Back to Courses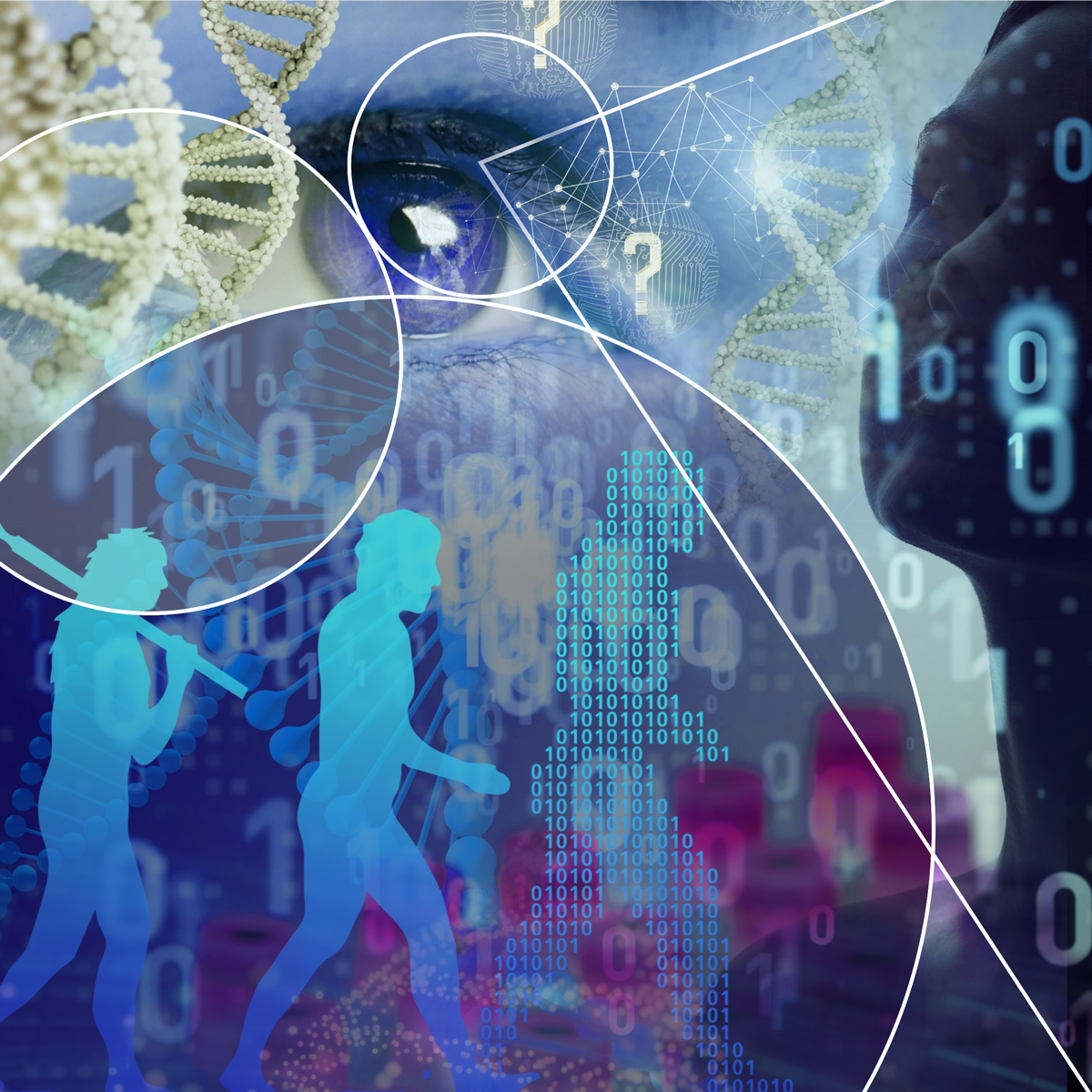
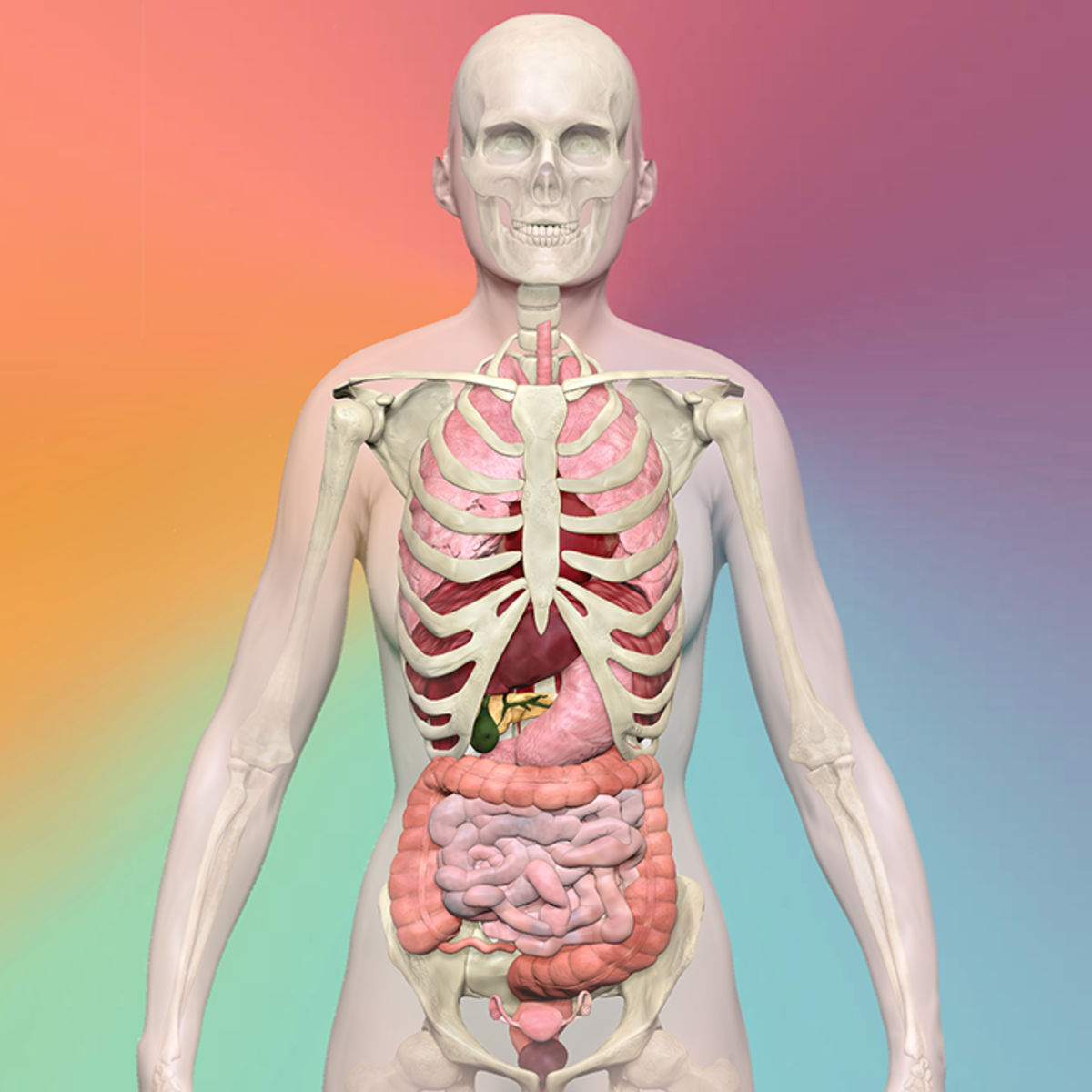
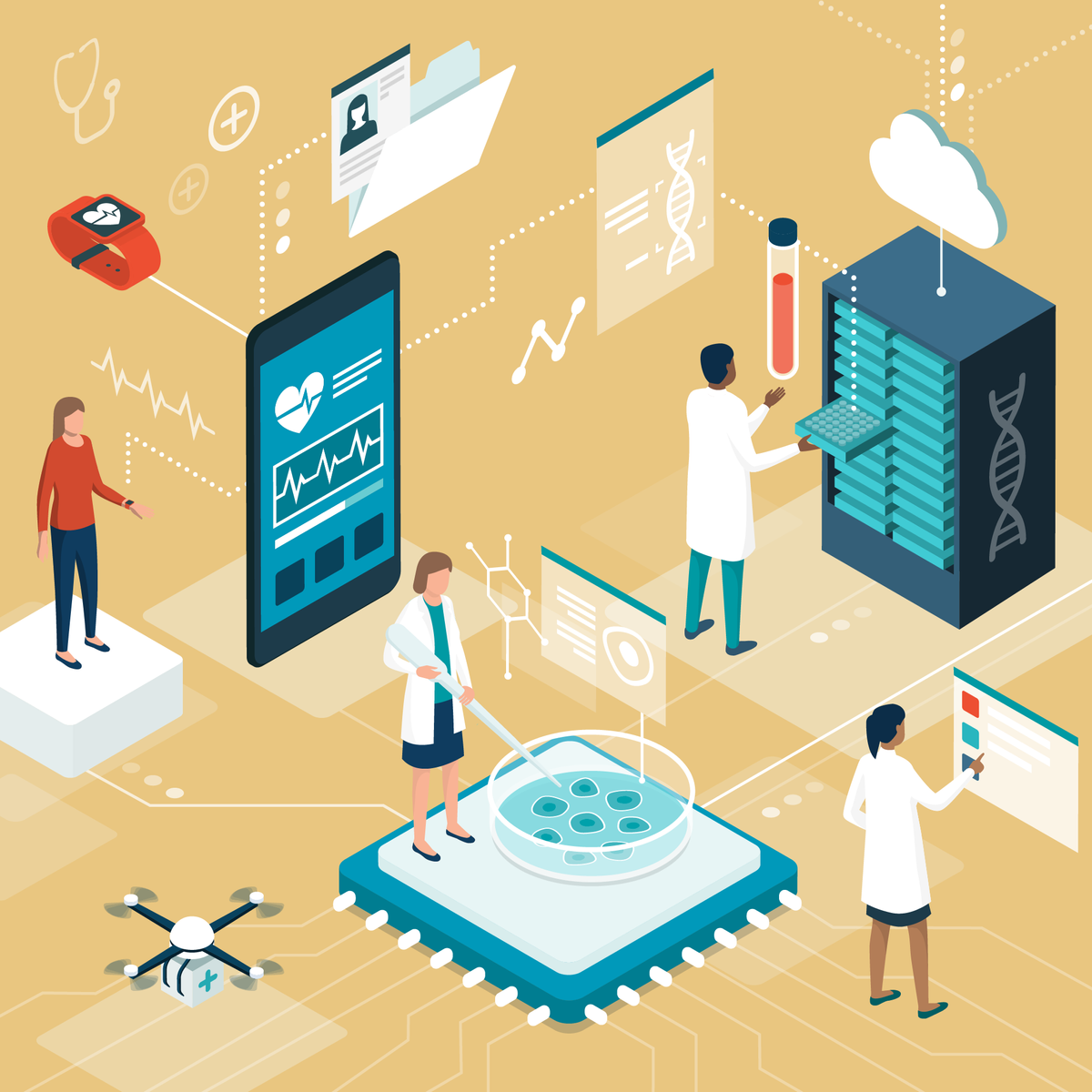
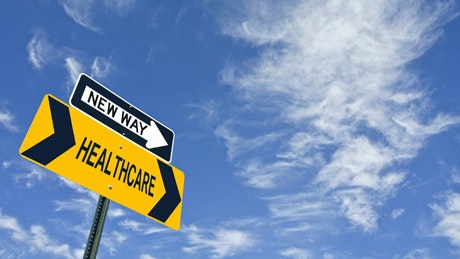
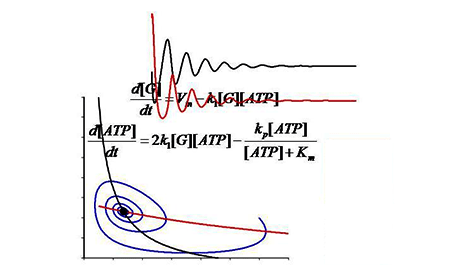
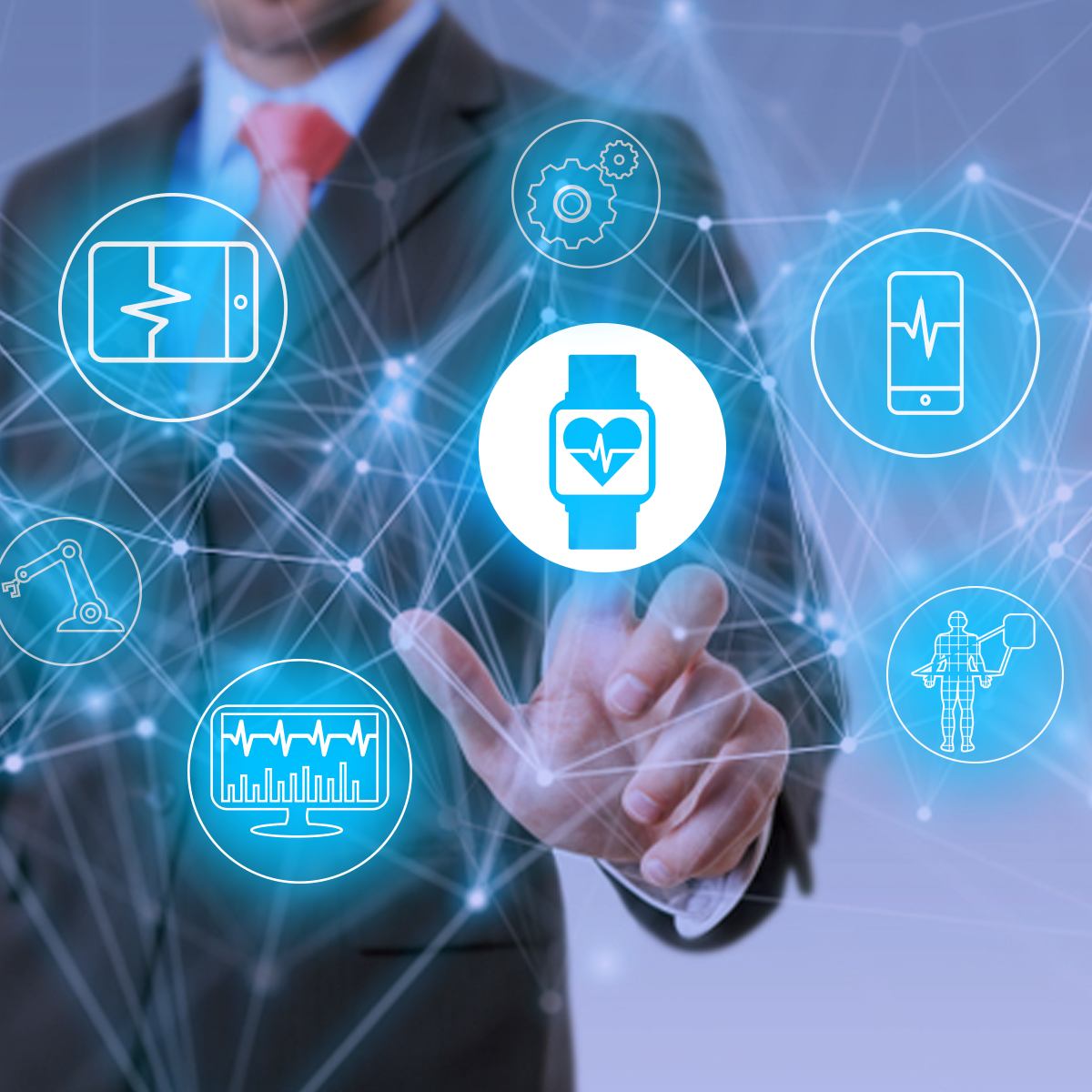
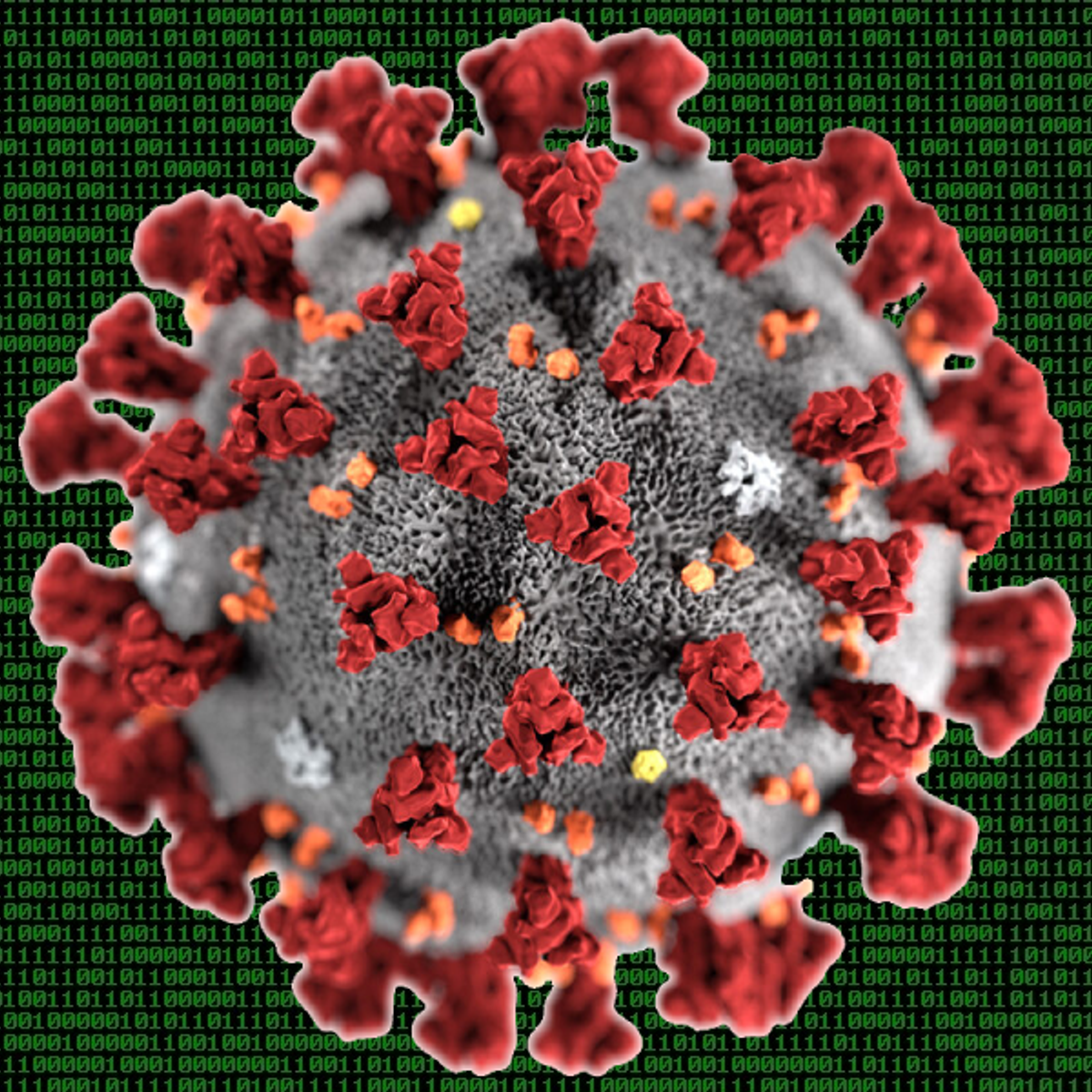
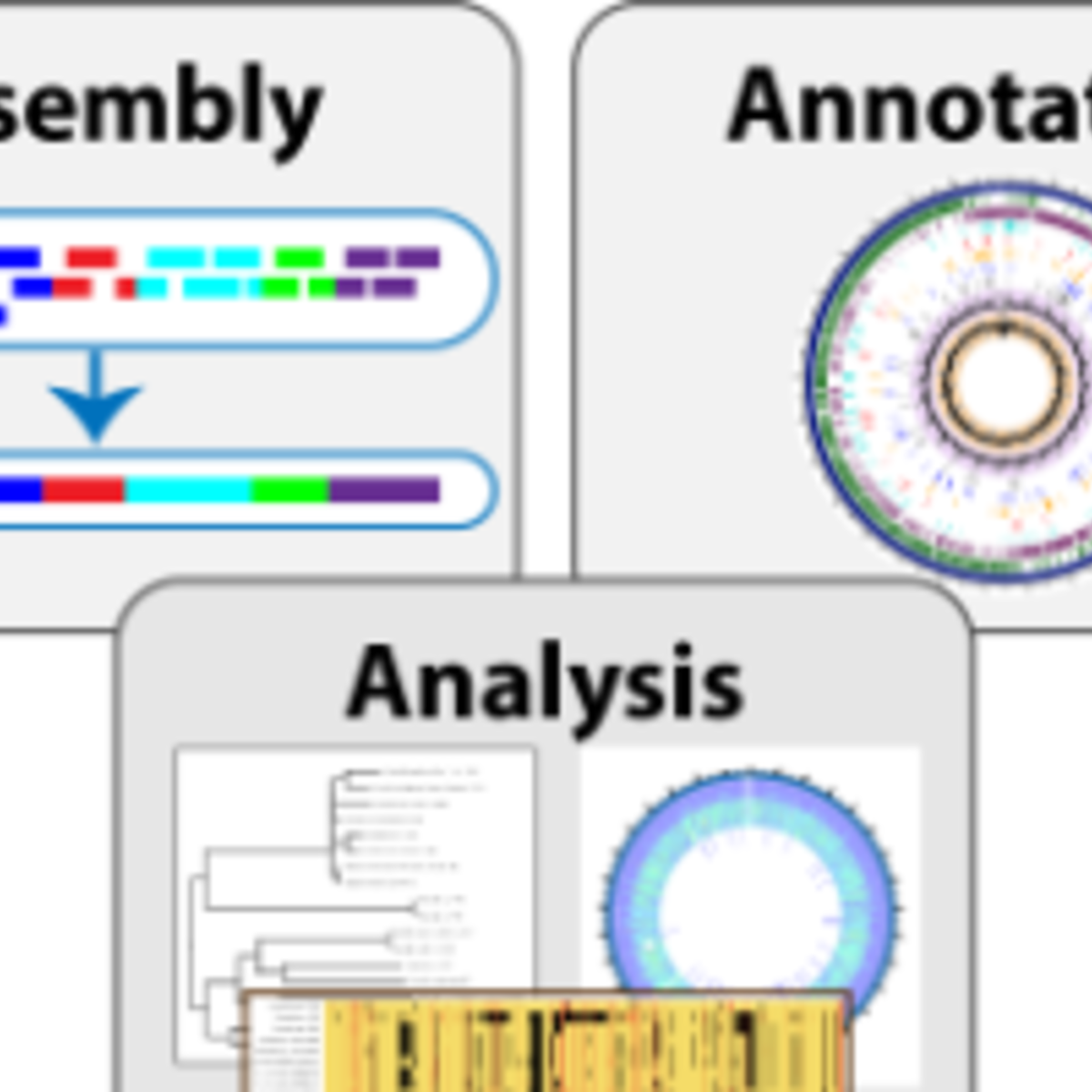
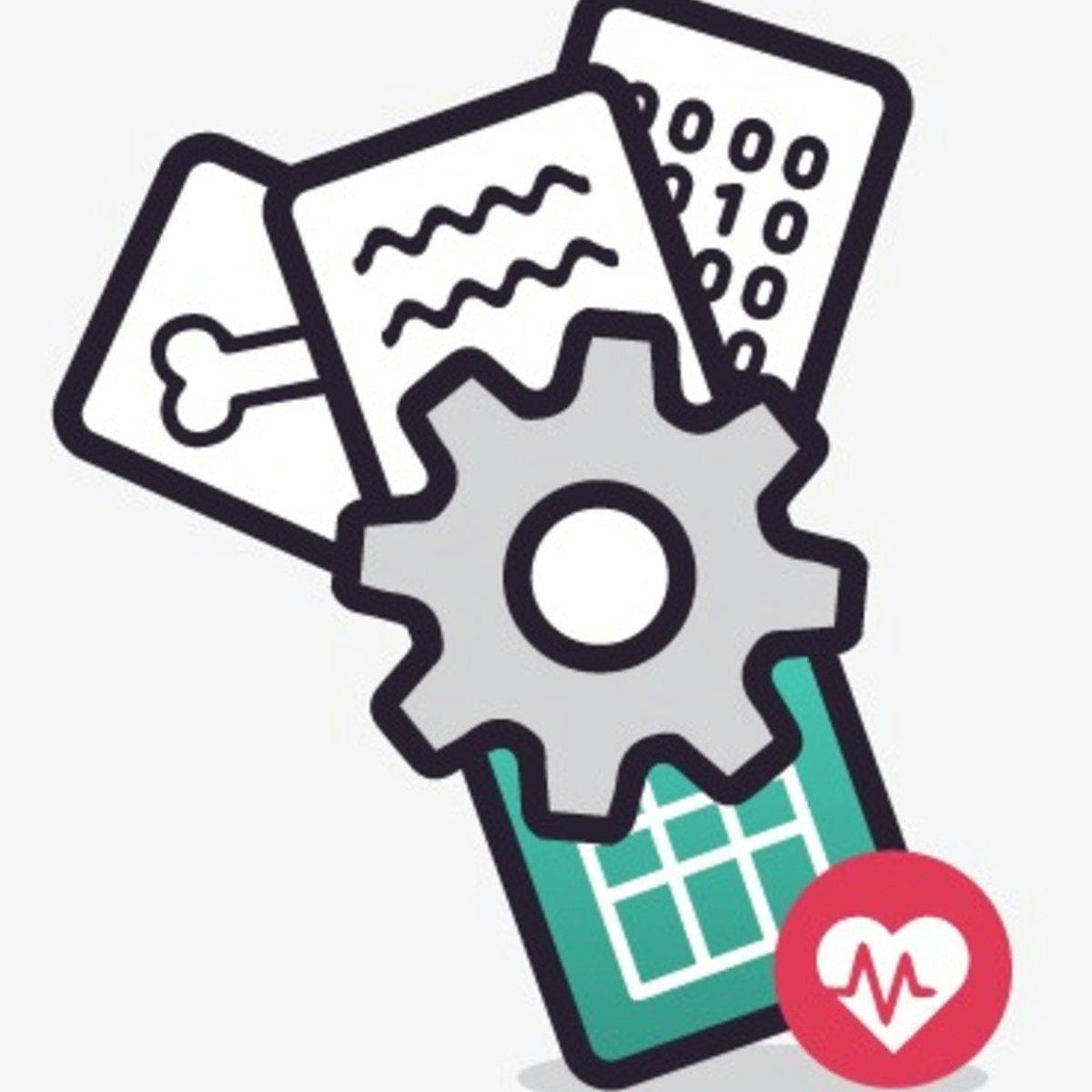
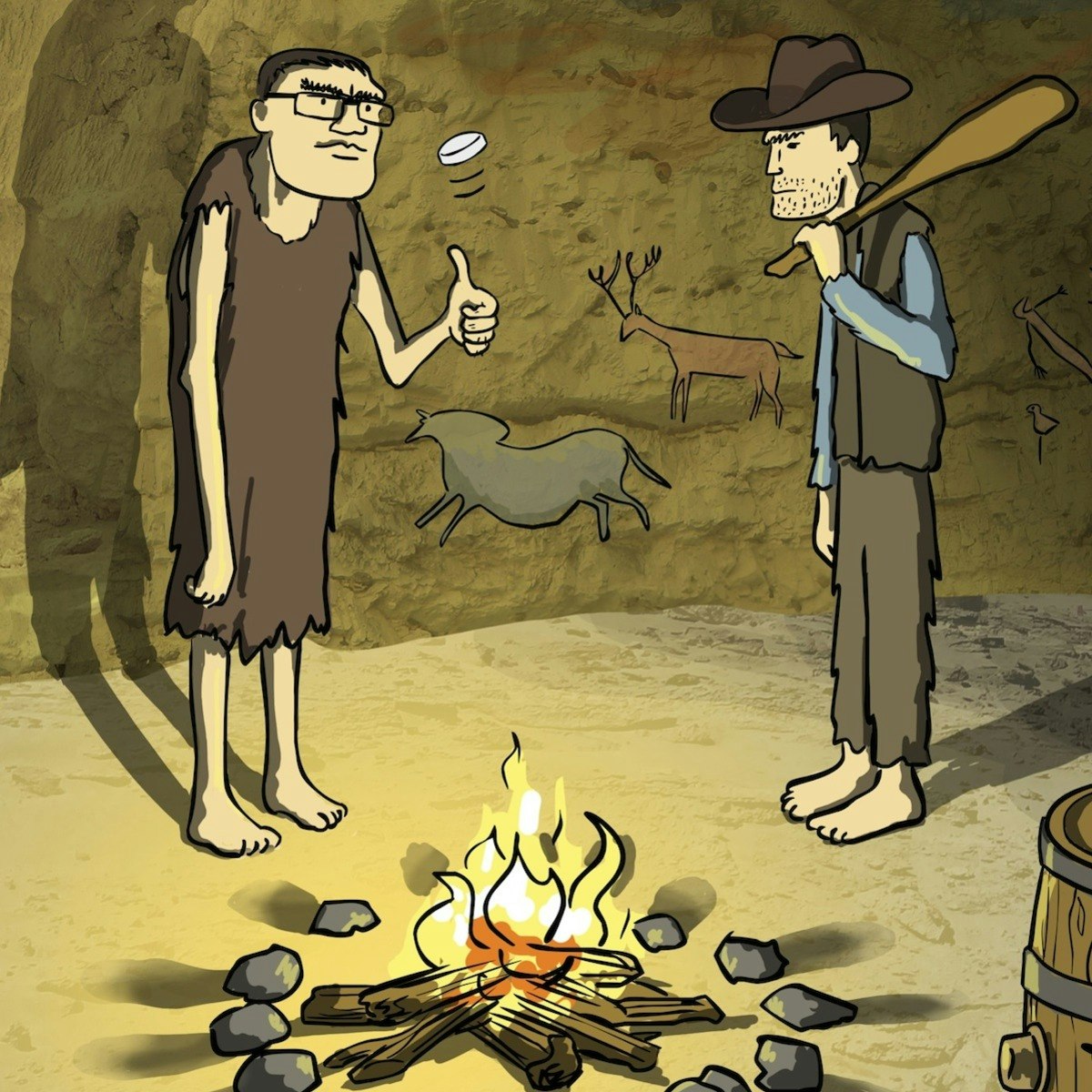
Health Informatics Courses - Page 7
Showing results 61-70 of 93
