Back to Courses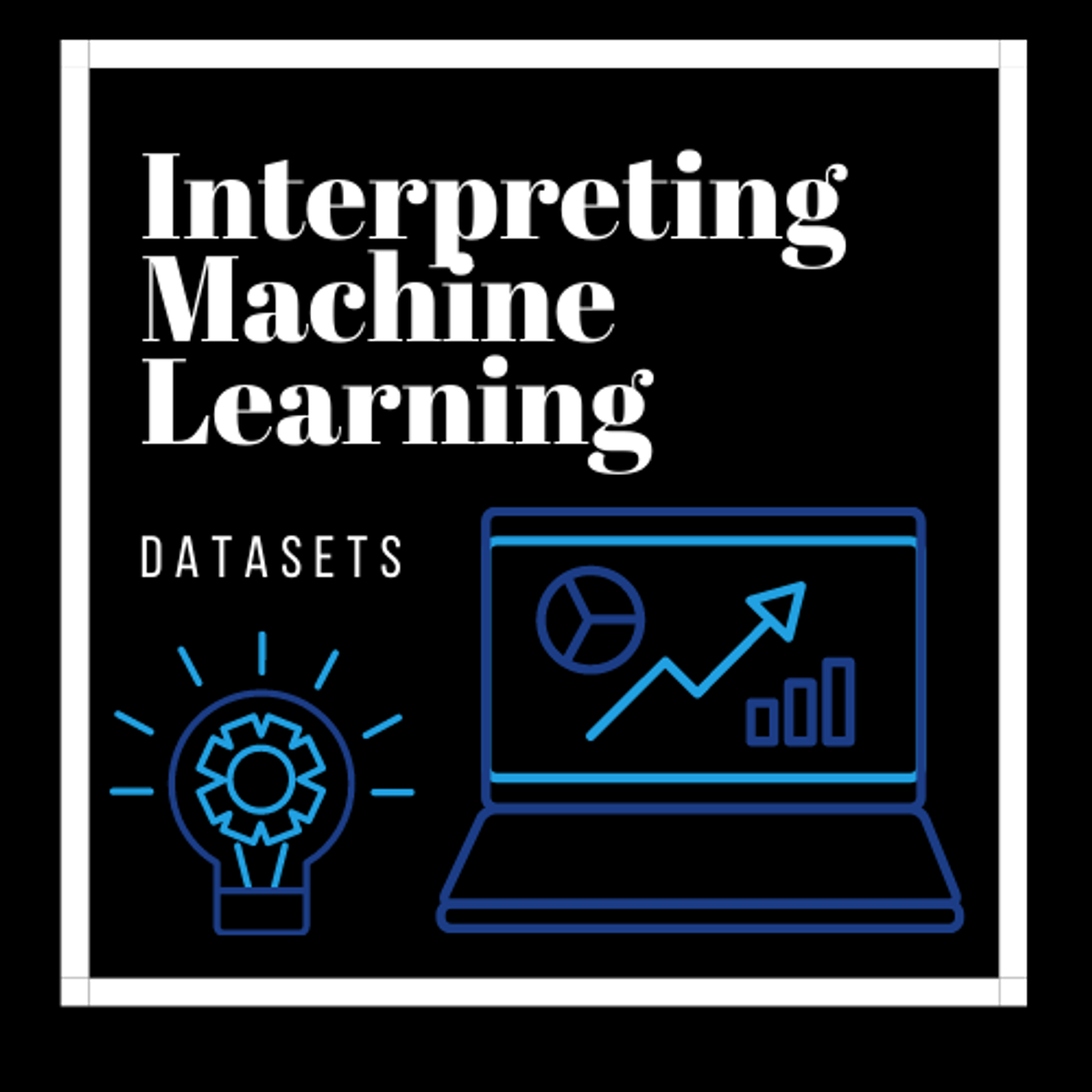
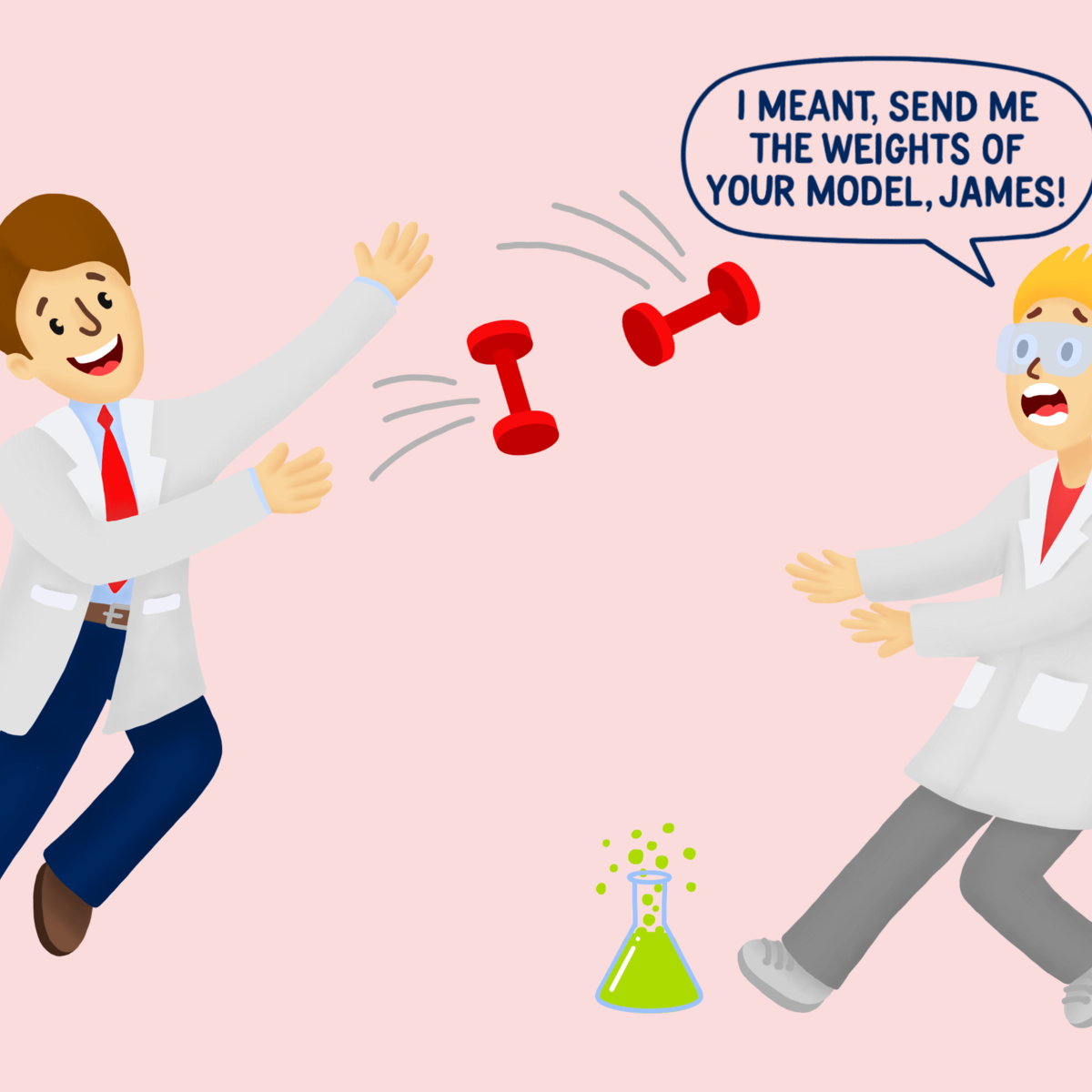
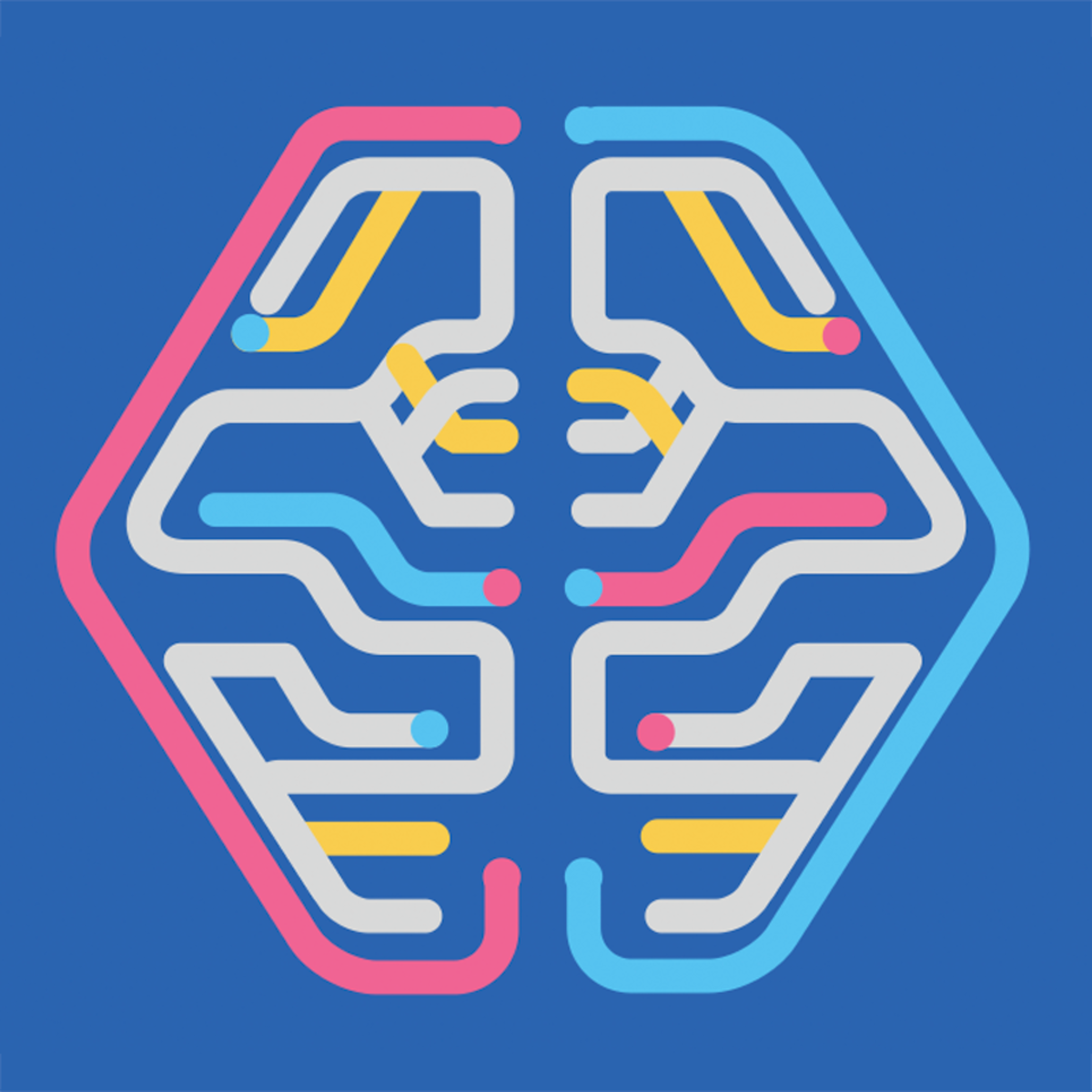
