slightly lower level implementation might work in tensorflow, and this project will give you a great starting point.
In order to be successful in this project, you should be familiar with python programming, TensorFlow basics, conceptual understanding of Neural Networks and gradient descent.
Note: This course works best for learners who are based in the North America region. We’re currently working on providing the same experience in other regions.
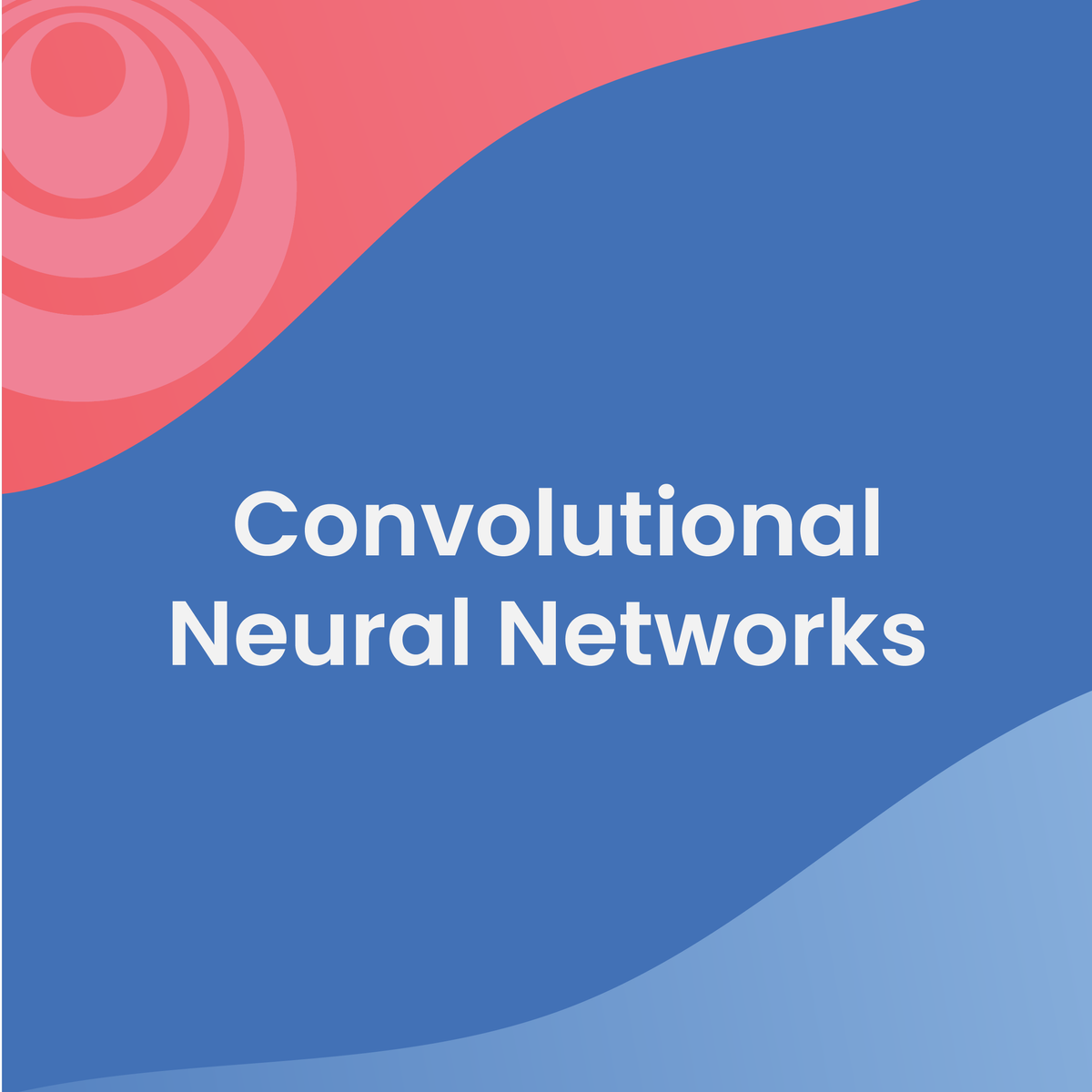
Convolutional Neural Networks
In the fourth course of the Deep Learning Specialization, you will understand how computer vision has evolved and become familiar with its exciting applications such as autonomous driving, face recognition, reading radiology images, and more.
By the end, you will be able to build a convolutional neural network, including recent variations such as residual networks; apply convolutional networks to visual detection and recognition tasks; and use neural style transfer to generate art and apply these algorithms to a variety of image, video, and other 2D or 3D data.
The Deep Learning Specialization is our foundational program that will help you understand the capabilities, challenges, and consequences of deep learning and prepare you to participate in the development of leading-edge AI technology. It provides a pathway for you to gain the knowledge and skills to apply machine learning to your work, level up your technical career, and take the definitive step in the world of AI.