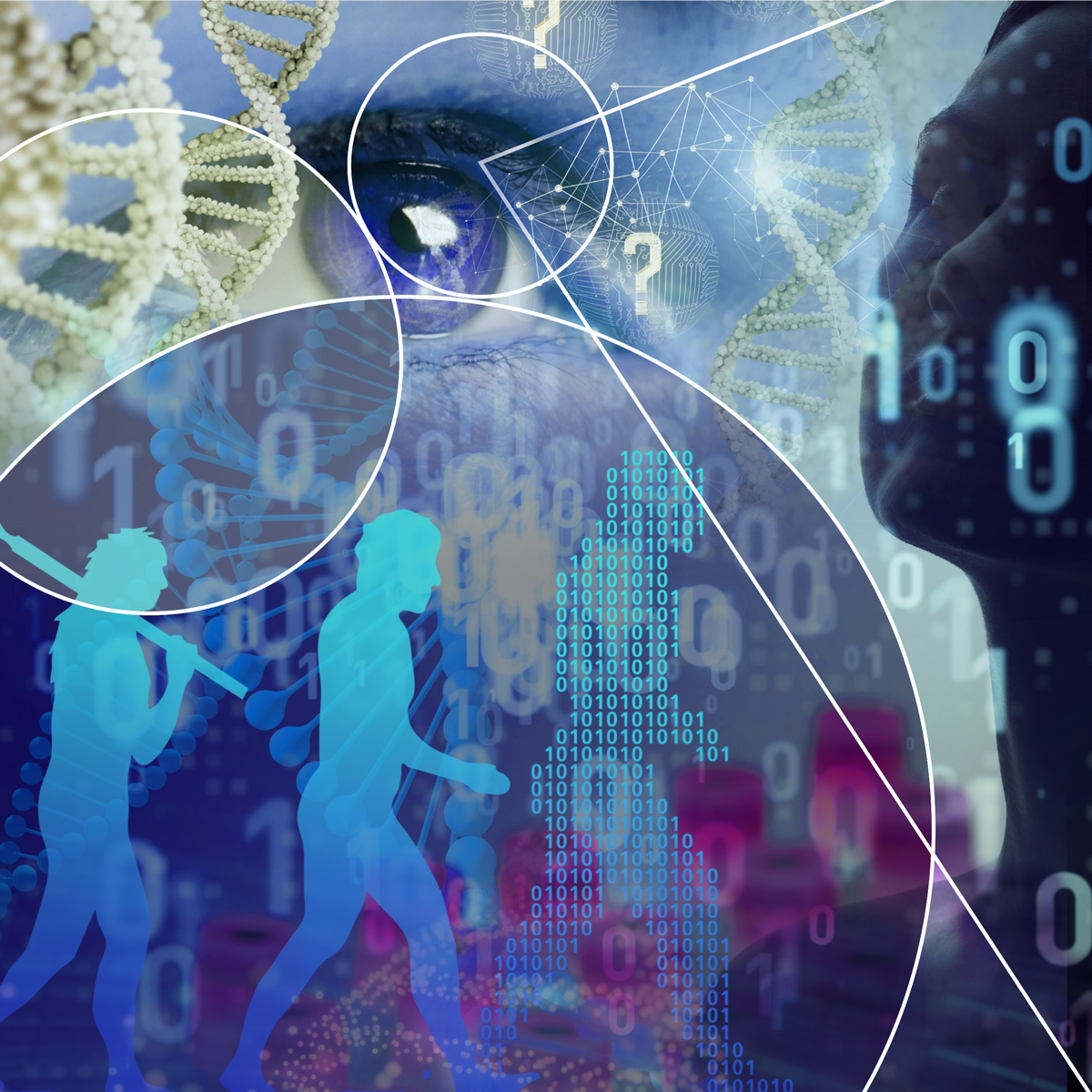
Personalised Medicine from a Nordic Perspective
The technical revolution has generated large amounts of data in healthcare and research, and a rapidly increasing knowledge about factors of importance for the individual’s health. This holds great potential to support a change from the one-size-fits-all paradigm to personalised or precision medicine, to guide and thereby improve each health decision of expected benefit for the patient.
The SARS-CoV-2 pandemic has contributed to a great public and political awareness of the importance of personalised medicine, where the influence of host factors like age, sex, obesity, smoking, co-morbidities etc. confer increased risk of serious COVID-19 illness. It is expected that in the near future, a more systematic and data-driven approach for prediction and risk stratification of COVID-19 patients and many other patient groups, will increase and improve due to better understanding of disease pathology, including the influence of genetic variability and biomarkers on disease risk and outcome.
The Nordic countries have unique welfare systems with general access to healthcare, and longitudinal nationwide health databases and biobanks. This infrastructure combined with unique person identifiers creates an optimal setting for personalised medicine development, and the Nordic model of research, translation, care and education can serve as a forefront example for the rest of the world.
