Back to Courses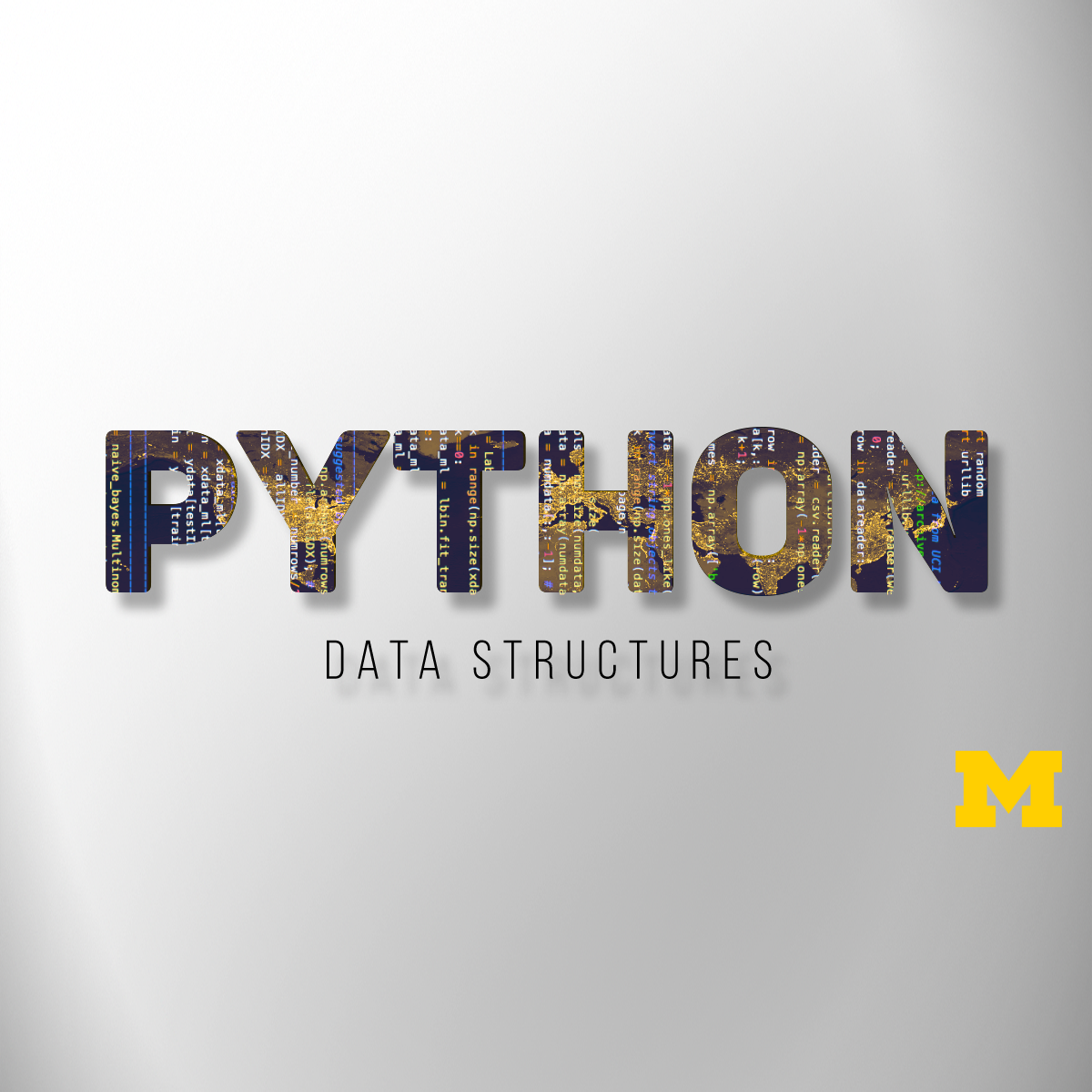
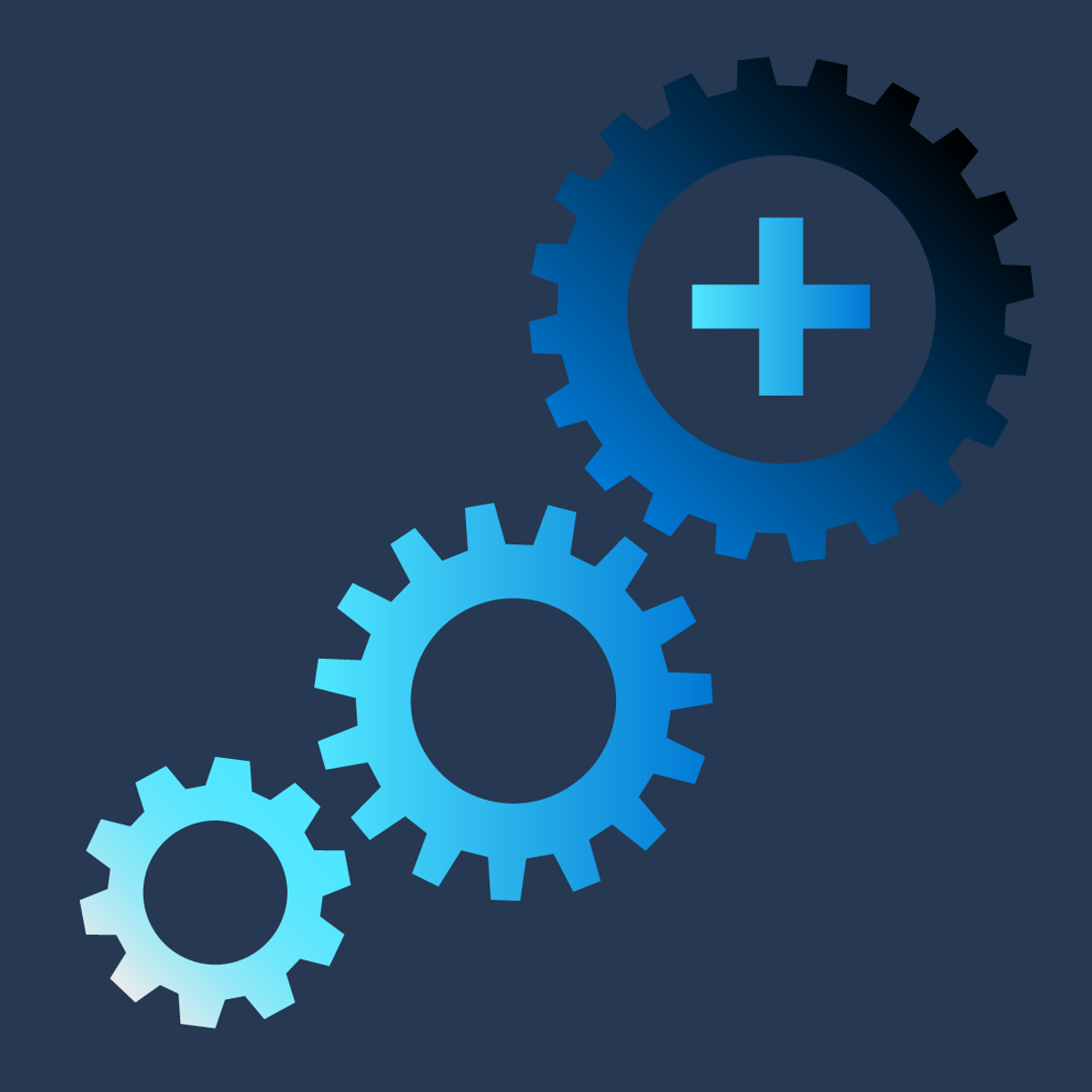
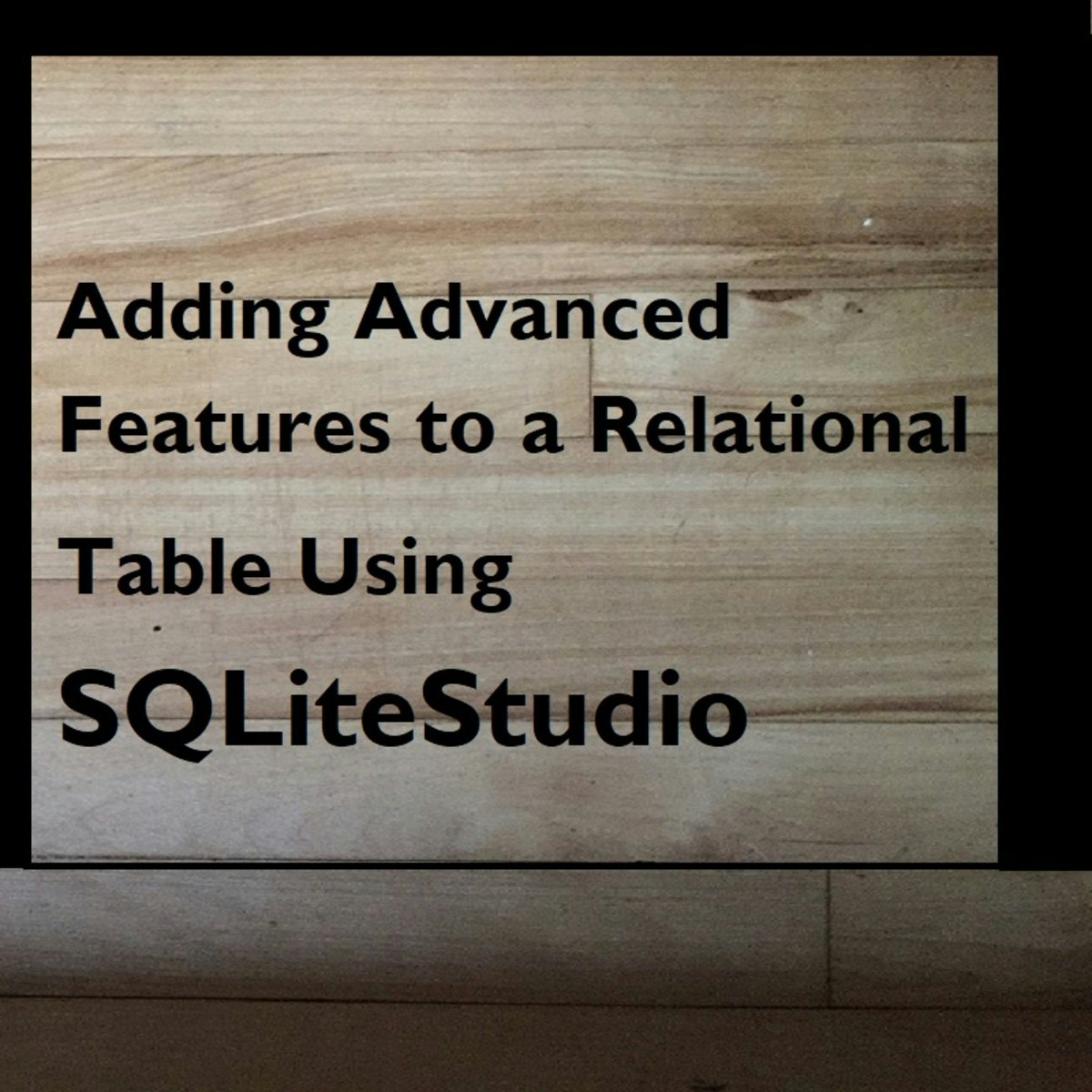
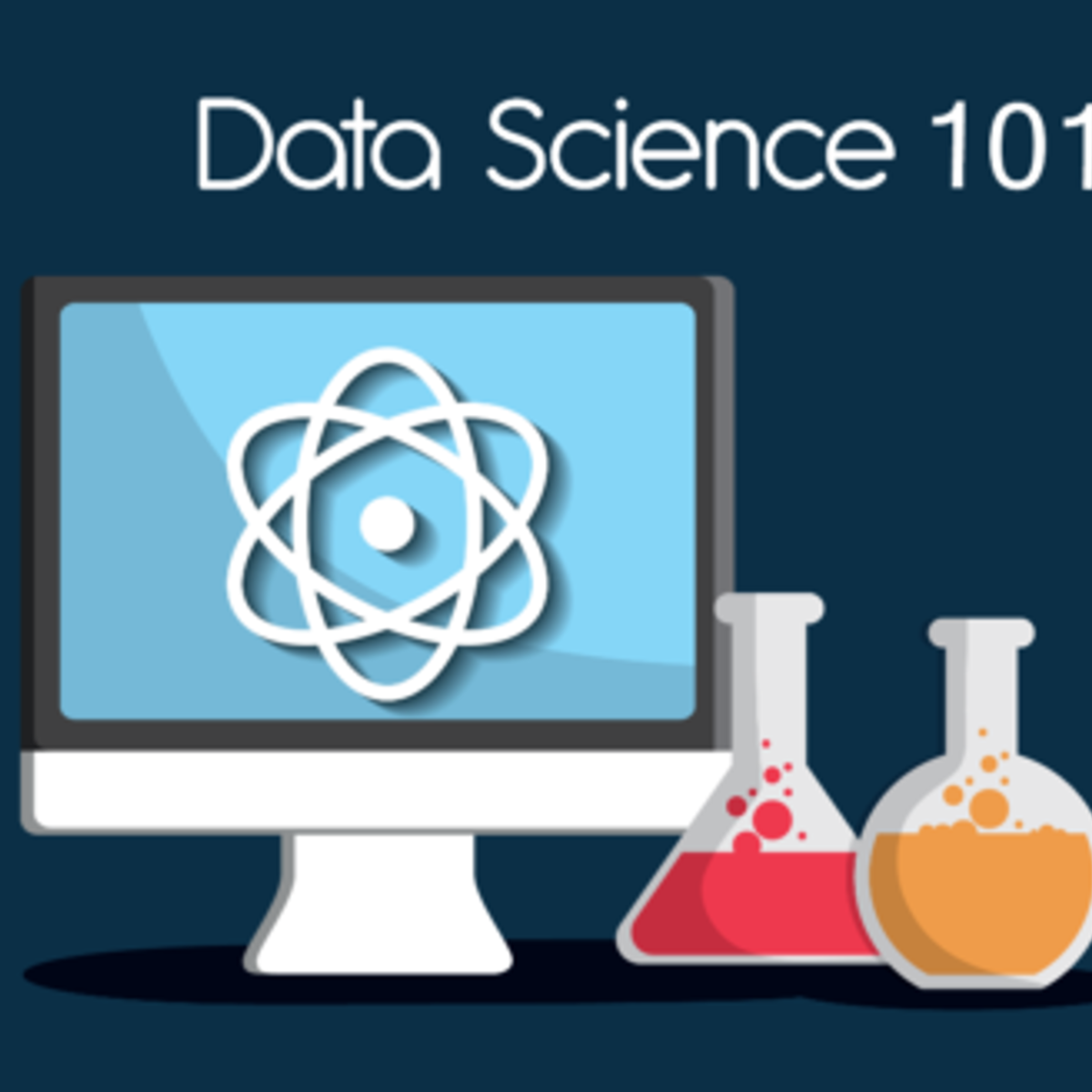
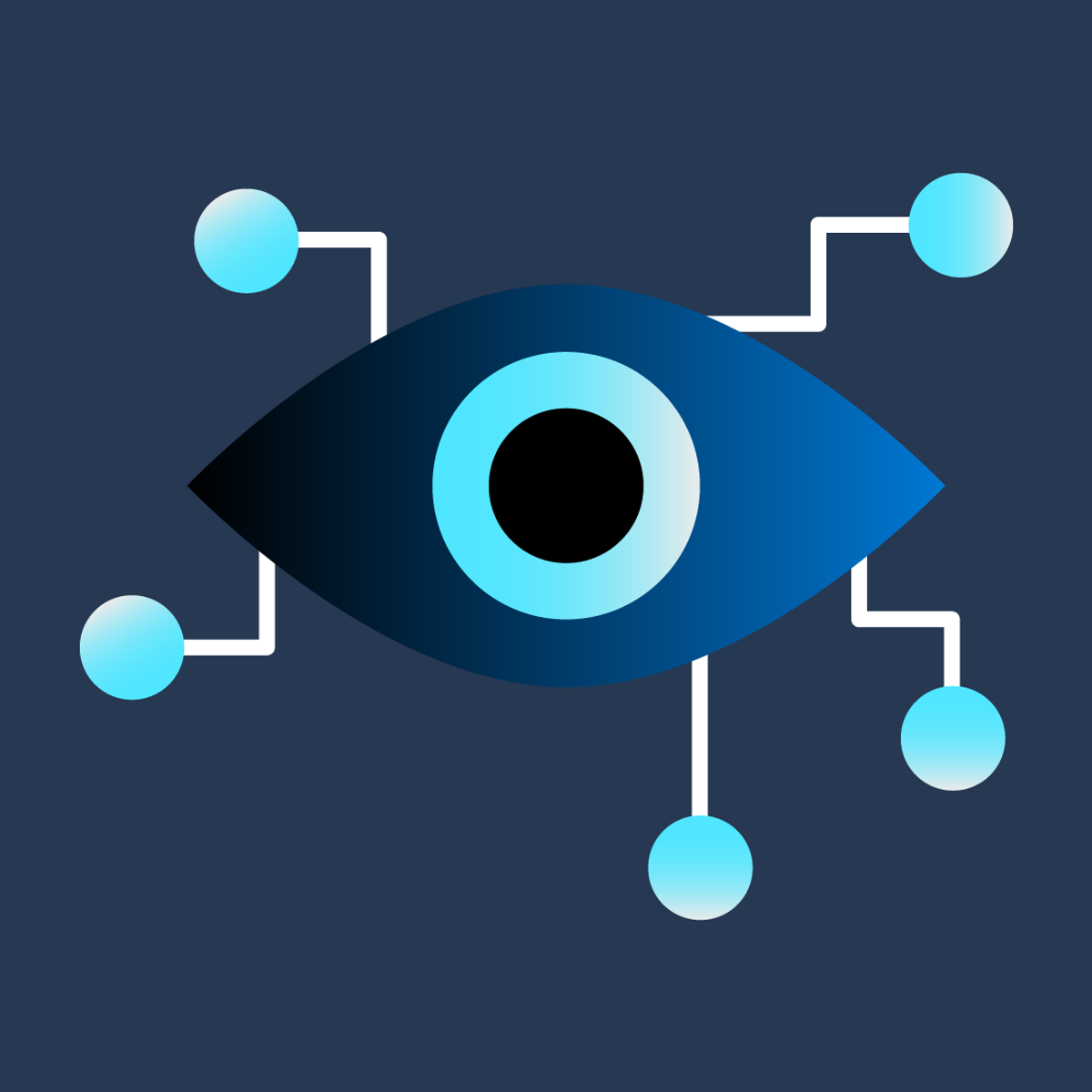
