Back to Courses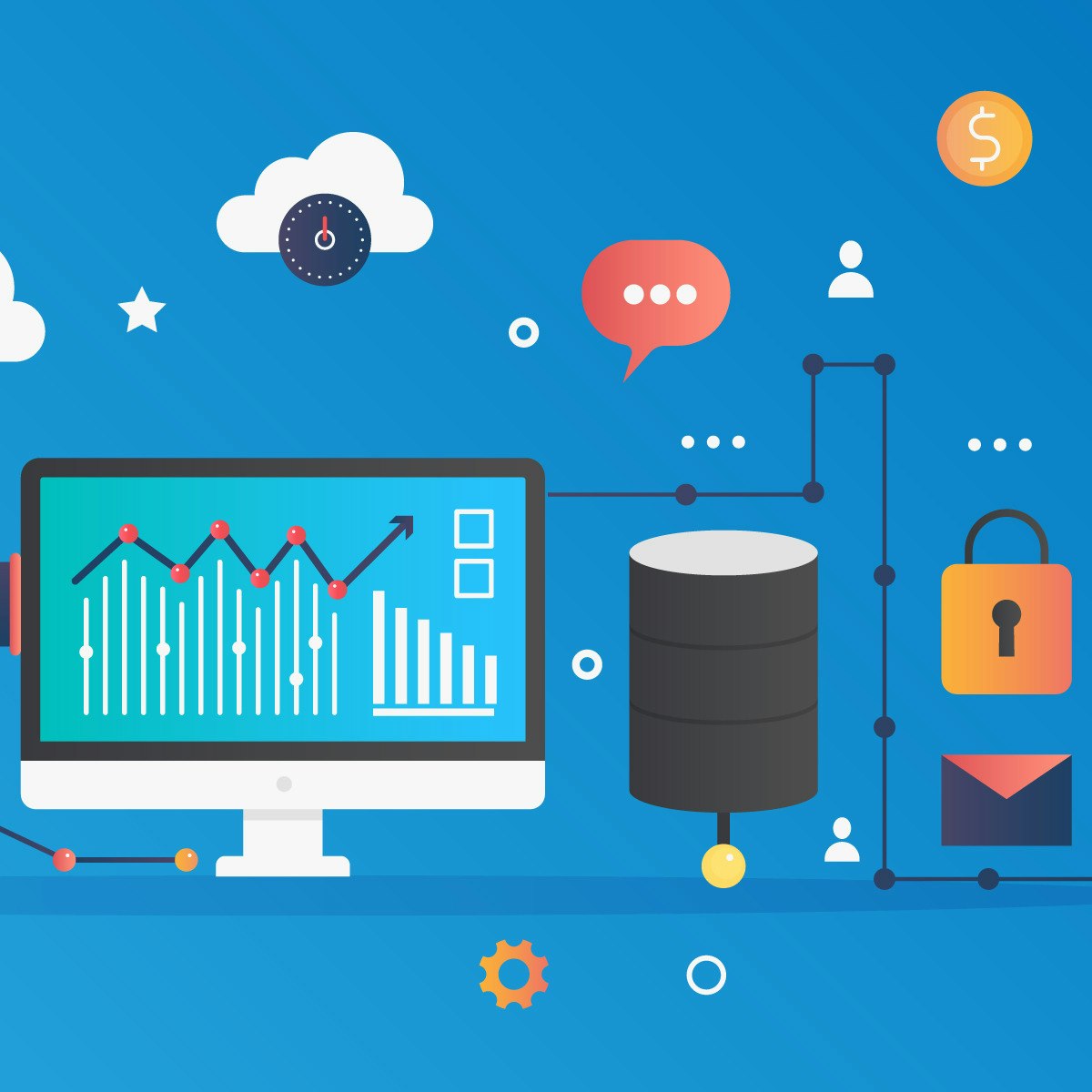
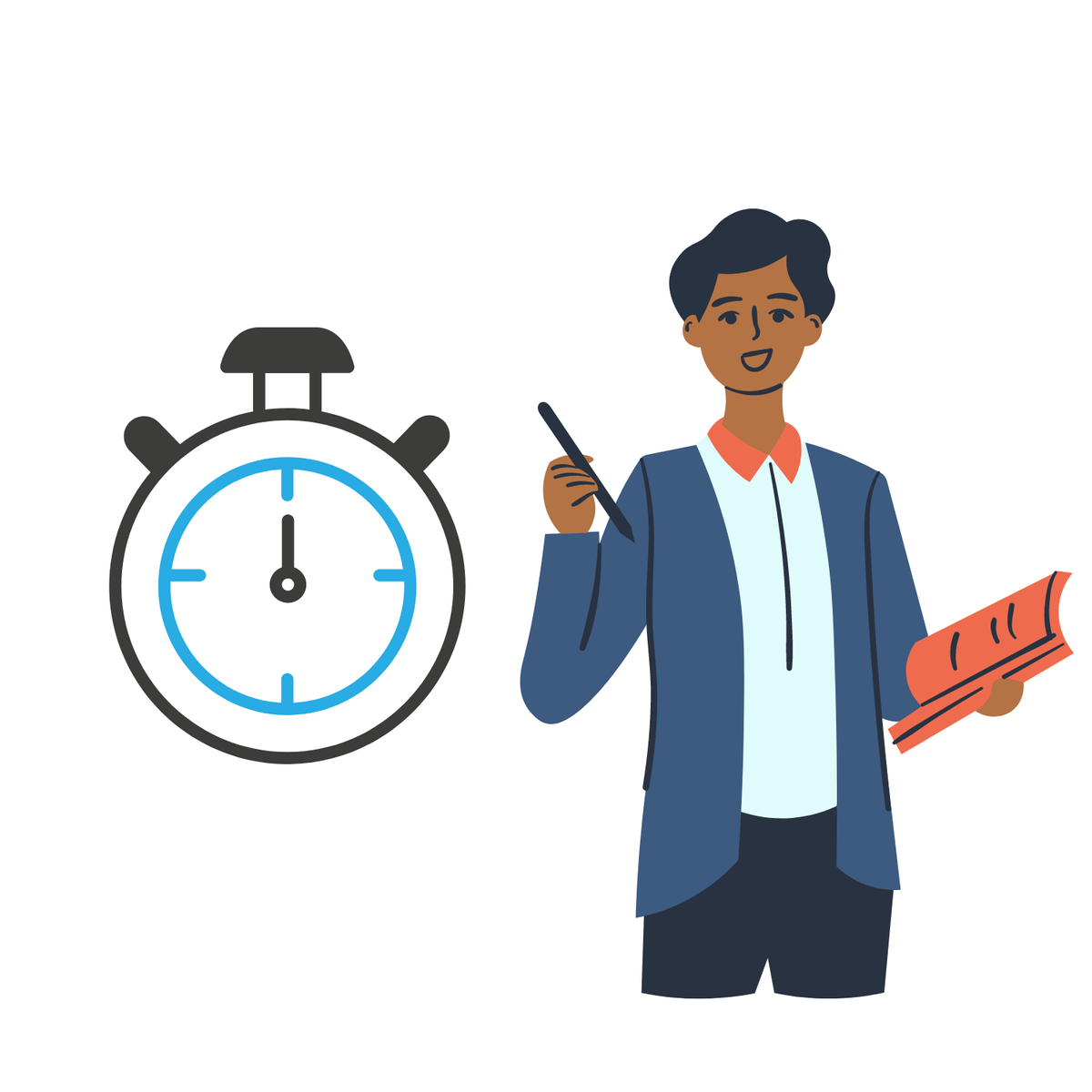
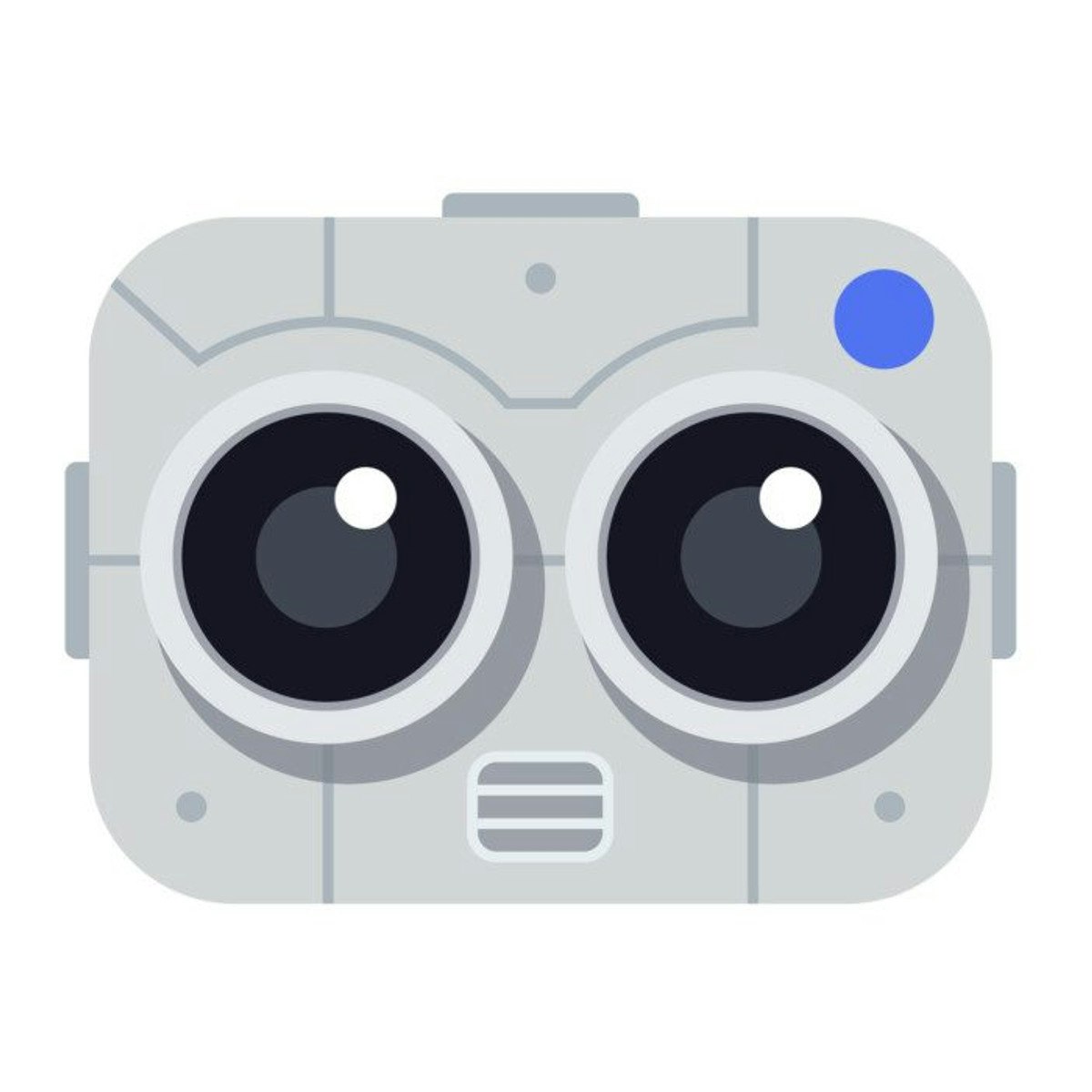
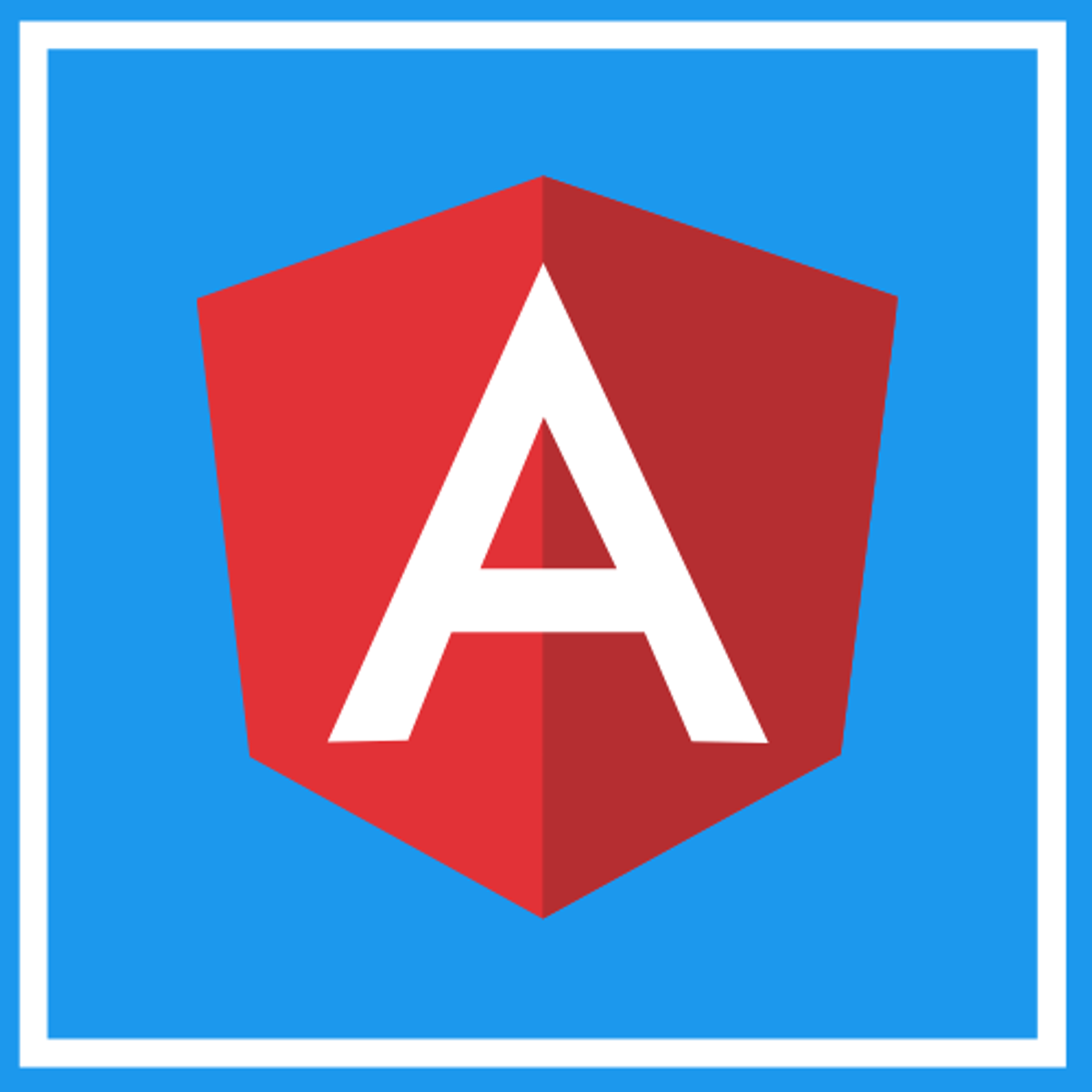
