Back to Courses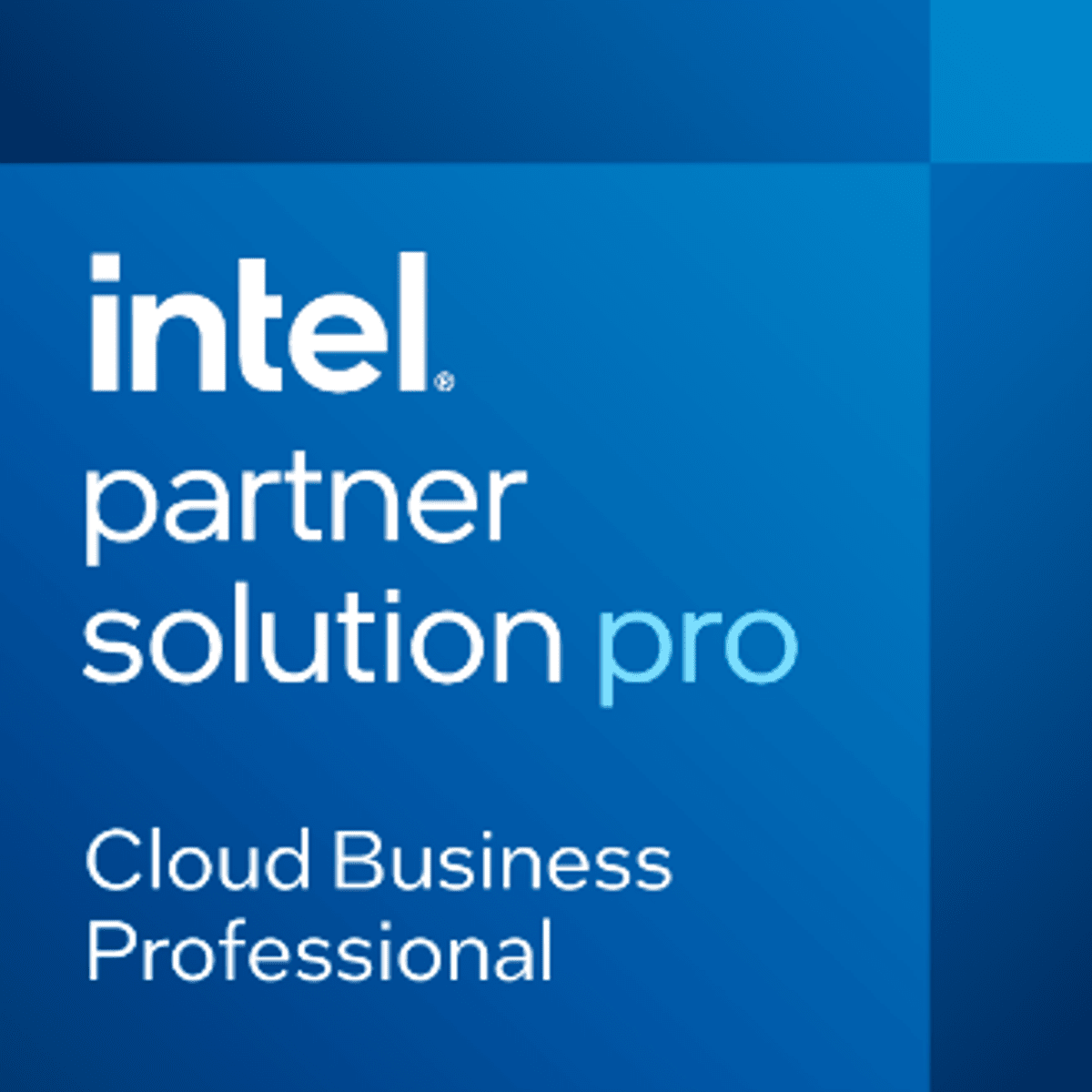
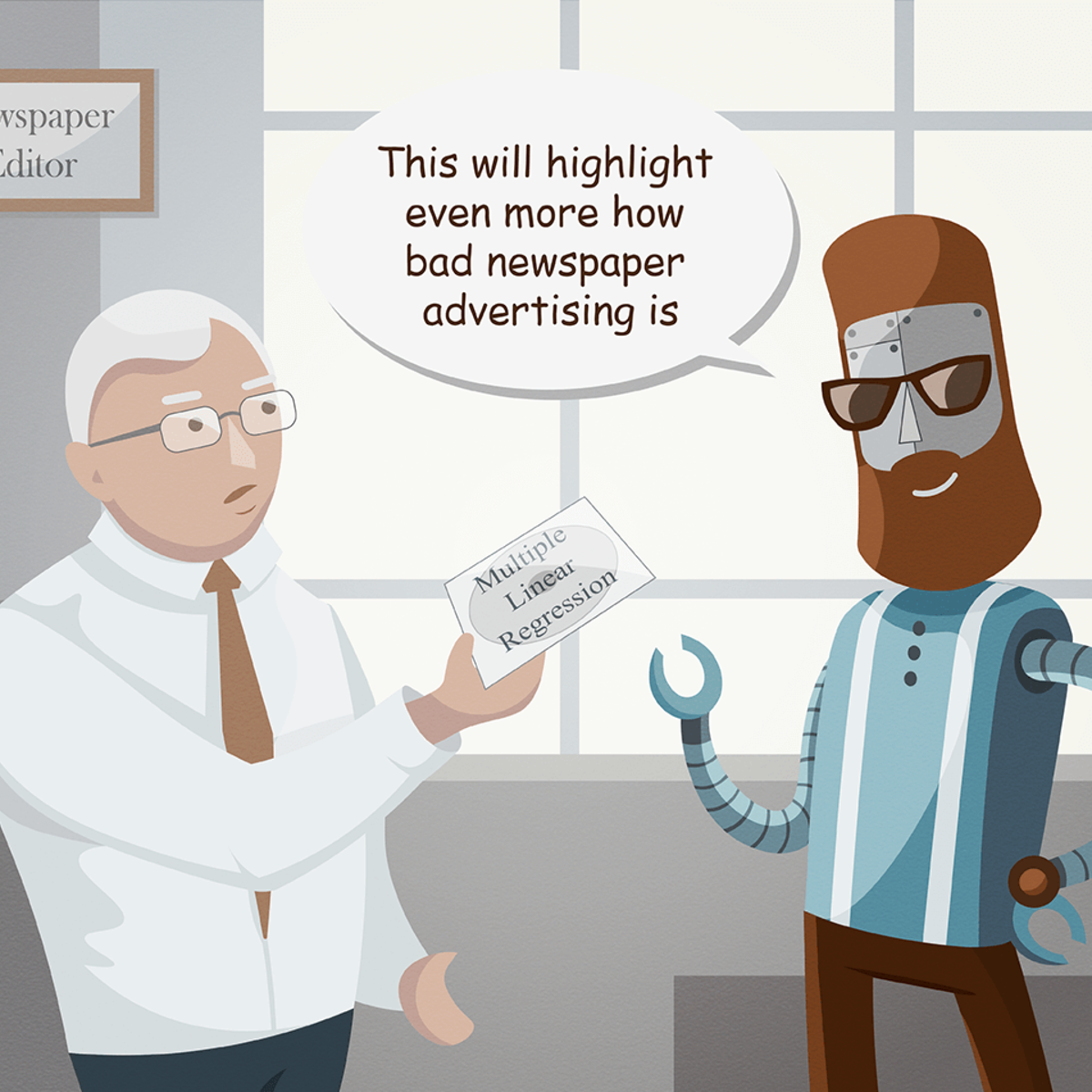
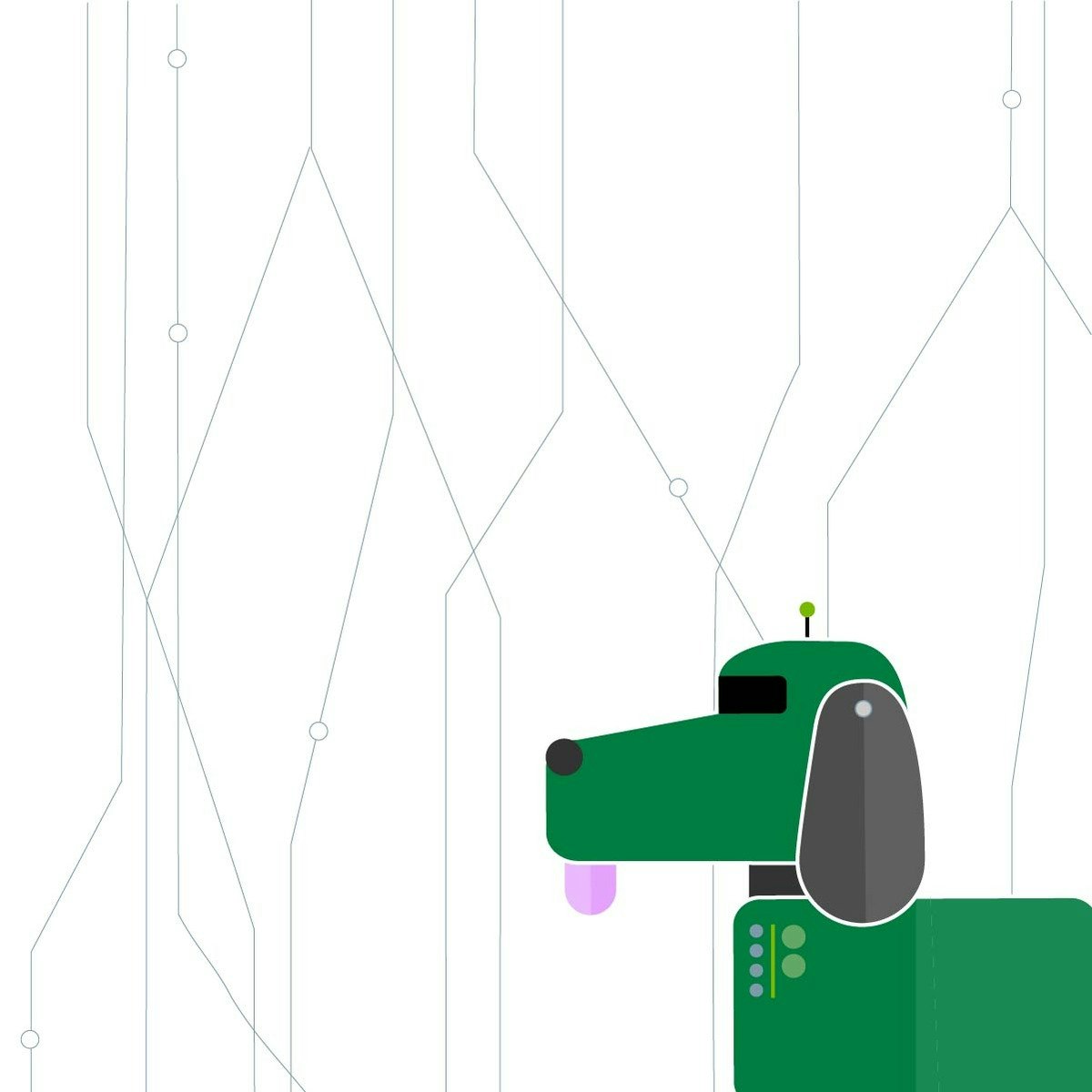
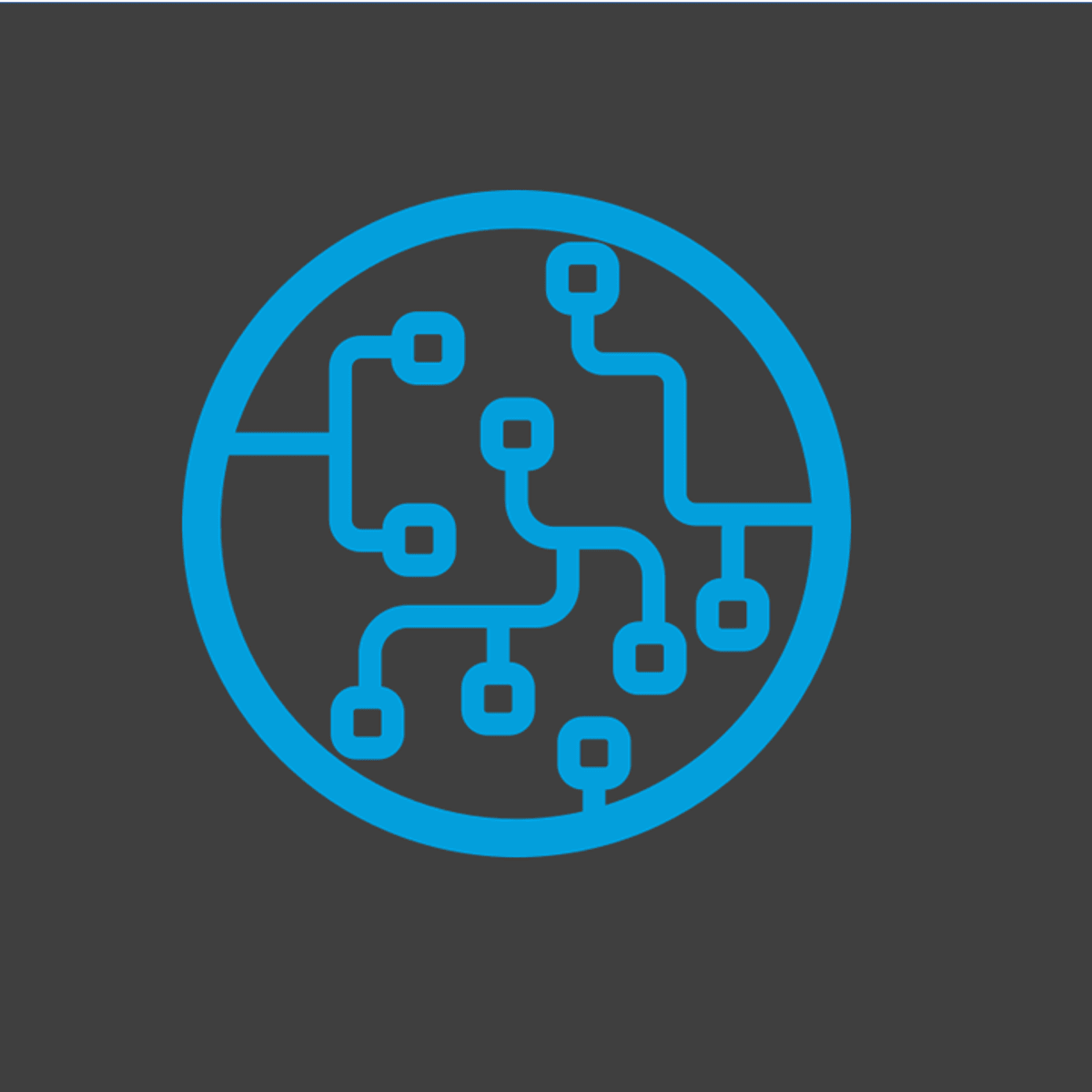