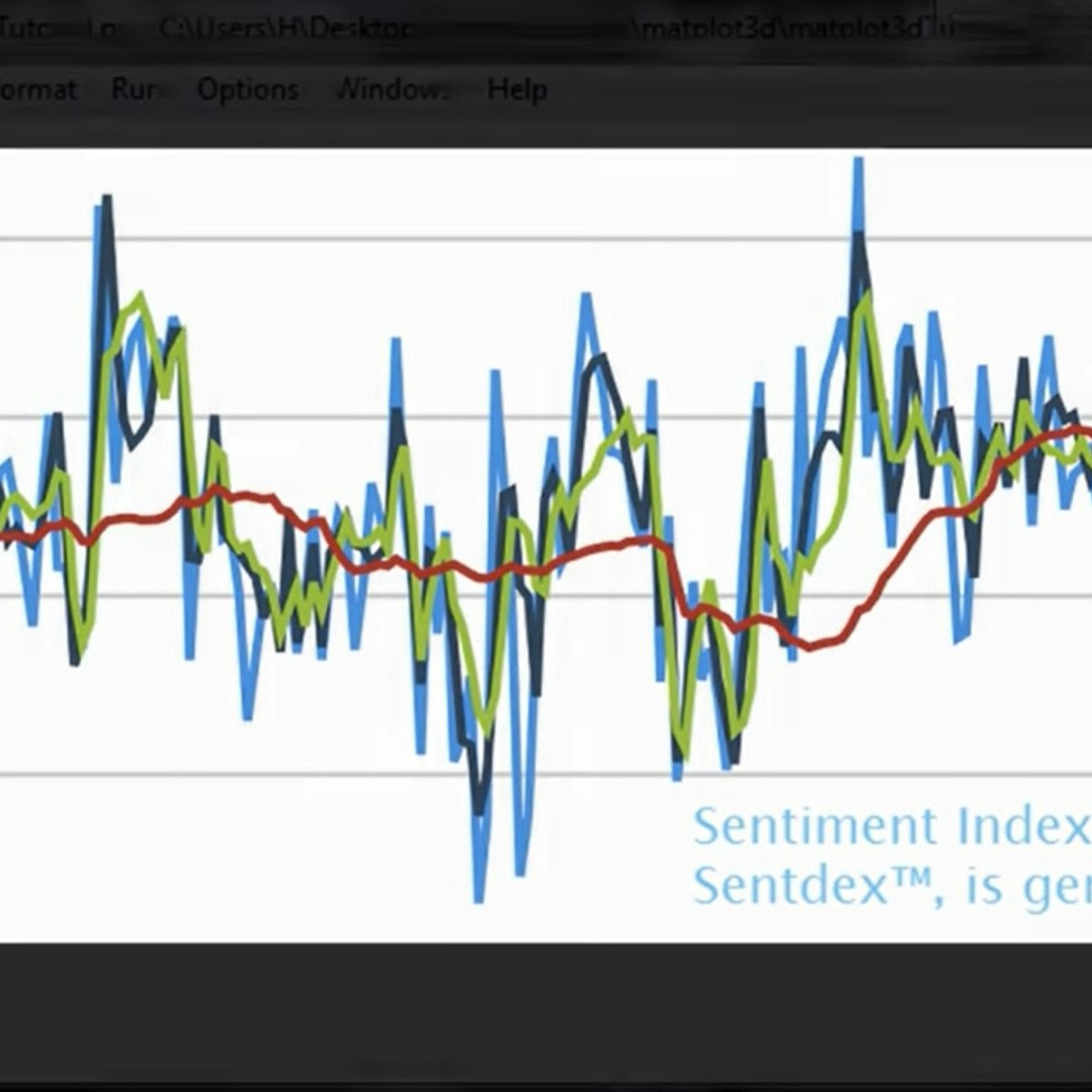
Scatter Plot for Data Scientists & Big Data Analysts-Visuals
This project gives you easy access to the invaluable learning techniques used by experts for visualization in statistics. We’ll learn about how to use wolfram language to draw curve in easiest way. We’ll also cover illustration and best practices shown by research to be most effective in helping you master plotting curves.
Using these approaches, no matter what your skill levels in topics you would like to master, you can change your thinking and change your life. If you’re already an expert, this peep under the mental hood will give you ideas for turbocharging successful learning, including counter-intuitive test-taking tips and insights that will help you make the best use of your time on homework and problem sets. If you’re struggling, you’ll see a structured treasure trove of practical techniques that walk you through what you need to do to get on track. If you’ve ever wanted to become better at anything, this project will help serve as your guide.
In this project we will take some illustrations and be able to Visualize the data by Scatter Plot using Wolfram Mathematica.
By the end of this project learners will:
Be able to plot basic examples (list of y values and x y pair)& several data items with legends (labeling each Plot and each data item)
Be able to plot values including 'units' and using individual 'color' for each point
Be able to plot the range where the data is non real are excluded & function ranges where it is selected automatically.