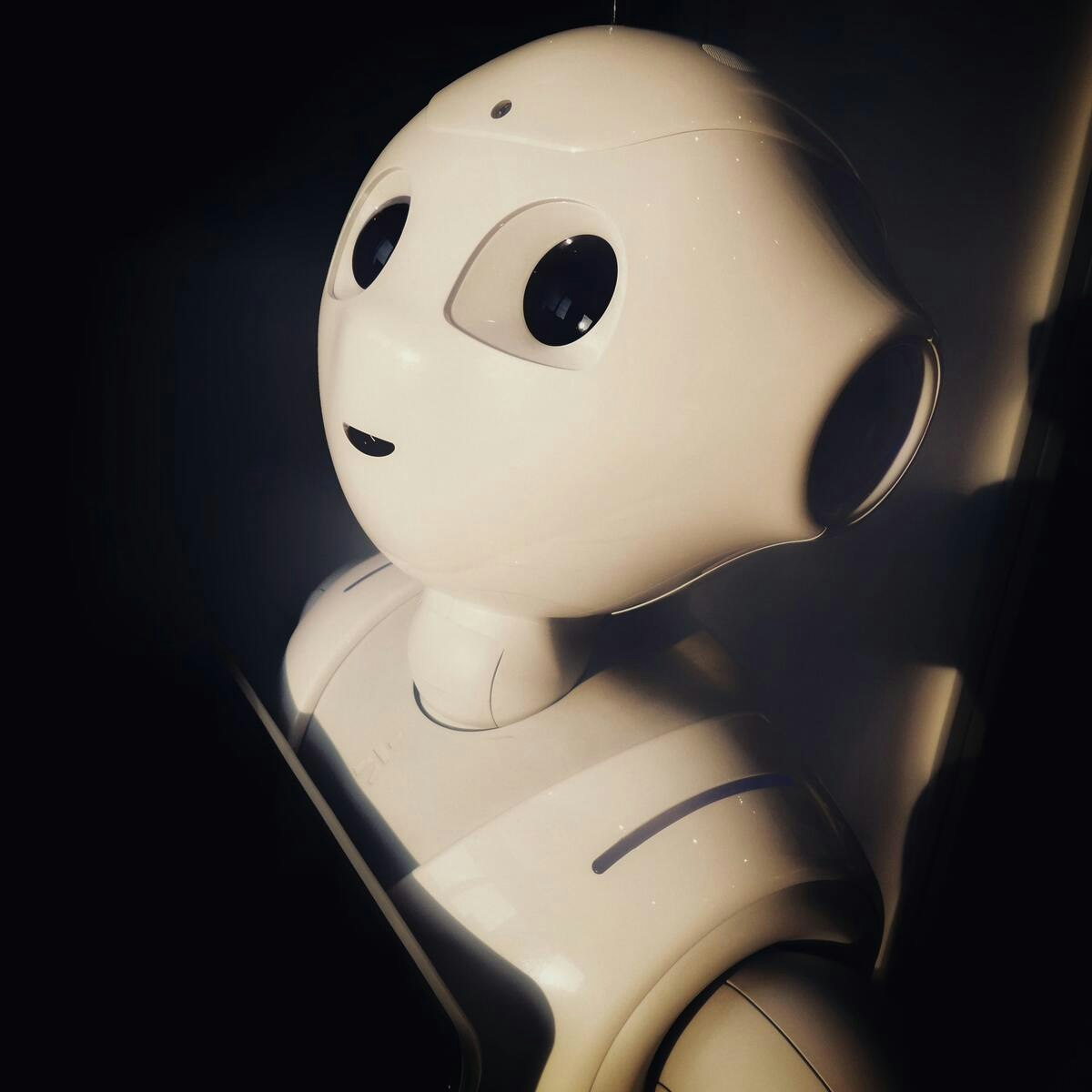
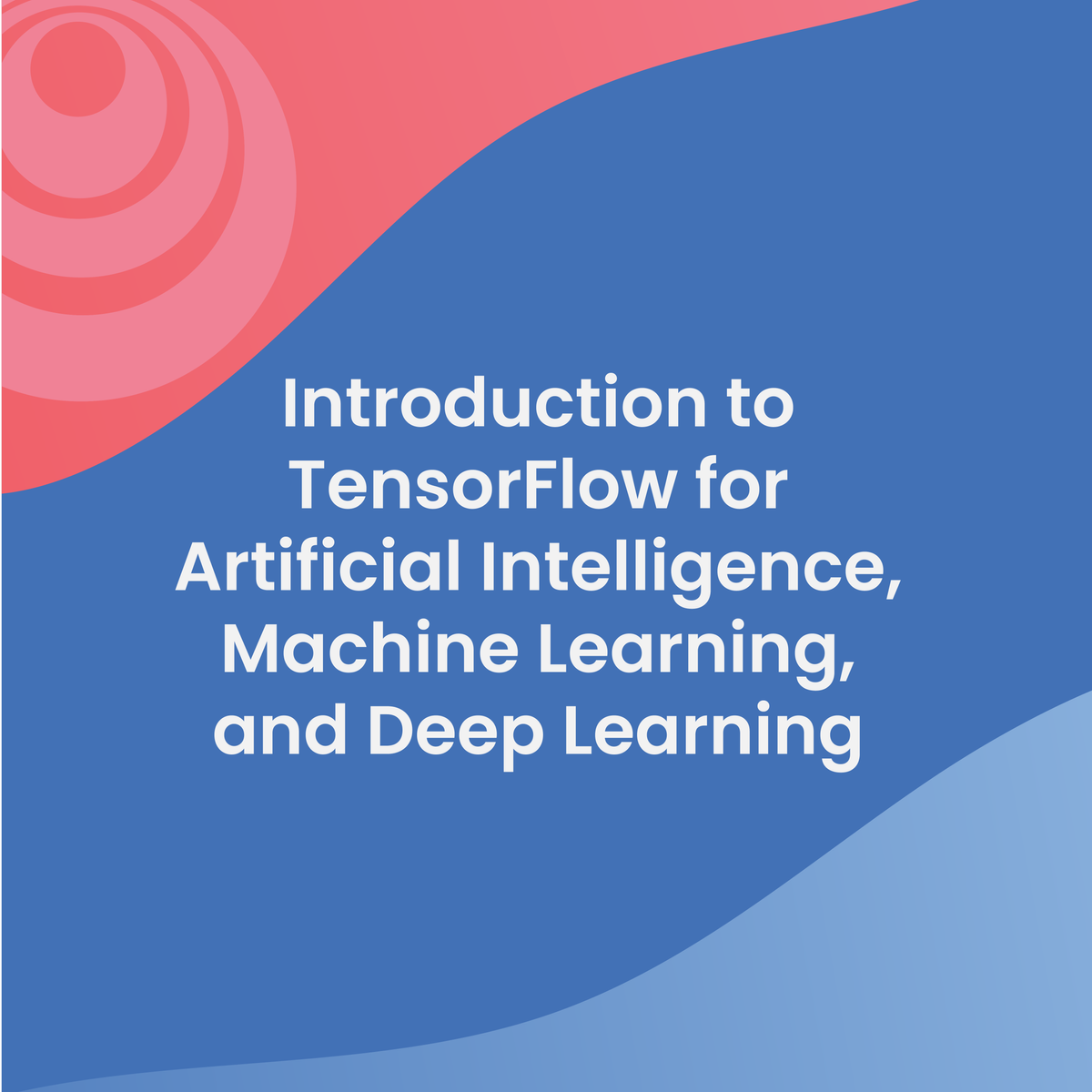
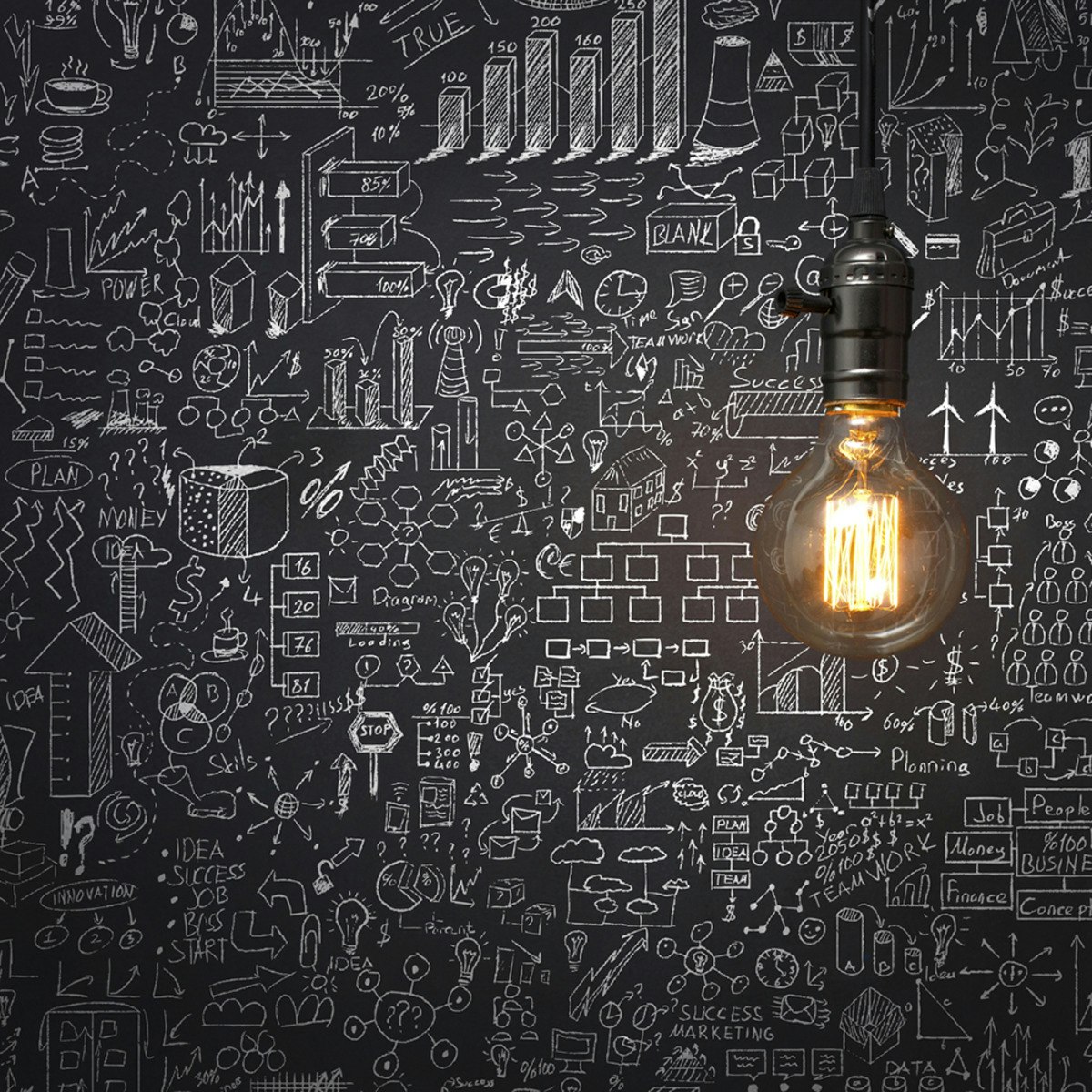
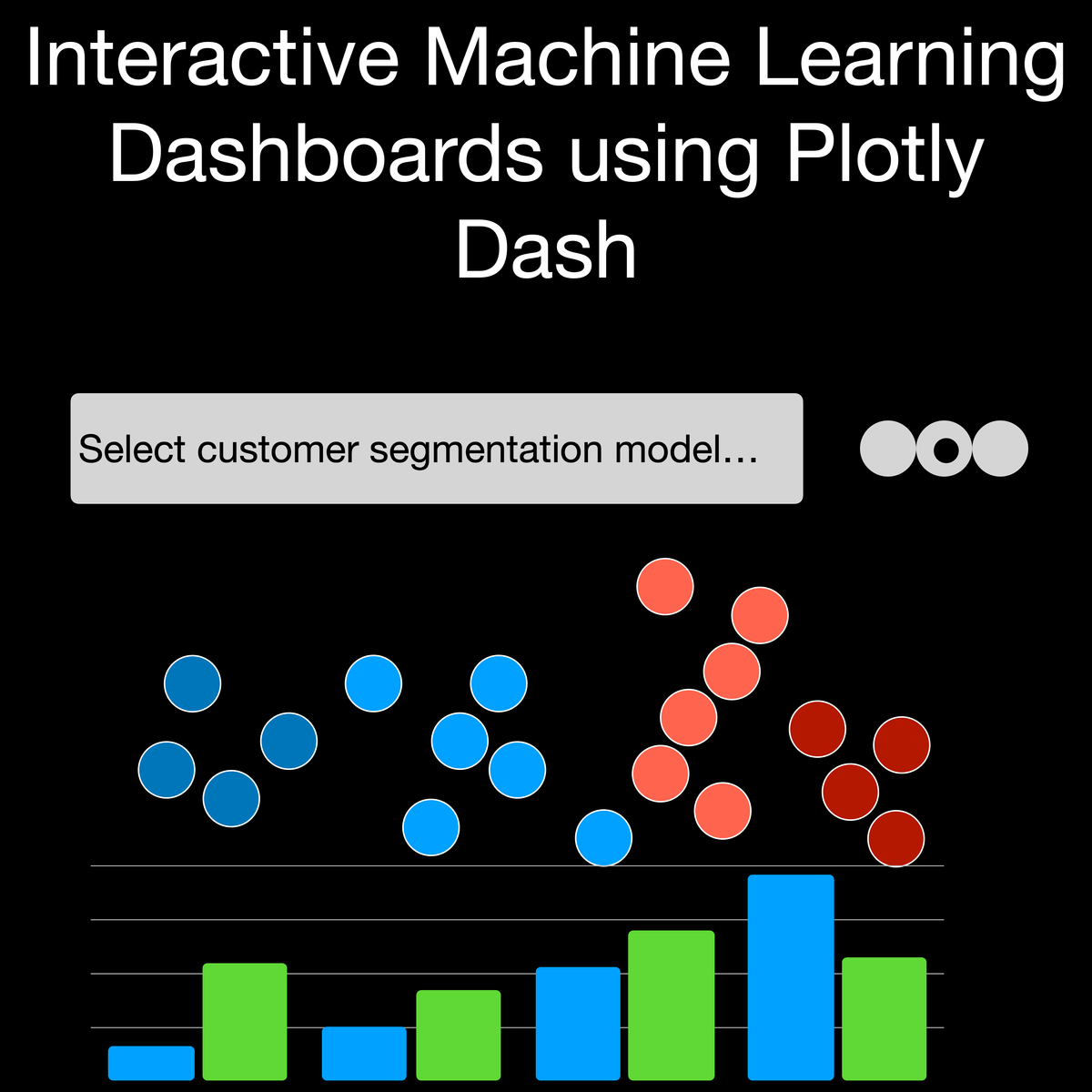
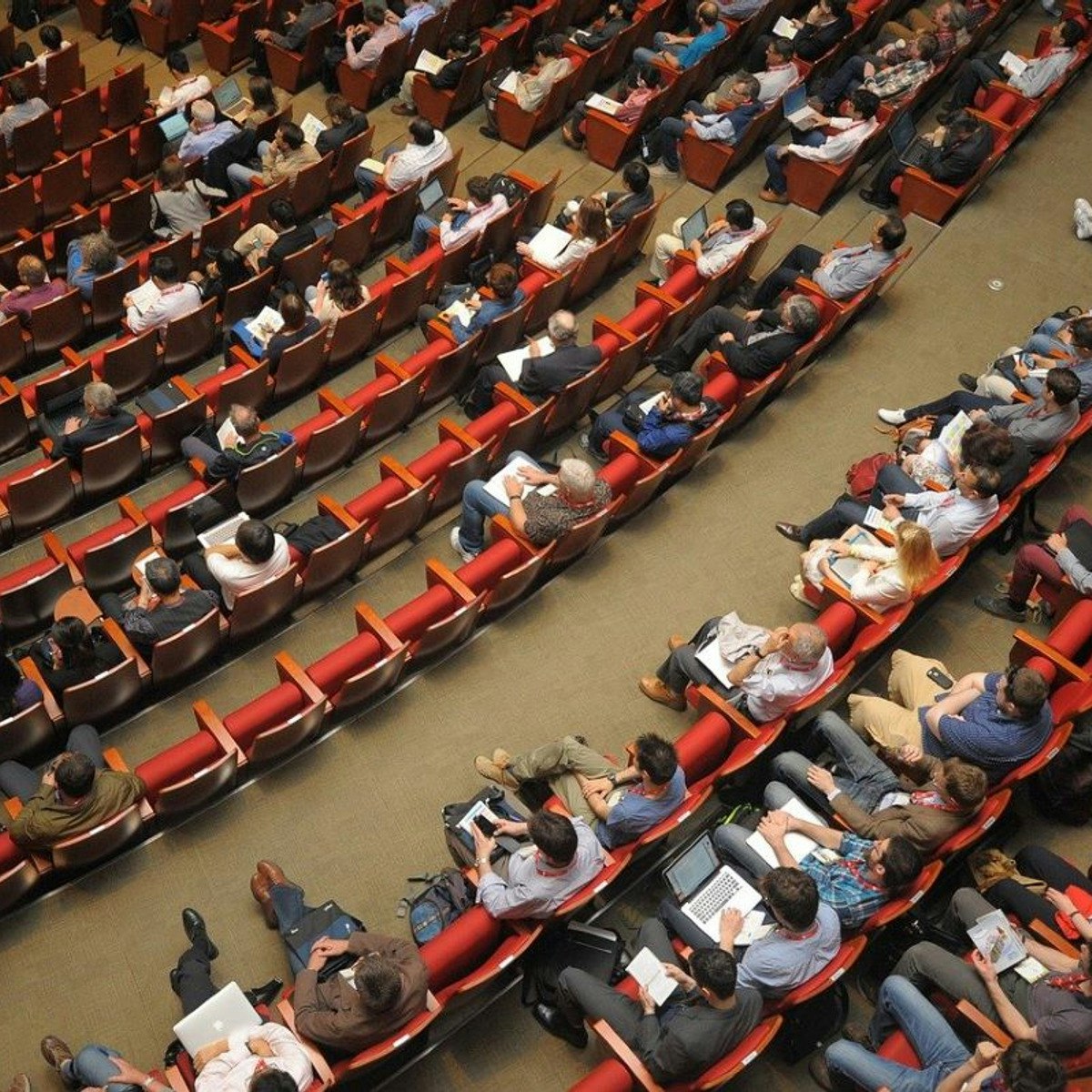
Data Science Courses - Page 6
Showing results 51-60 of 1407