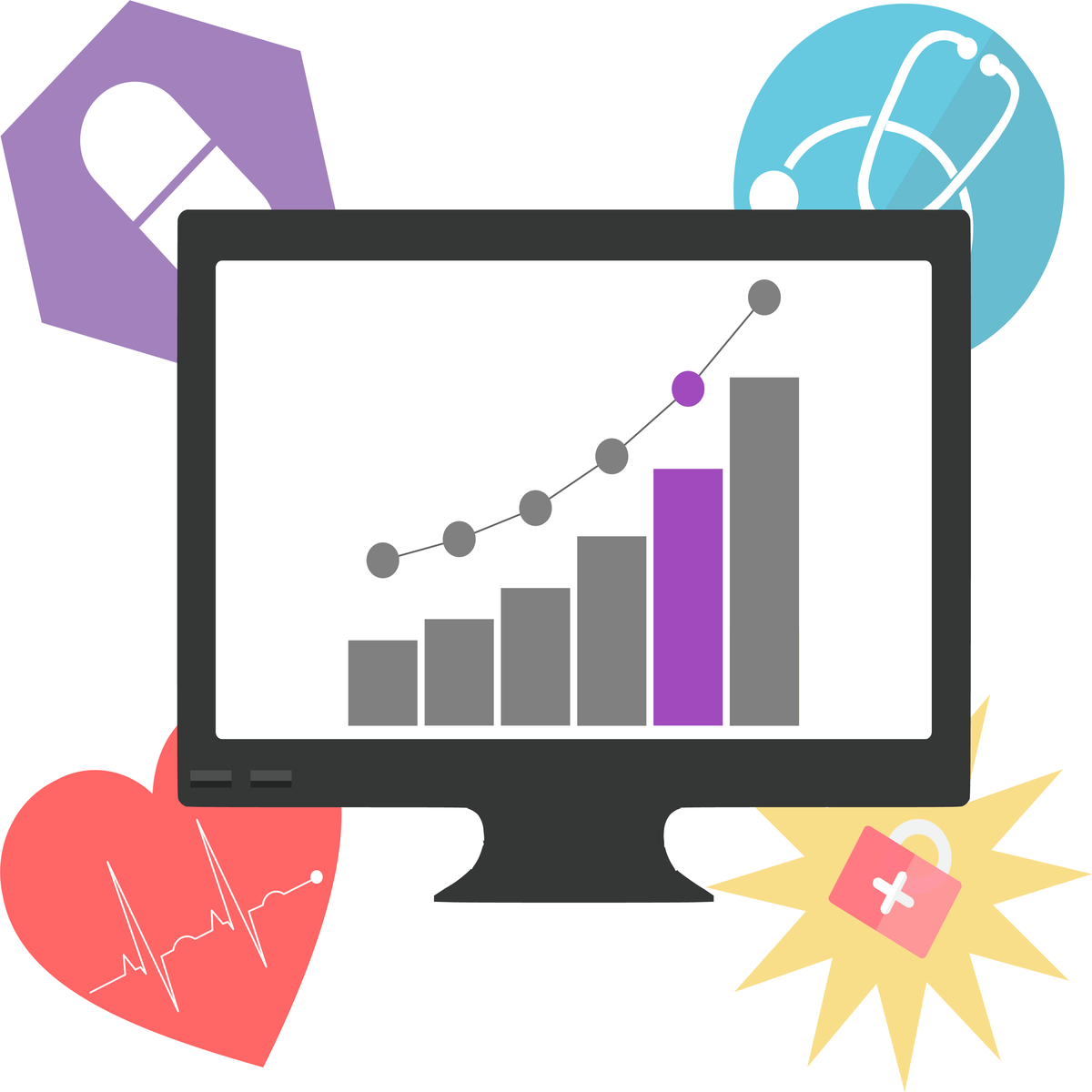
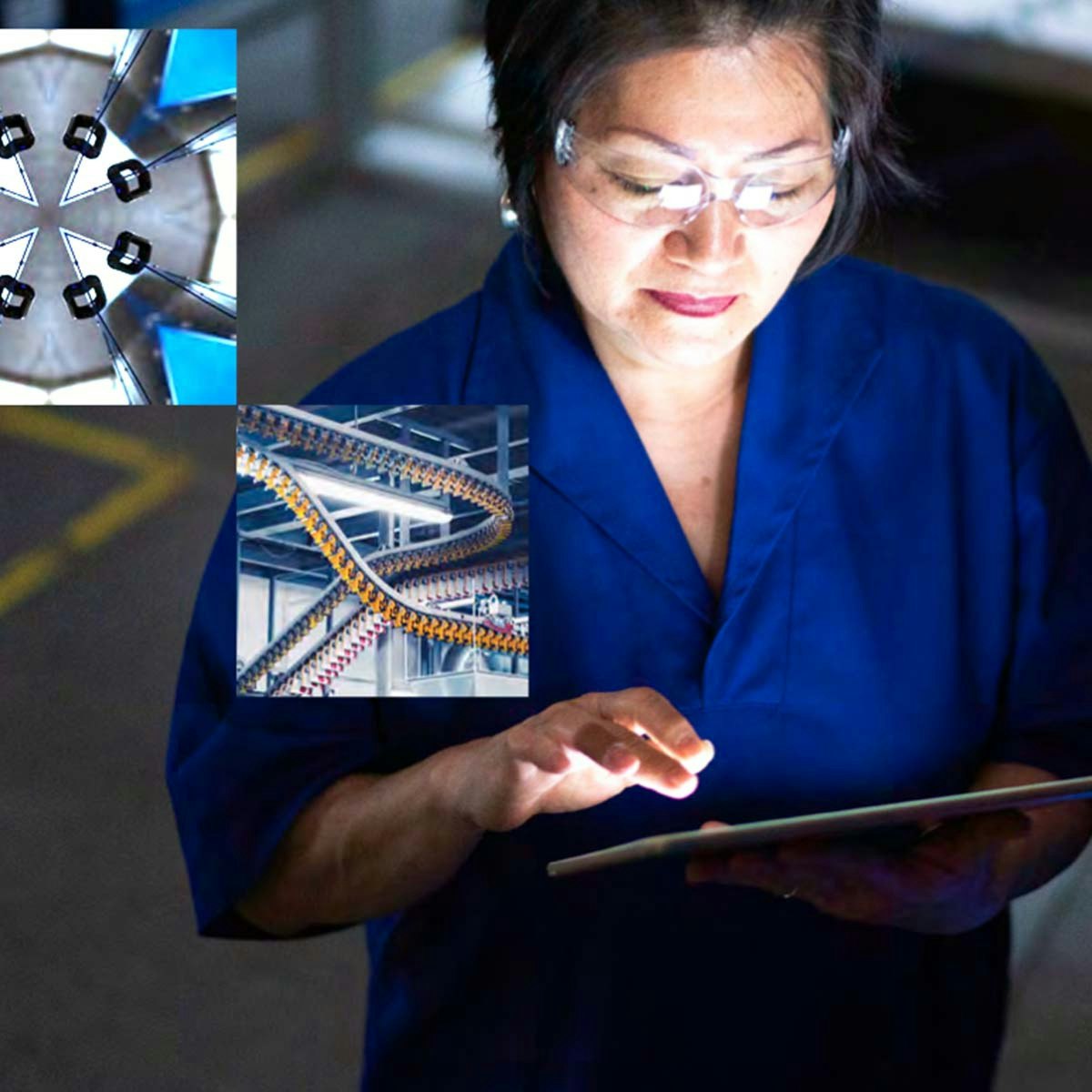
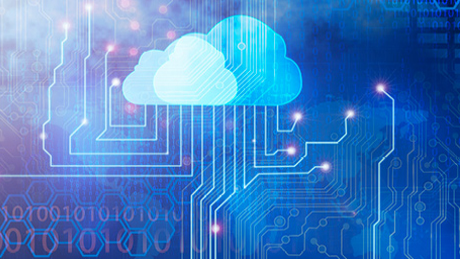
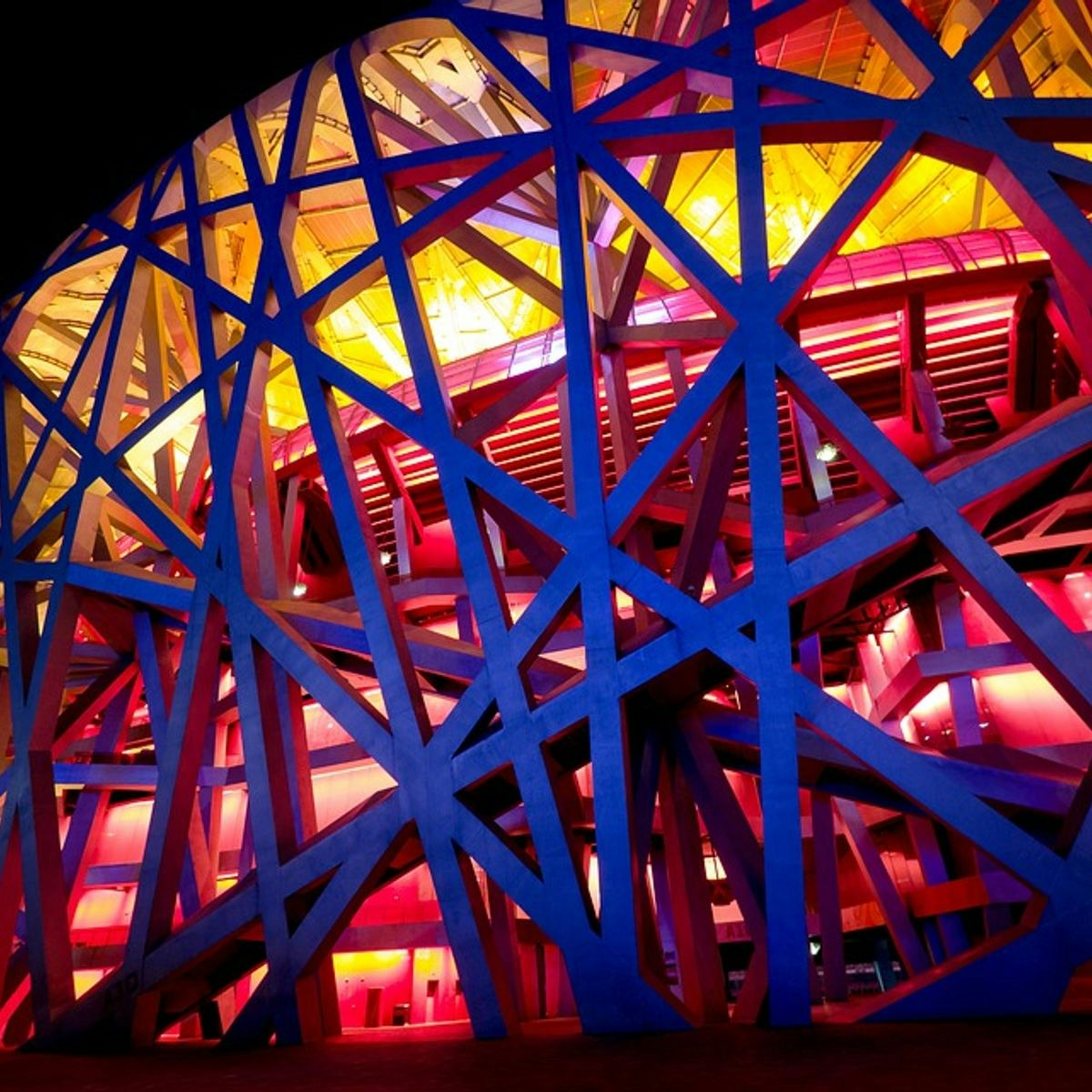
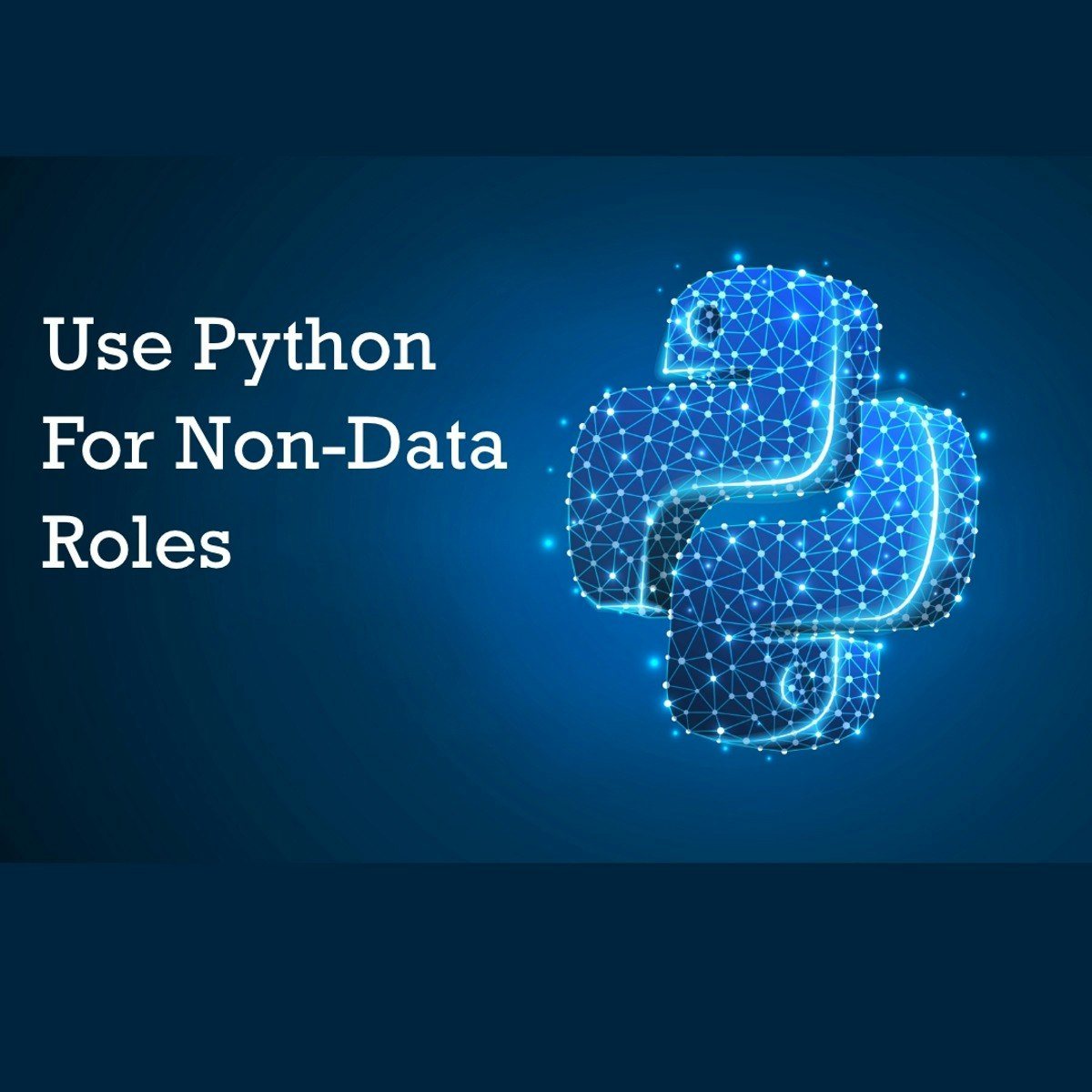