The course in Personalised medicine from a Nordic perspective will introduce, describe, define and discuss the concept of personalised medicine from the aspect of the patient, health-care and the infrastructure available to generate a learning environment that is integrated with everyday care of patients. The course also covers communication of risk and the ethical, legal and social aspects of personalised medicine and presents examples where personalised medicine approach is already used in routine care.
The course was initiated by Faculty leaders in the Education Working Group of Nordic Medical Schools and received funding from the Joint Committee of the Nordic Medical Research Councils (NOS-M). Experts from all the Nordic countries participate in the course:
Saedis Saevarsdottir, Sisse Ostrowski, Hans Tomas Björnsson, Richard Rosenquist Brandell, Henning Bundgaard, Engilbert Sigurðsson, Aarno Palotie, Ole A Andreassen, Runolfur Palsson, Alma Möller, Søren Brunak, Johan Askling, Carsten Utoft Niemann, Rudi Agius, Sofia Ernestam, Saemundur Oddsson, Henrik Ullum, Kari Stefansson, Patrick Sulem, Simon Rasmussen, Jens Lundgren, Anders Perner, Merete Lund Hetland, Heidi Bentzen, Henning Langberg, Sigurdur Kristinsson, Thor Aspelund, Jeanette Knox, David Arnar, Sigurdis Haraldsdottir, Hakon Heimer, Lone Frank, Mette Nordahl Svendsen, Bjorn Hofmann, and Morten Søgaard.
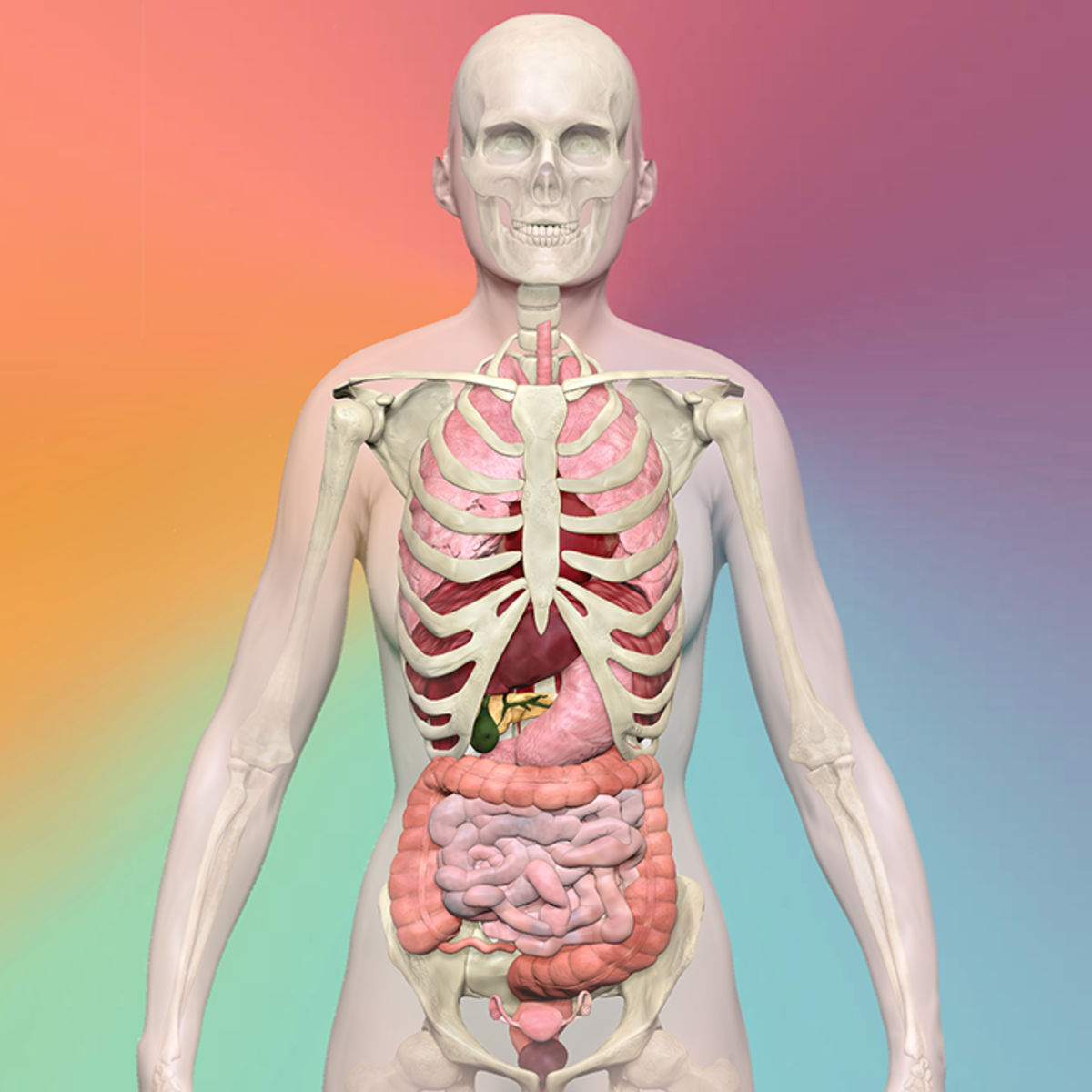
Biomedical Visualisation
Visualisation is a rapidly progressive specialty in academia, research and industry, and becoming the future of science. With the advancement of digital technologies and their applications, biomedical visualisation is an evolving and popular field. With new techniques and technologies to image, process and analyse data related to the human body, and its biological processes, it is at the forefront of the digital revolution. Why not view our course trailer video. Copy and paste this link into your browser https://youtu.be/vB_QcIVSiTs
By the end of this course, you will be able to:
Define and describe anatomical terminology related to the human body.
Describe the various body systems, what comprises them, and how they relate to function.
Create your own 3D modelling and animations using industry standard, open-source software (Blender).
Analyse various visualisation techniques and methods, and be able to apply them to areas of biomedical science.
This course is the first of its kind on Coursera. It explores the structure and function of the human body including terminology used. It showcases visualisation techniques available using technology to image and display data related to the body and biological processes.. It also provides training in the creation of animations, and examines the applicability of different types of reality.
You will learn from experts in these fields and with the knowledge you will gain from this course, be able to evaluate and apply how best to use visualisation in your own discipline.
No prior experience is necessary but should be able to learn at an intermediate level.
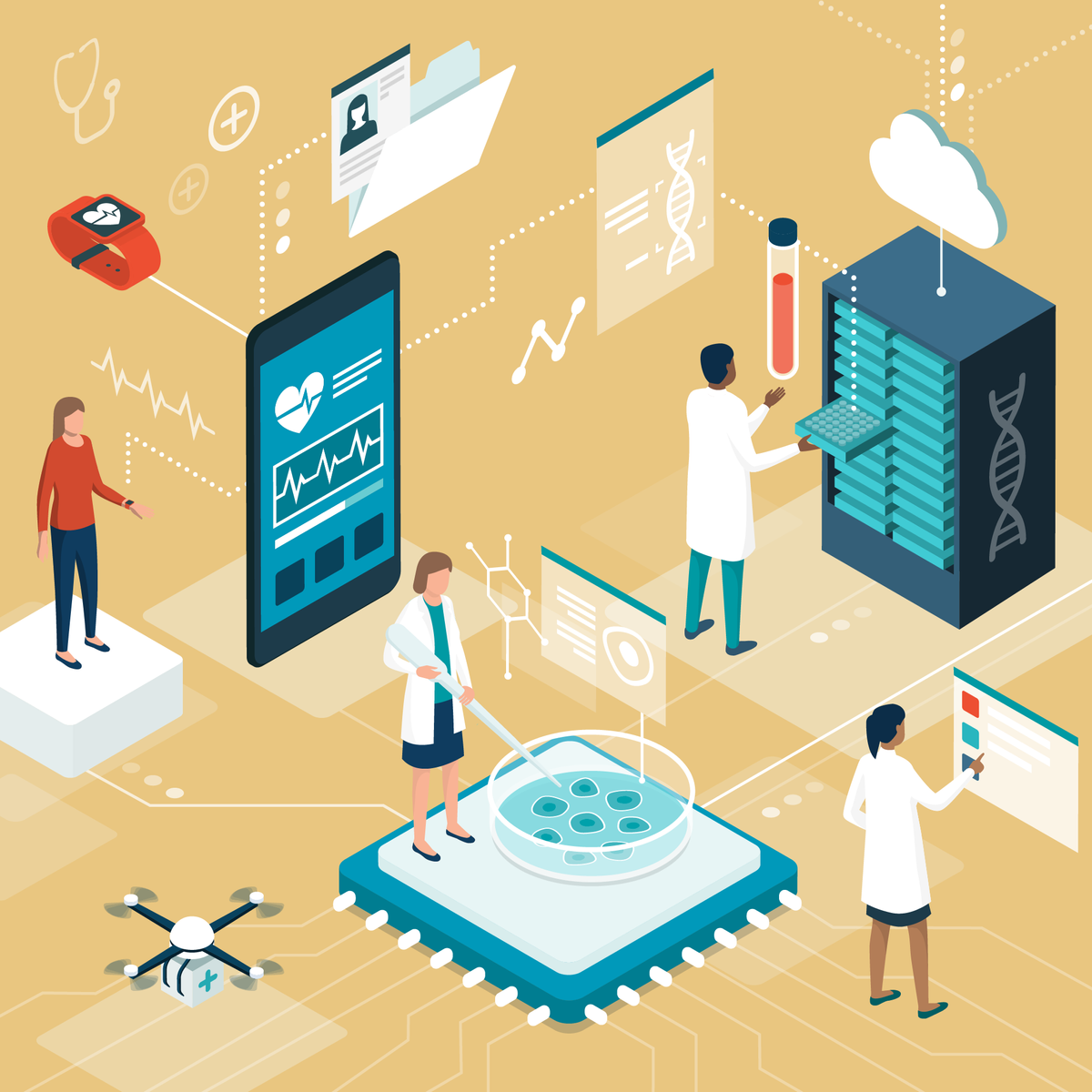
Evaluations of AI Applications in Healthcare
With artificial intelligence applications proliferating throughout the healthcare system, stakeholders are faced with both opportunities and challenges of these evolving technologies. This course explores the principles of AI deployment in healthcare and the framework used to evaluate downstream effects of AI healthcare solutions.