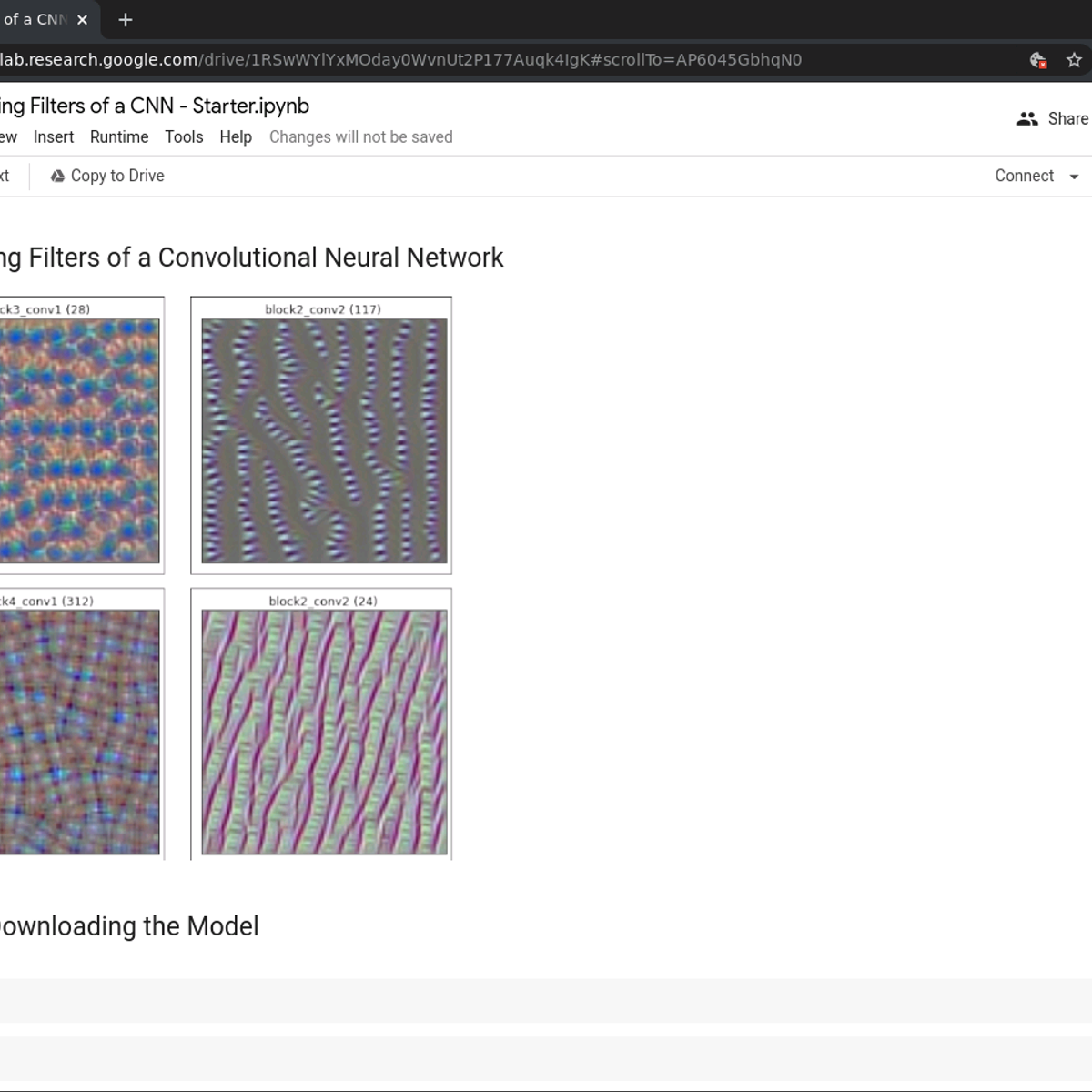
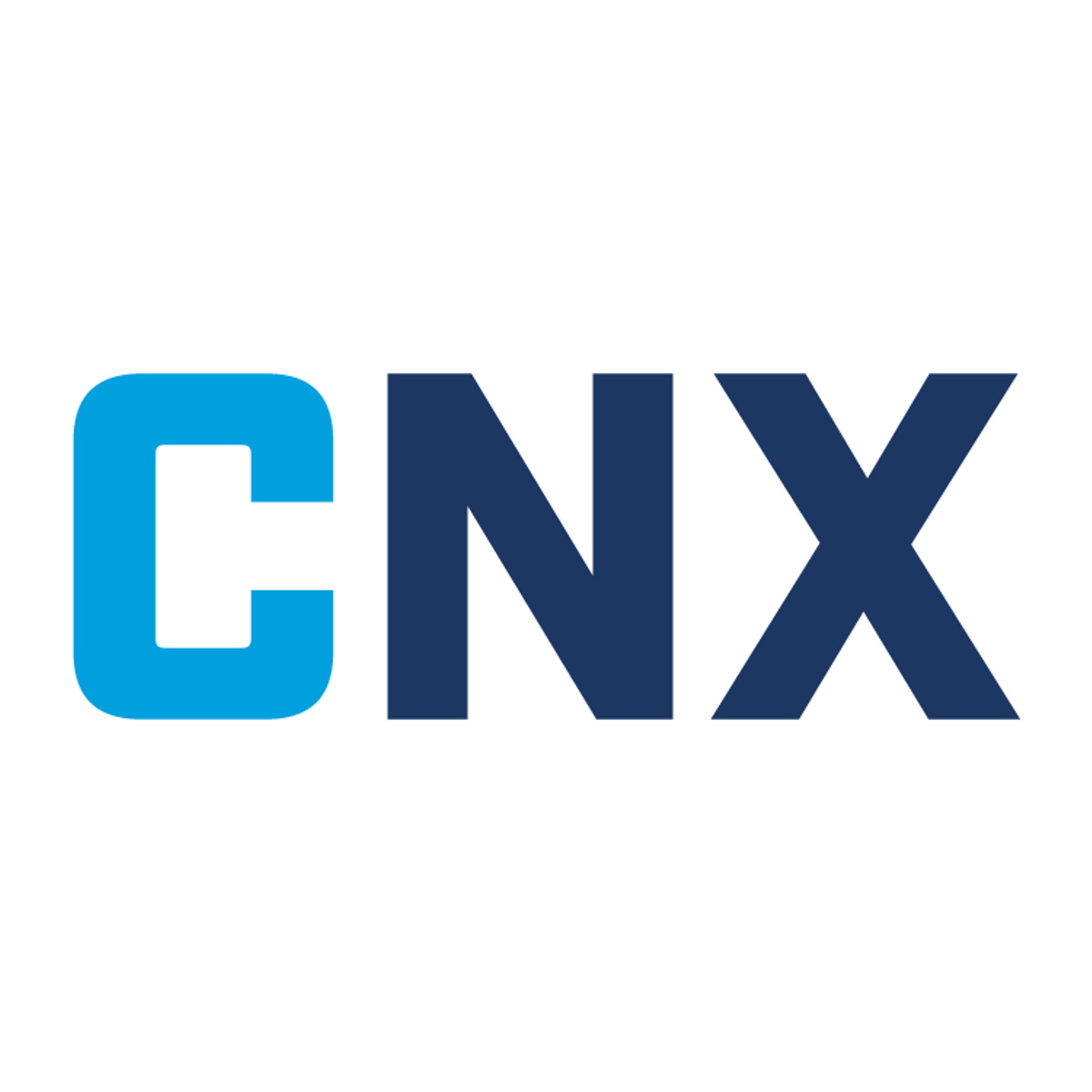
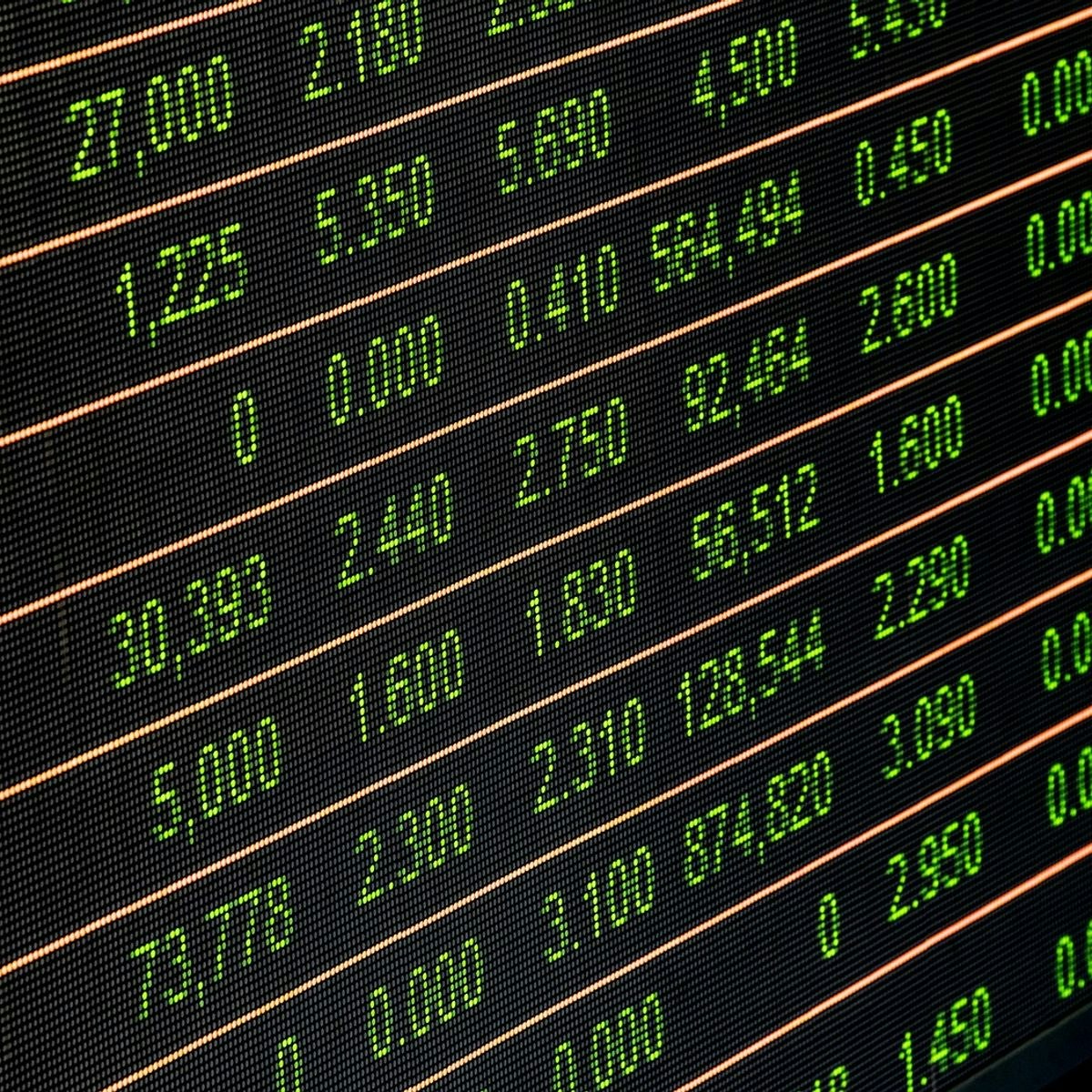