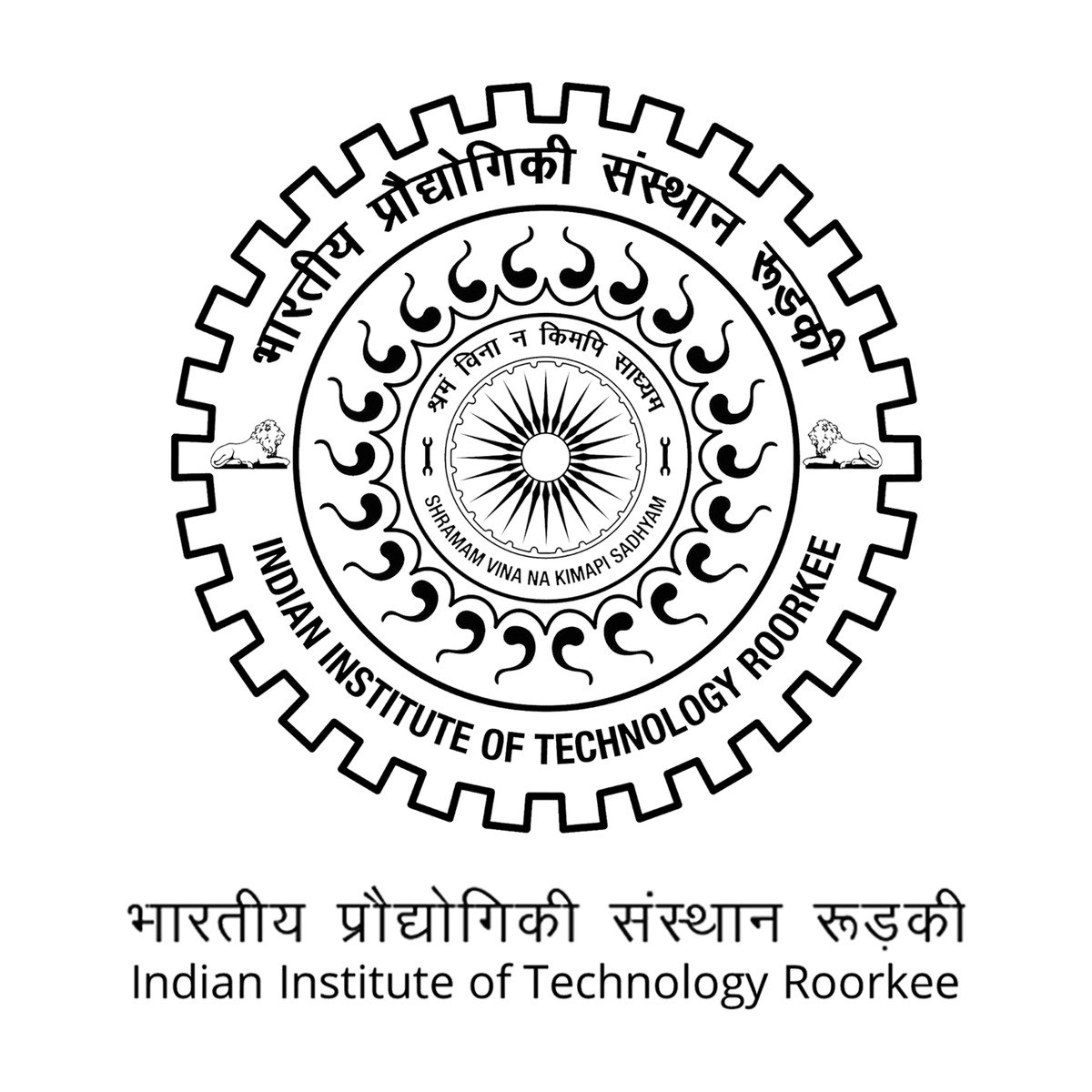
Data Science Courses - Page 62
Showing results 611-620 of 1407
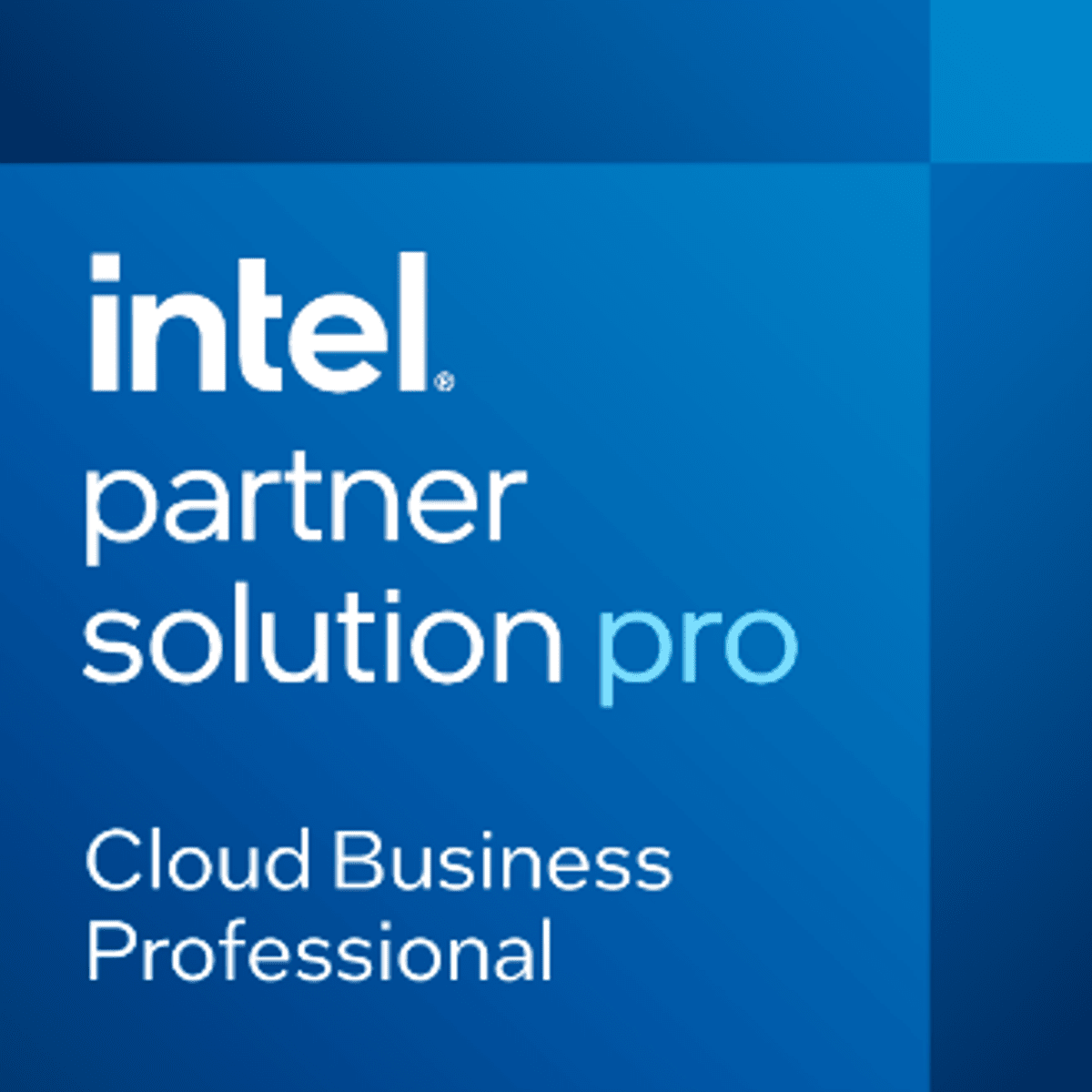
Business Case for Cloud Workloads
Learn what a workloads placement strategy is, why it is important, and how to demonstrate to customers the tangible value that Intel® technical optimizations offer as part of the workload placement process.