The Stanford University School of Medicine is accredited by the Accreditation Council for Continuing Medical Education (ACCME) to provide continuing medical education for physicians. Visit the FAQs below for important information regarding 1) Date of original release and Termination or expiration date; 2) Accreditation and Credit Designation statements; 3) Disclosure of financial relationships for every person in control of activity content.
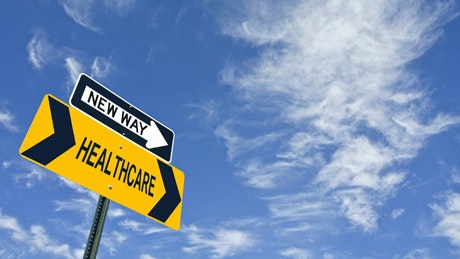
Interprofessional Healthcare Informatics
Interprofessional Healthcare Informatics is a graduate-level, hands-on interactive exploration of real informatics tools and techniques offered by the University of Minnesota and the University of Minnesota's National Center for Interprofessional Practice and Education. We will be incorporating technology-enabled educational innovations to bring the subject matter to life. Over the 10 modules, we will create a vital online learning community and a working healthcare informatics network.
We will explore perspectives of clinicians like dentists, physical therapists, nurses, and physicians in all sorts of practice settings worldwide. Emerging technologies, telehealth, gaming, simulations, and eScience are just some of the topics that we will consider.
Throughout the course, we’ll focus on creativity, controversy, and collaboration - as we collectively imagine and create the future within the rapidly evolving healthcare informatics milieu. All healthcare professionals and IT geeks are welcome!
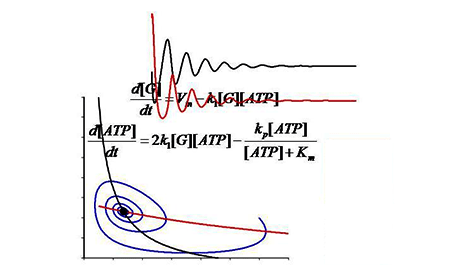
Dynamical Modeling Methods for Systems Biology
An introduction to dynamical modeling techniques used in contemporary Systems Biology research.
We take a case-based approach to teach contemporary mathematical modeling
techniques. The course is appropriate for advanced undergraduates and beginning graduate students. Lectures provide biological background and describe the development of both classical mathematical models and more recent representations of biological processes. The course will be useful for students who plan to use experimental techniques as their approach in the laboratory and employ computational modeling as a tool to draw deeper understanding of experiments. The course should also be valuable as an introductory overview for students planning to conduct original research in modeling biological systems.
This course focuses on dynamical modeling techniques used in Systems Biology research. These techniques are based on biological mechanisms, and simulations with these models generate predictions that can subsequently be tested experimentally. These testable predictions frequently provide novel insight into biological processes. The approaches taught here can be grouped into the following categories: 1) ordinary differential equation-based models, 2) partial differential equation-based models, and 3) stochastic models.
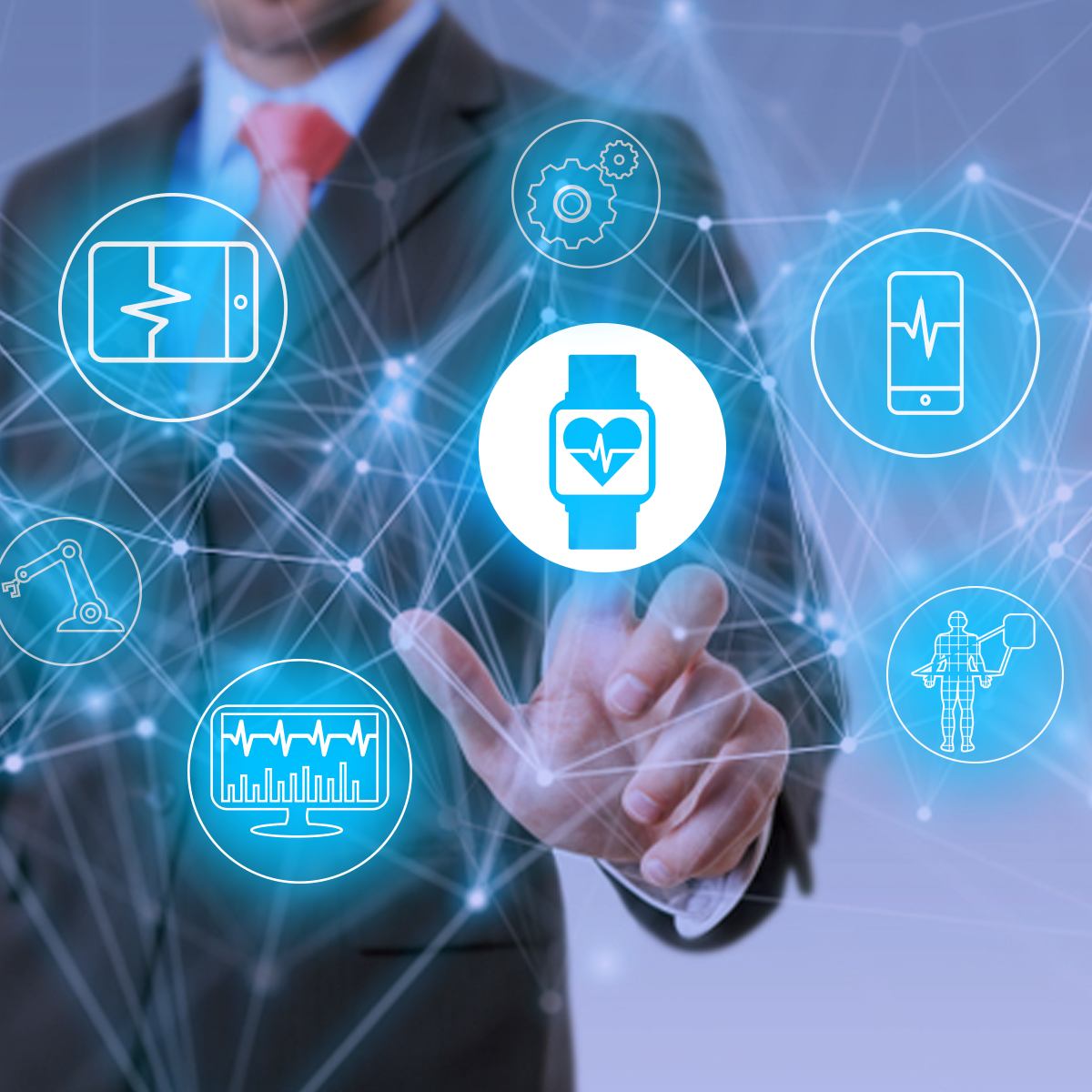
Business Application of Machine Learning and Artificial Intelligence in Healthcare
The future of healthcare is becoming dependent on our ability to integrate Machine Learning and Artificial Intelligence into our organizations. But it is not enough to recognize the opportunities of AI; we as leaders in the healthcare industry have to first determine the best use for these applications ensuring that we focus our investment on solving problems that impact the bottom line.
Throughout these four modules we will examine the use of decision support, journey mapping, predictive analytics, and embedding Machine Learning and Artificial Intelligence into the healthcare industry. By the end of this course you will be able to:
1. Determine the factors involved in decision support that can improve business performance across the provider/payer ecosystem.
2. Identify opportunities for business applications in healthcare by applying journey mapping and pain point analysis in a real world context.