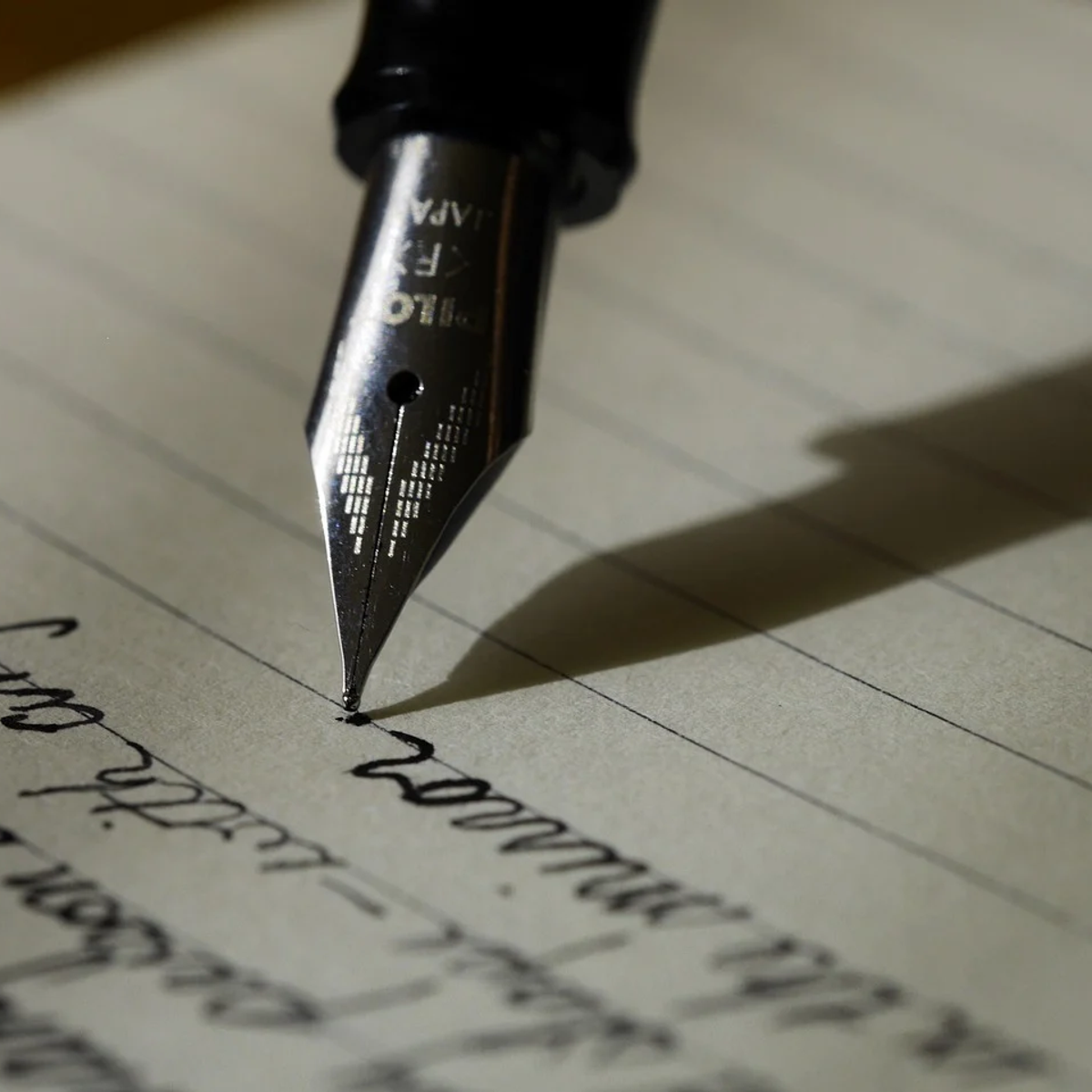
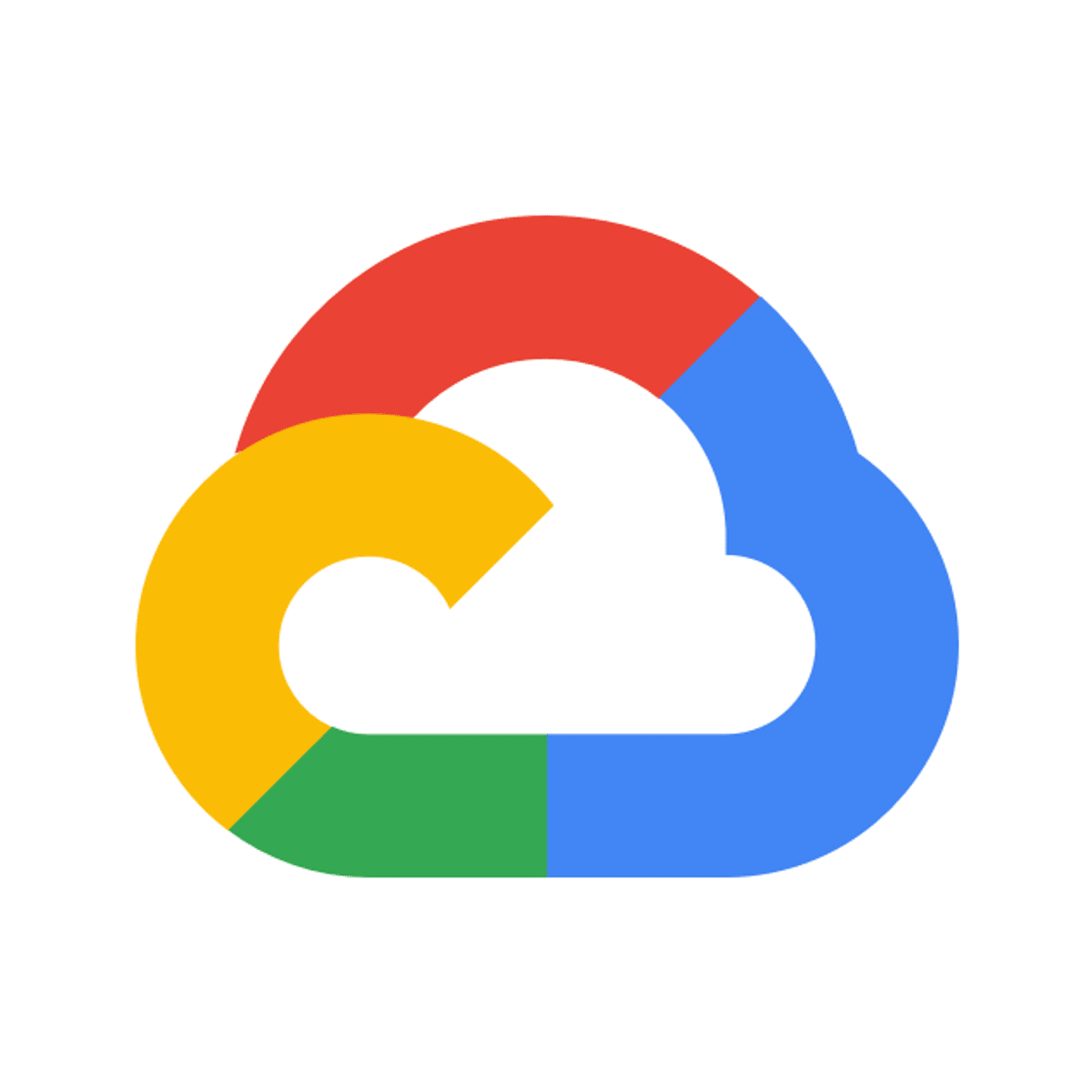
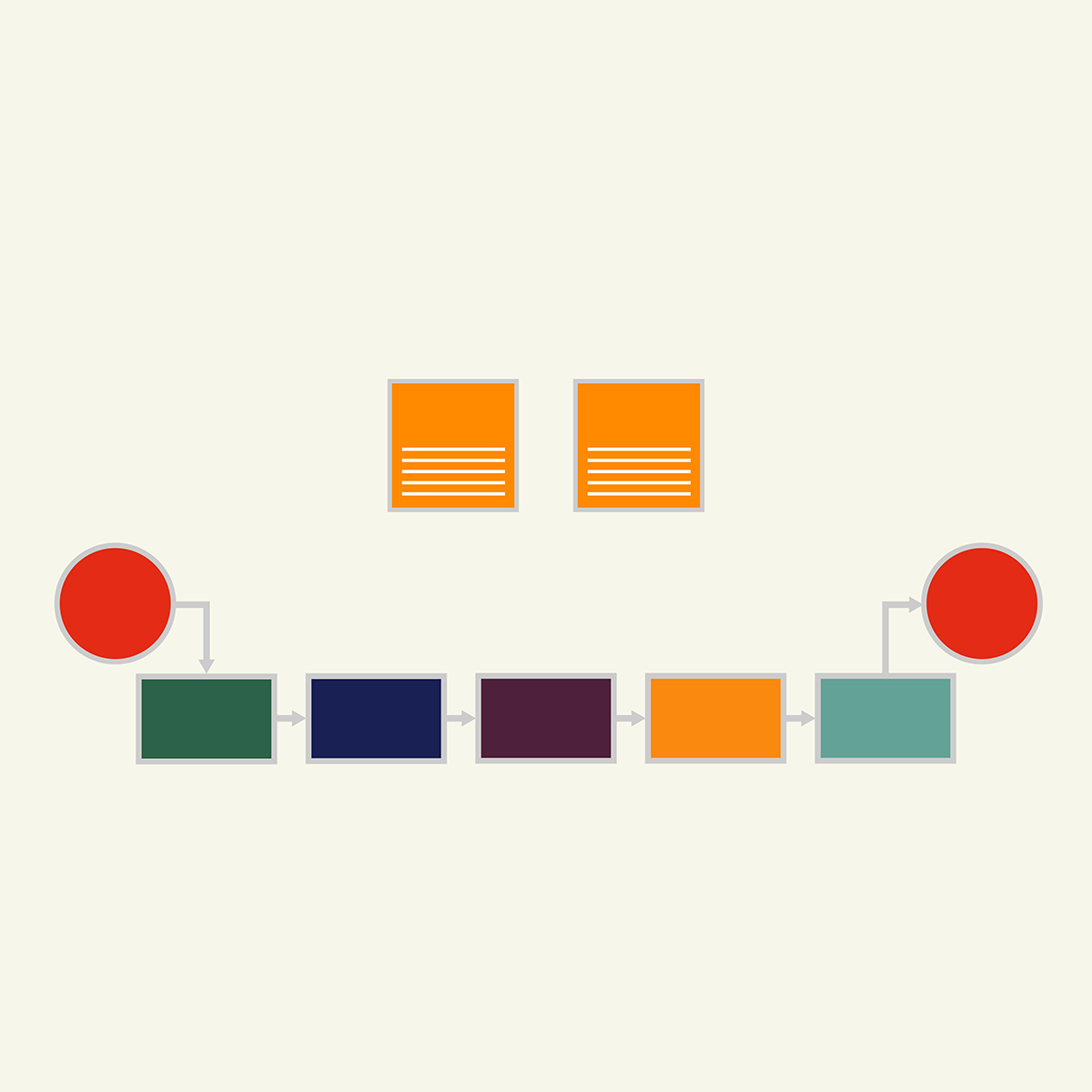
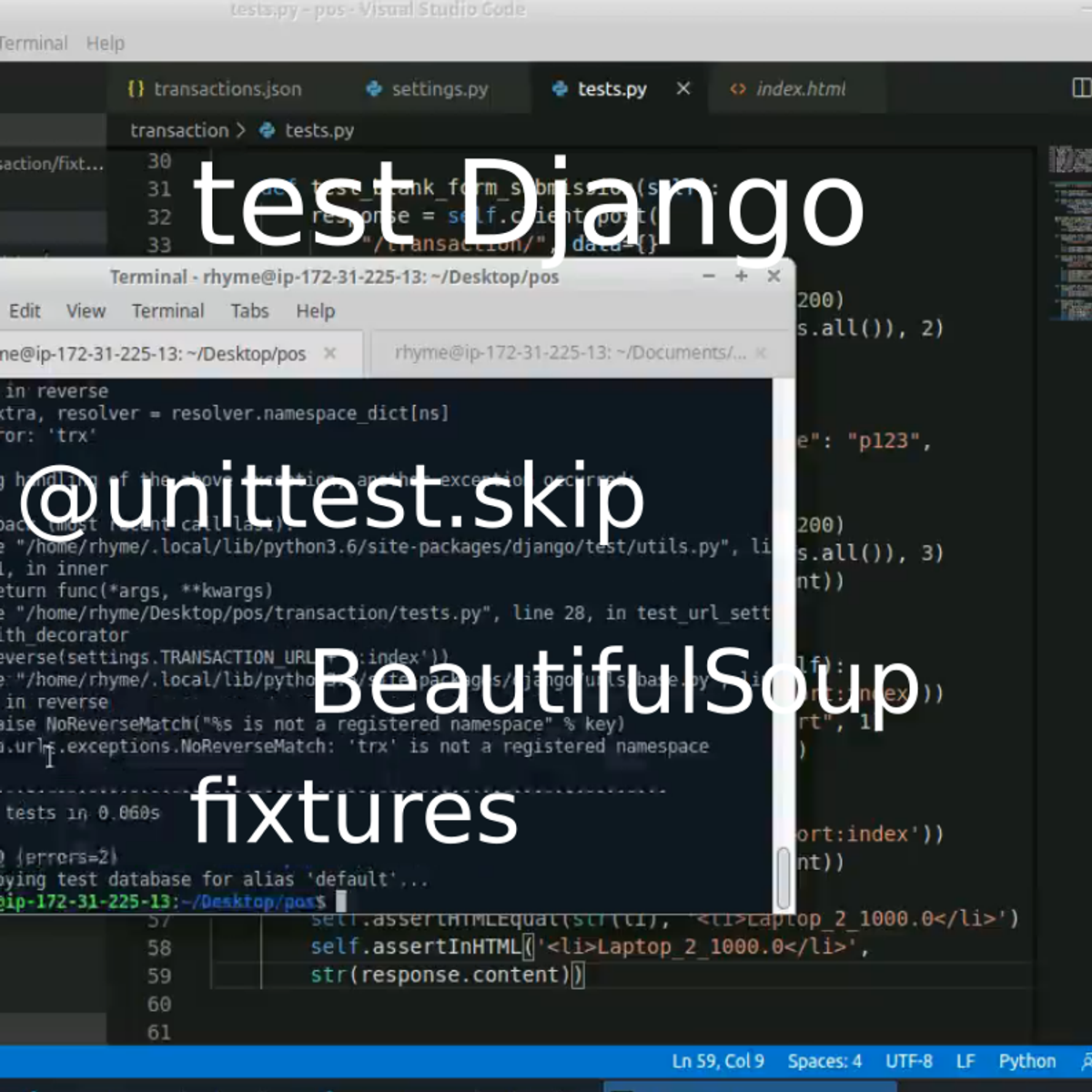