● Cloud Customer Business Drivers and Requirements: This lesson identifies what business and ecosystem drivers lead a business to adopt a cloud infrastructure and what requirements that should incorporate into their cloud business decisions. (Duration: 30 minutes)
● Cloud Services Business Impacts: In this lesson you will learn about cloud services considerations, cloud transformation business methodology, and the value of Intel technology in the cloud. (Duration: 30 minutes)
● Yes: This lesson describes the challenges businesses face in building a digital enterprise and how the cloud supported by Intel® technology help deliver strong value in meeting their business requirements. (Duration: 30 minutes)
● Aligning Cloud Business Needs - Use Case Discussions: This lesson provides role play scenarios describing how to handle four distinct cloud business use case discussions. (Duration: 41 minutes)
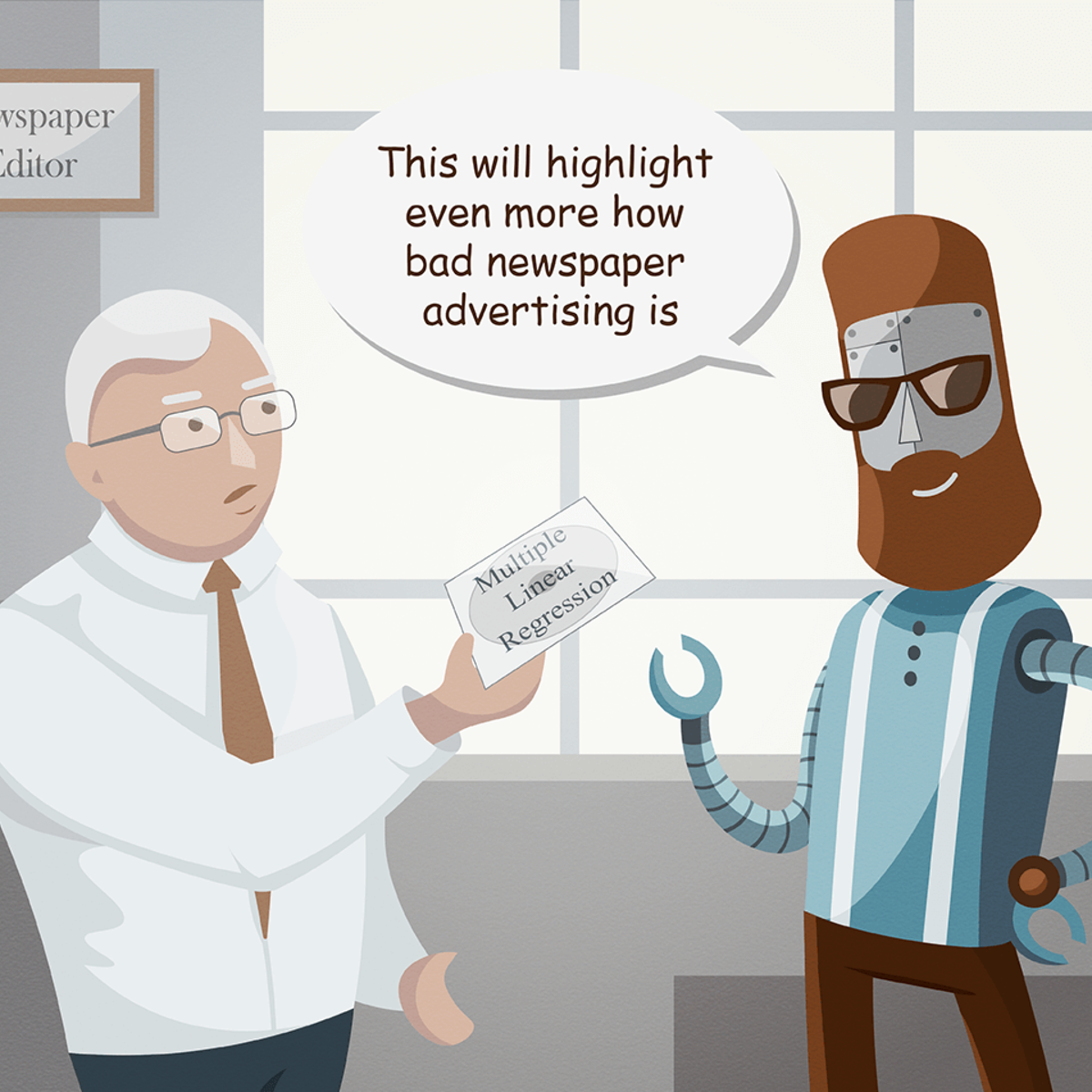
Multiple Linear Regression with scikit-learn
In this 2-hour long project-based course, you will build and evaluate multiple linear regression models using Python. You will use scikit-learn to calculate the regression, while using pandas for data management and seaborn for data visualization. The data for this project consists of the very popular Advertising dataset to predict sales revenue based on advertising spending through media such as TV, radio, and newspaper.
By the end of this project, you will be able to:
- Build univariate and multivariate linear regression models using scikit-learn
- Perform Exploratory Data Analysis (EDA) and data visualization with seaborn
- Evaluate model fit and accuracy using numerical measures such as R² and RMSE
- Model interaction effects in regression using basic feature engineering techniques
This course runs on Coursera's hands-on project platform called Rhyme. On Rhyme, you do projects in a hands-on manner in your browser. You will get instant access to pre-configured cloud desktops containing all of the software and data you need for the project. Everything is already set up directly in your internet browser so you can just focus on learning. For this project, this means instant access to a cloud desktop with Jupyter Notebooks and Python 3.7 with all the necessary libraries pre-installed.
Notes:
- You will be able to access the cloud desktop 5 times. However, you will be able to access instructions videos as many times as you want.
- This course works best for learners who are based in the North America region. We’re currently working on providing the same experience in other regions.
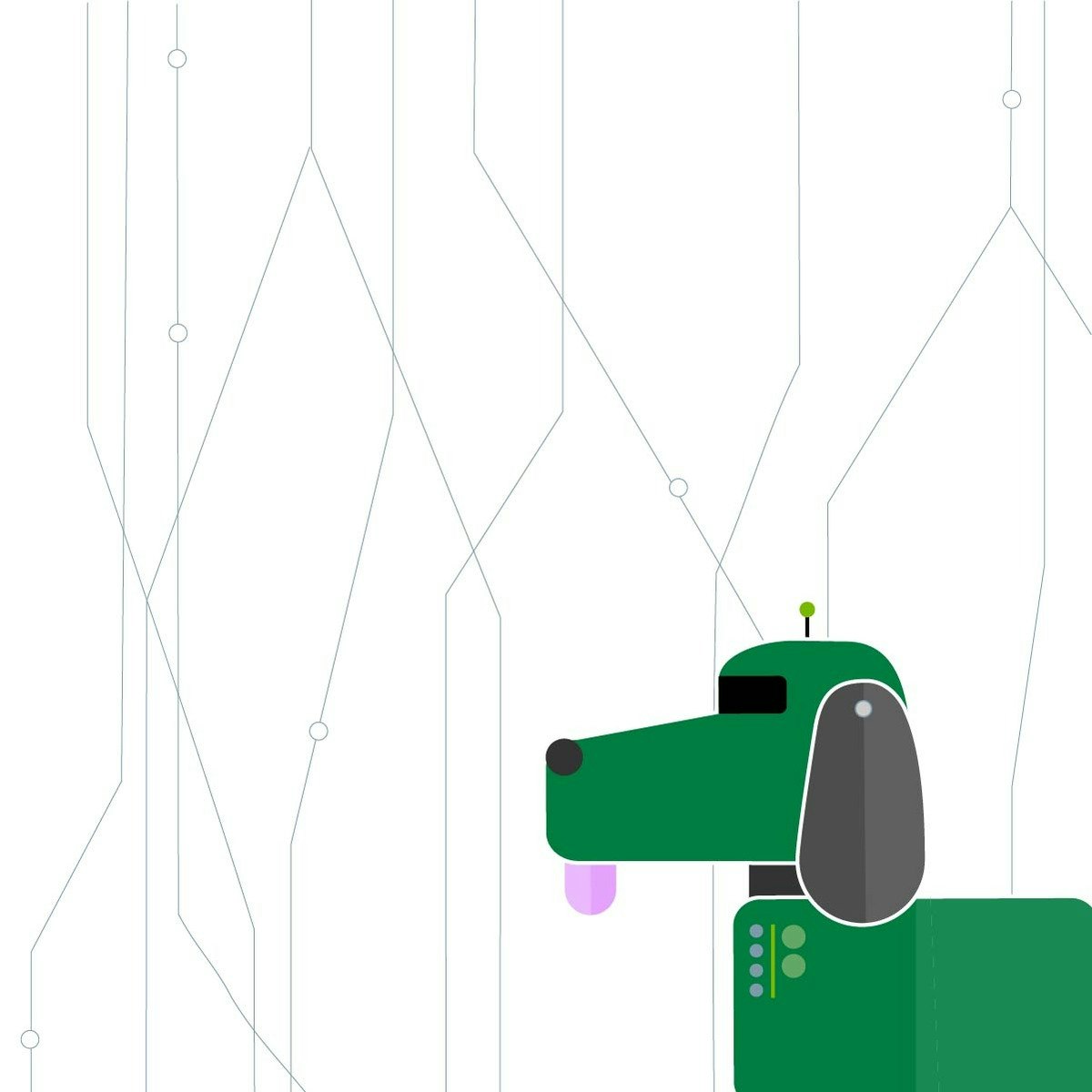
A Complete Reinforcement Learning System (Capstone)
In this final course, you will put together your knowledge from Courses 1, 2 and 3 to implement a complete RL solution to a problem. This capstone will let you see how each component---problem formulation, algorithm selection, parameter selection and representation design---fits together into a complete solution, and how to make appropriate choices when deploying RL in the real world. This project will require you to implement both the environment to stimulate your problem, and a control agent with Neural Network function approximation. In addition, you will conduct a scientific study of your learning system to develop your ability to assess the robustness of RL agents. To use RL in the real world, it is critical to (a) appropriately formalize the problem as an MDP, (b) select appropriate algorithms, (c ) identify what choices in your implementation will have large impacts on performance and (d) validate the expected behaviour of your algorithms. This capstone is valuable for anyone who is planning on using RL to solve real problems.
