Back to Courses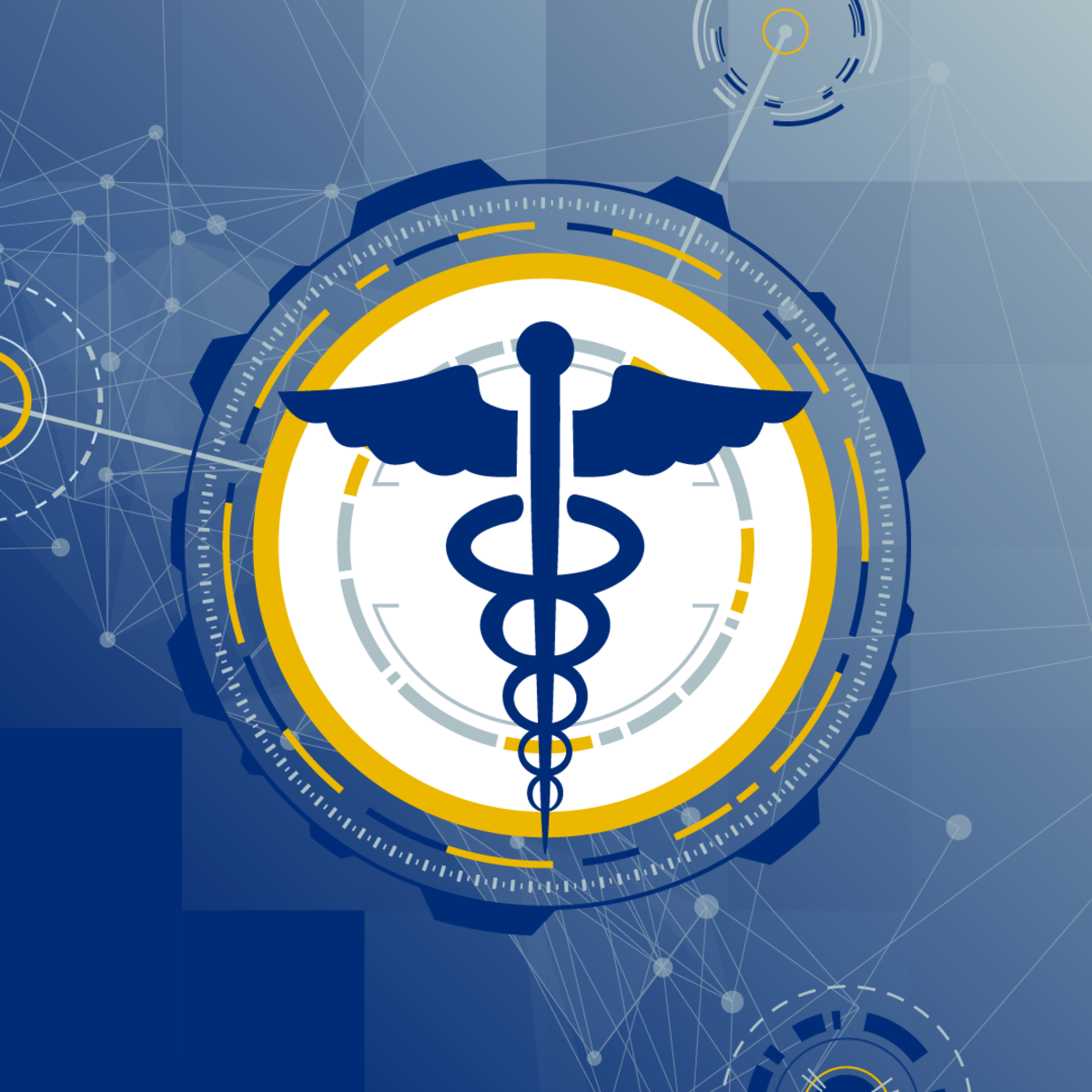
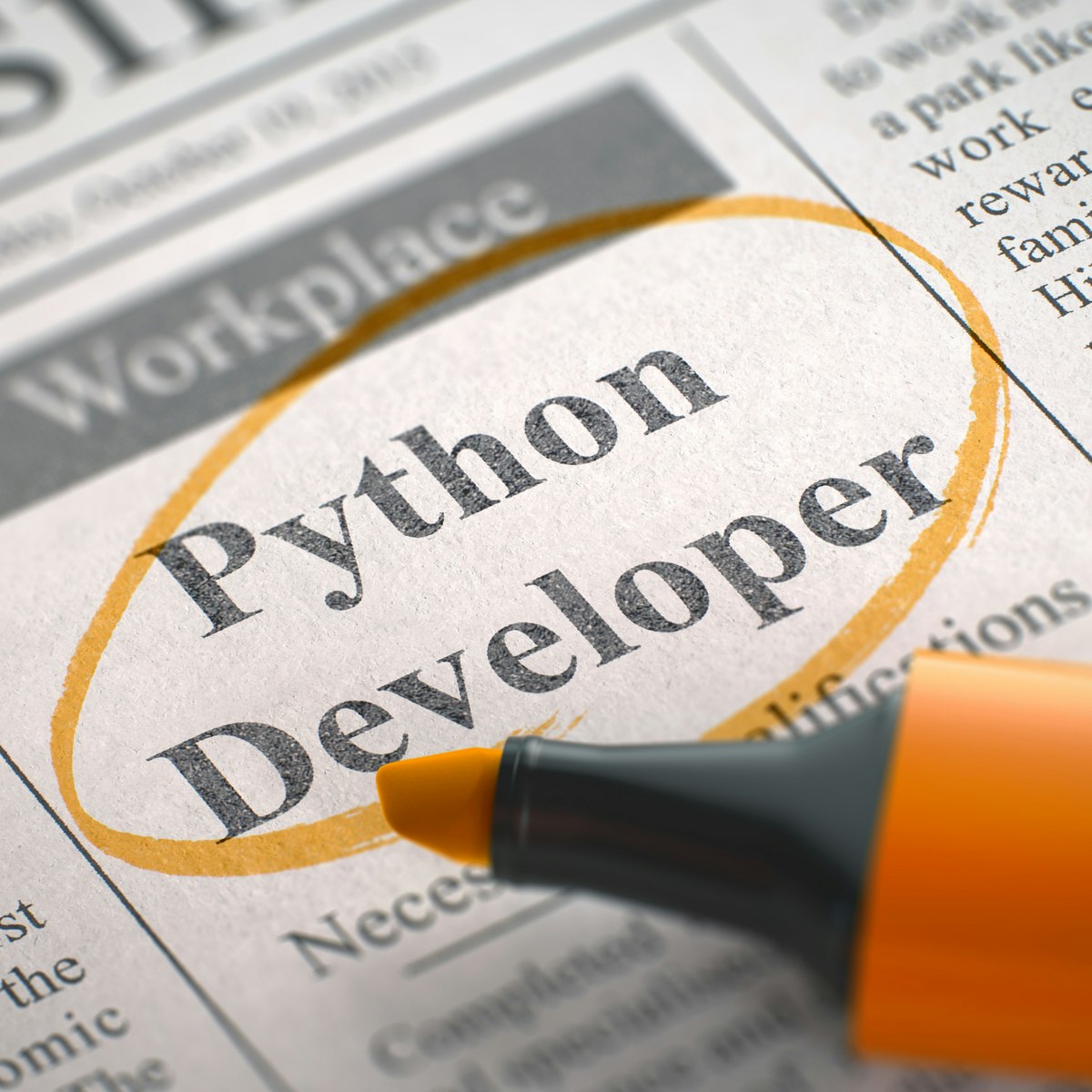
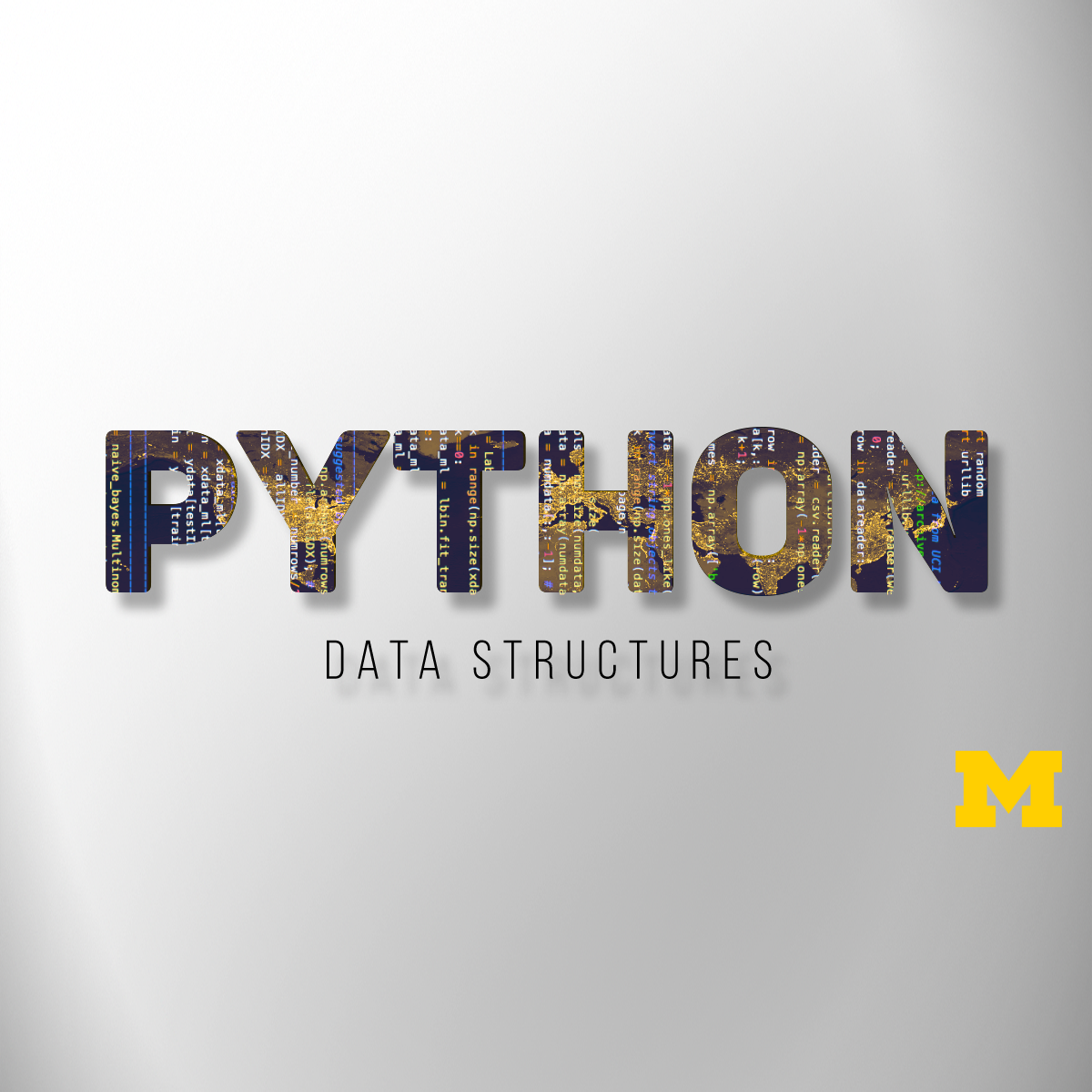
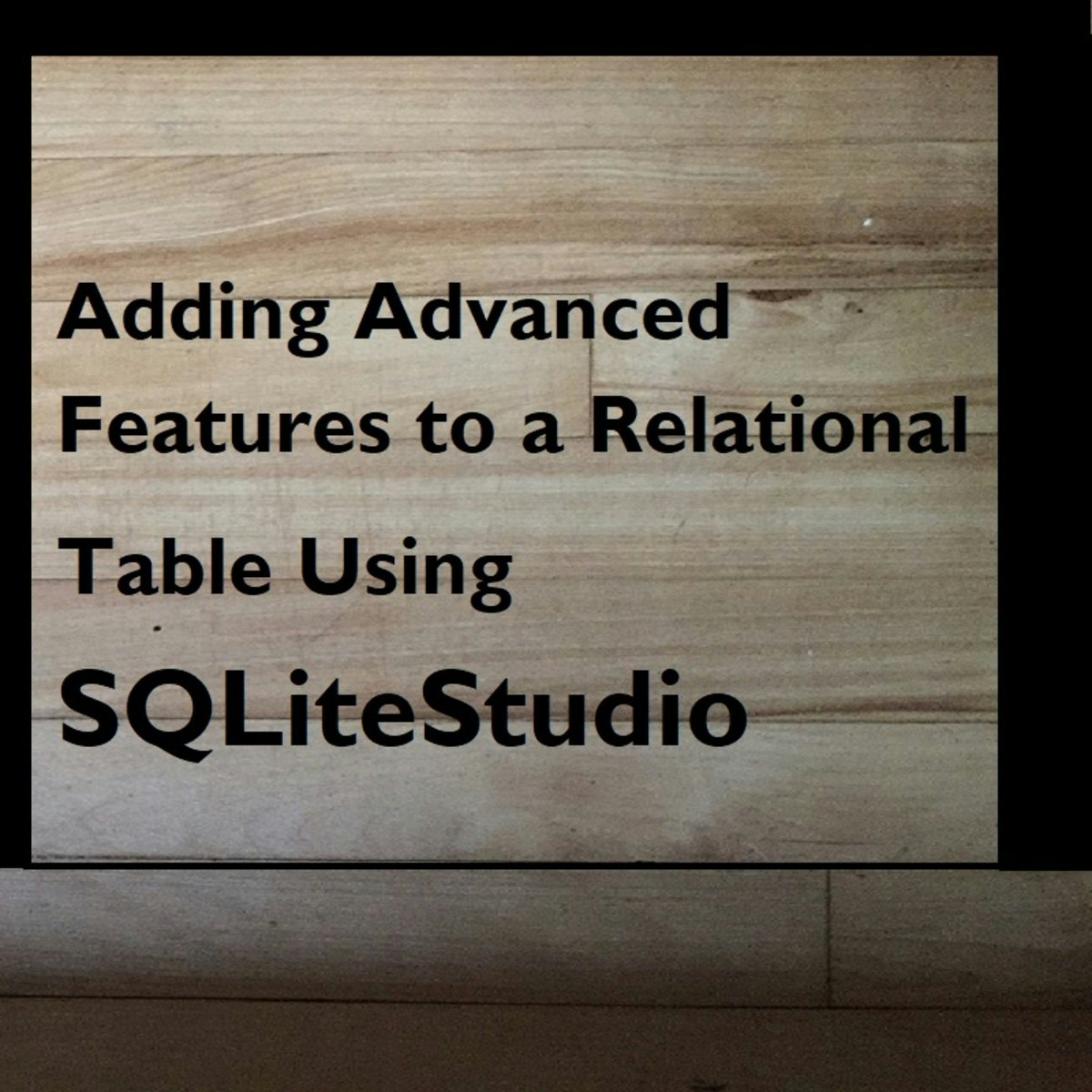
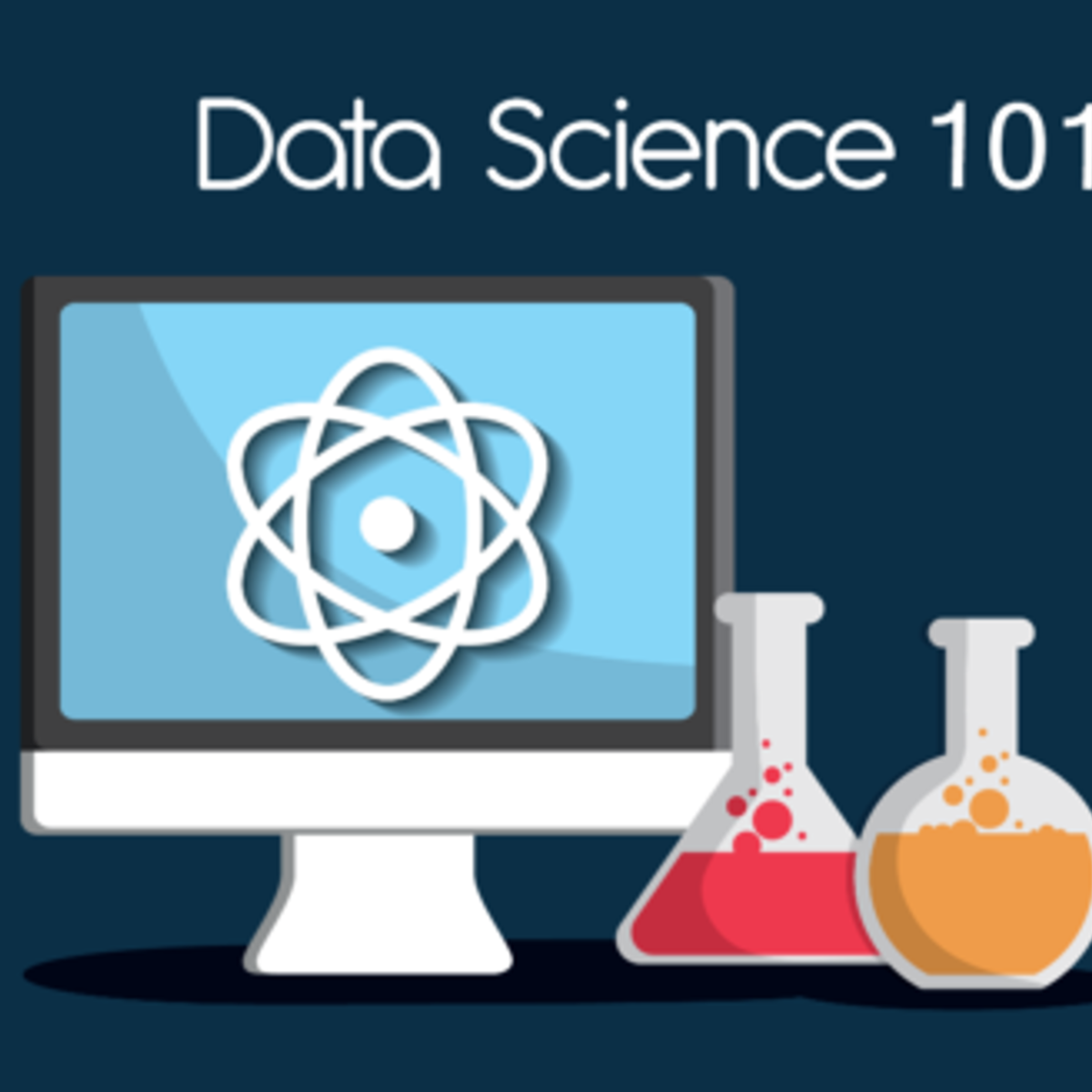
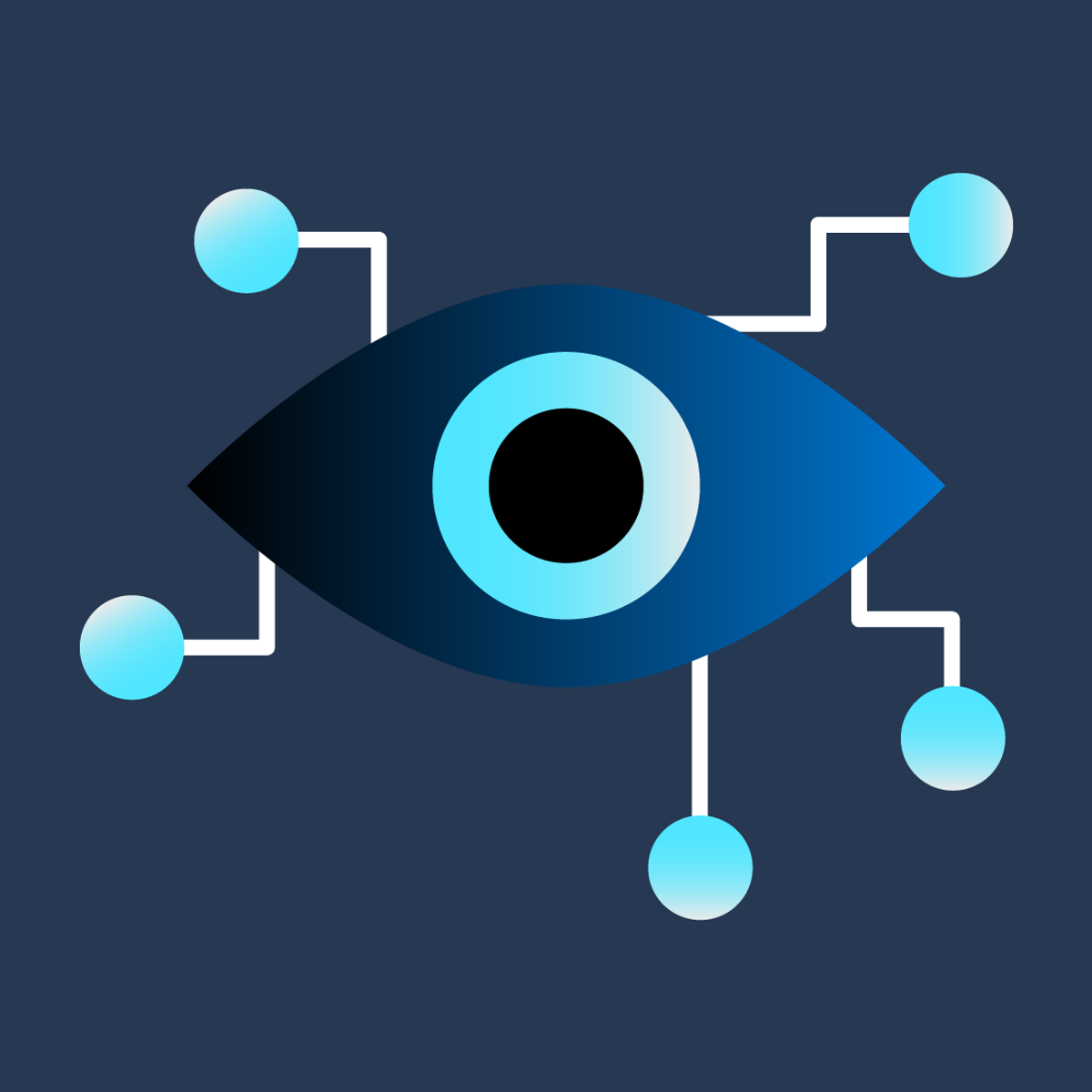
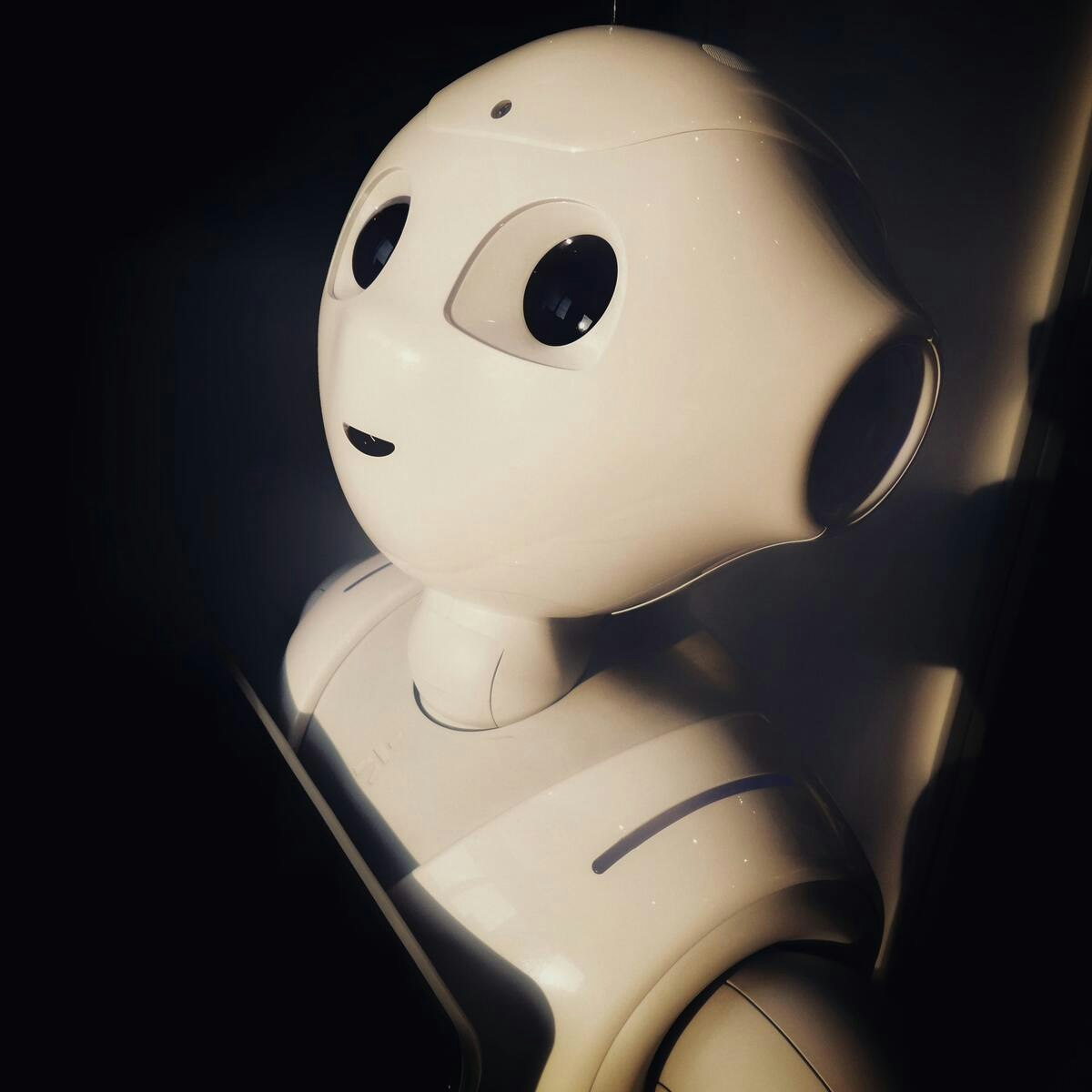
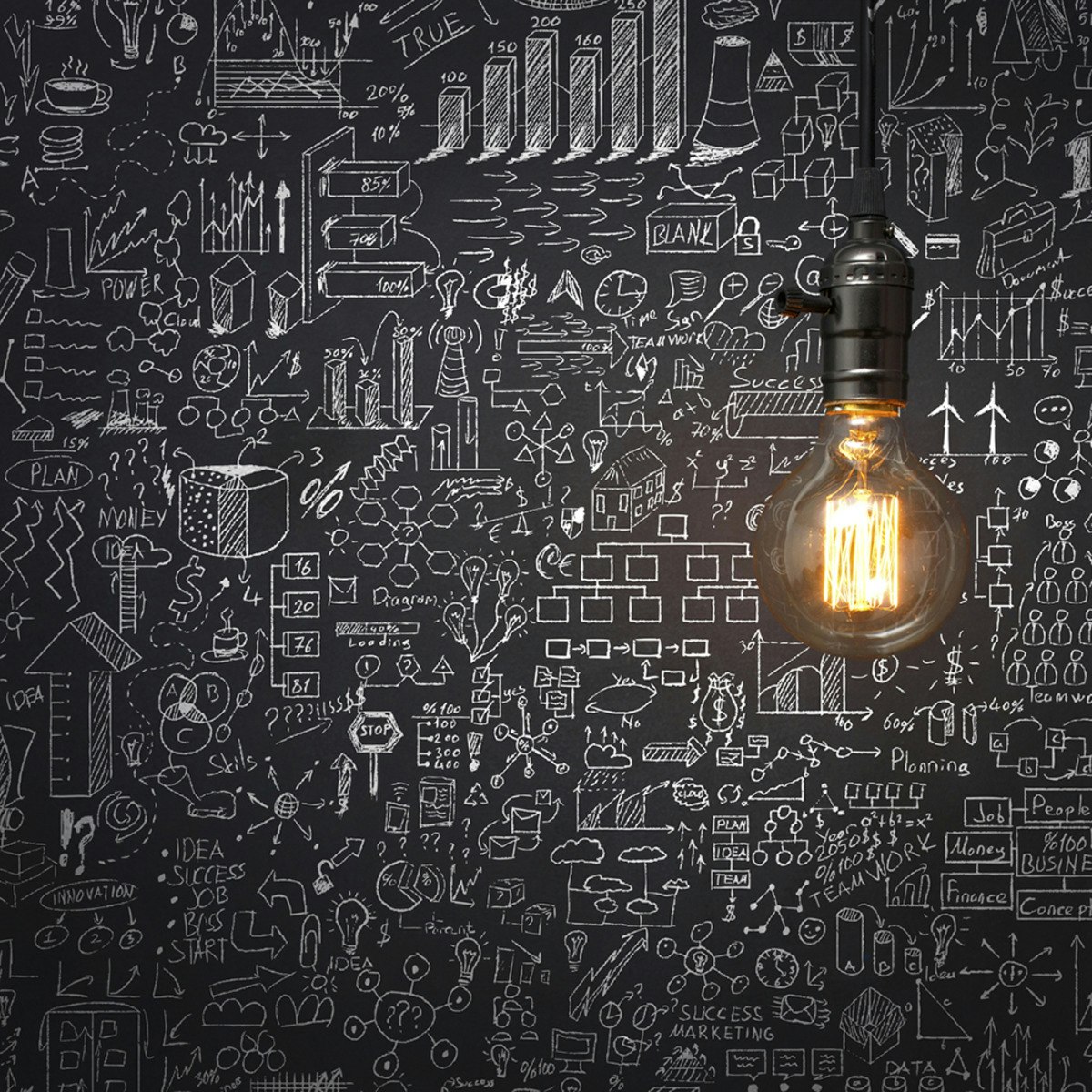