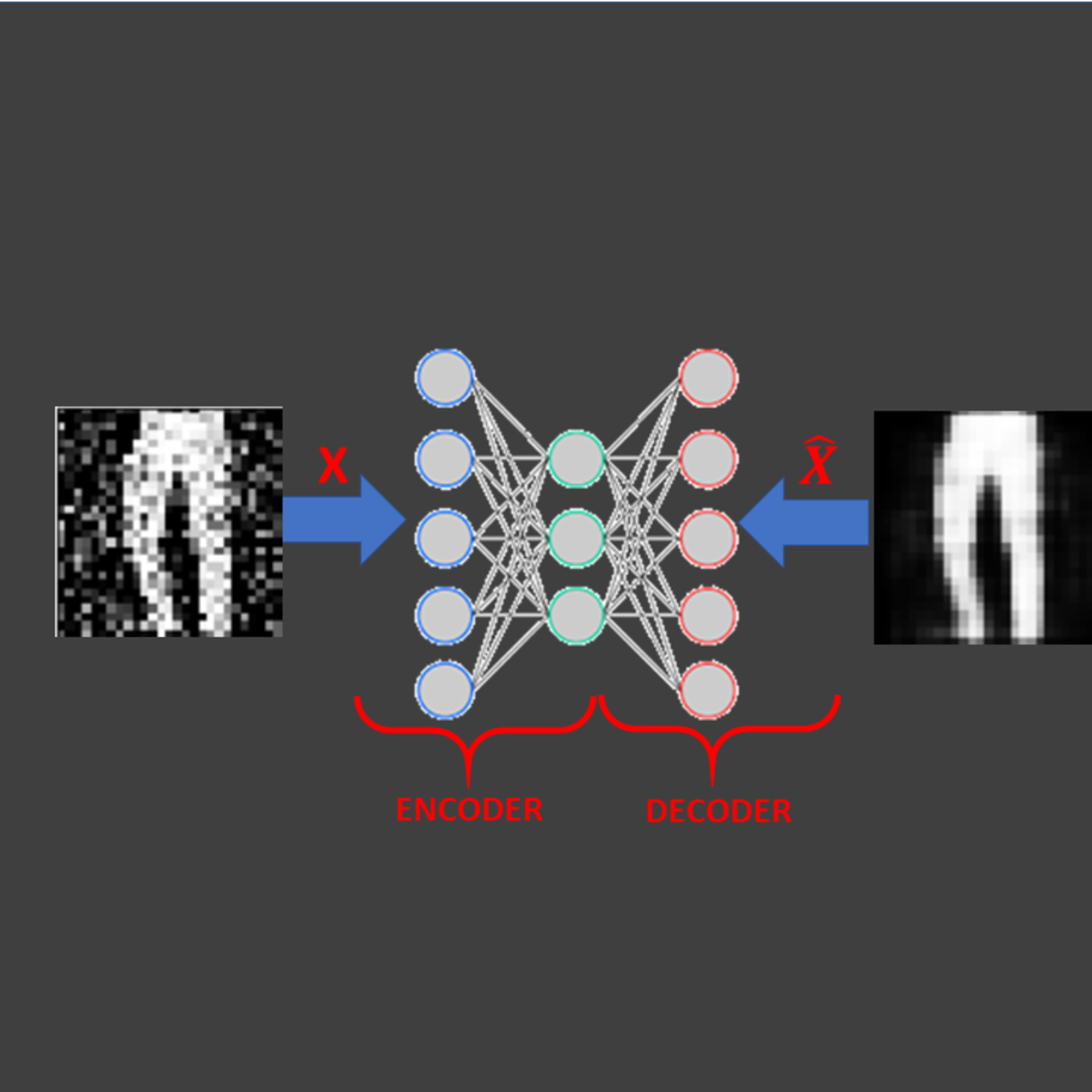
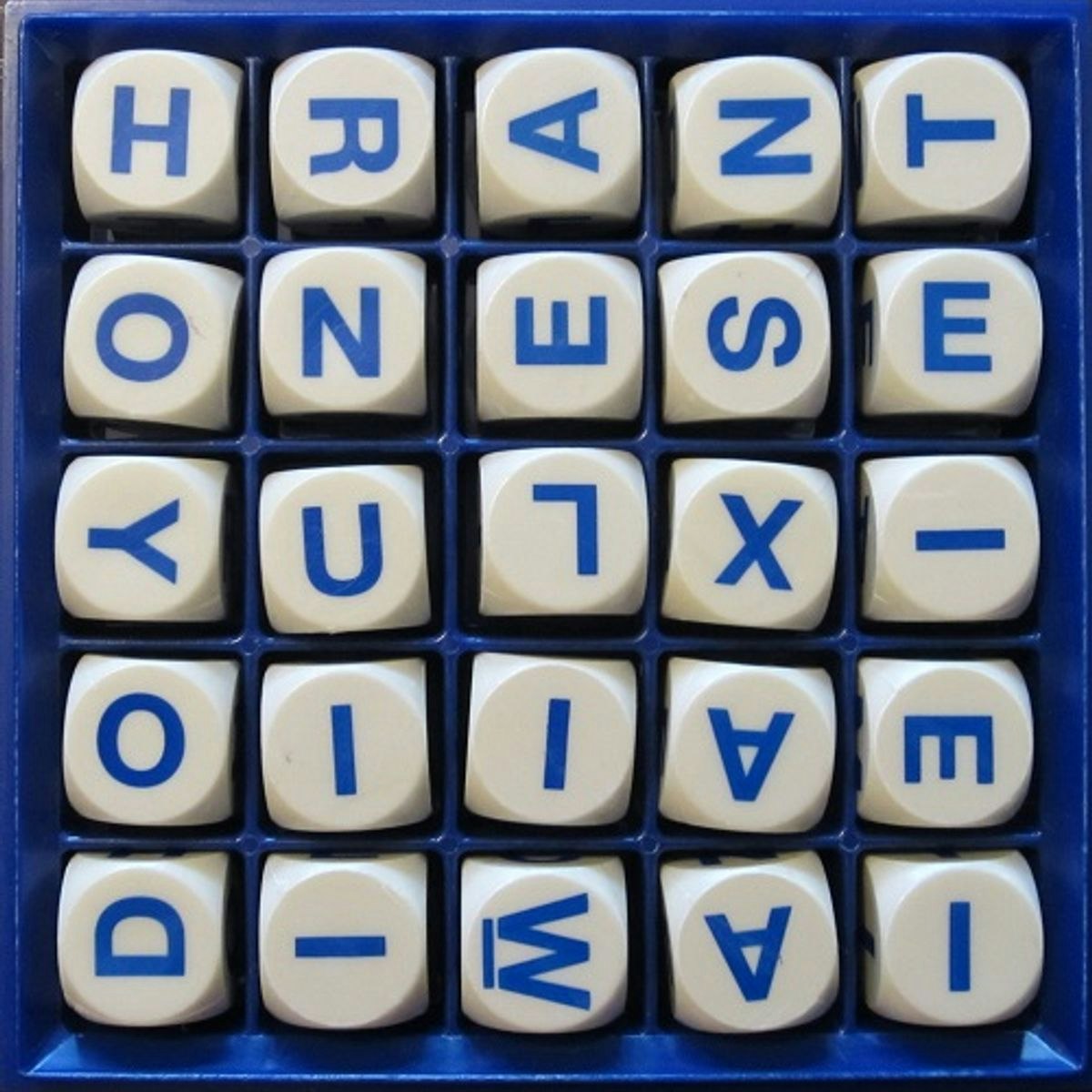
Software Development Courses - Page 2
Showing results 11-20 of 1266