To be successful in this course, you will need to have completed Courses 1, 2, and 3 of this Specialization or the equivalent.
By the end of this course, you will be able to:
Complete an RL solution to a problem, starting from problem formulation, appropriate algorithm selection and implementation and empirical study into the effectiveness of the solution.
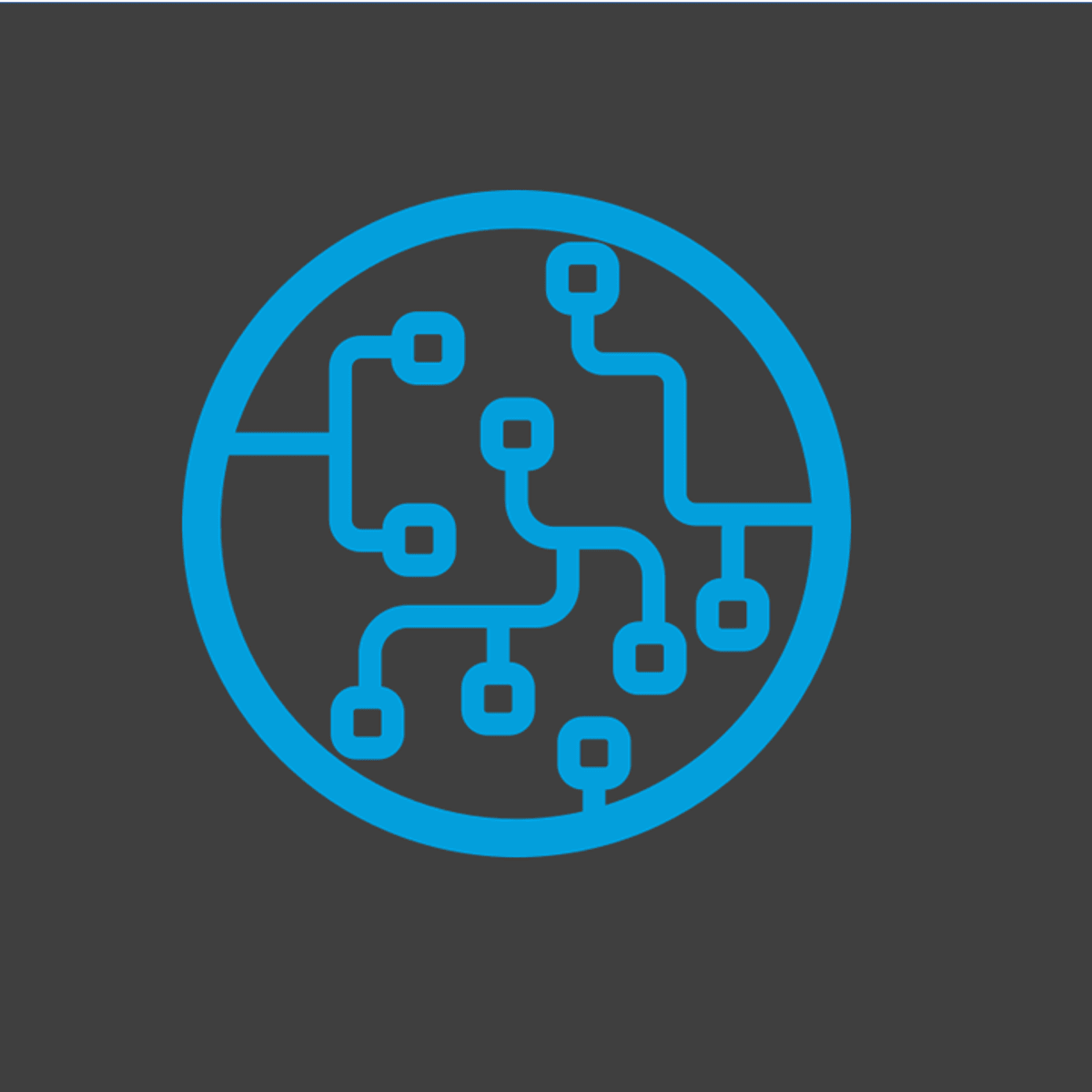
Machine/Deep Learning for Mining Quality Prediction-Enhanced
In this hands-on project, we will train machine learning and deep learning models to predict the % of Silica Concentrate in the Iron ore concentrate per minute. This project could be practically used in Mining Industry to get the % Silica Concentrate at much faster rate compared to the traditional methods.
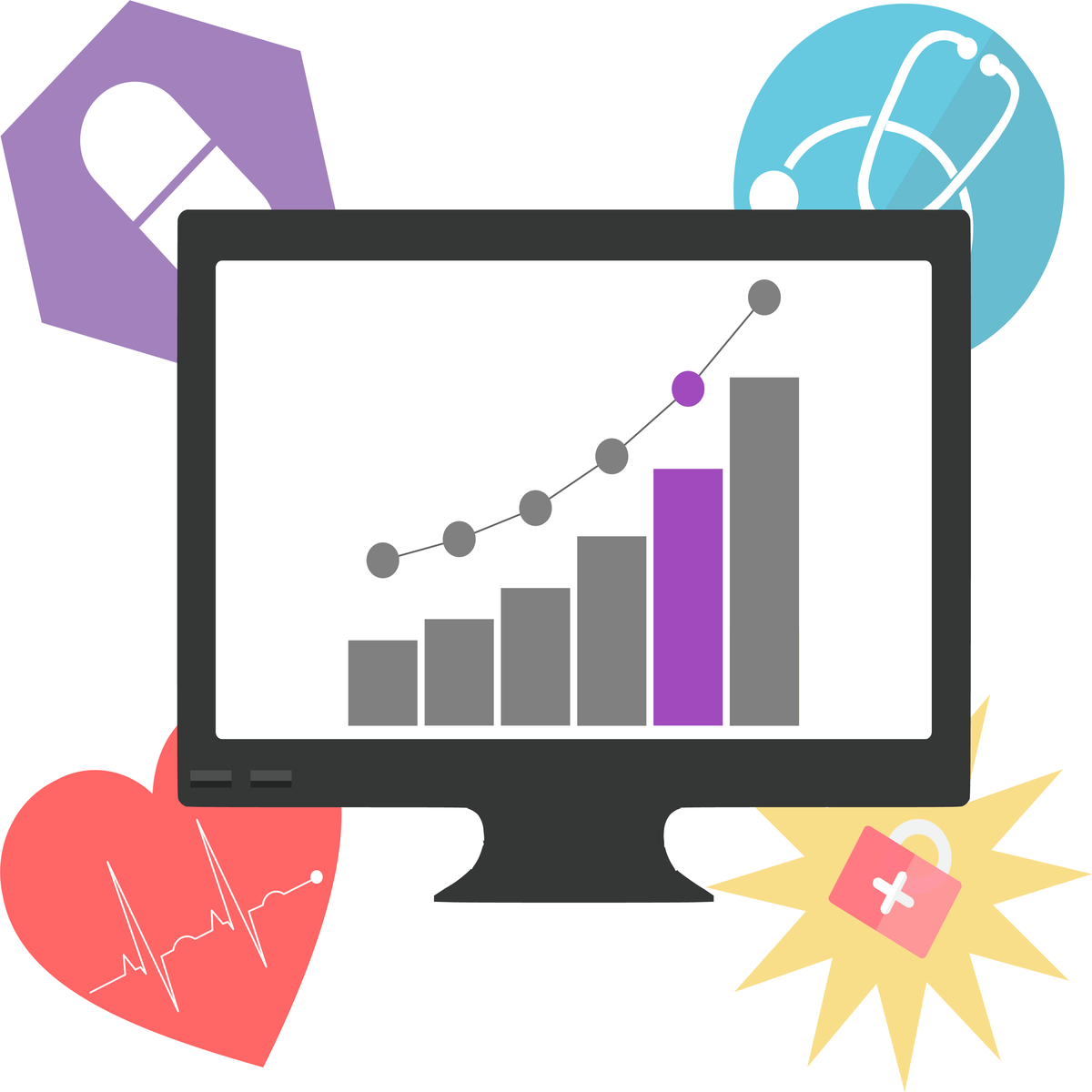
Predictive Modeling and Transforming Clinical Practice
This course teaches you the fundamentals of transforming clinical practice using predictive models. This course examines specific challenges and methods of clinical implementation, that clinical data scientists must be aware of when developing their predictive models.