3. Identify differences in methods and techniques in order to appropriately apply to pain points using case studies.
4. Critically assess the opportunities to leverage decision support in adapting to trends in the industry.
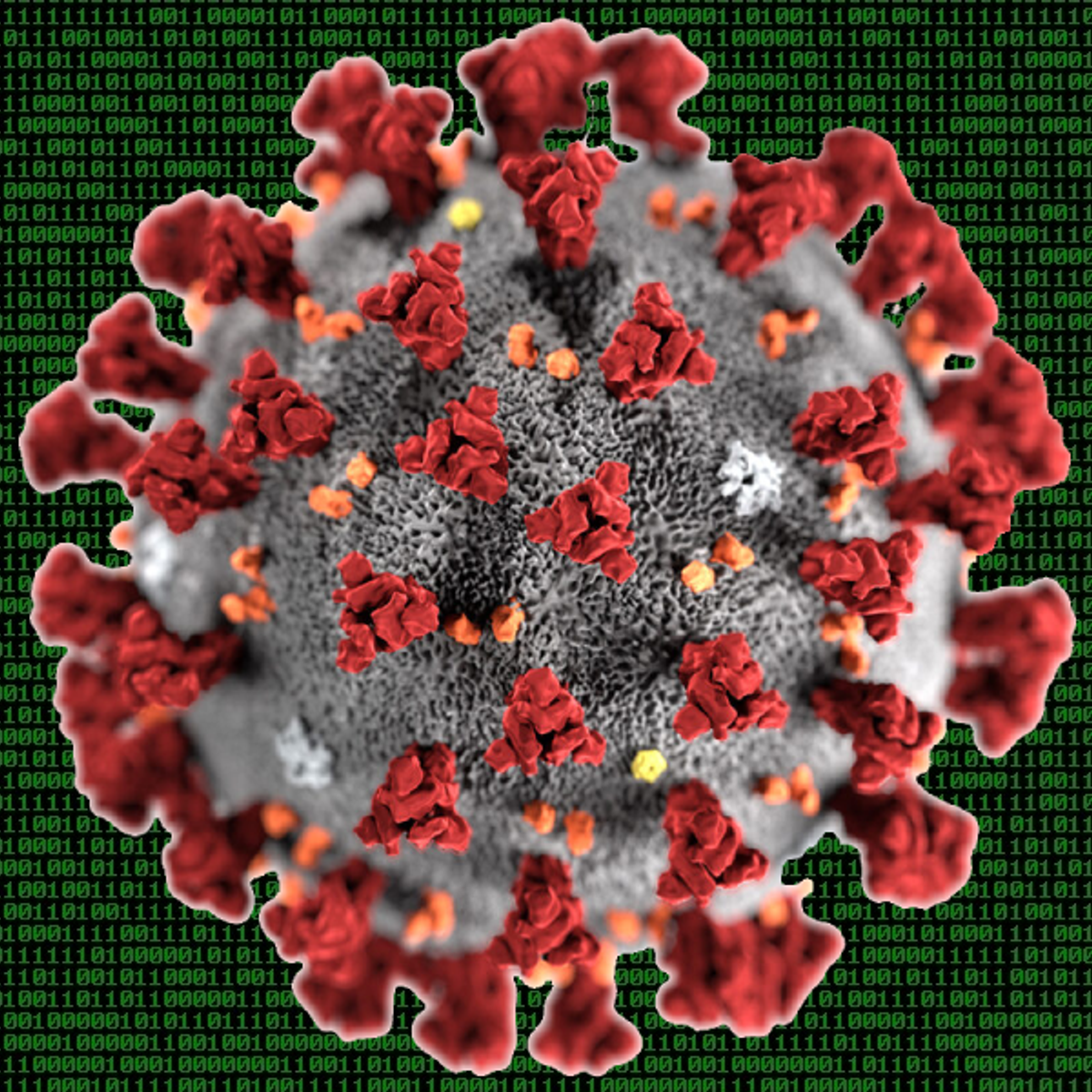
Hacking COVID-19 — Course 2: Decoding SARS-CoV-2's Secrets
In this course, you will follow in the footsteps of the bioinformaticians investigating the COVID-19 outbreak by annotating the SARS-CoV-2 genome and using the annotation to design a COVID-19 diagnostic test. Whether you’re new to the world of computational biology, or you’re a bioinformatics expert seeking to learn about its applications in the COVID-19 pandemic, or somewhere in between, this course is for you! As you go through this journey, we will introduce and explain genomic concepts and give you many opportunities to practice your skills, and we will provide a series of problems with gradually increasing complexity. This second course will only discuss the annotation of the SARS-CoV-2 genome, but future courses in this series will explore follow-up bioinformatics analyses used in the COVID-19 pandemic.
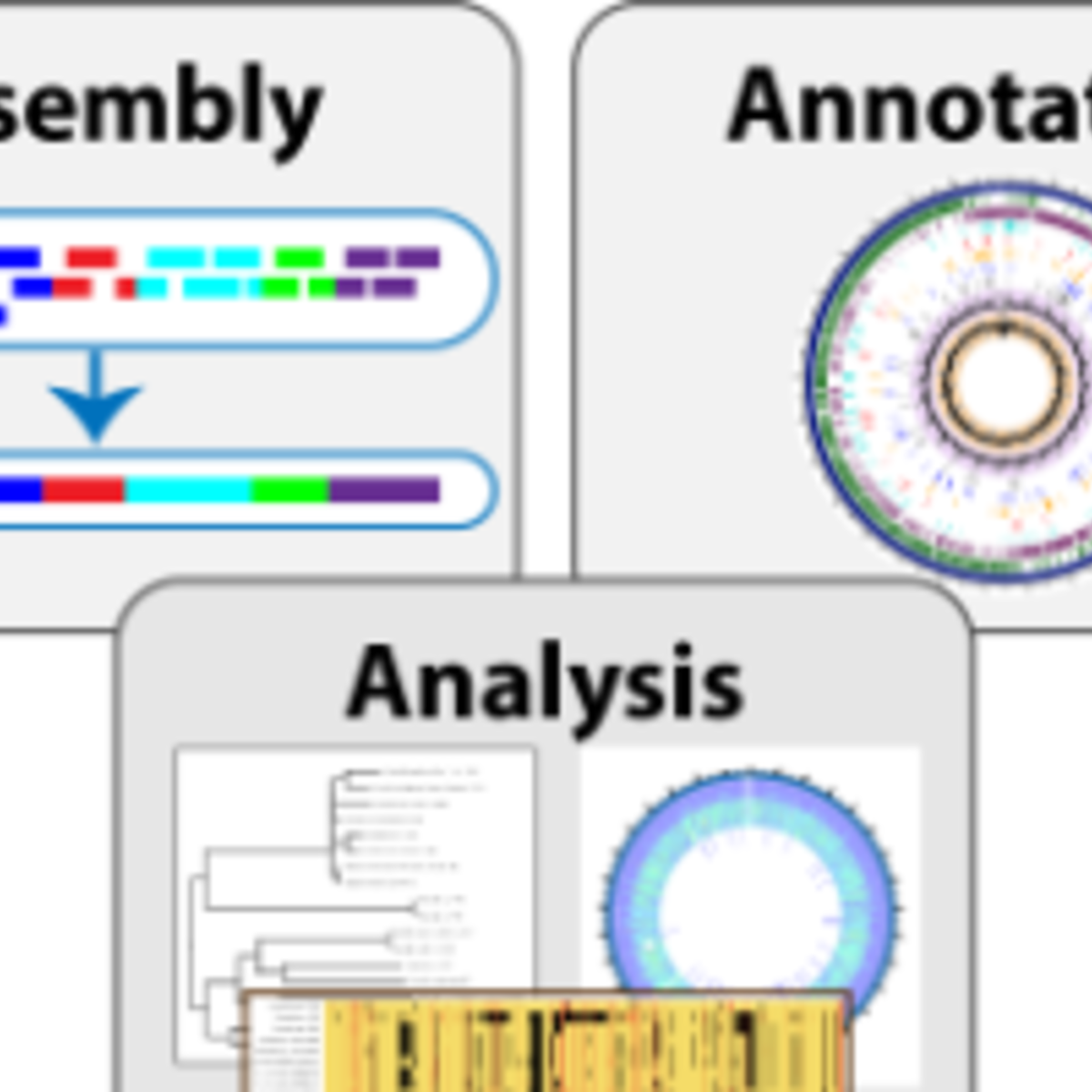
Bacterial Bioinformatics
This course provides demonstrations and exercises for performing common genomics-based analysis tasks of bacterial sequence data. It uses PATRIC, the PathoSystems Resource Integration Center, as the platform for analysis. PATRIC is the NIH/NIAID-funded bacterial Bioinformatics Resource Center, providing comprehensive bacterial genomic data with integrated analysis tools and visualizations. PATRIC also provides a private workspace where users can upload and analyze their own data.
Course participants will gain skills needed to do comparative analysis of bacterial genomes, starting with raw sequence data.The lessons in the first module cover genome assembly, annotation, phylogenetic tree construction, and protein family / proteome comparisons. Each lesson builds on the previous, creating a complete baseline analysis workflow.