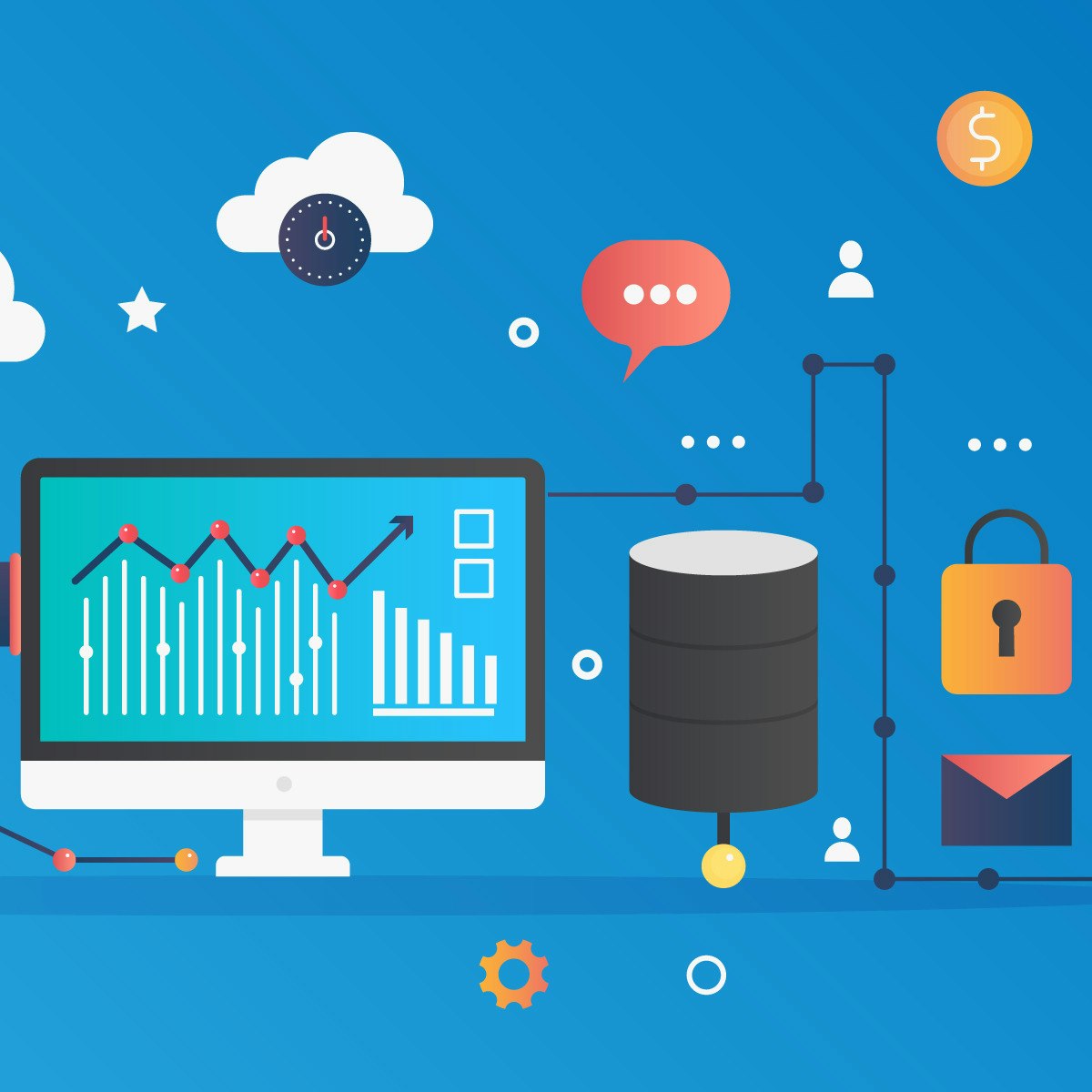
Relational Database Administration (DBA)
Ongoing and proactive management is critical to the security and performance of database management systems.