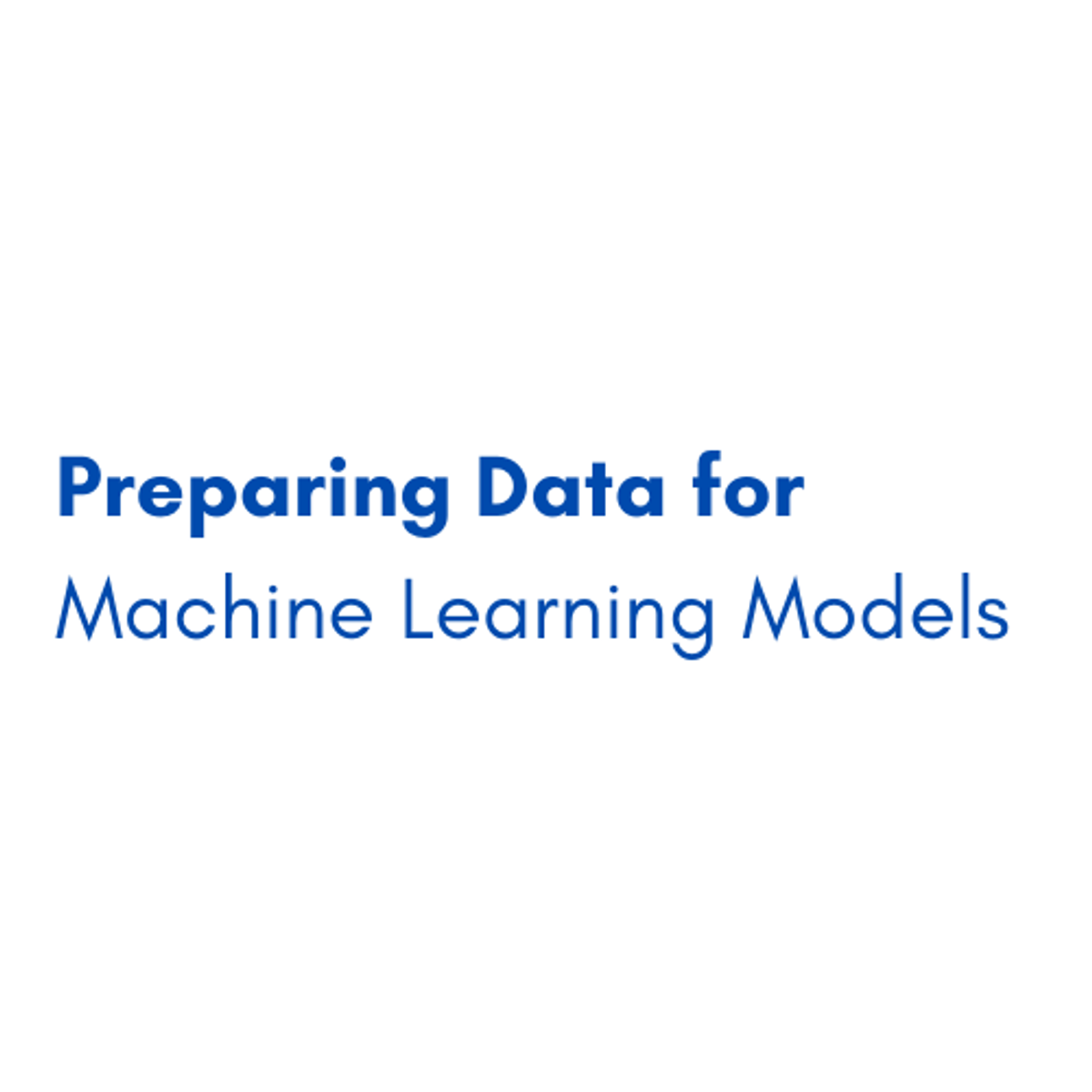
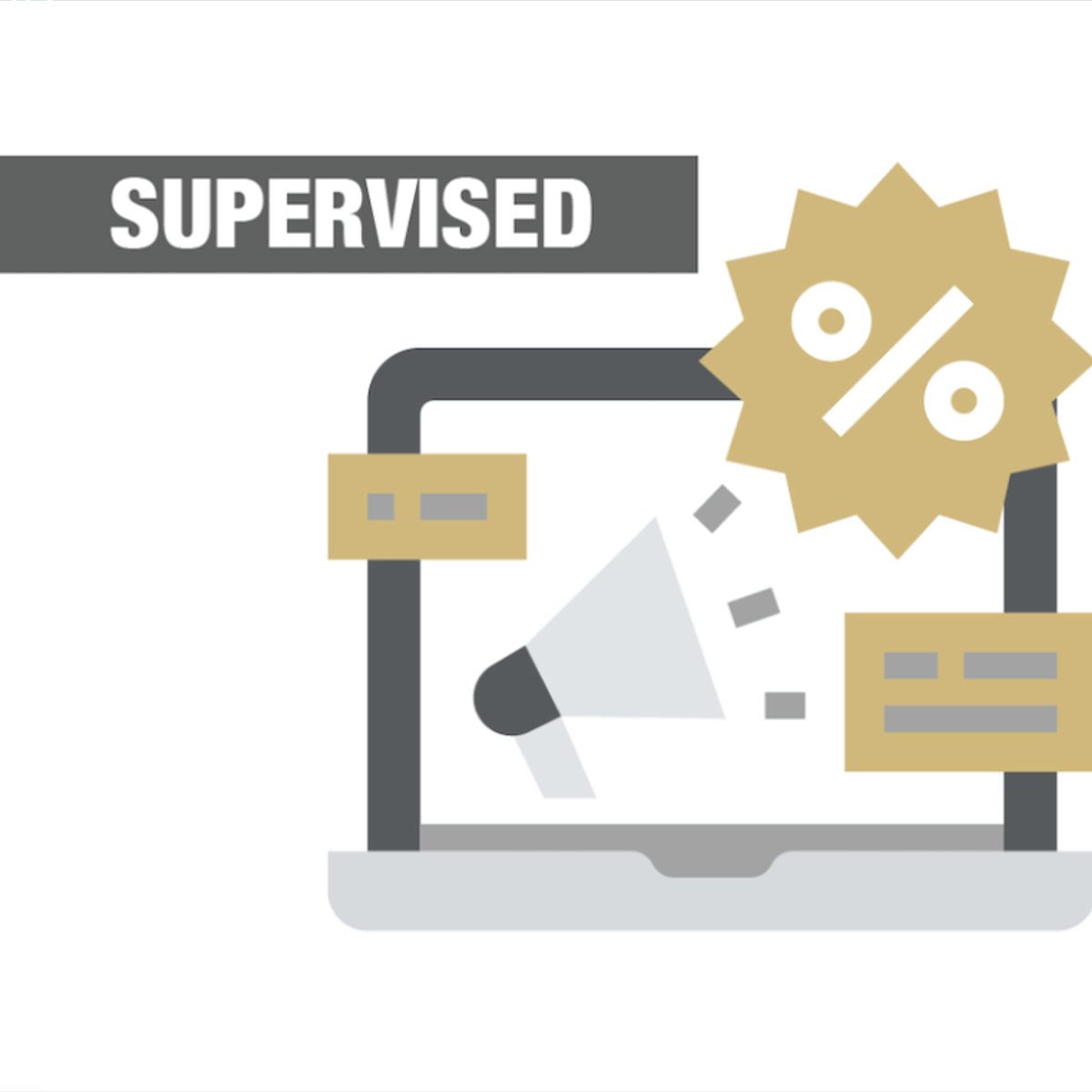
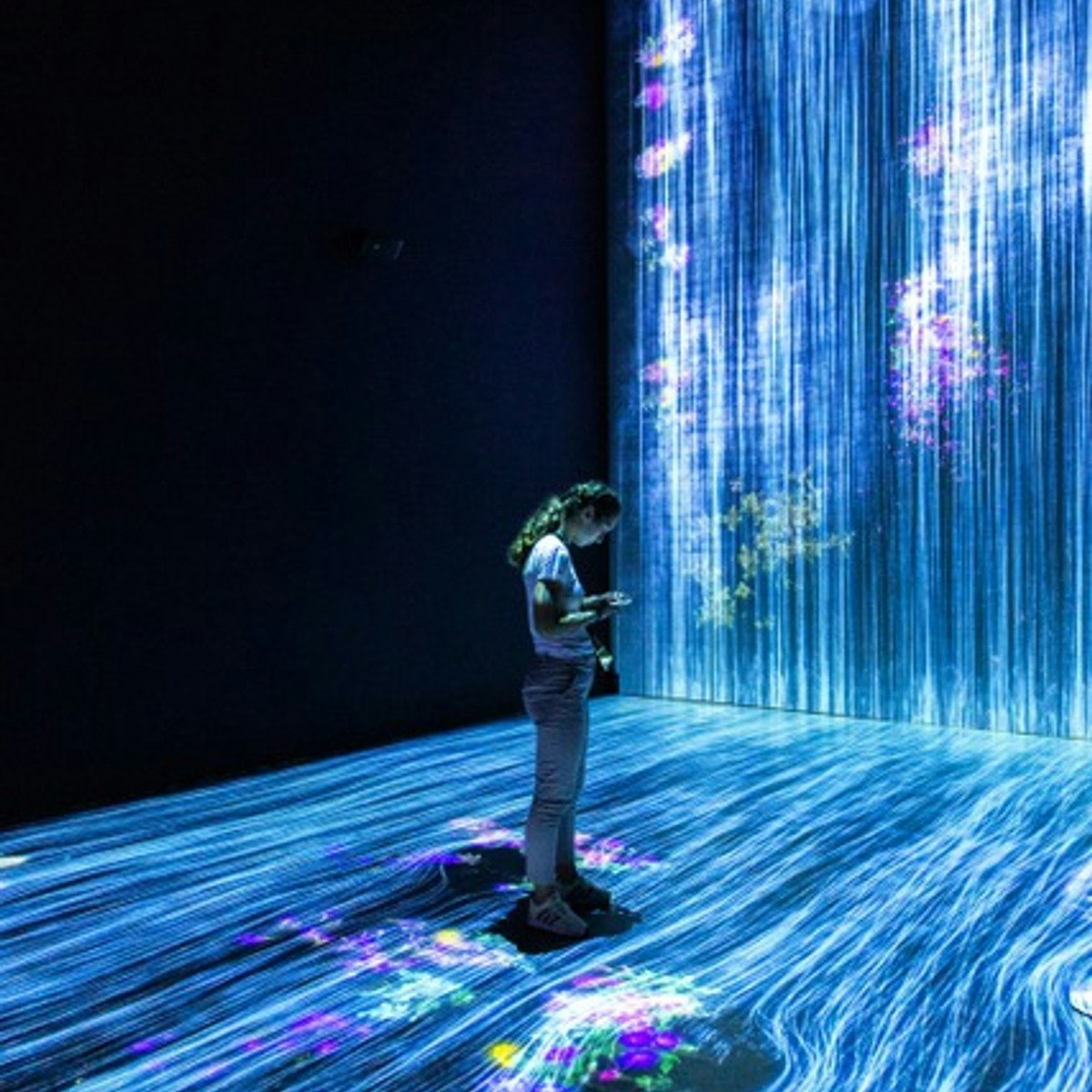