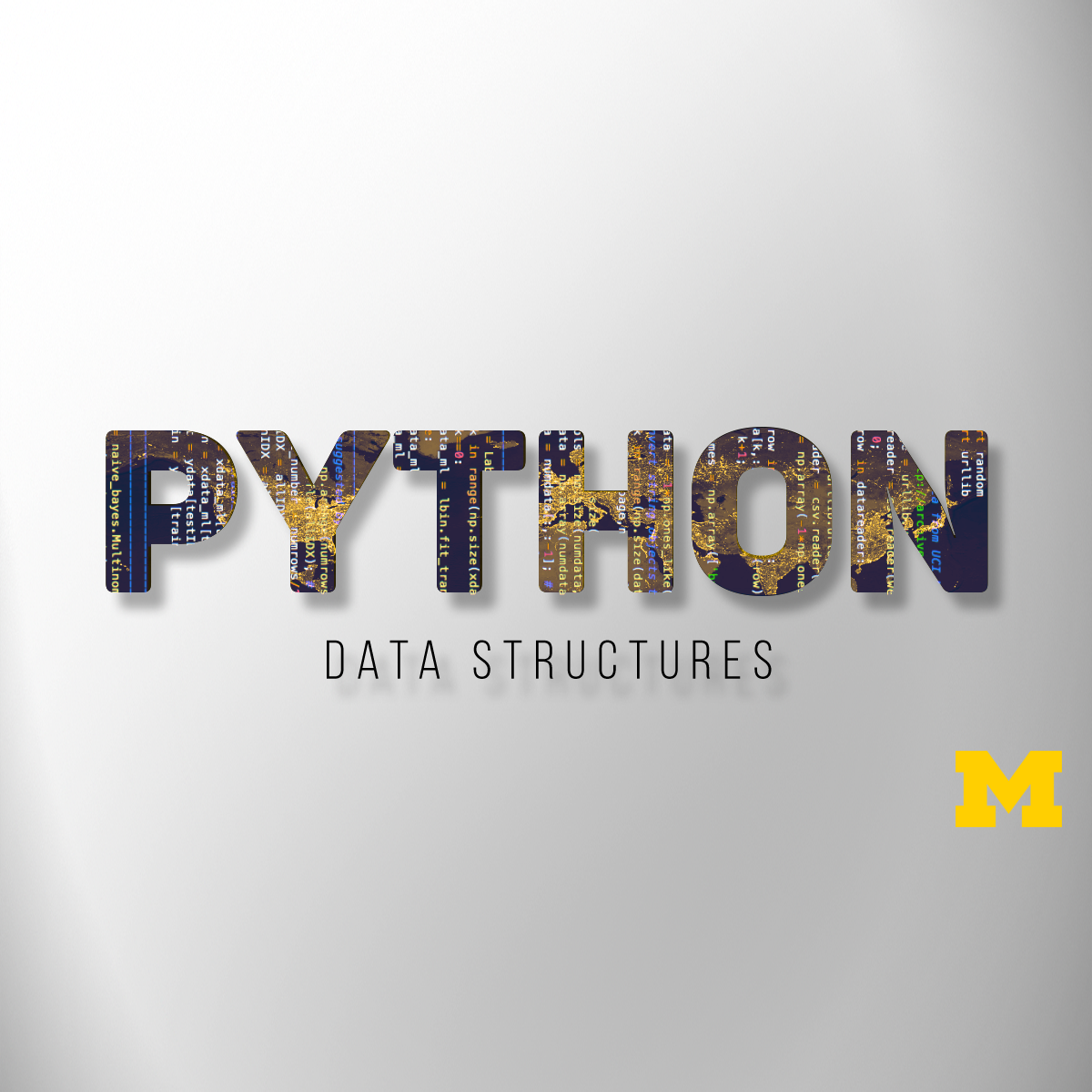
Python Data Structures
This course will introduce the core data structures of the Python programming language. We will move past the basics of procedural programming and explore how we can use the Python built-in data structures such as lists, dictionaries, and tuples to perform increasingly complex data analysis. This course will cover Chapters 6-10 of the textbook “Python for Everybody”. This course covers Python 3.