Database administration is the function of managing the operational aspects of database systems and maintaining them. Database administrators work to ensure that applications make the most efficient use of databases and that physical resources are used adequately and efficiently.
In this course, you will discover some of the activities, techniques, and best practices for managing a database. You will learn about configuring and upgrading database server software and related products. You will also learn about database security; how to implement user authentication, assign roles, and assign object-level permissions. You will also gain an understanding of how to perform backup and restore procedures in case of system failures.
You will learn about how to optimize databases for performance, monitor databases, collect diagnostic data, and access error information to help you resolve issues that may occur. Many of these tasks are repetitive, so you will learn how to schedule maintenance activities and regular diagnostic tests and send automated messages of the success or failure of a task.
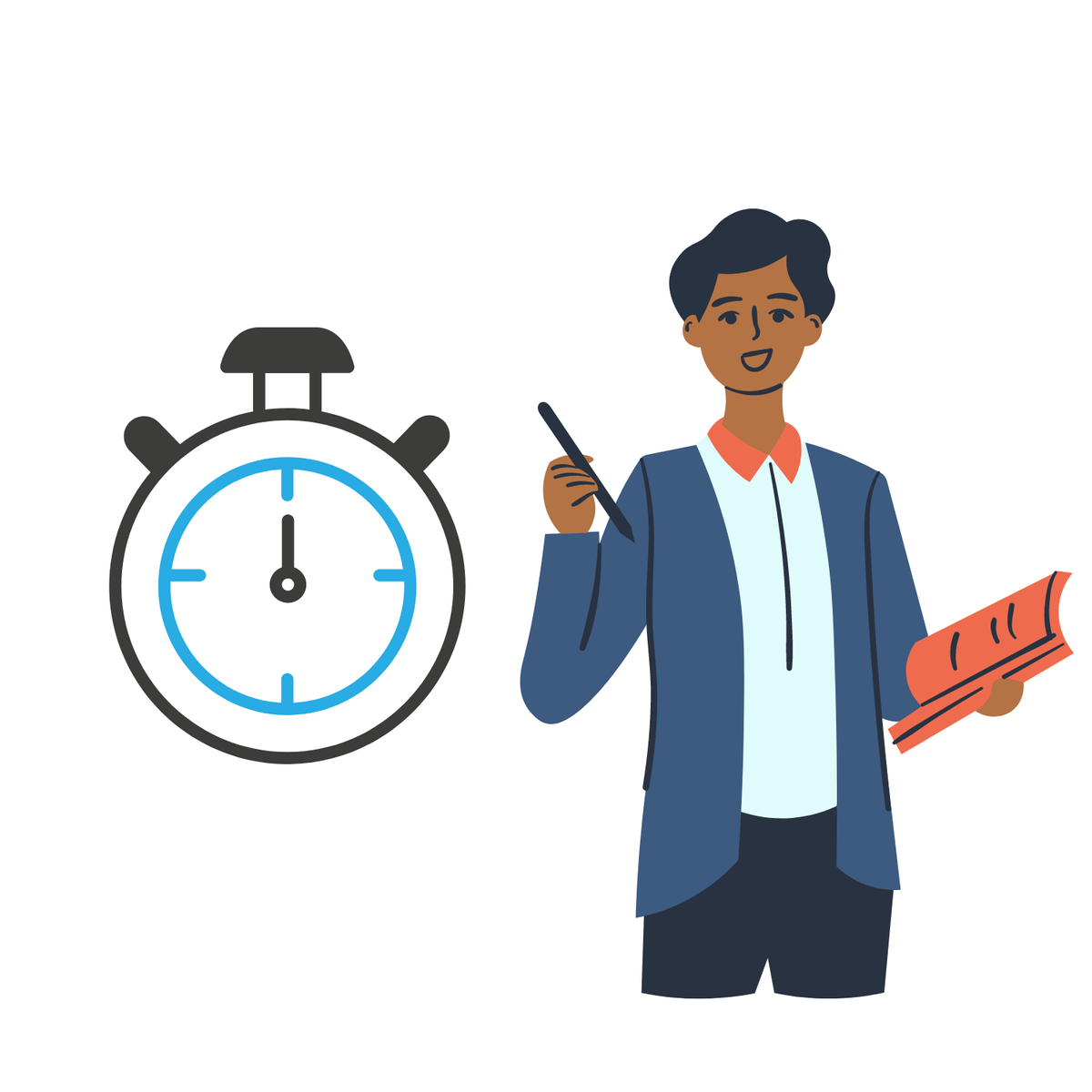
Waits in Selenium Test Automation Tool
One of the biggest challenges QAs and Developers face in test automation is synchronizing application under test and test automation code.
Selenium provides multiple wait methods (like Implicit and Explicit waits) to synchronize the application under test and test automation code.
In this two hours guided project, through hands-on, practical experience, you will go through concepts using Page load timeout, usage of implicit, explicit and Fluent waits.
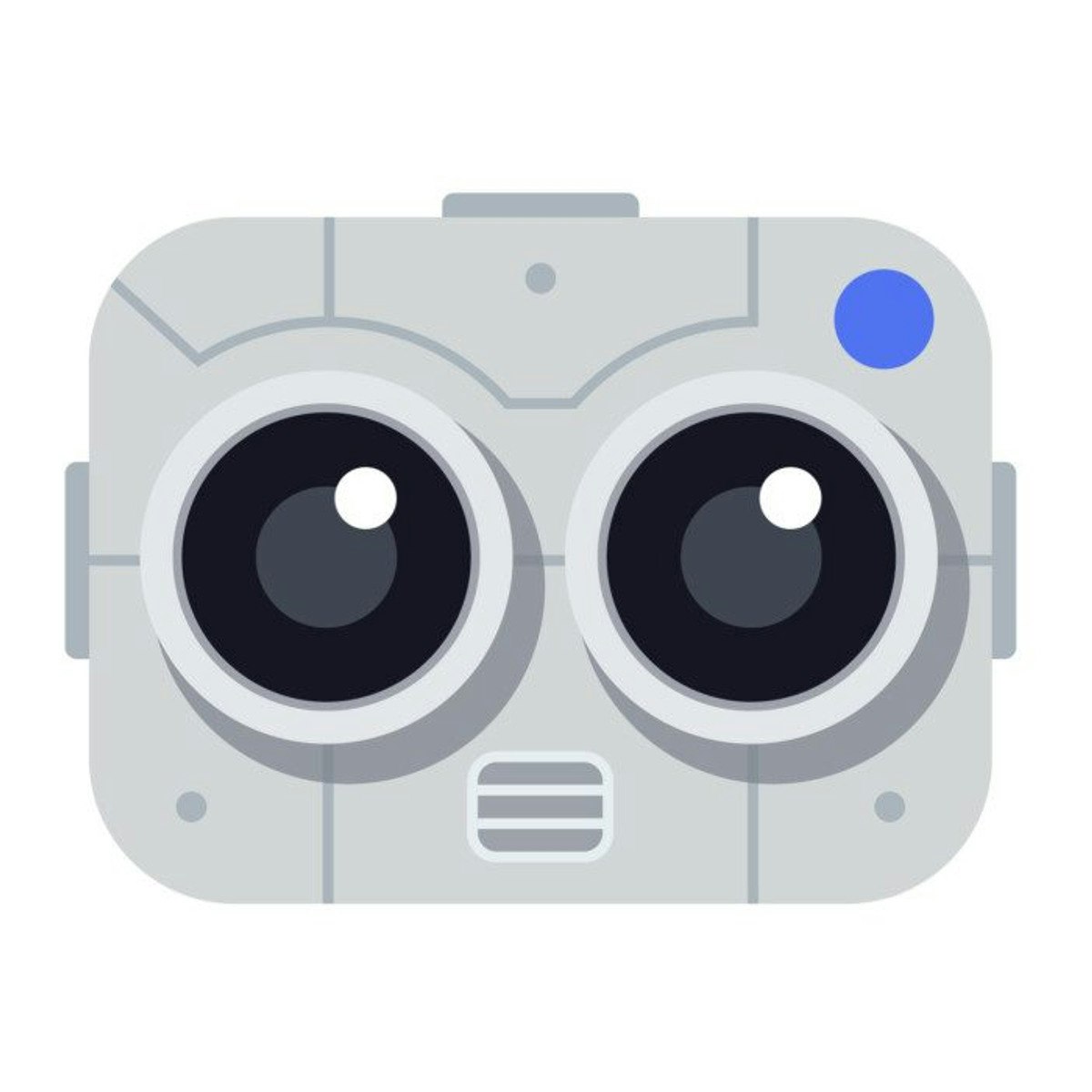
Object Detection Using Facebook's Detectron2
In this 2-hour long project-based course, you will learn how to train an Object Detection Model using Facebook's Detectron2. Detectron2 is a research platform and a production library for deep learning, built by Facebook AI Research (FAIR). We will be building an Object Detection Language Identification Model to identify English and Hindi texts written which can be extended to different use cases. We will look at the entire cycle of Model Development and Evaluation in Detectron2. We will first look at how to load a dataset, visualize it and prepare it as an input to the Deep Learning Model.
We will then look at how we can build a Faster R-CNN model in Detectron2 and customize it. We will then configure the parameters & hyperparameters of the model. We will then move on to training the Model and subsequently to model inference and evaluation.
Note: This course works best for learners who are based in the North America region. We’re currently working on providing the same experience in other regions.