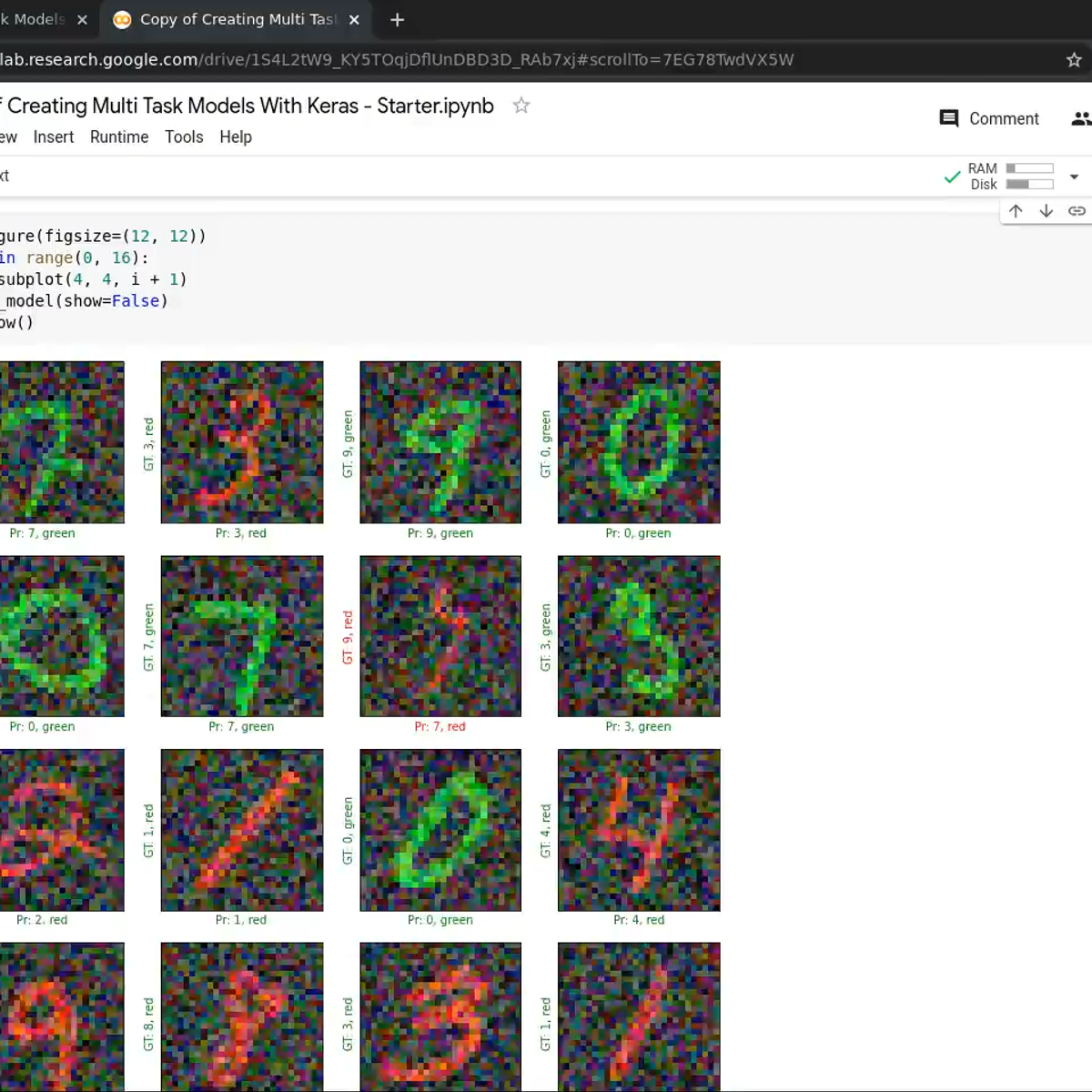
Machine Learning Courses - Page 4
Showing results 31-40 of 485