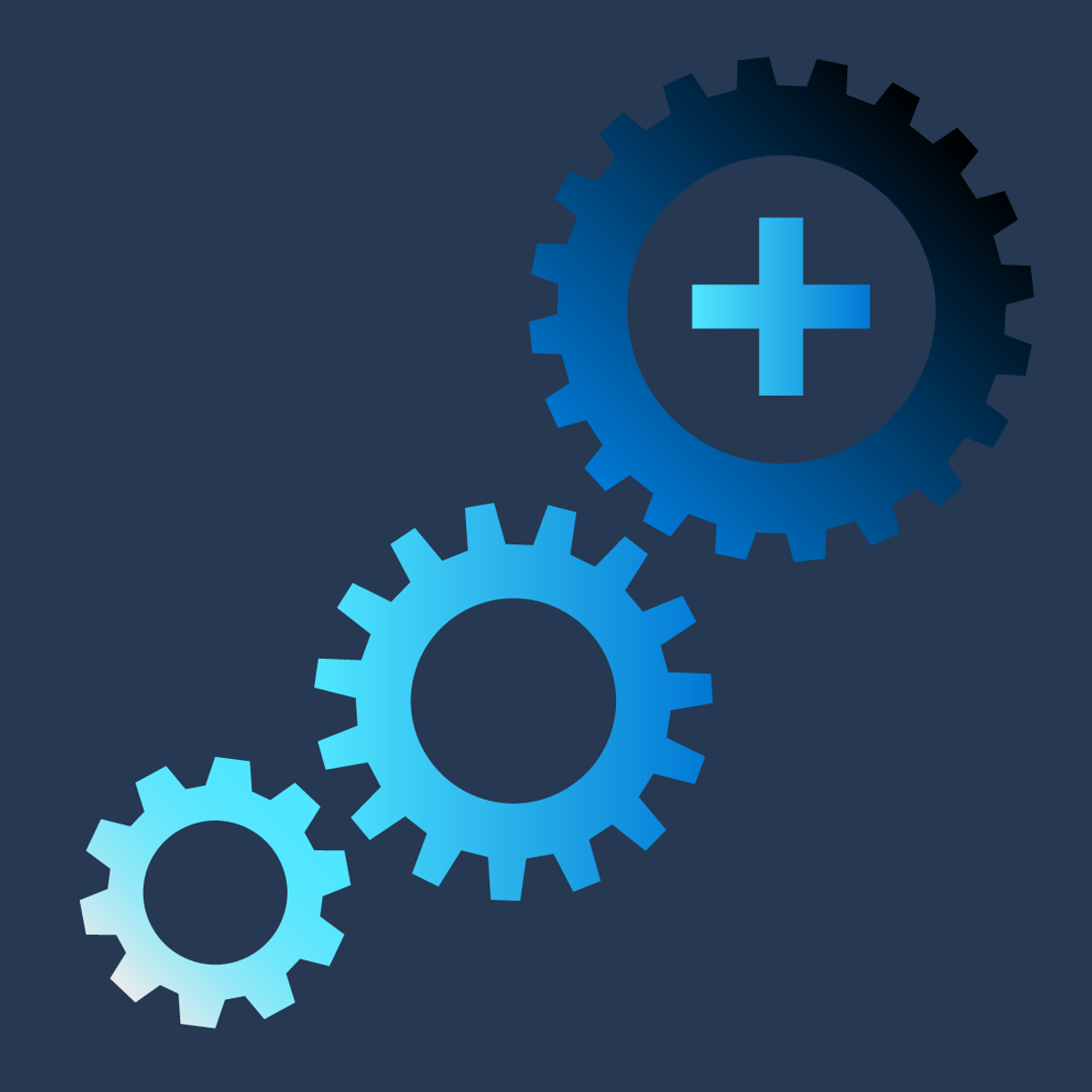
Microsoft Azure Machine Learning for Data Scientists
Machine learning is at the core of artificial intelligence, and many modern applications and services depend on predictive machine learning models. Training a machine learning model is an iterative process that requires time and compute resources. Automated machine learning can help make it easier. In this course, you will learn how to use Azure Machine Learning to create and publish models without writing code.
This is the second course in a five-course program that prepares you to take the DP-100: Designing and Implementing a Data Science Solution on Azurecertification exam.
The certification exam is an opportunity to prove knowledge and expertise operate machine learning solutions at a cloud-scale using Azure Machine Learning. This specialization teaches you to leverage your existing knowledge of Python and machine learning to manage data ingestion and preparation, model training and deployment, and machine learning solution monitoring in Microsoft Azure. Each course teaches you the concepts and skills that are measured by the exam.
This Specialization is intended for data scientists with existing knowledge of Python and machine learning frameworks like Scikit-Learn, PyTorch, and Tensorflow, who want to build and operate machine learning solutions in the cloud. It teaches data scientists how to create end-to-end solutions in Microsoft Azure. Students will learn how to manage Azure resources for machine learning; run experiments and train models; deploy and operationalize machine learning solutions, and implement responsible machine learning. They will also learn to use Azure Databricks to explore, prepare, and model data; and integrate Databricks machine learning processes with Azure Machine Learning.
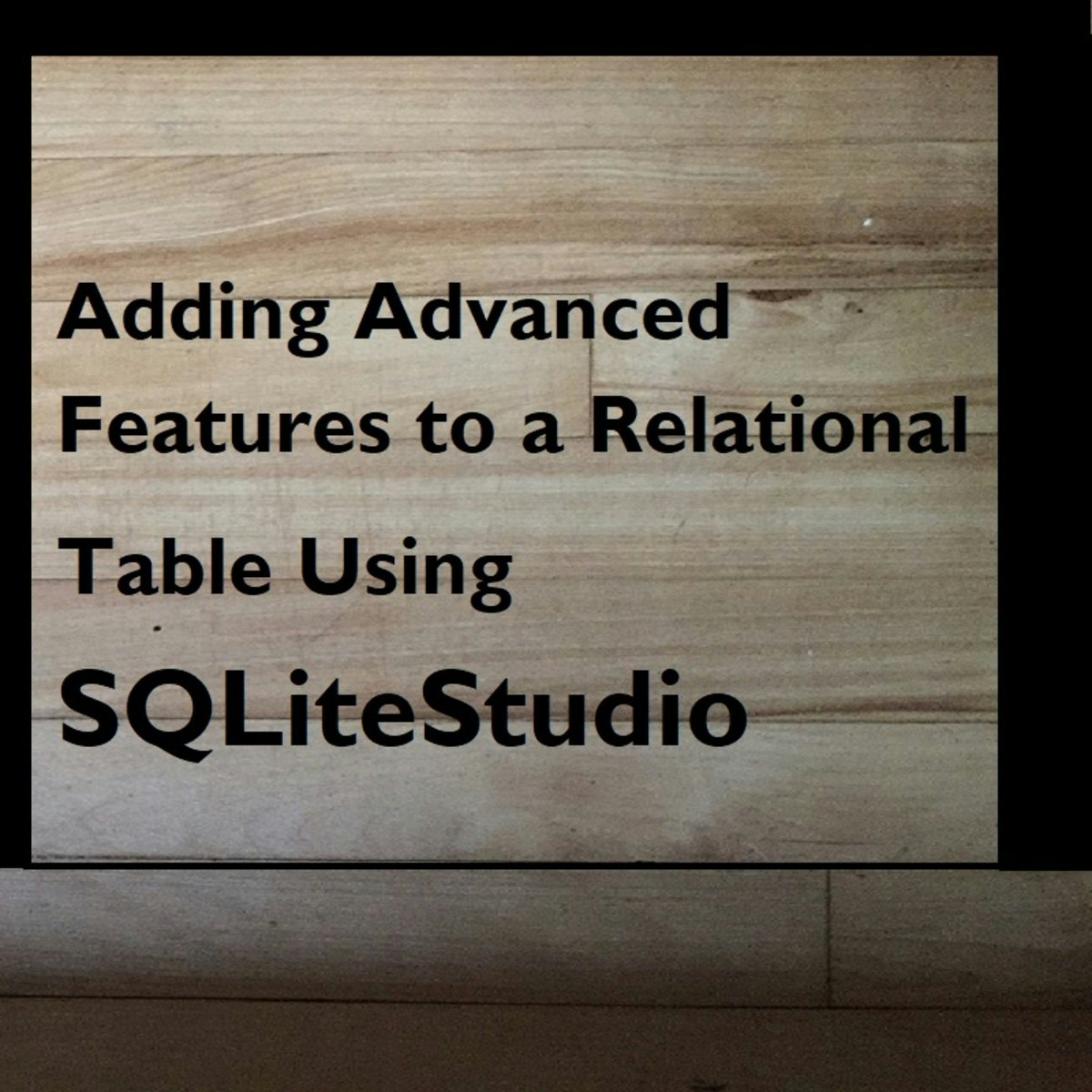
Advanced Features with Relational Database Tables Using SQLiteStudio
In this course, you’ll increase your knowledge of and experience with relational tables as you explore alternative ways of getting data into tables. You’ll also look at some of the advanced features that can give relational tables super powers. As you learn about the new features, you’ll use SQLiteStudio to apply them to your tables. Those features will enable your tables to more efficiently manage data—while keeping your data safe and accurate.
Tables are great for data storage. The concept of organizing data in rows and columns is familiar to most people. Accountants use spreadsheets to organize financial data, making it easier to budget and track expenses. Parents use lists with columns to track their family’s schedules so that everyone gets to participate in outside activities. Even the Internal Revenue Service gets in the game by using tax tables to provide tax amounts for a variety of incomes. Even a simple grocery list is tabular in nature. Each row is an item, with one column having the item's name/description, and a second column noting the quantity needed. It’s no surprise that database designers like to use tables in a relational database to organize and store data. In the Design and Create a Relational Database Table Using SQLiteStudio course you learned about tables. You created and populated a relational table using the SQLiteStudio database management system. That was a great beginning. Now it's time for the next step!