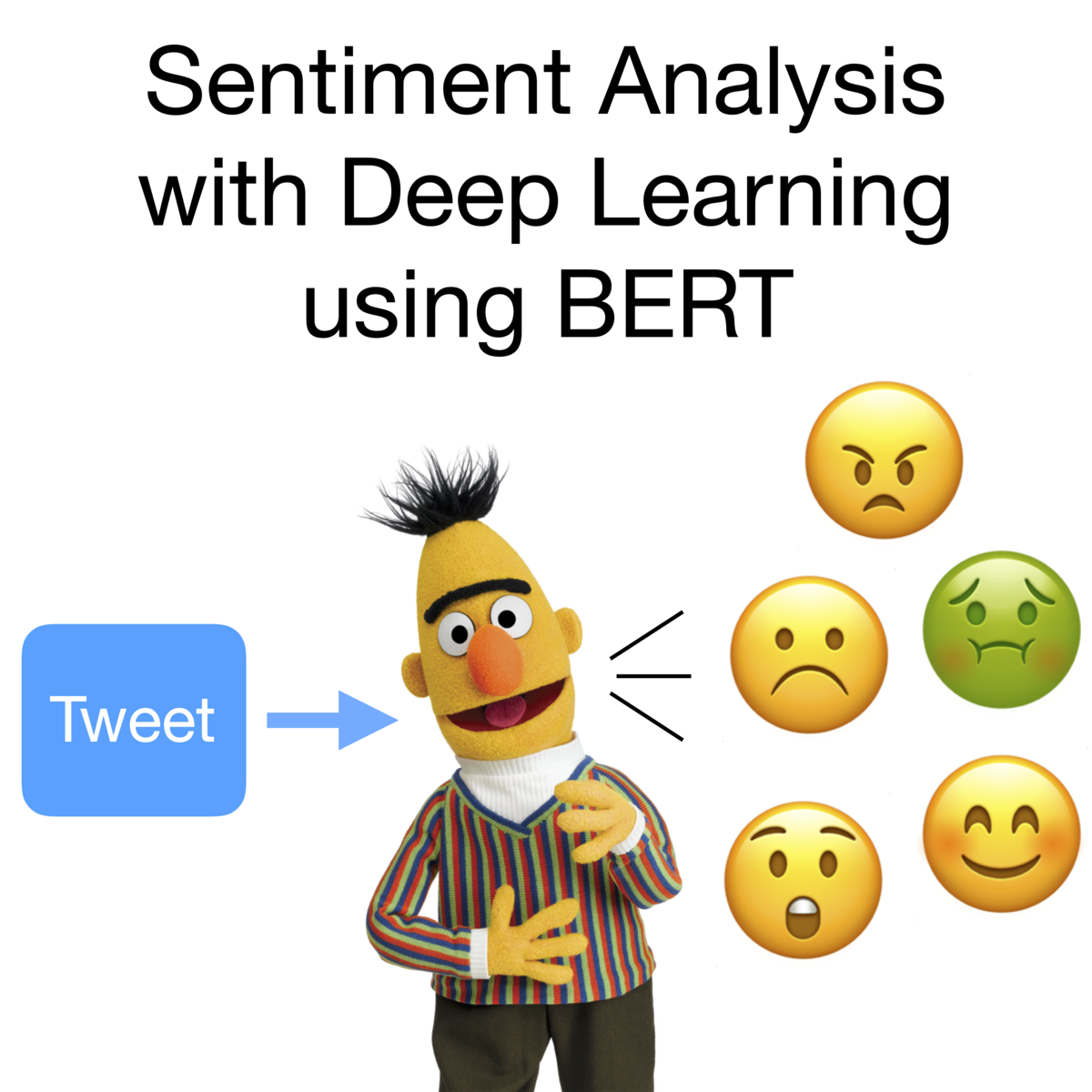
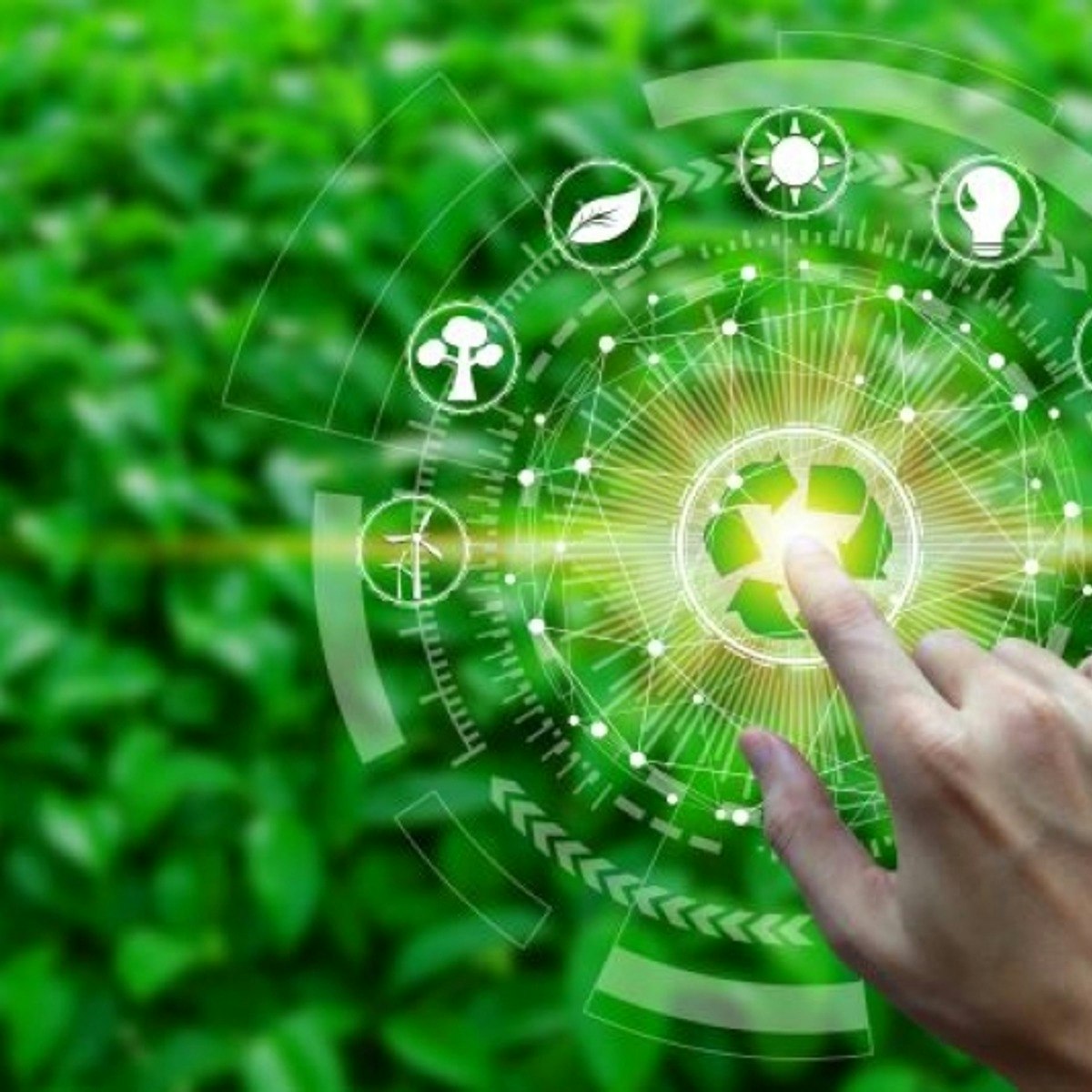
Data Science Courses - Page 7
Showing results 61-70 of 1407
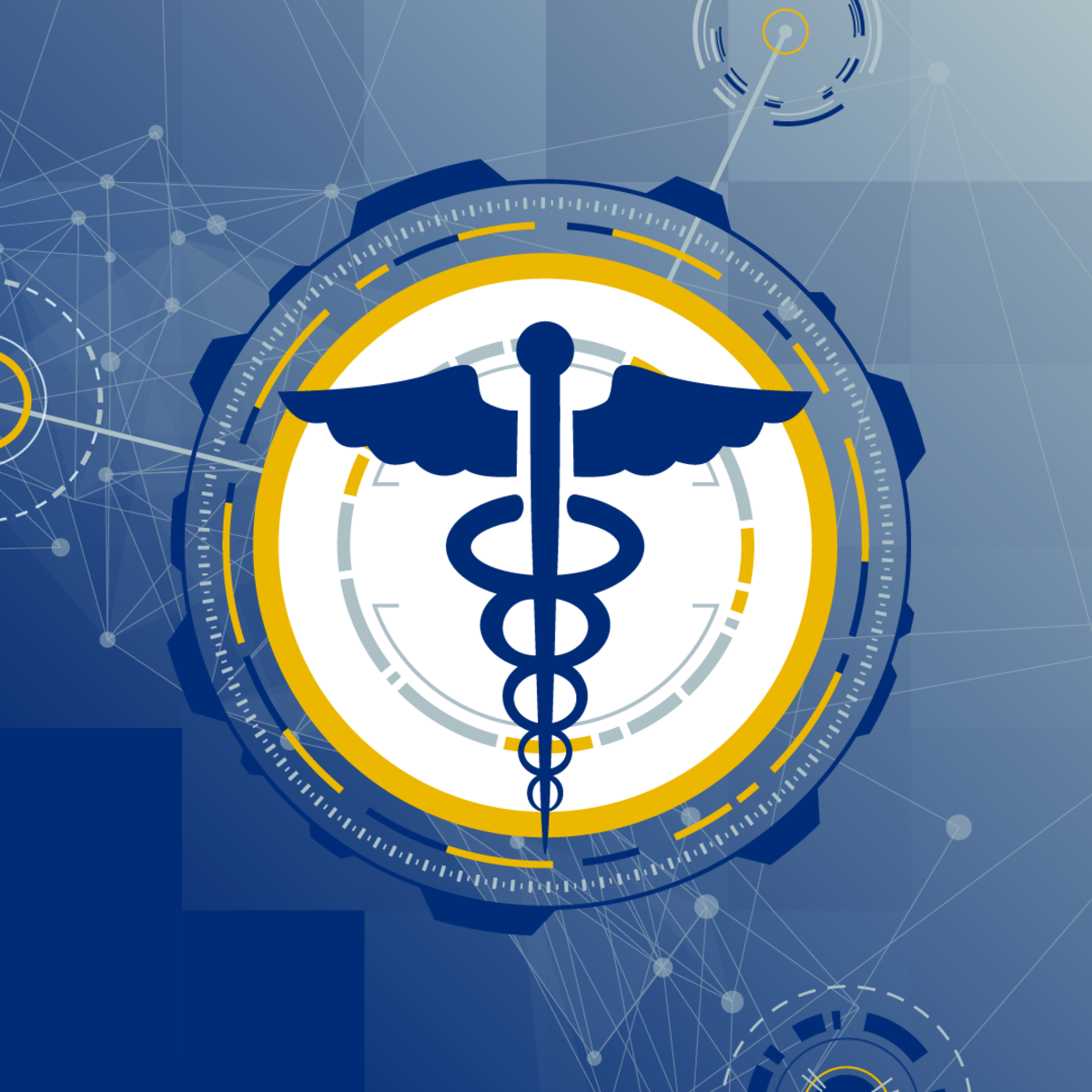
The Data Science of Health Informatics
Health data are notable for how many types there are, how complex they are, and how serious it is to get them straight. These data are used for treatment of the patient from whom they derive, but also for other uses. Examples of such secondary use of health data include population health (e.g., who requires more attention), research (e.g., which drug is more effective in practice), quality (e.g., is the institution meeting benchmarks), and translational research (e.g., are new technologies being applied appropriately). By the end of this course, students will recognize the different types of health and healthcare data, will articulate a coherent and complete question, will interpret queries designed for secondary use of EHR data, and will interpret the results of those queries.