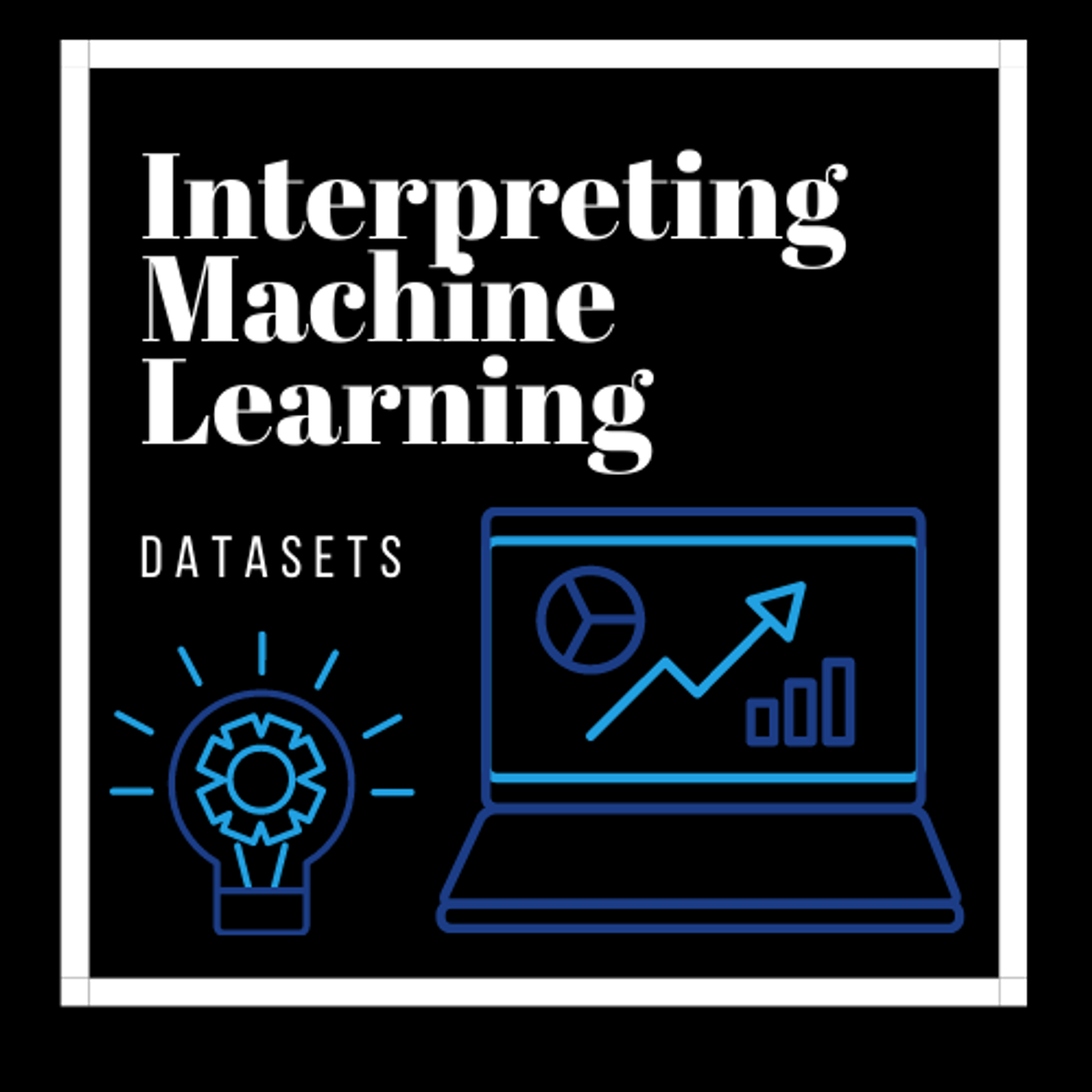
Interpreting Machine Learning datasets
In this 2-hour long project-based course, you will learn how to interpret the dataset for machine learning, how different features impact on a mode and how to evaluate them.