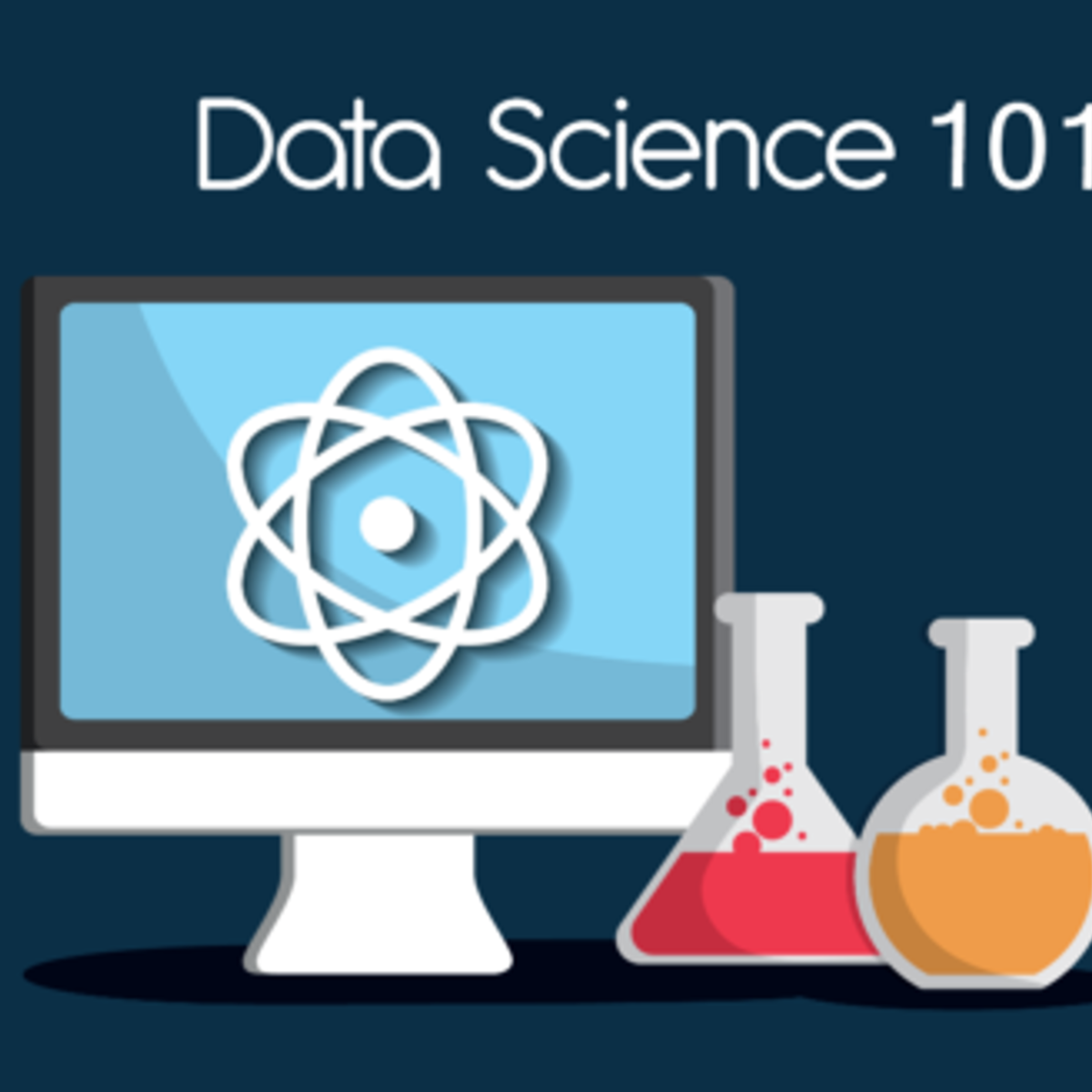
What is Data Science?
Do you want to know why Data Science has been labelled as the sexiest profession of the 21st century? After taking this course you will be able to answer this question, and get a thorough understanding of what is Data Science, what data scientists do, and learn about career paths in the field.
The art of uncovering the insights and trends in data has been around since ancient times. The ancient Egyptians used census data to increase efficiency in tax collection and they accurately predicted the flooding of the Nile river every year. Since then, people using data to derive insights and predict outcomes have carved out a unique and distinct field for the work they do. This field is data science.
In today's world, we use Data Science to find patterns in data, and make meaningful, data driven conclusions and predictions.
This course is for everyone, and teaches concepts like Machine Learning, Deep Learning, and Neural Networks and how companies apply data science in business.
You will meet several data scientists, who will share their insights and experiences in Data Science. By taking this introductory course, you will begin your journey into the thriving field that is Data Science!
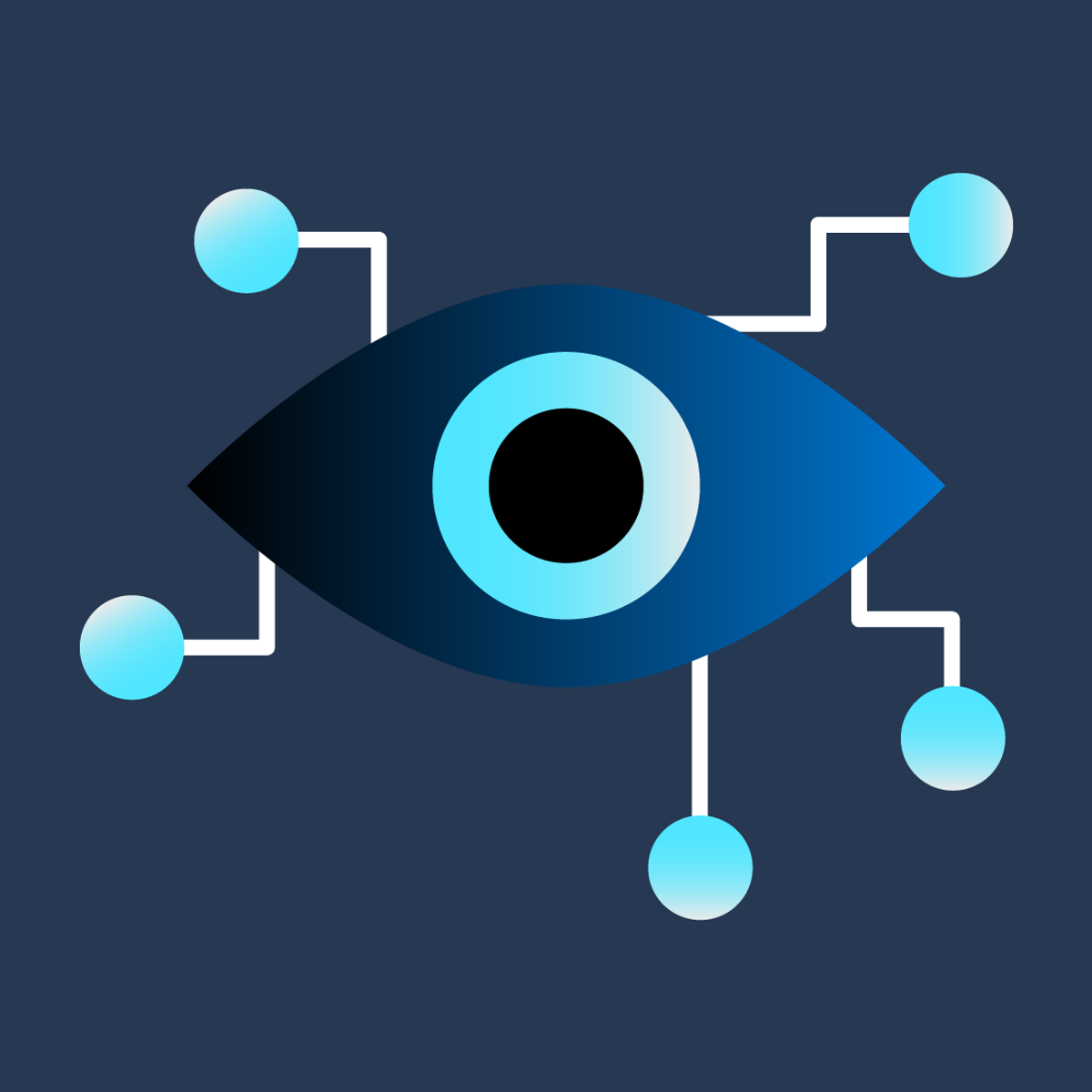
Computer Vision in Microsoft Azure
In Microsoft Azure, the Computer Vision cognitive service uses pre-trained models to analyze images, enabling software developers to easily build applications"see" the world and make sense of it. This ability to process images is the key to creating software that can emulate human visual perception. In this course, you'll explore some of these capabilities as you learn how to use the Computer Vision service to analyze images.
This course will help you prepare for Exam AI-900: Microsoft Azure AI Fundamentals. This is the third course in a five-course program that prepares you to take the AI-900 certification exam. This course teaches you the core concepts and skills that are assessed in the AI fundamentals exam domains. This beginner course is suitable for IT personnel who are just beginning to work with Microsoft Azure and want to learn about Microsoft Azure offerings and get hands-on experience with the product. Microsoft Azure AI Fundamentals can be used to prepare for other Azure role-based certifications like Microsoft Azure Data Scientist Associate or Microsoft Azure AI Engineer Associate, but it is not a prerequisite for any of them.
This course is intended for candidates with both technical and non-technical backgrounds. Data science and software engineering experience is not required; however, some general programming knowledge or experience would be beneficial. To be successful in this course, you need to have basic computer literacy and proficiency in the English language. You should be familiar with basic computing concepts and terminology, general technology concepts, including concepts of machine learning and artificial intelligence.