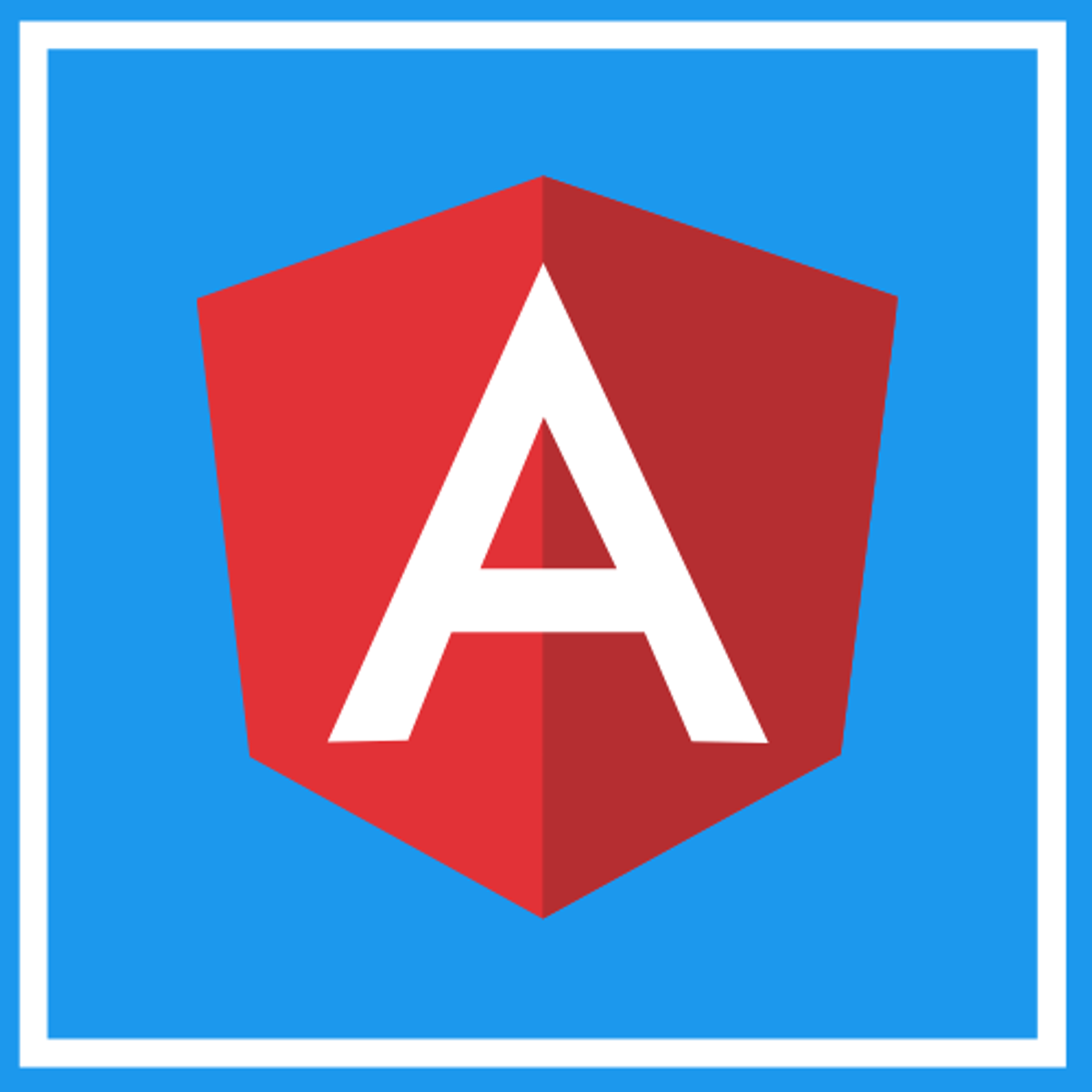
Build ATM User Interface using Routing in Angular
In this beginner level project, you will implement and build ATM user interface using routing, understand Parent and Child Routing and understand WildCard Routes in Angular which will be helpful in applying routing and navigating pages in modern web layouts. The pre-requisite for this guided project is have background in HTML,CSS, JavaScript/TypeScript and basics on building blocks of Angular Applications.
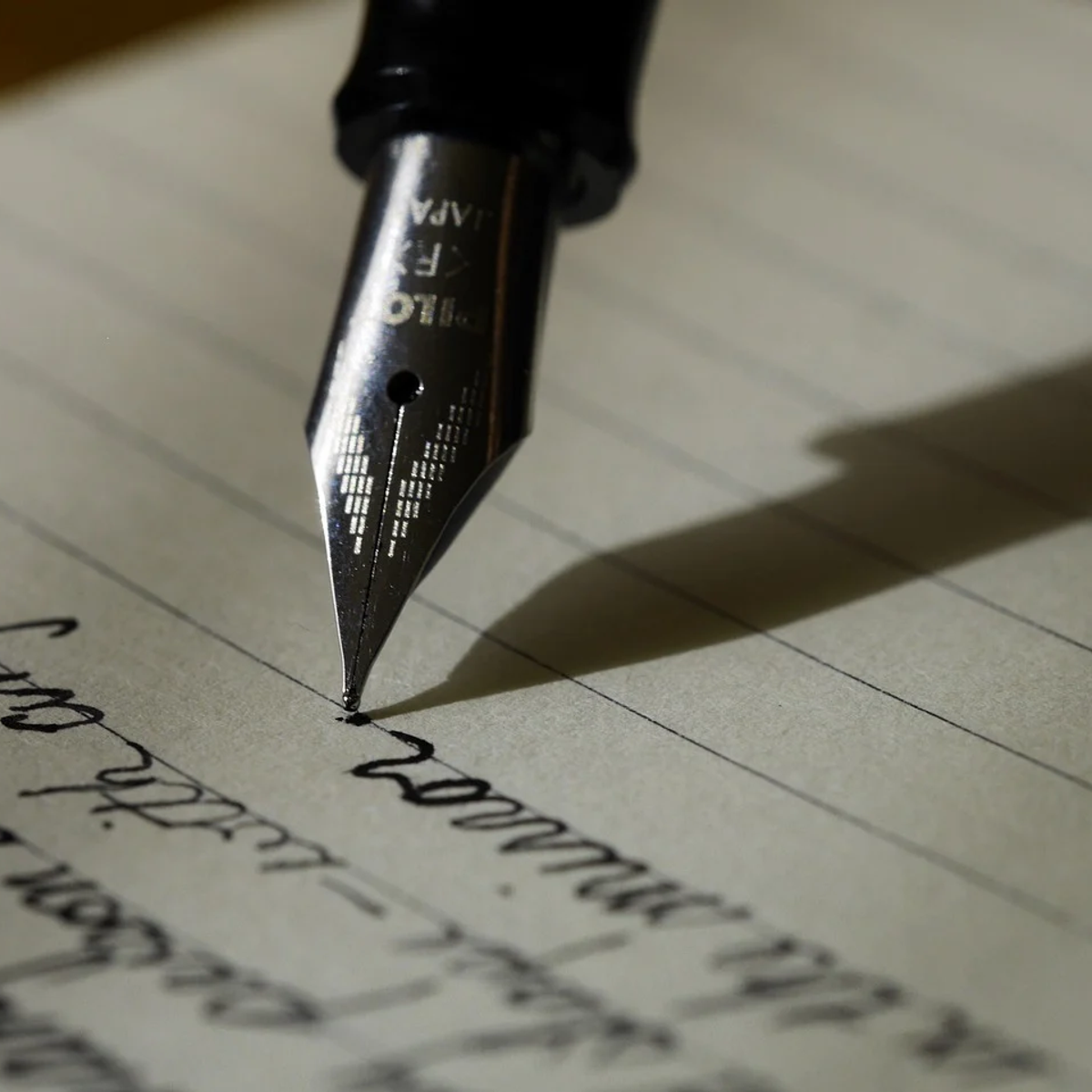
Introduction to Text Classification in R with quanteda
In this guided project you will learn how to import textual data stored in raw text files into R, turn these files into a corpus (a collection of textual documents), reshape them into paragraphs from documents and tokenize the text all using the R software package quanteda. You will then learn how to classify the texts using the Naive Bayes algorithm.
This guided project is for beginners interested in quantitative text analysis in R. It assumes no knowledge of textual analysis and focuses on exploring textual data (US Presidential Concession Speeches). Users should have a basic understanding of the statistical programming language R.
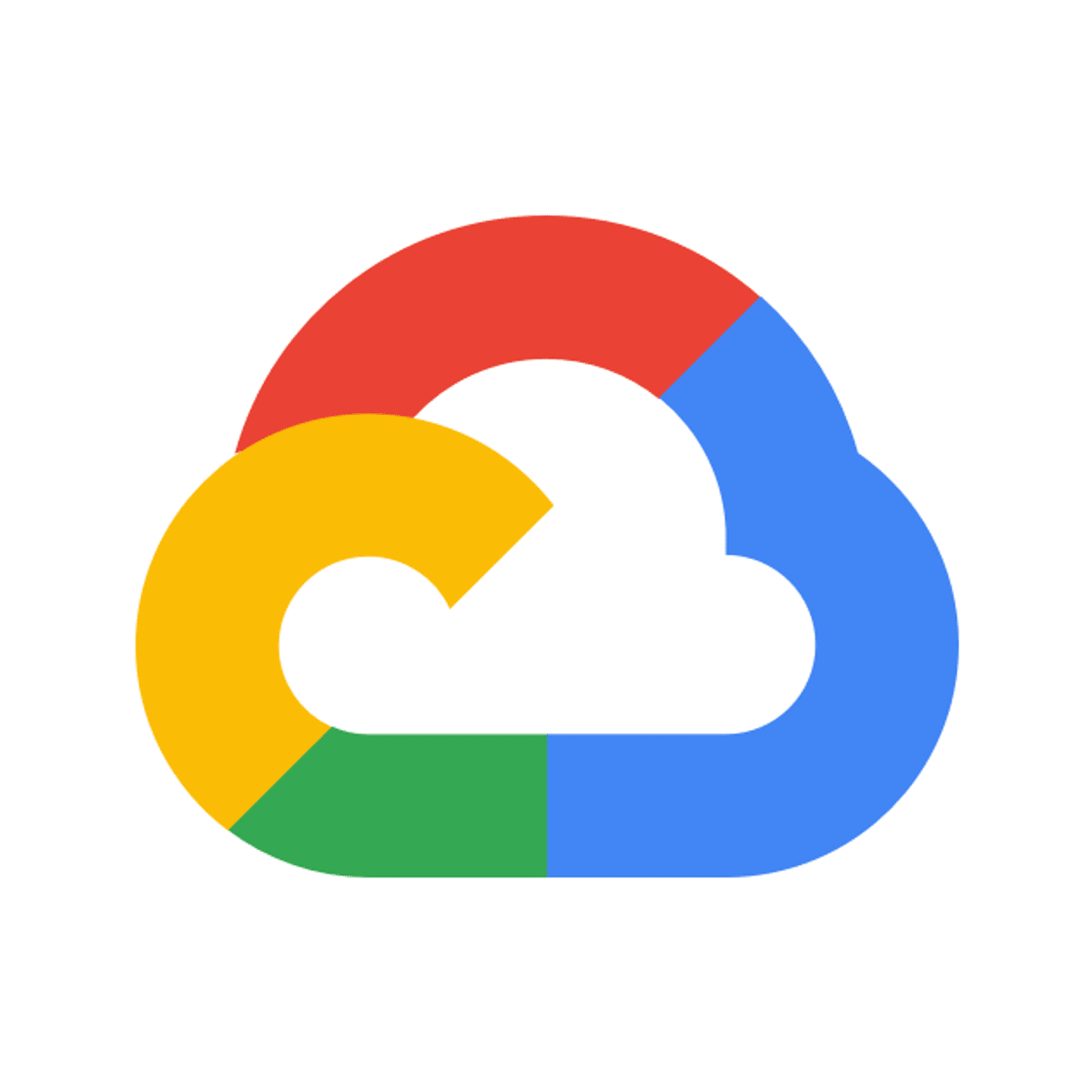
Build an End-to-End Data Capture Pipeline using Document AI
This is a self-paced lab that takes place in the Google Cloud console. In this lab you use Cloud Functions and Pub/Sub to create an end-to-end document processing pipeline using Document AI. The Document AI API is a document understanding solution that takes unstructured data, such as documents and emails, and makes the data easier to understand, analyze, and consume.