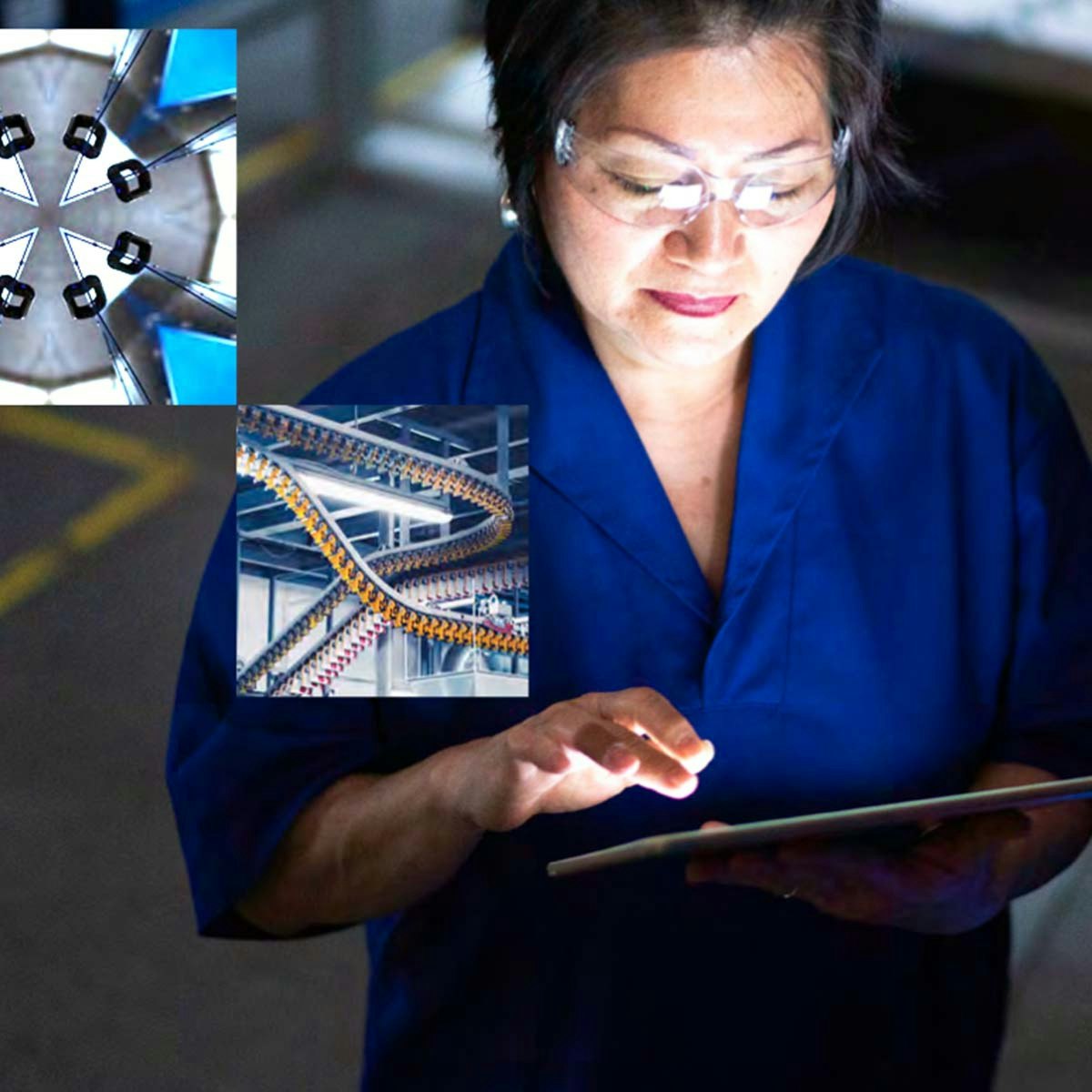
Machine Teaching for Autonomous AI
Just as teachers help students gain new skills, the same is true of artificial intelligence (AI). Machine learning algorithms can adapt and change, much like the learning process itself. Using the machine teaching paradigm, a subject matter expert (SME) can teach AI to improve and optimize a variety of systems and processes. The result is an autonomous AI system.
In this course, you’ll learn how automated systems make decisions and how to approach building an AI system that will outperform current capabilities. Since 87% of machine learning systems fail in the proof-concept phase, it’s important you understand how to analyze an existing system and determine whether it’d be a good fit for machine teaching approaches. For your course project, you’ll select an appropriate use case, interview a SME about a process, and then flesh out a story for why and how you might go about building an autonomous AI system.
At the end of this course, you’ll be able to:
• Describe the concept of machine teaching
• Explain the role that SMEs play in training advanced AI
• Evaluate the pros and cons of leveraging human expertise in the design of AI systems
• Differentiate between automated and autonomous decision-making systems
• Describe the limitations of automated systems and humans in real-time decision-making
• Select use cases where autonomous AI will outperform both humans and automated systems
• Propose an autonomous AI solution to a real-world problem
• Validate your design against existing expertise and techniques for solving problems
This course is part of a specialization called Autonomous AI for Industry, which will launch in fall 2022.
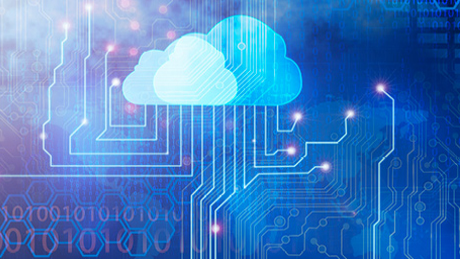
Data Manipulation at Scale: Systems and Algorithms
Data analysis has replaced data acquisition as the bottleneck to evidence-based decision making --- we are drowning in it. Extracting knowledge from large, heterogeneous, and noisy datasets requires not only powerful computing resources, but the programming abstractions to use them effectively. The abstractions that emerged in the last decade blend ideas from parallel databases, distributed systems, and programming languages to create a new class of scalable data analytics platforms that form the foundation for data science at realistic scales.
In this course, you will learn the landscape of relevant systems, the principles on which they rely, their tradeoffs, and how to evaluate their utility against your requirements. You will learn how practical systems were derived from the frontier of research in computer science and what systems are coming on the horizon. Cloud computing, SQL and NoSQL databases, MapReduce and the ecosystem it spawned, Spark and its contemporaries, and specialized systems for graphs and arrays will be covered.
You will also learn the history and context of data science, the skills, challenges, and methodologies the term implies, and how to structure a data science project. At the end of this course, you will be able to:
Learning Goals:
1. Describe common patterns, challenges, and approaches associated with data science projects, and what makes them different from projects in related fields.
2. Identify and use the programming models associated with scalable data manipulation, including relational algebra, mapreduce, and other data flow models.
3. Use database technology adapted for large-scale analytics, including the concepts driving parallel databases, parallel query processing, and in-database analytics
4. Evaluate key-value stores and NoSQL systems, describe their tradeoffs with comparable systems, the details of important examples in the space, and future trends.
5. “Think” in MapReduce to effectively write algorithms for systems including Hadoop and Spark. You will understand their limitations, design details, their relationship to databases, and their associated ecosystem of algorithms, extensions, and languages.
write programs in Spark
6. Describe the landscape of specialized Big Data systems for graphs, arrays, and streams
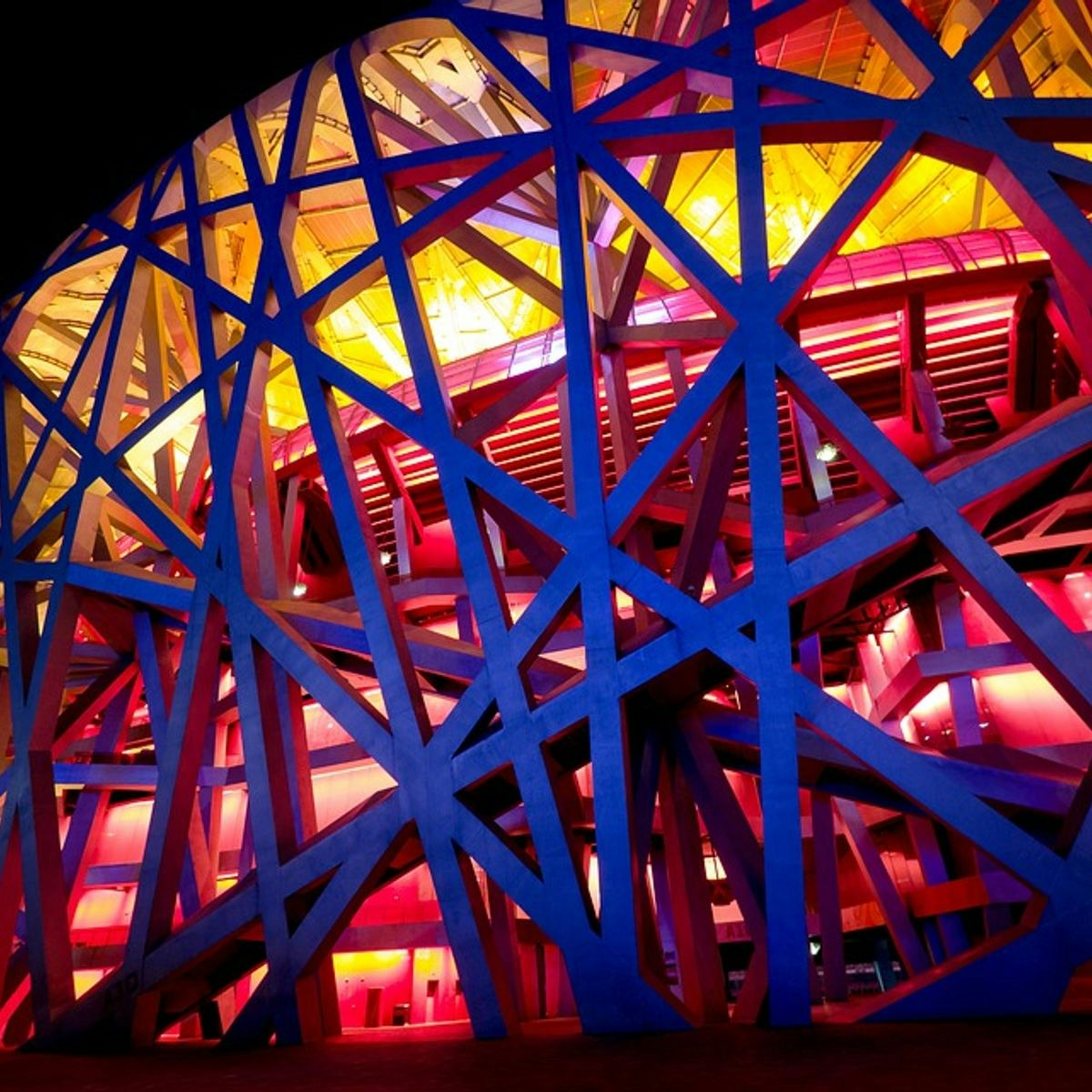
Calculus through Data & Modeling: Differentiation Rules
Calculus through Data & Modeling: Differentiation Rules continues the study of differentiable calculus by developing new rules for finding derivatives without having to use the limit definition directly. These differentiation rules will enable the calculation of rates of change with relative ease the derivatives of polynomials, rational functions, algebraic functions, exponential and logarithmic functions, and trigonometric and inverse trigonometric functions. Once these rules are developed, they are then applied to solve problems involving rates of change and the approximation of functions.