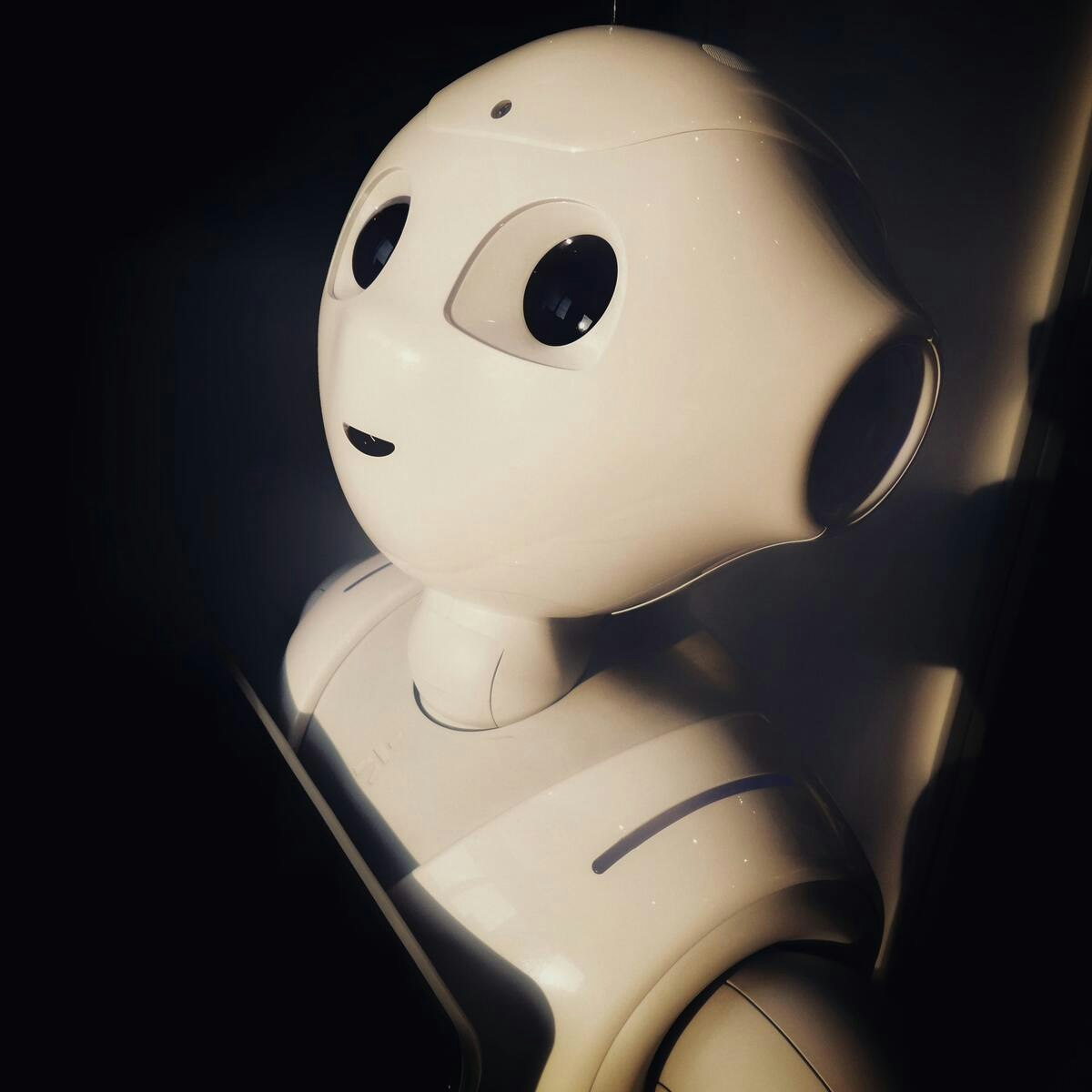
Machine Learning with H2O Flow
This is a hands-on, guided introduction to using H2O Flow for machine learning. By the end of this project, you will be able to train and evaluate machine learning models with H2O Flow and AutoML, without writing a single line of code! You will use the point and click, web-based interface to H2O called Flow to solve a business analytics problem with machine learning.
H2O is a leading open-source machine learning and artificial intelligence platform trusted by data scientists and machine learning practitioners. It has APIs available in R, Python, Scala, and also a web-based point and click interface called Flow. H2O's AutoML automates the process of training and tuning a large selection of models, allowing the user to focus on other aspects of the data science and machine learning pipelines such as data pre-processing, feature engineering, and model deployment.
To get the most out of this project, we recommend that you have an understanding of basic machine learning theory, and have trained machine learning models.
Note: This course works best for learners who are based in the North America region. We’re currently working on providing the same experience in other regions.
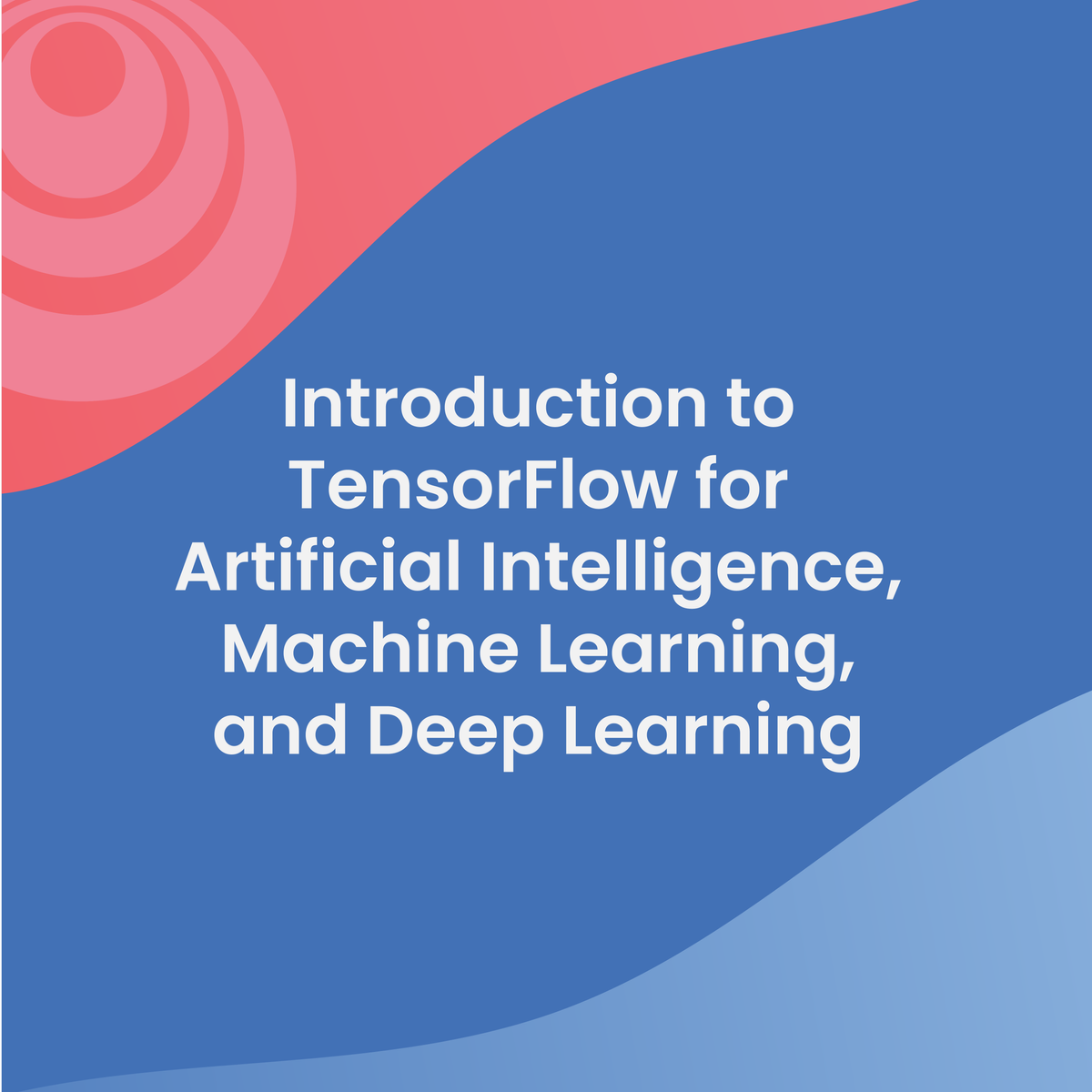
Introduction to TensorFlow for Artificial Intelligence, Machine Learning, and Deep Learning
If you are a software developer who wants to build scalable AI-powered algorithms, you need to understand how to use the tools to build them. This course is part of the upcoming Machine Learning in Tensorflow Specialization and will teach you best practices for using TensorFlow, a popular open-source framework for machine learning.
The Machine Learning course and Deep Learning Specialization from Andrew Ng teach the most important and foundational principles of Machine Learning and Deep Learning. This new deeplearning.ai TensorFlow Specialization teaches you how to use TensorFlow to implement those principles so that you can start building and applying scalable models to real-world problems. To develop a deeper understanding of how neural networks work, we recommend that you take the Deep Learning Specialization.
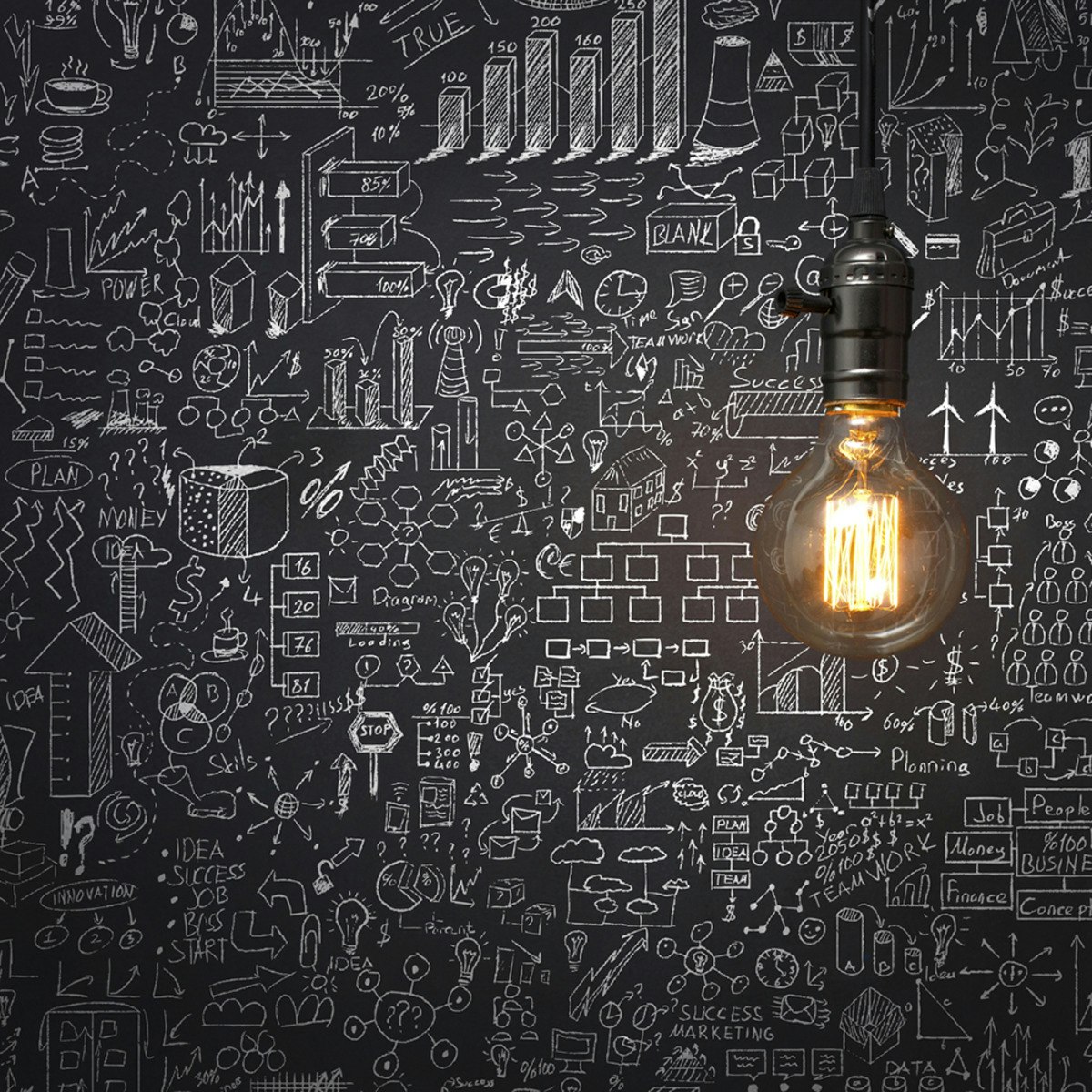
Hands-on Text Mining and Analytics
This course provides an unique opportunity for you to learn key components of text mining and analytics aided by the real world datasets and the text mining toolkit written in Java. Hands-on experience in core text mining techniques including text preprocessing, sentiment analysis, and topic modeling help learners be trained to be a competent data scientists.