In this lab, you will create a document processing pipeline that will automatically process documents that are uploaded to Cloud Storage. The pipeline consists of a primary Cloud Function that processes new files that are uploaded to Cloud Storage using a Document AI form processor and then saves form data detected in those files to BigQuery. If the form data includes any address fields the address data is then written to a Pub/Sub topic that in turn triggers a second Cloud Function that uses to Geocoding API to provide geographic coordinate data for the address that is also written to BigQuery.
This is a simple pipeline that uses a general form processor that will detect basic form data, such as a labelled field containing address information. Document AI processors that use one of the specialized parsers that are beyond the scope of this lab provide enhanced entity information for specific document types even when those documents do not include labelled fields. For example, a Document AI Invoice parser can provide detailed address and supplier information, from an unlabelled invoice document because it understands the layout of invoices.
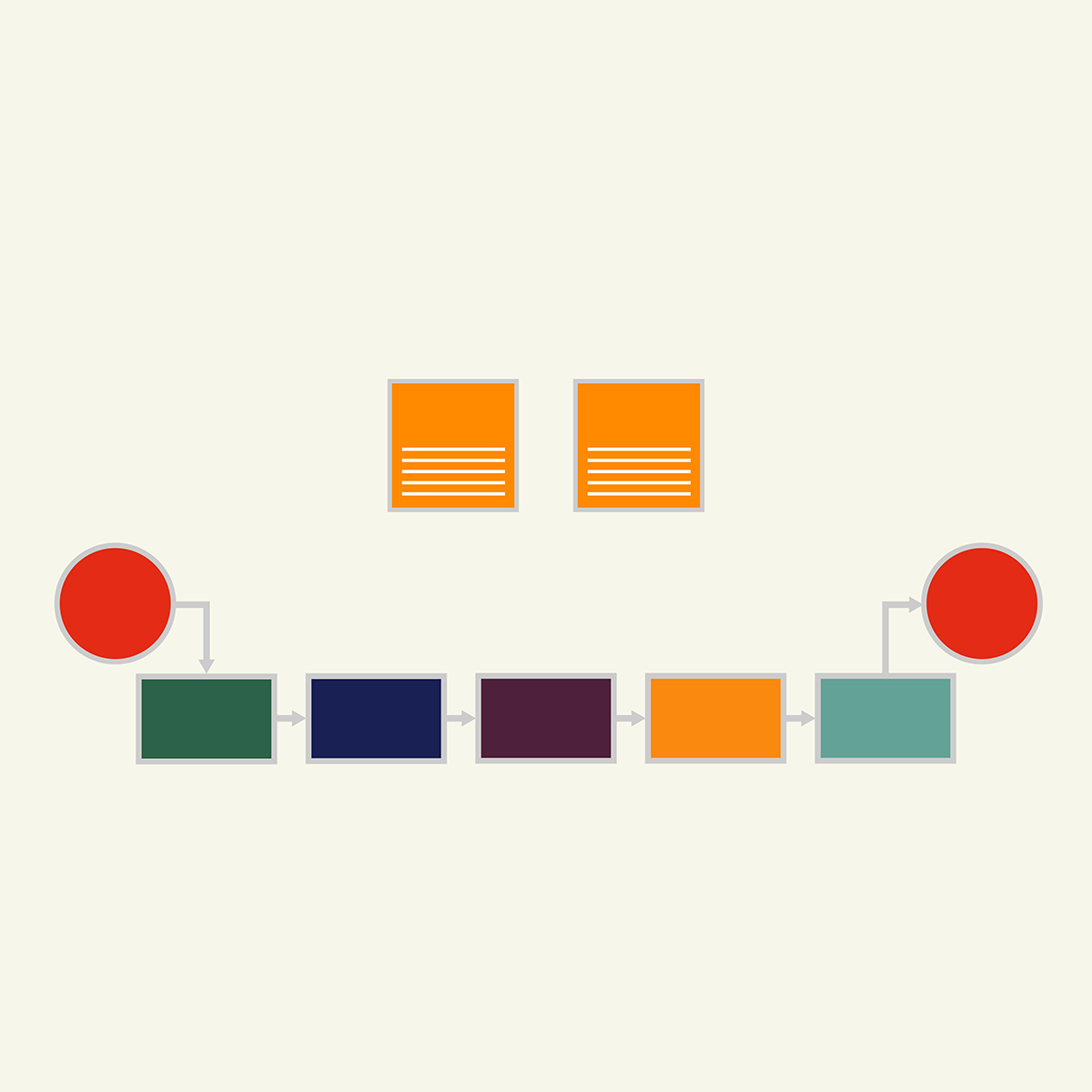
Continuous Delivery & DevOps
Amazon famously delivers new code every 11.6 seconds. Just a few years ago, this was unthinkable: many ‘cutting edge’ firms would release software quarterly. When it comes to digital innovation, velocity is critical and many would say it’s the most reliable determinant of success.
Bringing an organization to the state of the art (or even functional capability) in this area requires strong work in a combination of disciplines and a combination of both technical and managerial skills. There is no single cookie-cutter approach for achieving this capability. Much like agile, the right focus and formulation depends a lot on the facts and circumstances of the team. This course, developed at the Darden School of Business at the University of Virginia and taught by top-ranked faculty, will provide you with the interdisciplinary skill set to cultivate a continuous deployment capability in your organization.
After completing this course, you will be able to:
1. Diagnose a team’s delivery pipeline and bring forward prioritized recommendations to improve it
2. Explain the skill sets and roles involved in DevOps and how they contribute toward a continuous delivery capability
3. Review and deliver automation tests across the development stack
4. Explain the key jobs of system operations and how today’s leading techniques and tools apply to them
5. Explain how high-functioning teams use DevOps and related methods to reach a continuous delivery capability
6. Facilitate prioritized, iterative team progress on improving a delivery pipeline
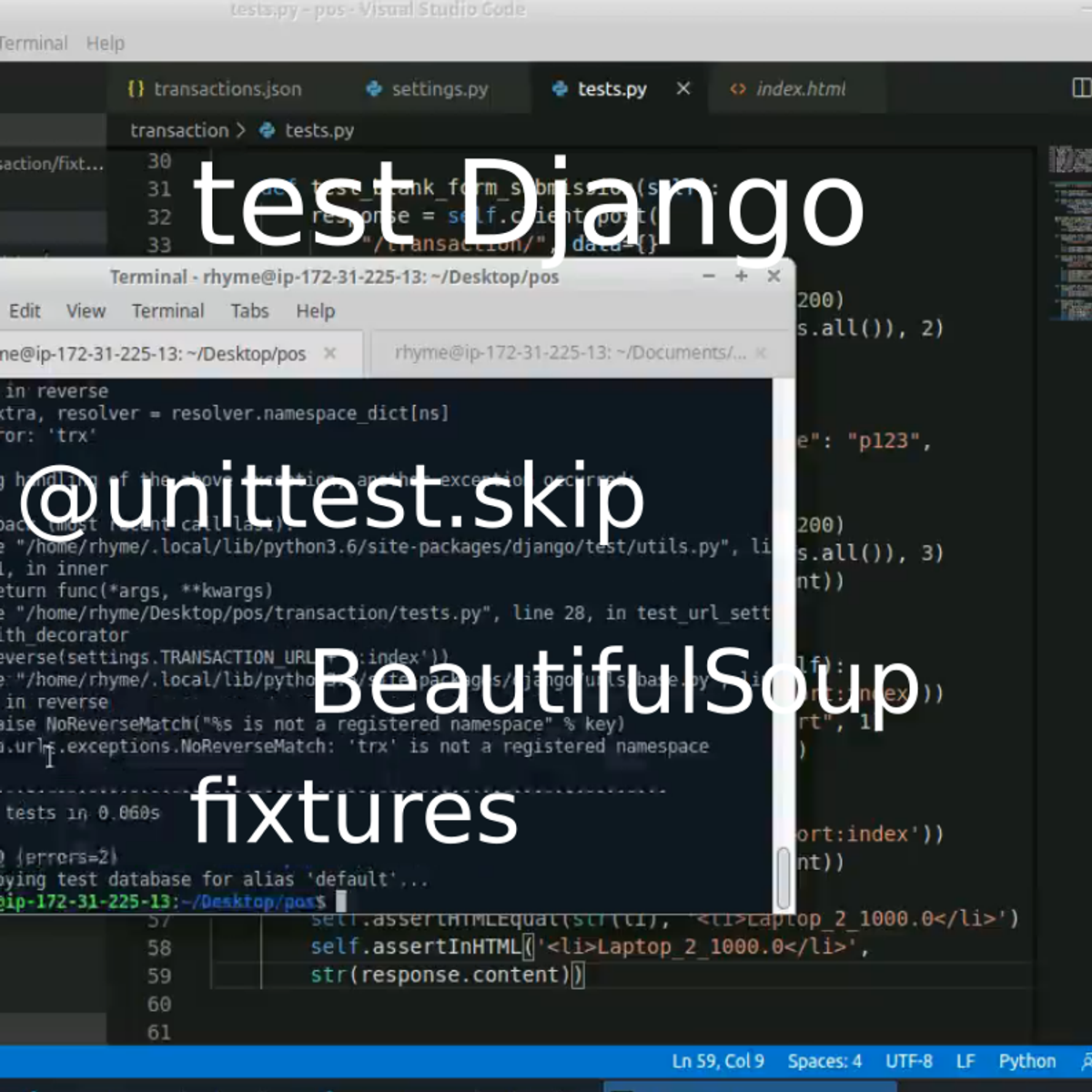
Apply advanced testing for your Django web application
In this 1-hour long project-based course, you will learn some of the advanced features of Django testing framework. For a given sample Django project, you will learn how to feed the testing database with data using fixtures. You will use different methods to customize the project-wide settings during a test. You will learn how to test form submission, and understand how to test the response object for strings. In particular, you will use the Beautiful Soup python library to test the response object for the presence of HTML tags. Finally, you will learn how to instruct Django to skip tests.