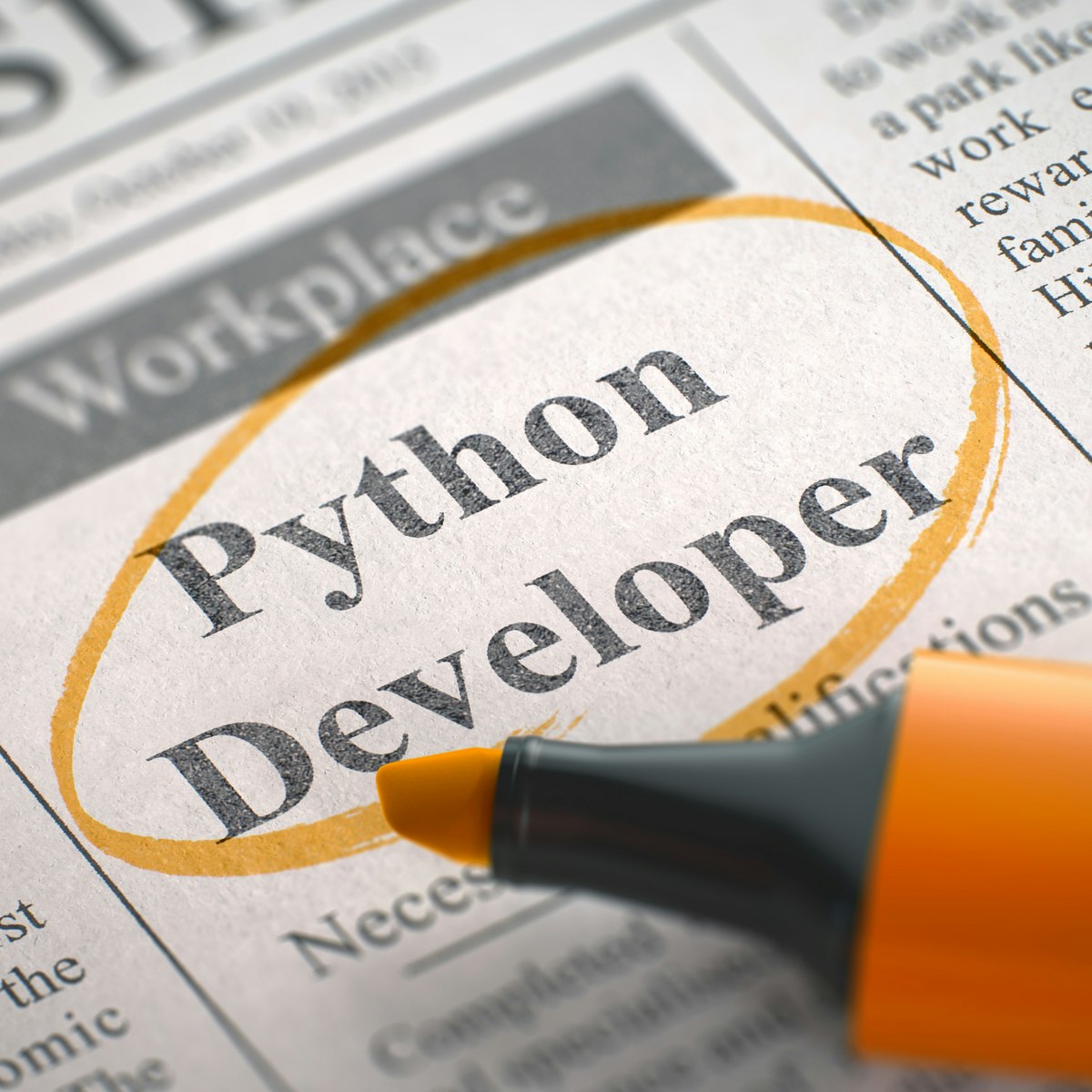
Python Data Visualization
This if the final course in the specialization which builds upon the knowledge learned in Python Programming Essentials, Python Data Representations, and Python Data Analysis. We will learn how to install external packages for use within Python, acquire data from sources on the Web, and then we will clean, process, analyze, and visualize that data. This course will combine the skills learned throughout the specialization to enable you to write interesting, practical, and useful programs.
By the end of the course, you will be comfortable installing Python packages, analyzing existing data, and generating visualizations of that data. This course will complete your education as a scripter, enabling you to locate, install, and use Python packages written by others. You will be able to effectively utilize tools and packages that are widely available to amplify your effectiveness and write useful programs.
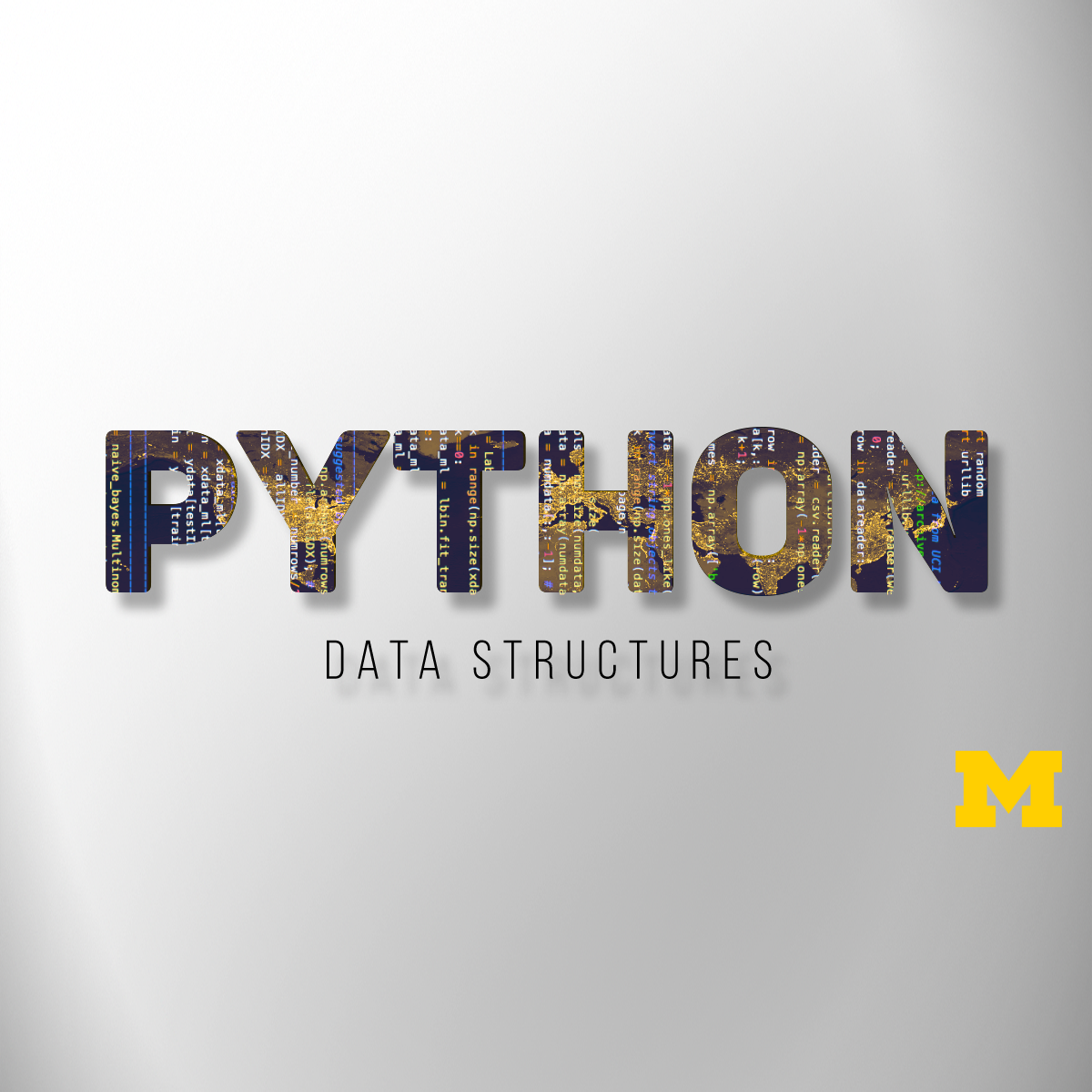
Python Data Structures
This course will introduce the core data structures of the Python programming language. We will move past the basics of procedural programming and explore how we can use the Python built-in data structures such as lists, dictionaries, and tuples to perform increasingly complex data analysis. This course will cover Chapters 6-10 of the textbook “Python for Everybody”. This course covers Python 3.