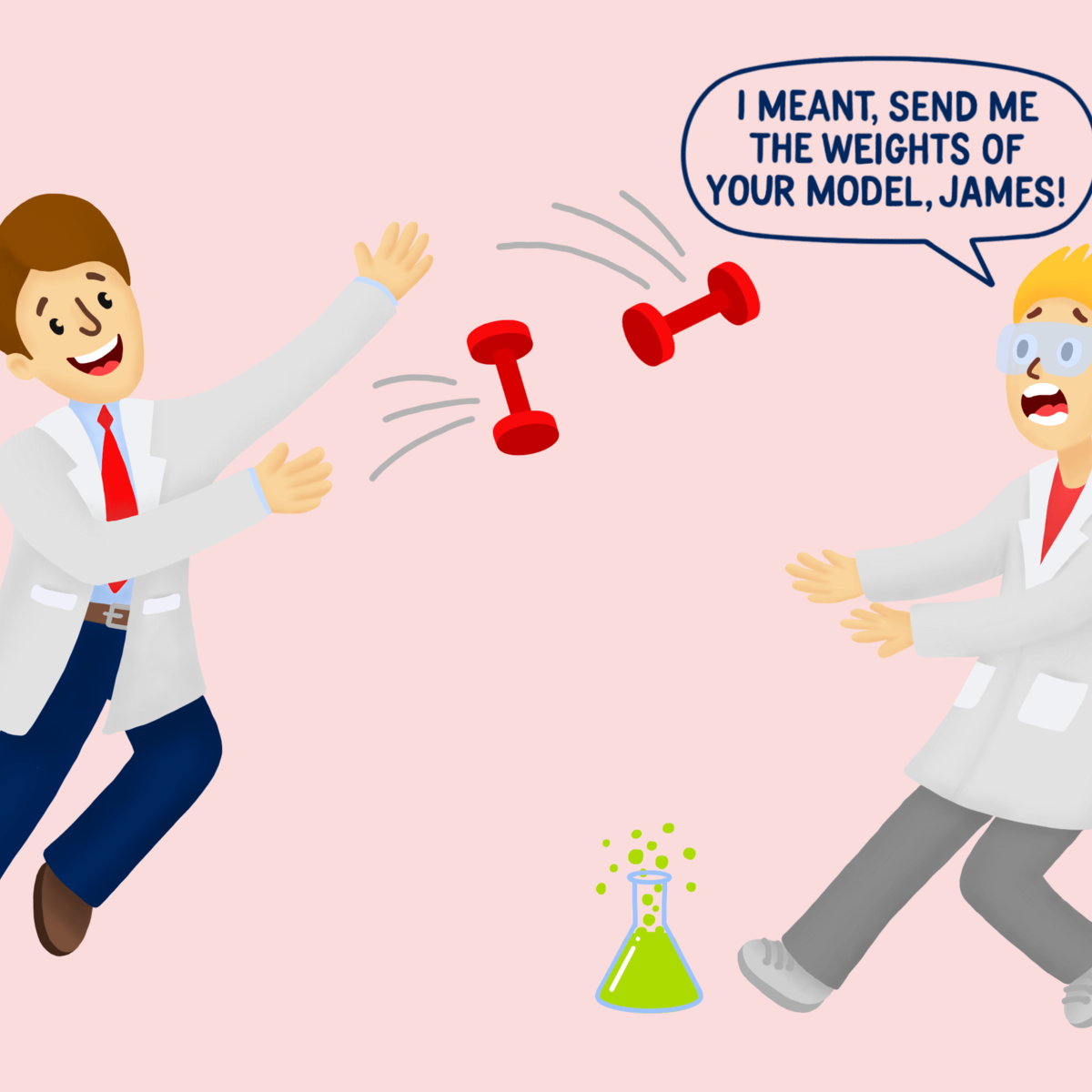
Save, Load and Export Models with Keras
In this 1 hour long project based course, you will learn to save, load and restore models with Keras. In Keras, we can save just the model weights, or we can save weights along with the entire model architecture. We can also export the models to TensorFlow's Saved Mode format which is very useful when serving a model in production, and we can load models from the Saved Model format back in Keras as well.
In order to be successful in this project, you should be familiar with python programming, and basics of neural networks.
Note: This course works best for learners who are based in the North America region. We’re currently working on providing the same experience in other regions.