Note: This course works best for learners who are based in the North America region. We’re currently working on providing the same experience in other regions.
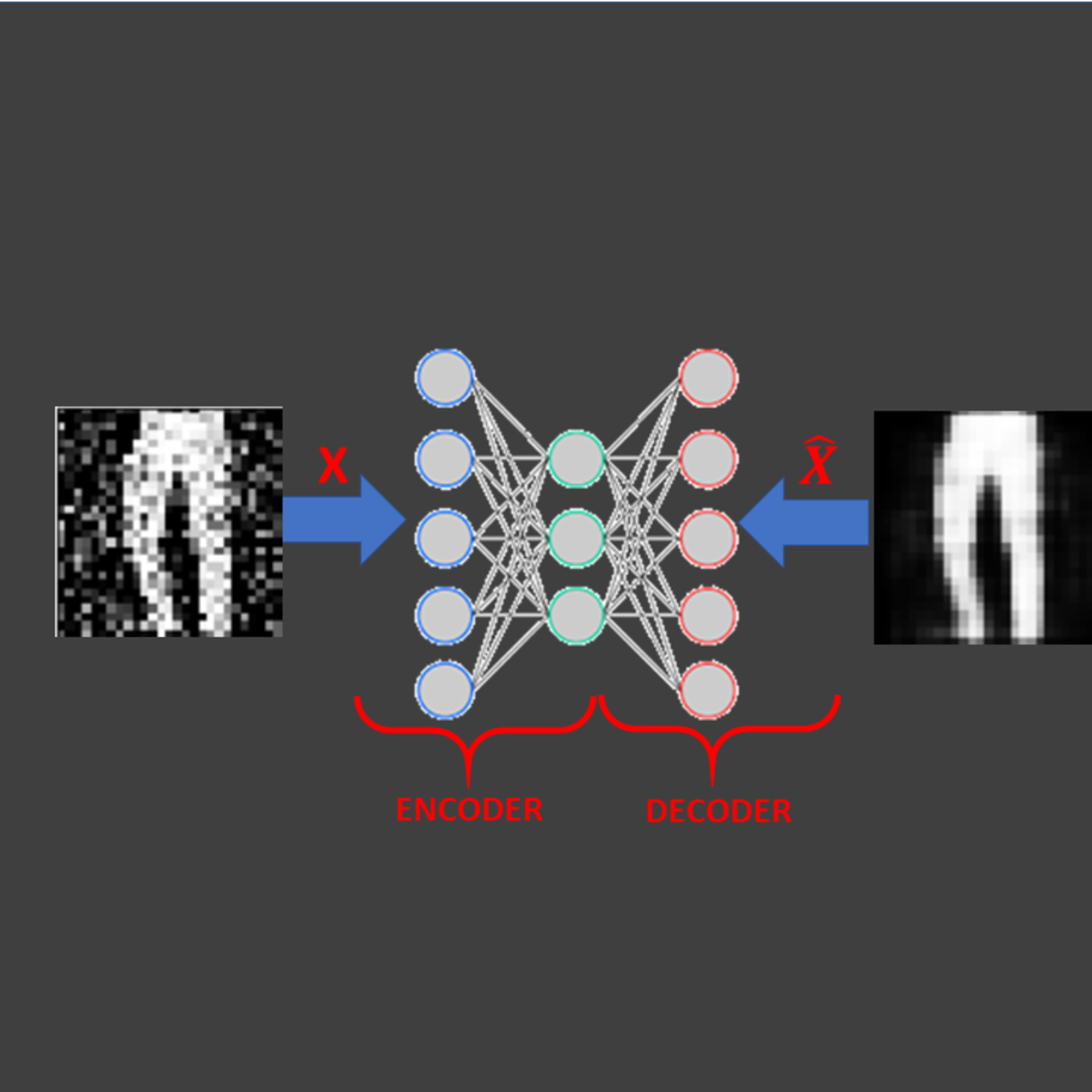
Image Denoising Using AutoEncoders in Keras and Python
In this 1-hour long project-based course, you will be able to:
- Understand the theory and intuition behind Autoencoders
- Import Key libraries, dataset and visualize images
- Perform image normalization, pre-processing, and add random noise to images
- Build an Autoencoder using Keras with Tensorflow 2.0 as a backend
- Compile and fit Autoencoder model to training data
- Assess the performance of trained Autoencoder using various KPIs
Note: This course works best for learners who are based in the North America region. We’re currently working on providing the same experience in other regions.
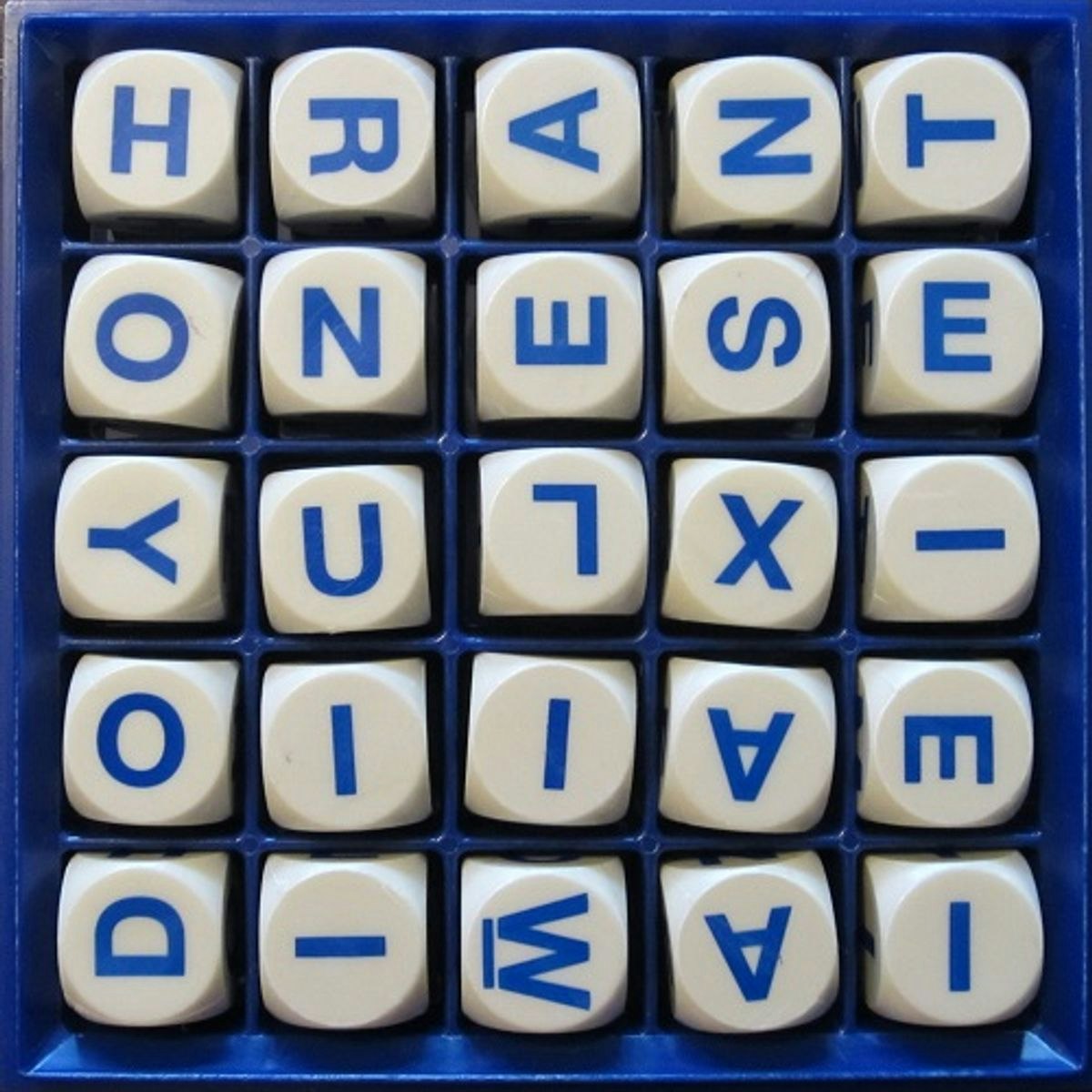
Create a Boggle Word Solver using recursion in Python
In this 1-hour long project-based course, you will have created a Boggle Word Solver in Python by defining various functions that load a 4x4 game board based on input, recursively searches in all allowed directions for plausible words using Depth First Traversal, and then prints out the valid words based on length constraints and by cross-checking whether the word exists in the stored dictionary. You will also learn to store the dictionary in a trie data structure which makes for more efficient lookups.
This guided project is aimed at learners who are wanting to learn or practice recursion and graph traversal concepts in Python by developing a fun game. Understanding DFS and recursion is essential and will greatly expand your programming potential as they are used at the base of various algorithms. Implementing a prefix tree will also help you understand a new efficient data structure.
Note: This course works best for learners who are based in the North America region. We’re currently working on providing the same experience in other regions.
Popular Internships and Jobs by Categories
Browse
© 2024 BoostGrad | All rights reserved