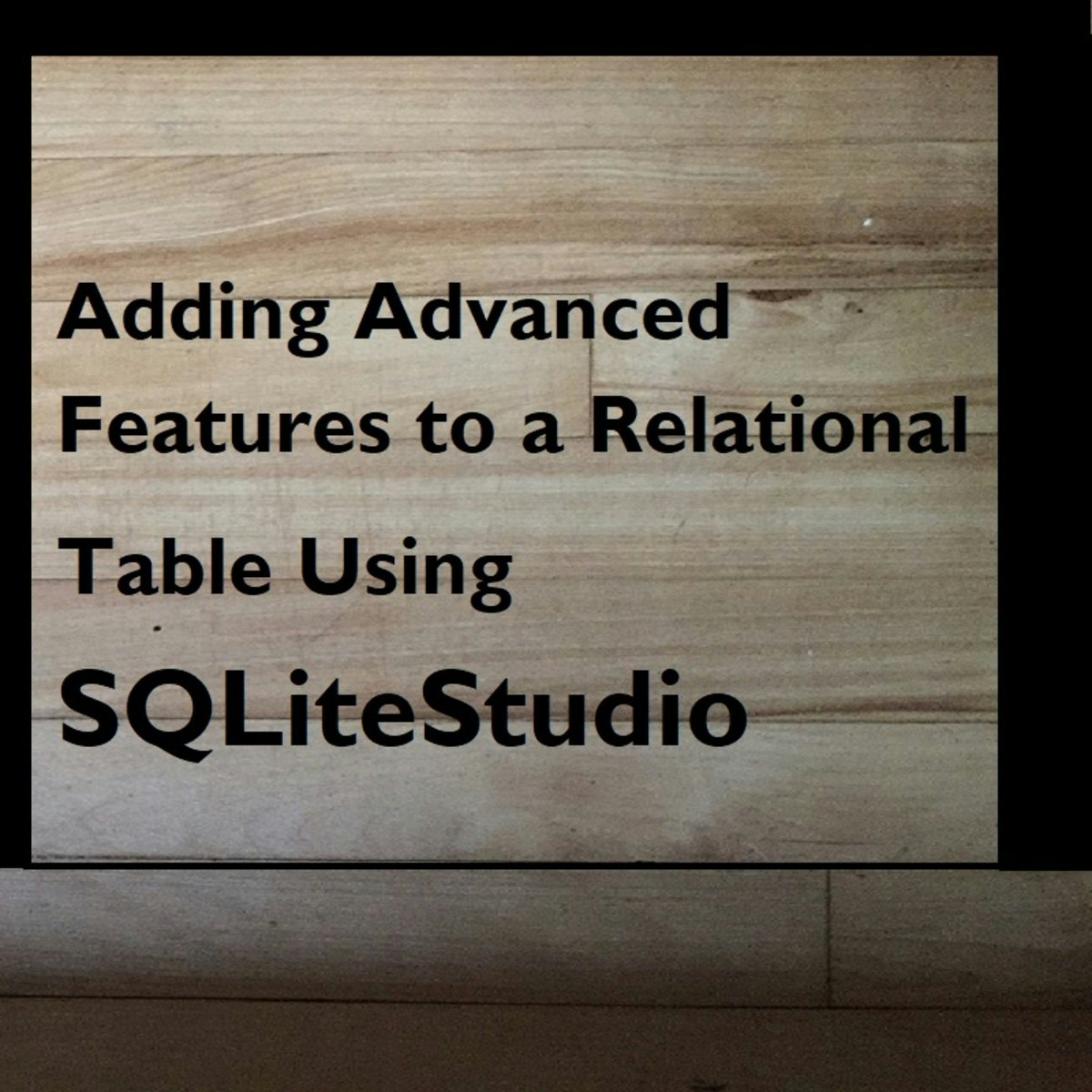
Advanced Features with Relational Database Tables Using SQLiteStudio
In this course, you’ll increase your knowledge of and experience with relational tables as you explore alternative ways of getting data into tables. You’ll also look at some of the advanced features that can give relational tables super powers. As you learn about the new features, you’ll use SQLiteStudio to apply them to your tables. Those features will enable your tables to more efficiently manage data—while keeping your data safe and accurate.
Tables are great for data storage. The concept of organizing data in rows and columns is familiar to most people. Accountants use spreadsheets to organize financial data, making it easier to budget and track expenses. Parents use lists with columns to track their family’s schedules so that everyone gets to participate in outside activities. Even the Internal Revenue Service gets in the game by using tax tables to provide tax amounts for a variety of incomes. Even a simple grocery list is tabular in nature. Each row is an item, with one column having the item's name/description, and a second column noting the quantity needed. It’s no surprise that database designers like to use tables in a relational database to organize and store data. In the Design and Create a Relational Database Table Using SQLiteStudio course you learned about tables. You created and populated a relational table using the SQLiteStudio database management system. That was a great beginning. Now it's time for the next step!
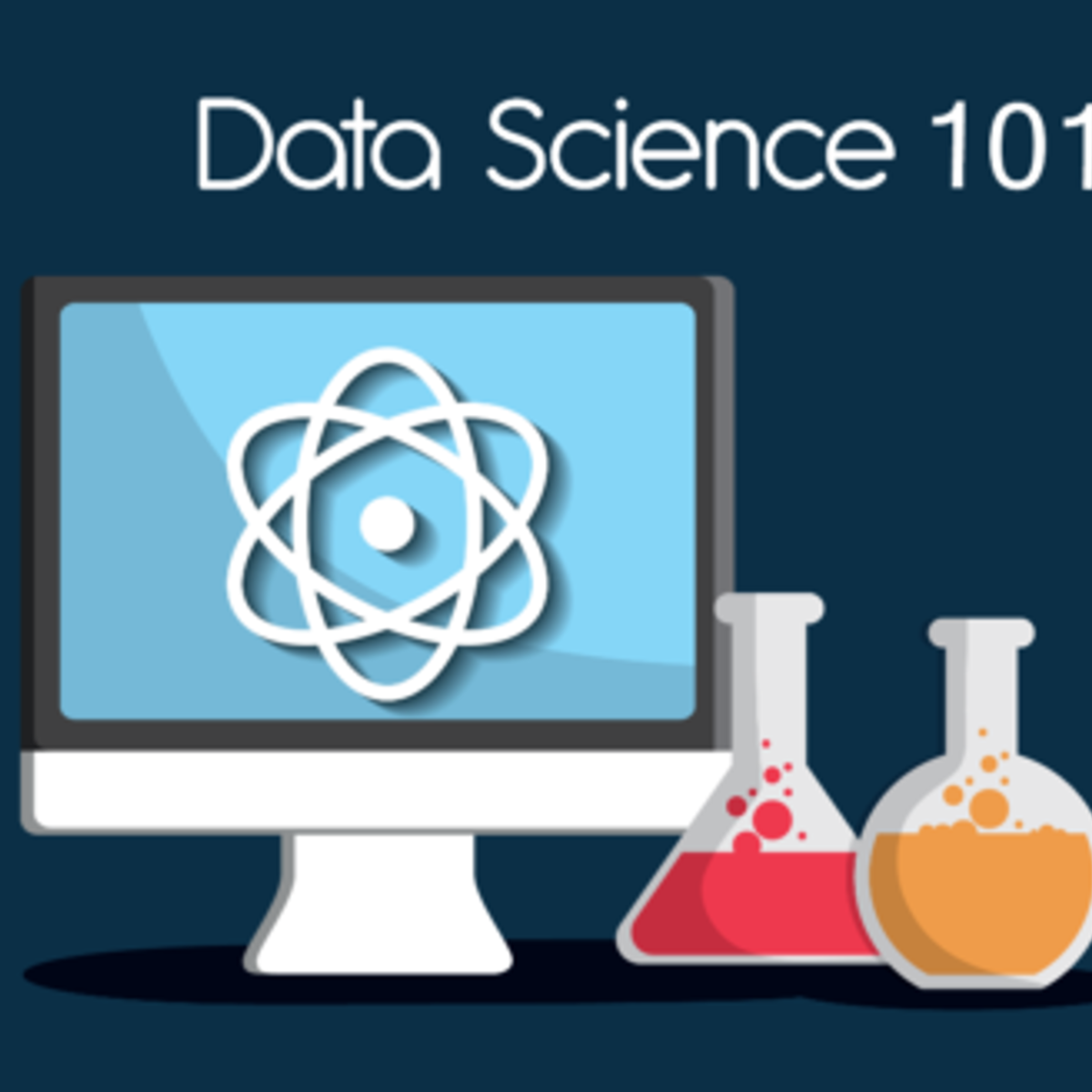
What is Data Science?
Do you want to know why Data Science has been labelled as the sexiest profession of the 21st century? After taking this course you will be able to answer this question, and get a thorough understanding of what is Data Science, what data scientists do, and learn about career paths in the field.
The art of uncovering the insights and trends in data has been around since ancient times. The ancient Egyptians used census data to increase efficiency in tax collection and they accurately predicted the flooding of the Nile river every year. Since then, people using data to derive insights and predict outcomes have carved out a unique and distinct field for the work they do. This field is data science.
In today's world, we use Data Science to find patterns in data, and make meaningful, data driven conclusions and predictions.
This course is for everyone, and teaches concepts like Machine Learning, Deep Learning, and Neural Networks and how companies apply data science in business.
You will meet several data scientists, who will share their insights and experiences in Data Science. By taking this introductory course, you will begin your journey into the thriving field that is Data Science!