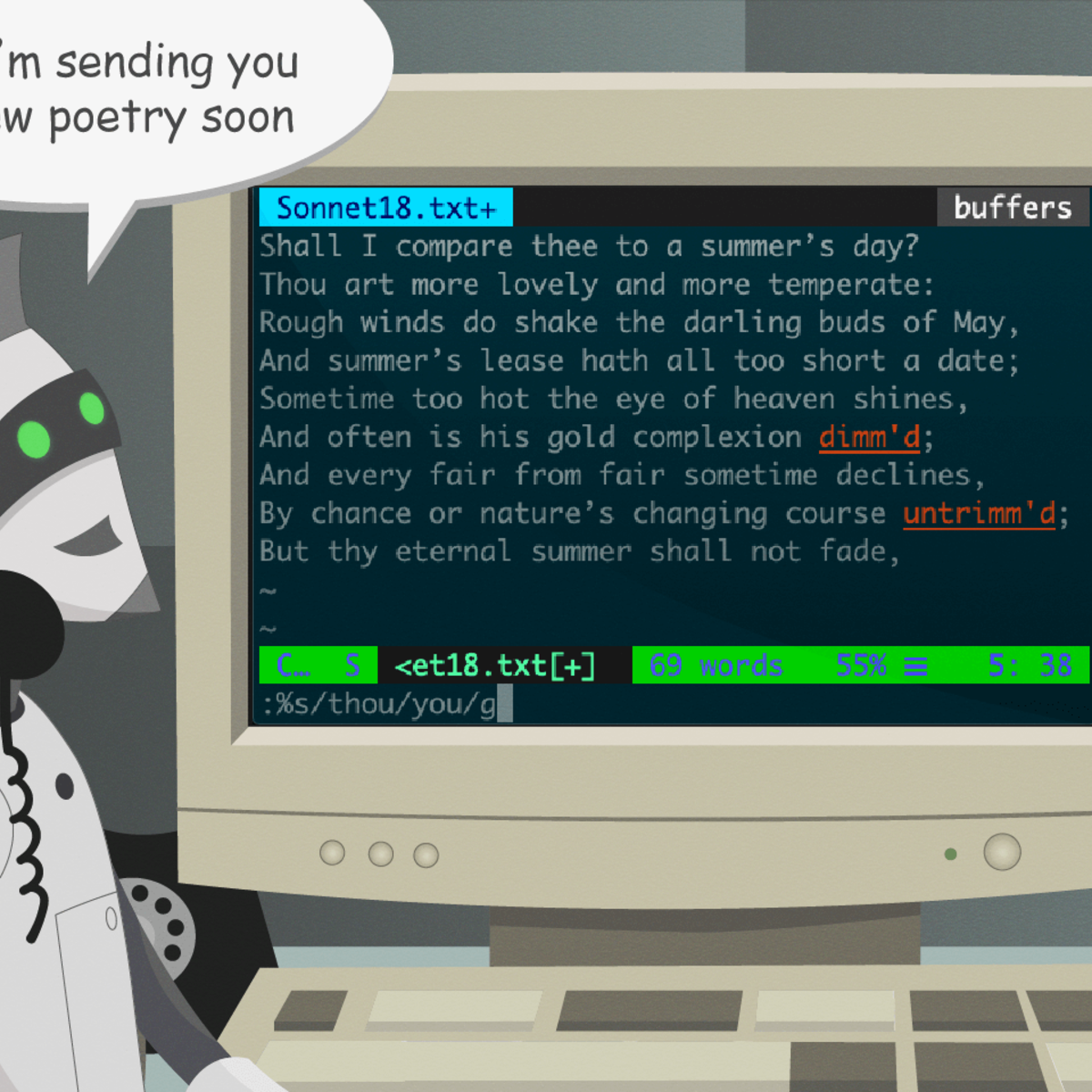
Analyze Text Data with Yellowbrick
Welcome to this project-based course on Analyzing Text Data with Yellowbrick. Tasks such as assessing document similarity, topic modelling and other text mining endeavors are predicated on the notion of "closeness" or "similarity" between documents. In this course, we define various distance metrics (e.g. Euclidean, Hamming, Cosine, Manhattan, etc) and understand their merits and shortcomings as they relate to document similarity. We will apply these metrics on documents within a specific corpus and visualize our results. By the end of this course, you will be able to confidently use visual diagnostic tools from Yellowbrick to steer your machine learning workflow, vectorize text data using TF-IDF, and cluster documents using embedding techniques and appropriate metrics.
This course runs on Coursera's hands-on project platform called Rhyme. On Rhyme, you do projects in a hands-on manner in your browser. You will get instant access to pre-configured cloud desktops containing all of the software and data you need for the project. Everything is already set up directly in your internet browser so you can just focus on learning. For this project, you’ll get instant access to a cloud desktop with Python, Jupyter, Yellowbrick, and scikit-learn pre-installed.
Notes:
- You will be able to access the cloud desktop 5 times. However, you will be able to access instructions videos as many times as you want.
- This course works best for learners who are based in the North America region. We’re currently working on providing the same experience in other regions.
Popular Internships and Jobs by Categories
Browse
© 2024 BoostGrad | All rights reserved