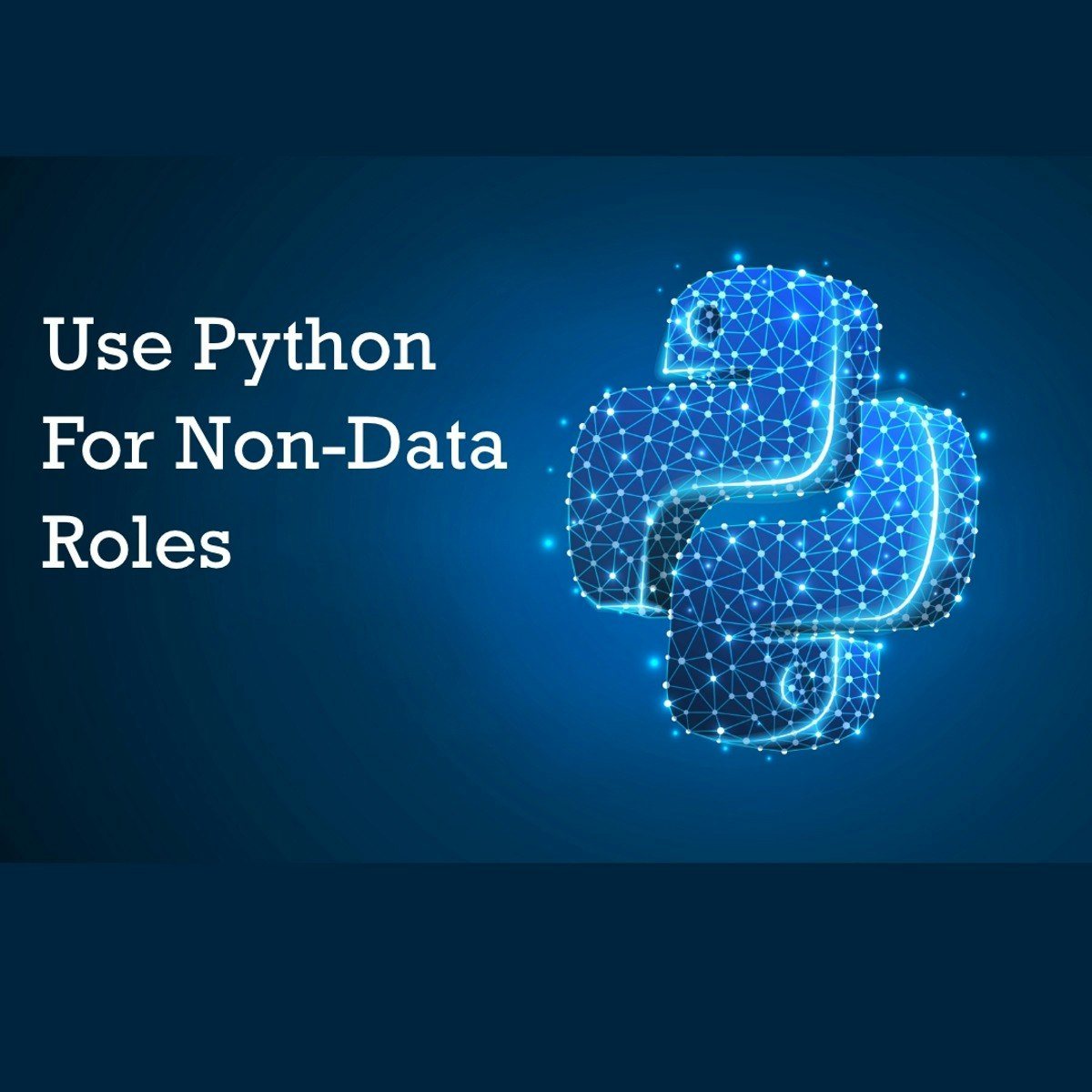
Use Python for Non-Data Role
Even if you are not a person with a data specific role, knowing how to work with data is becoming a highly in-demand skill. More and more companies are collecting data, storing data, and making data-based decisions. From marketing to human resources, to finance and operations, knowing the basics of how to use a data language such as Python will make you even more desirable, valuable, and useful to your company. In this project, learners will learn how to get started with Python. They will learn how to upload Excel sets into Python; how to create lists, dictionaries, and tables; and even how to create Gantt charts with Python. This will arm people in all kinds of roles to use Python to perform data tasks.
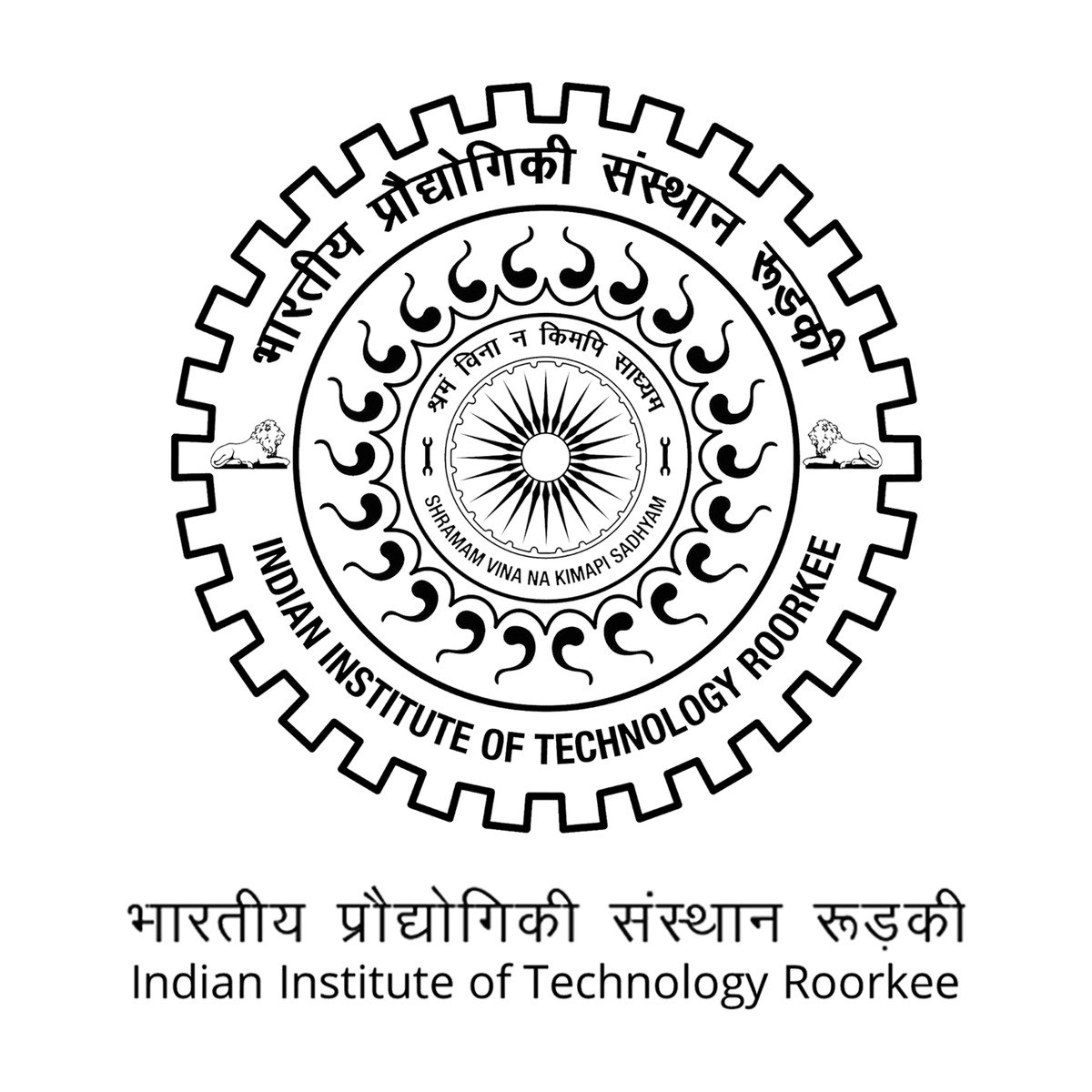
Data Mining for Smart Cities
Internet of things (IoT) has become a significant component of urban life, giving rise to “smart cities.” These smart cities aim to transform present-day urban conglomerates into citizen-friendly and environmentally sustainable living spaces. The digital infrastructure of smart cities generates a huge amount of data that could help us better understand operations and other significant aspects of city life.
In this course, you will become aware of various data mining and machine learning techniques and the various dataset on which they can be applied. You will learn how to implement data mining in Python and interpret the results to extract actionable knowledge. The course includes hands-on experiments using various real-life datasets to enable you to experiment on your domain-related novel datasets. You will use Python 3 programming language to read and preprocess the data and then implement various data mining tasks on the cleaned data to obtain desired results. Subsequently, you will visualize the results for the most efficient description.
Popular Internships and Jobs by Categories
Find Jobs & Internships
Browse
© 2024 BoostGrad | All rights reserved