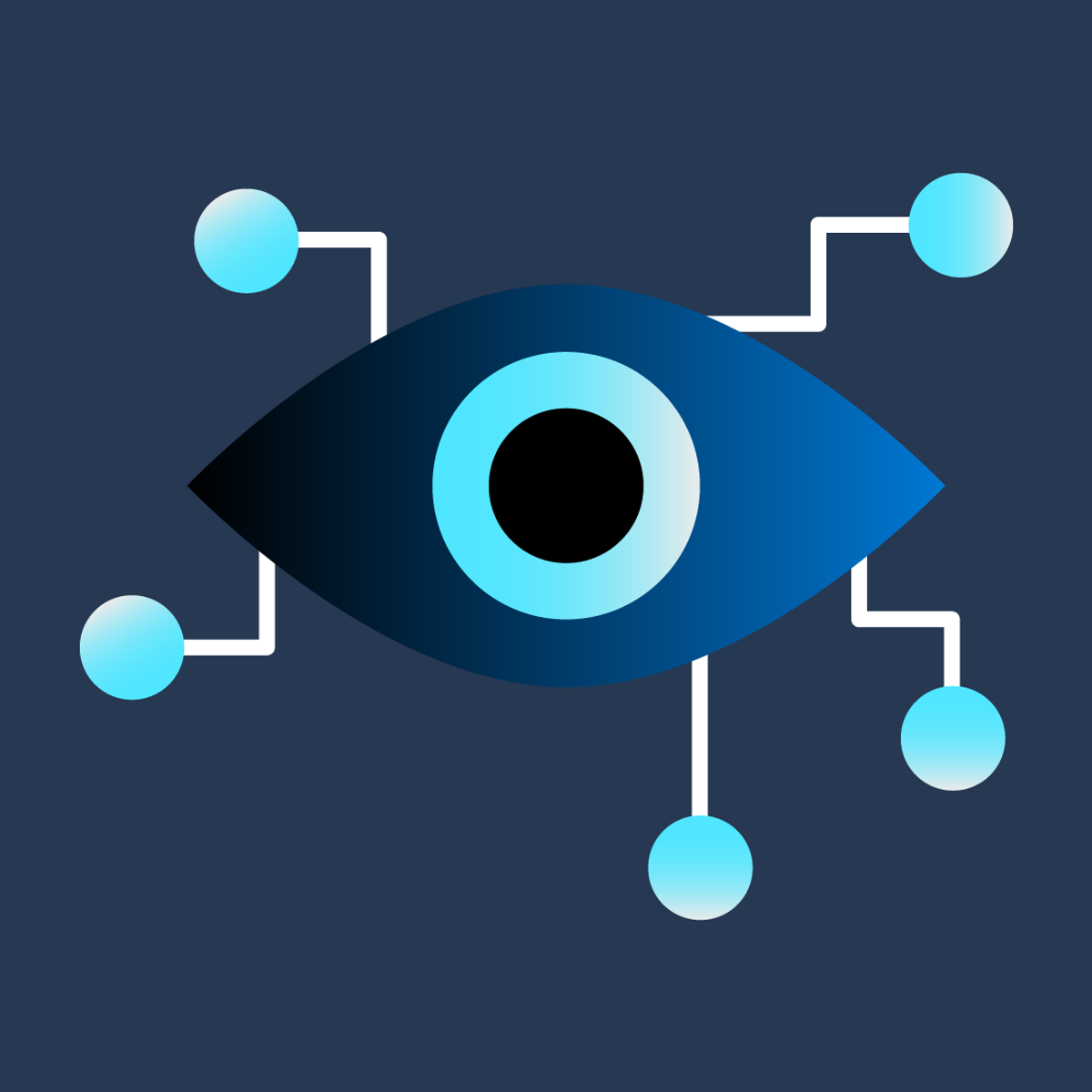
Computer Vision in Microsoft Azure
In Microsoft Azure, the Computer Vision cognitive service uses pre-trained models to analyze images, enabling software developers to easily build applications"see" the world and make sense of it. This ability to process images is the key to creating software that can emulate human visual perception. In this course, you'll explore some of these capabilities as you learn how to use the Computer Vision service to analyze images.
This course will help you prepare for Exam AI-900: Microsoft Azure AI Fundamentals. This is the third course in a five-course program that prepares you to take the AI-900 certification exam. This course teaches you the core concepts and skills that are assessed in the AI fundamentals exam domains. This beginner course is suitable for IT personnel who are just beginning to work with Microsoft Azure and want to learn about Microsoft Azure offerings and get hands-on experience with the product. Microsoft Azure AI Fundamentals can be used to prepare for other Azure role-based certifications like Microsoft Azure Data Scientist Associate or Microsoft Azure AI Engineer Associate, but it is not a prerequisite for any of them.
This course is intended for candidates with both technical and non-technical backgrounds. Data science and software engineering experience is not required; however, some general programming knowledge or experience would be beneficial. To be successful in this course, you need to have basic computer literacy and proficiency in the English language. You should be familiar with basic computing concepts and terminology, general technology concepts, including concepts of machine learning and artificial intelligence.
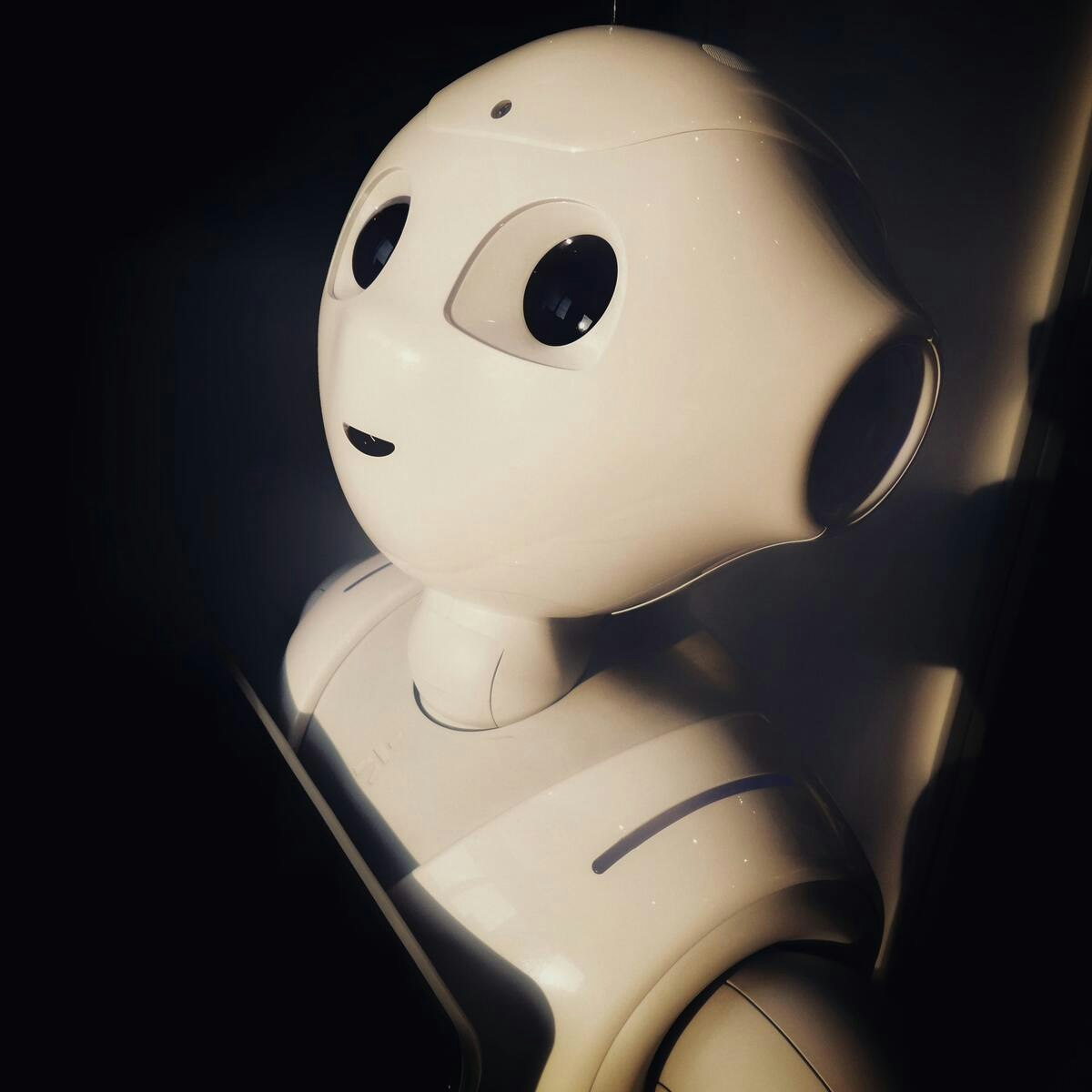
Machine Learning with H2O Flow
This is a hands-on, guided introduction to using H2O Flow for machine learning. By the end of this project, you will be able to train and evaluate machine learning models with H2O Flow and AutoML, without writing a single line of code! You will use the point and click, web-based interface to H2O called Flow to solve a business analytics problem with machine learning.
H2O is a leading open-source machine learning and artificial intelligence platform trusted by data scientists and machine learning practitioners. It has APIs available in R, Python, Scala, and also a web-based point and click interface called Flow. H2O's AutoML automates the process of training and tuning a large selection of models, allowing the user to focus on other aspects of the data science and machine learning pipelines such as data pre-processing, feature engineering, and model deployment.
To get the most out of this project, we recommend that you have an understanding of basic machine learning theory, and have trained machine learning models.
Note: This course works best for learners who are based in the North America region. We’re currently working on providing the same experience in other regions.
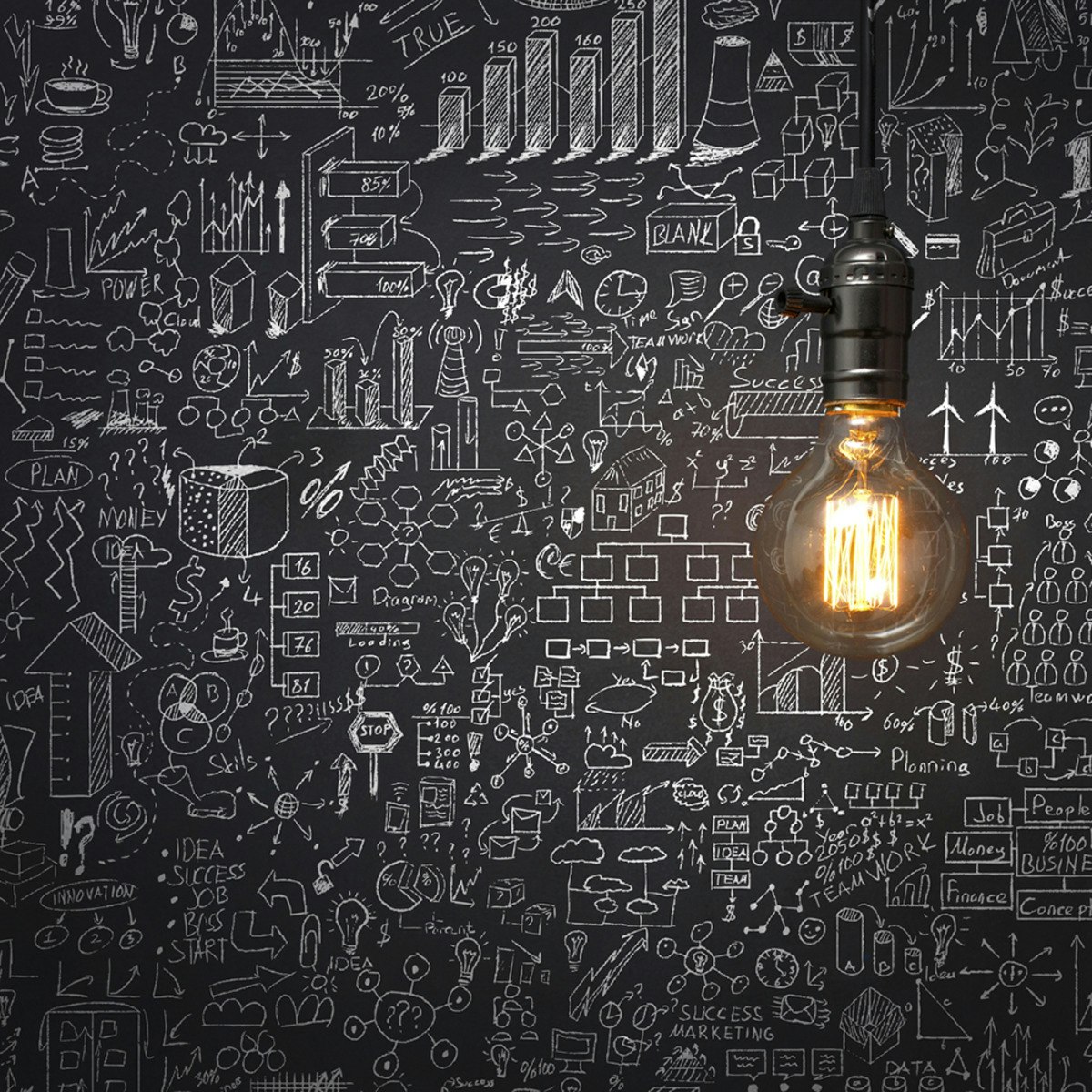
Hands-on Text Mining and Analytics
This course provides an unique opportunity for you to learn key components of text mining and analytics aided by the real world datasets and the text mining toolkit written in Java. Hands-on experience in core text mining techniques including text preprocessing, sentiment analysis, and topic modeling help learners be trained to be a competent data scientists.