Empowered by bringing lecture notes together with lab sessions based on the y-TextMiner toolkit developed for the class, learners will be able to develop interesting text mining applications.
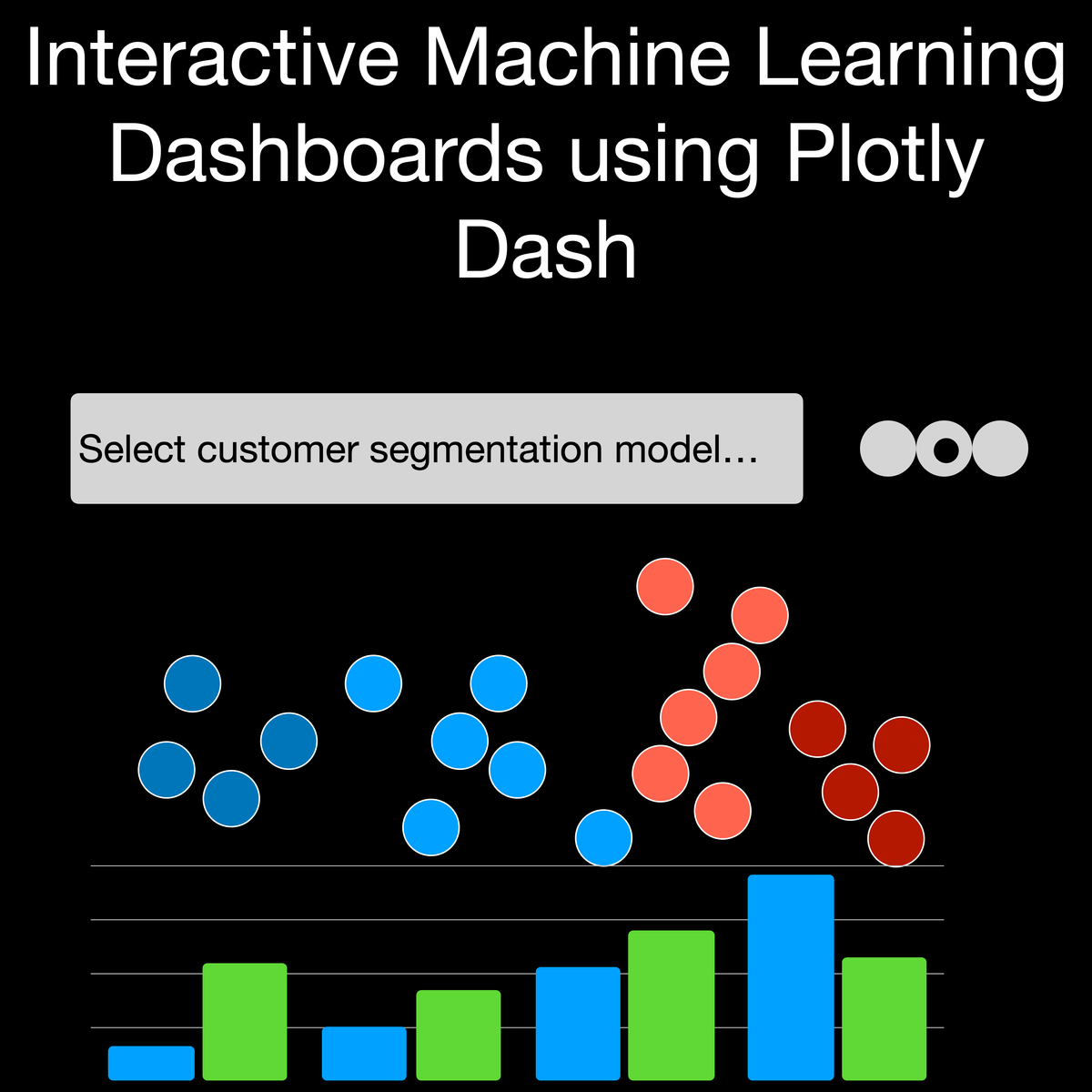
Interactive Machine Learning Dashboards using Plotly Dash
In this 2 hour long project-based course, you will learn how to create an HTML outline of a Plotly Dash dashboard. You will design interactive dropdown lists, radio buttons, and most importantly, scatter plots and bar charts that respond to your mouse's hover. You will learn how to visualize dimensionality reduction results intuitively and interactively, and see how these models can be used in Customer Segmentation. Furthermore, we will discuss how to critically evaluate these models, and what to look out for in a well-performing model.
Note: This course works best for learners who are based in the North America region. We’re currently working on providing the same experience in other regions.
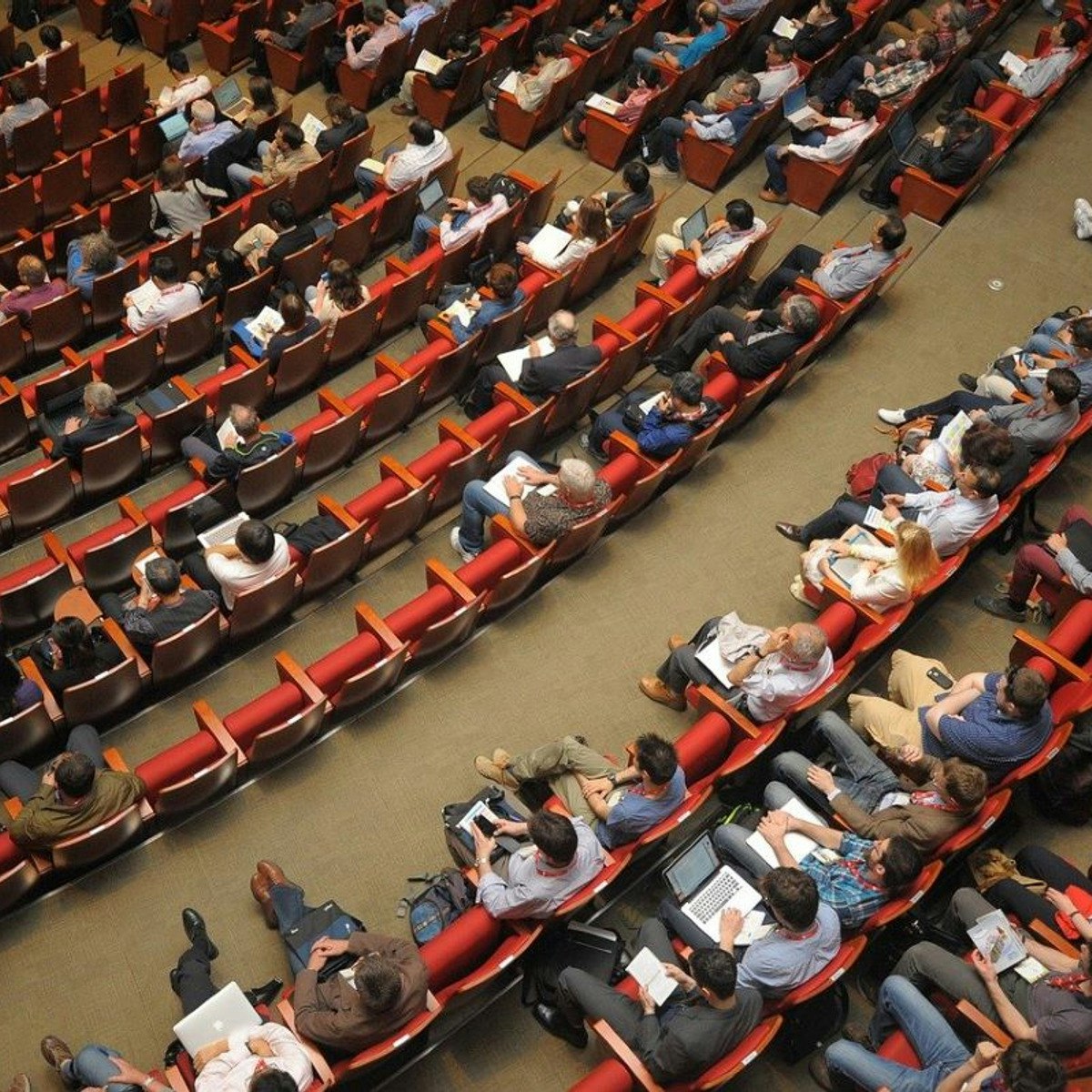
Quantitative Text Analysis and Evaluating Lexical Style in R
By the end of this project, you will learn about the concept of lexical style in textual analysis in R. You will know how to load and pre-process a data set of text documents by converting the data set into a corpus and document feature matrix. You will know how to calculate the type to token ration which evaluates the level of complexity of a text, and know how to isolate terms of particular lexical interest in a text and visualize the variation in frequency of such terms in texts over time.
Popular Internships and Jobs by Categories
Browse
© 2024 BoostGrad | All rights reserved