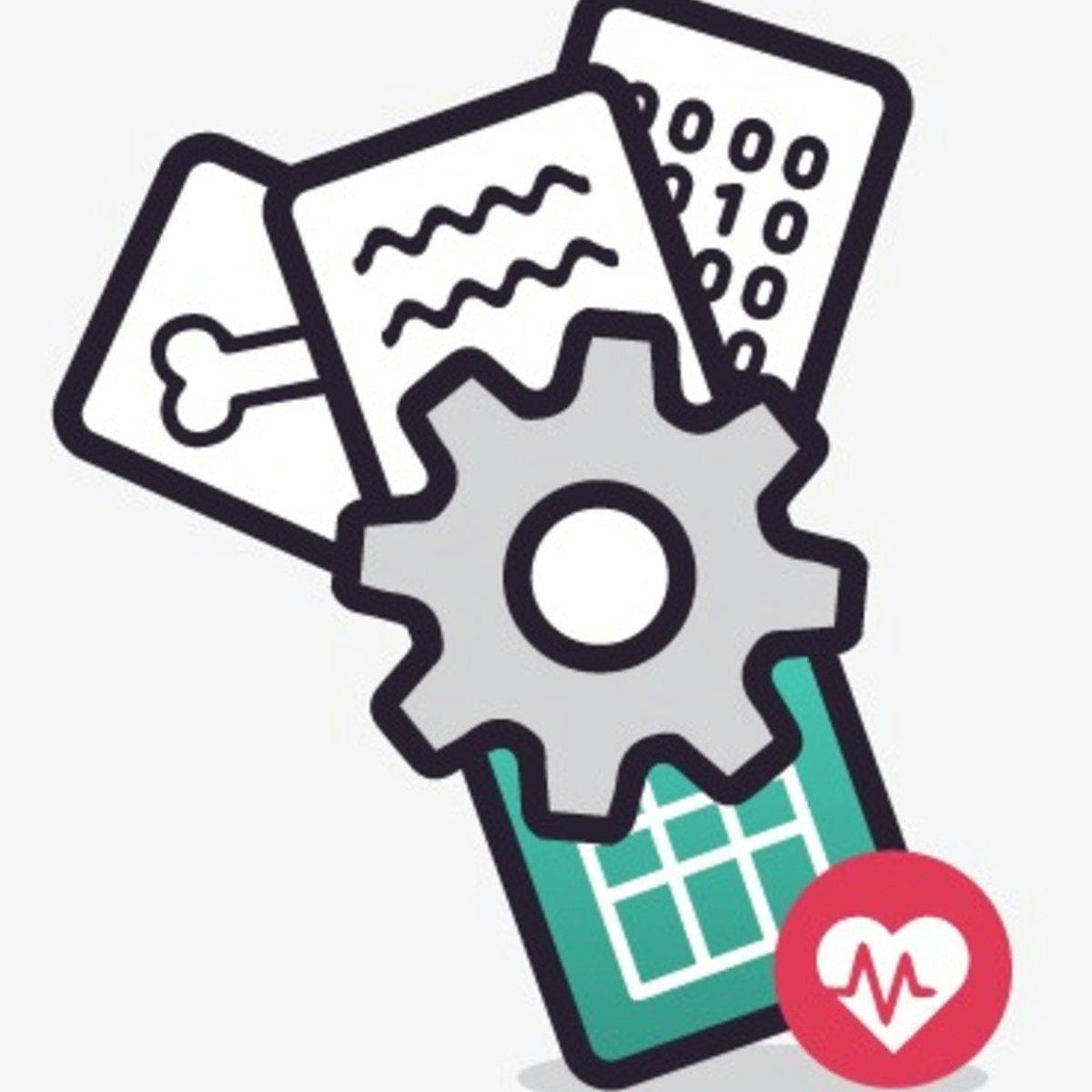
Foundations of mining non-structured medical data
The goal of this course is to understand the foundations of Big Data and the data that is being generated in the health domain and how the use of technology would help to integrate and exploit all those data to extract meaningful information that can be later used in different sectors of the health domain from physicians to management, from patients to caregivers, etc.
The course offers a high-level perspective of the importance of the medical context within the European context, the types of data that are managed in the health (clinical) context, the challenges to be addressed in the mining of unstructured medical data (text and image) as well as the opportunities from the analytical point of view with an introduction to the basics of data analytics field.
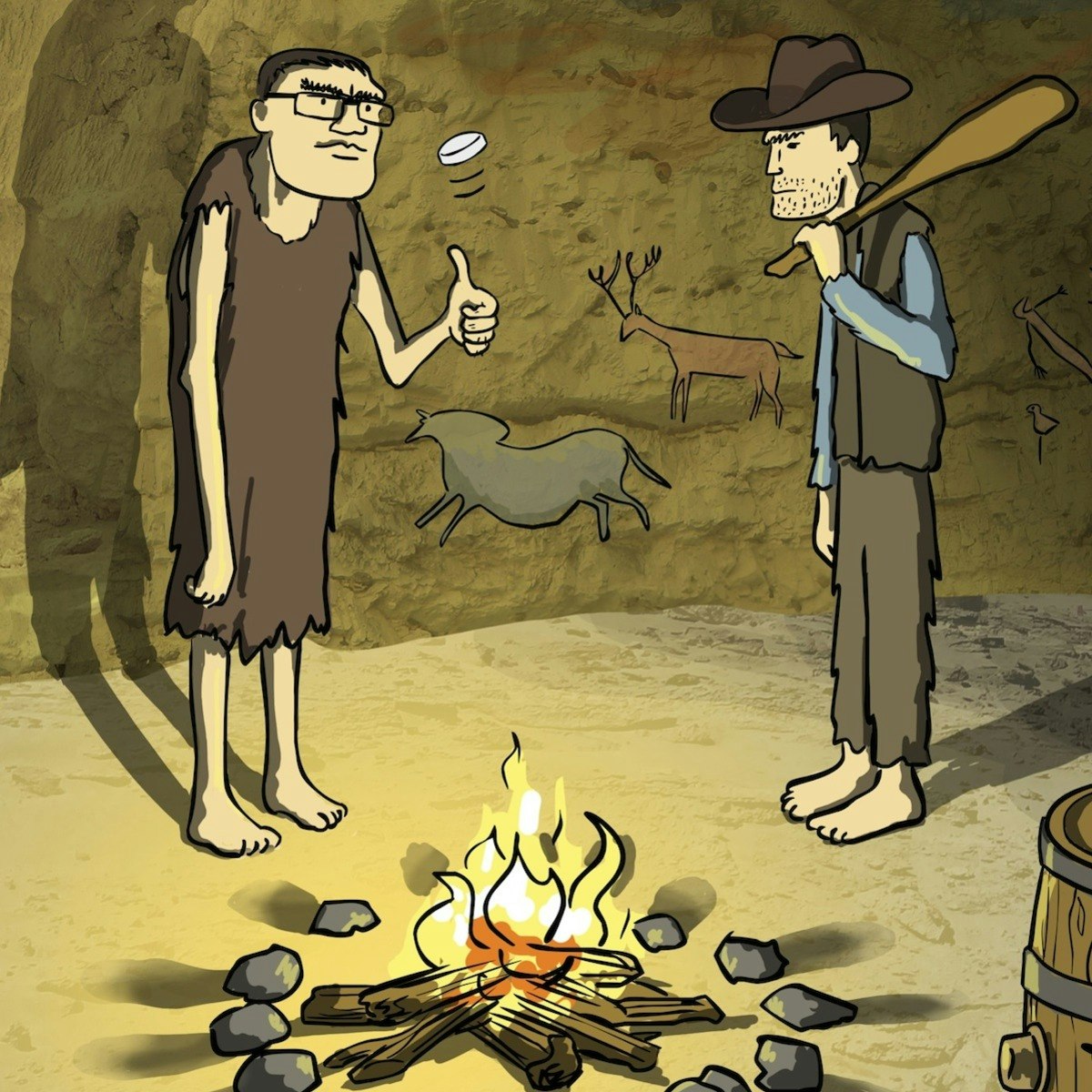
Genomic Data Science and Clustering (Bioinformatics V)
How do we infer which genes orchestrate various processes in the cell? How did humans migrate out of Africa and spread around the world? In this class, we will see that these two seemingly different questions can be addressed using similar algorithmic and machine learning techniques arising from the general problem of dividing data points into distinct clusters.
In the first half of the course, we will introduce algorithms for clustering a group of objects into a collection of clusters based on their similarity, a classic problem in data science, and see how these algorithms can be applied to gene expression data.
In the second half of the course, we will introduce another classic tool in data science called principal components analysis that can be used to preprocess multidimensional data before clustering in an effort to greatly reduce the number dimensions without losing much of the "signal" in the data.
Finally, you will learn how to apply popular bioinformatics software tools to solve a real problem in clustering.
Popular Internships and Jobs by Categories
Browse
© 2024 BoostGrad | All rights reserved