Empowered by bringing lecture notes together with lab sessions based on the y-TextMiner toolkit developed for the class, learners will be able to develop interesting text mining applications.
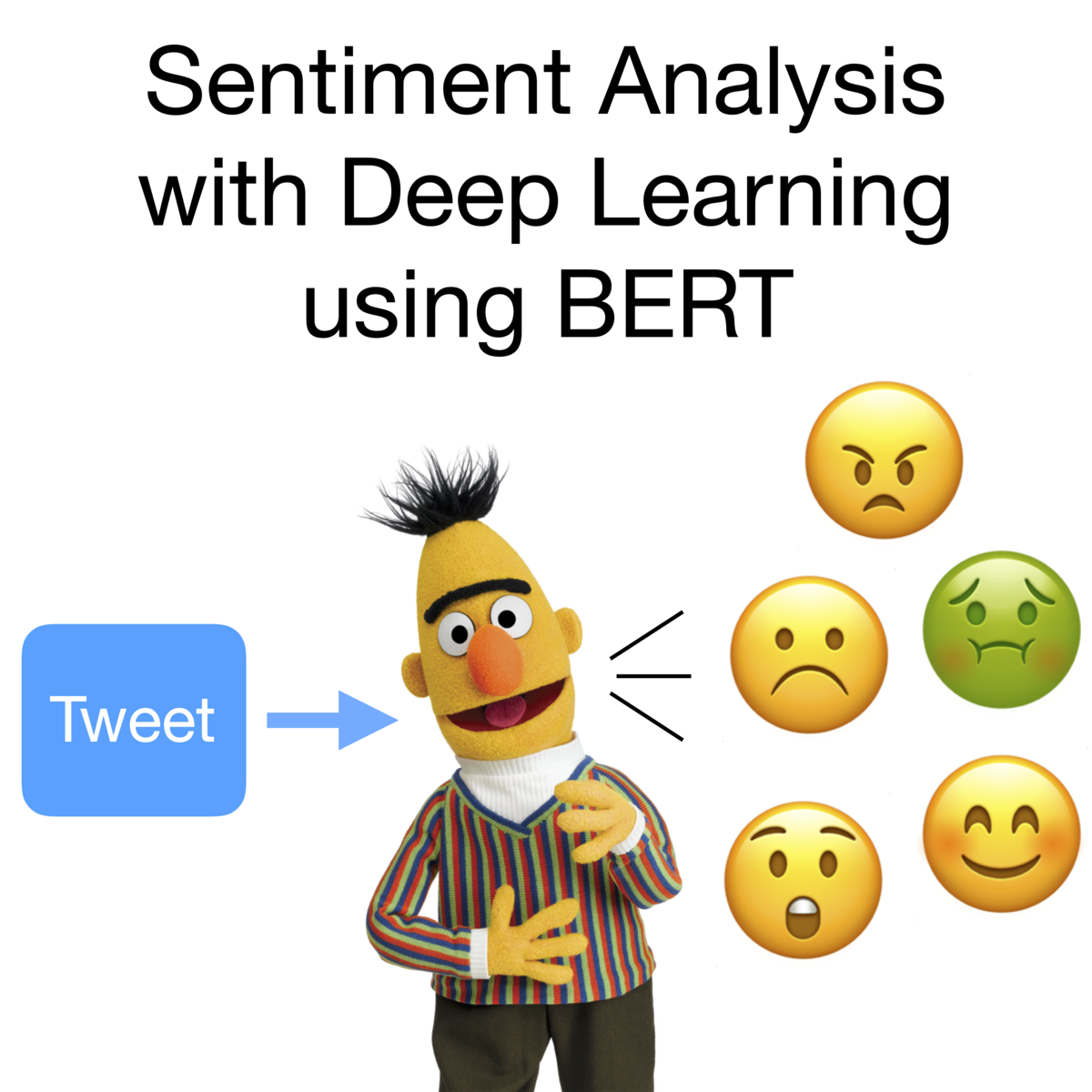
Sentiment Analysis with Deep Learning using BERT
In this 2-hour long project, you will learn how to analyze a dataset for sentiment analysis. You will learn how to read in a PyTorch BERT model, and adjust the architecture for multi-class classification. You will learn how to adjust an optimizer and scheduler for ideal training and performance. In fine-tuning this model, you will learn how to design a train and evaluate loop to monitor model performance as it trains, including saving and loading models. Finally, you will build a Sentiment Analysis model that leverages BERT's large-scale language knowledge.
Note: This course works best for learners who are based in the North America region. We’re currently working on providing the same experience in other regions.
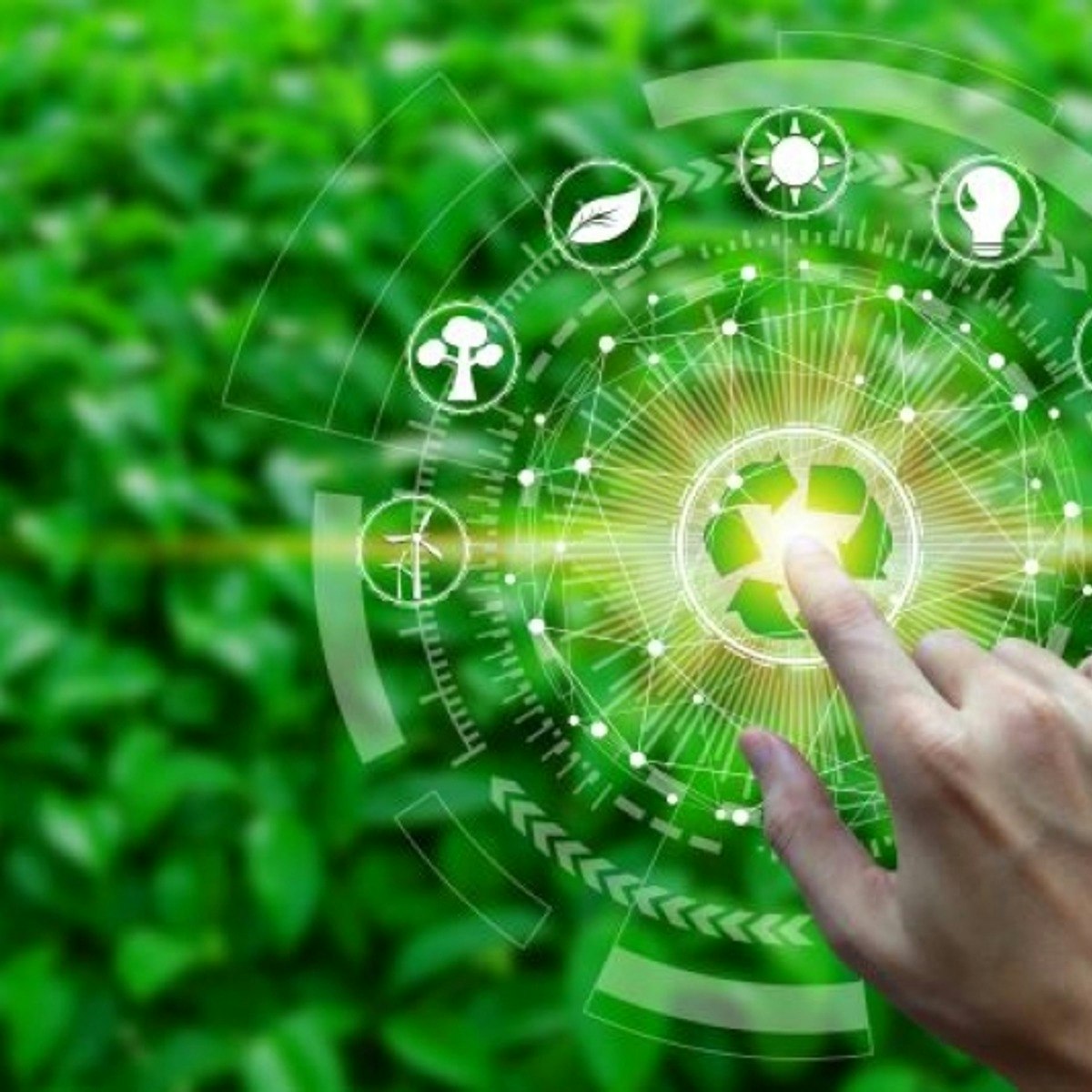
Doing Economics: Measuring Climate Change
This course will give you practical experience in working with real-world data, with applications to important policy issues in today’s society. Each week, you will learn specific data handling skills in Excel and use these techniques to analyse climate change data, with appropriate readings to provide background information on the data you are working with. You will also learn about the consequences of climate change and how governments can address this issue.
After completing this course, you should be able to:
• Understand how data can be used to assess the extent of climate change
• Produce appropriate bar charts, line charts, and scatterplots to visualise data
• Calculate and interpret summary statistics (mean, median, variance, percentile, correlation)
• Explain the challenges with designing and implementing policies that address climate change
No prior knowledge in economics or statistics is required for this course. No knowledge of Excel is required, except a familiarity with the interface and how to enter and clear data.