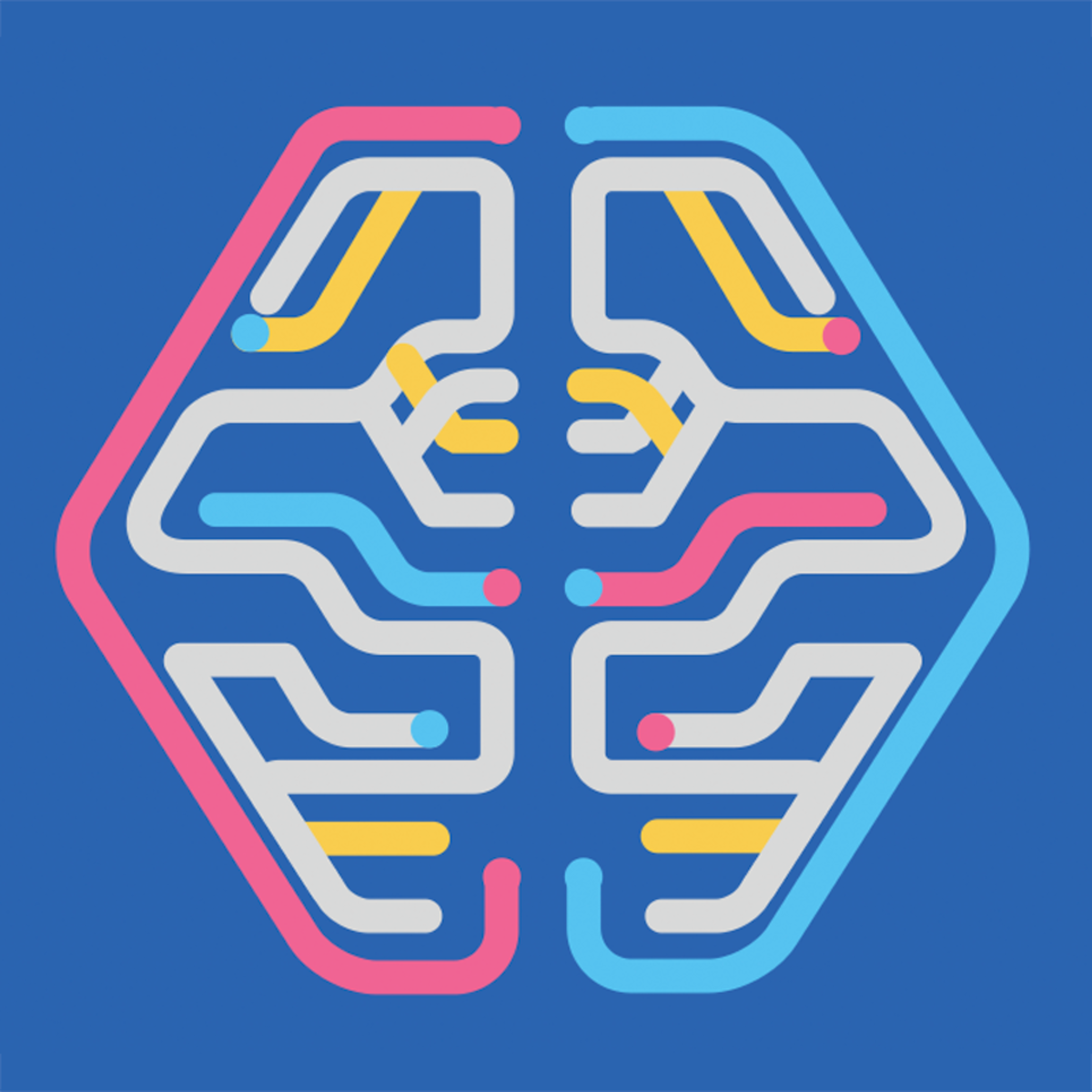
TensorFlow on Google Cloud
This course covers designing and building a TensorFlow input data pipeline, building ML models with TensorFlow and Keras, improving the accuracy of ML models, writing ML models for scaled use, and writing specialized ML models.
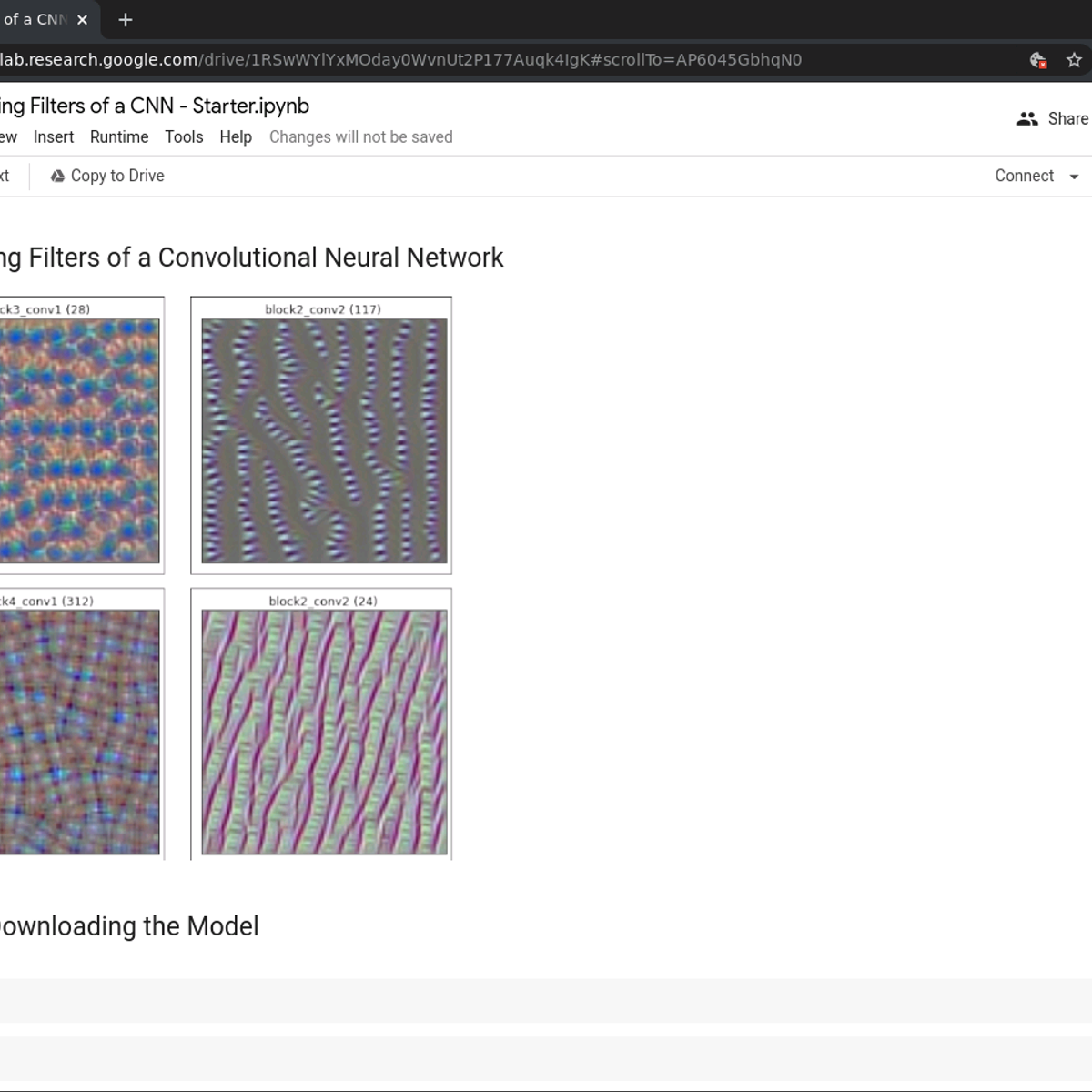
Visualizing Filters of a CNN using TensorFlow
In this short, 1 hour long guided project, we will use a Convolutional Neural Network - the popular VGG16 model, and we will visualize various filters from different layers of the CNN. We will do this by using gradient ascent to visualize images that maximally activate specific filters from different layers of the model.
We will be using TensorFlow as our machine learning framework. The project uses the Google Colab environment which is a fantastic tool for creating and running Jupyter Notebooks in the cloud, and Colab even provides free GPUs for your notebooks.
You will need prior programming experience in Python. This is a practical, hands on guided project for learners who already have theoretical understanding of Neural Networks, Convolutional Neural Networks, and optimization algorithms like gradient descent but want to understand how to use the TensorFlow to visualize various filters of a CNN.
Note: This course works best for learners who are based in the North America region. We’re currently working on providing the same experience in other regions.
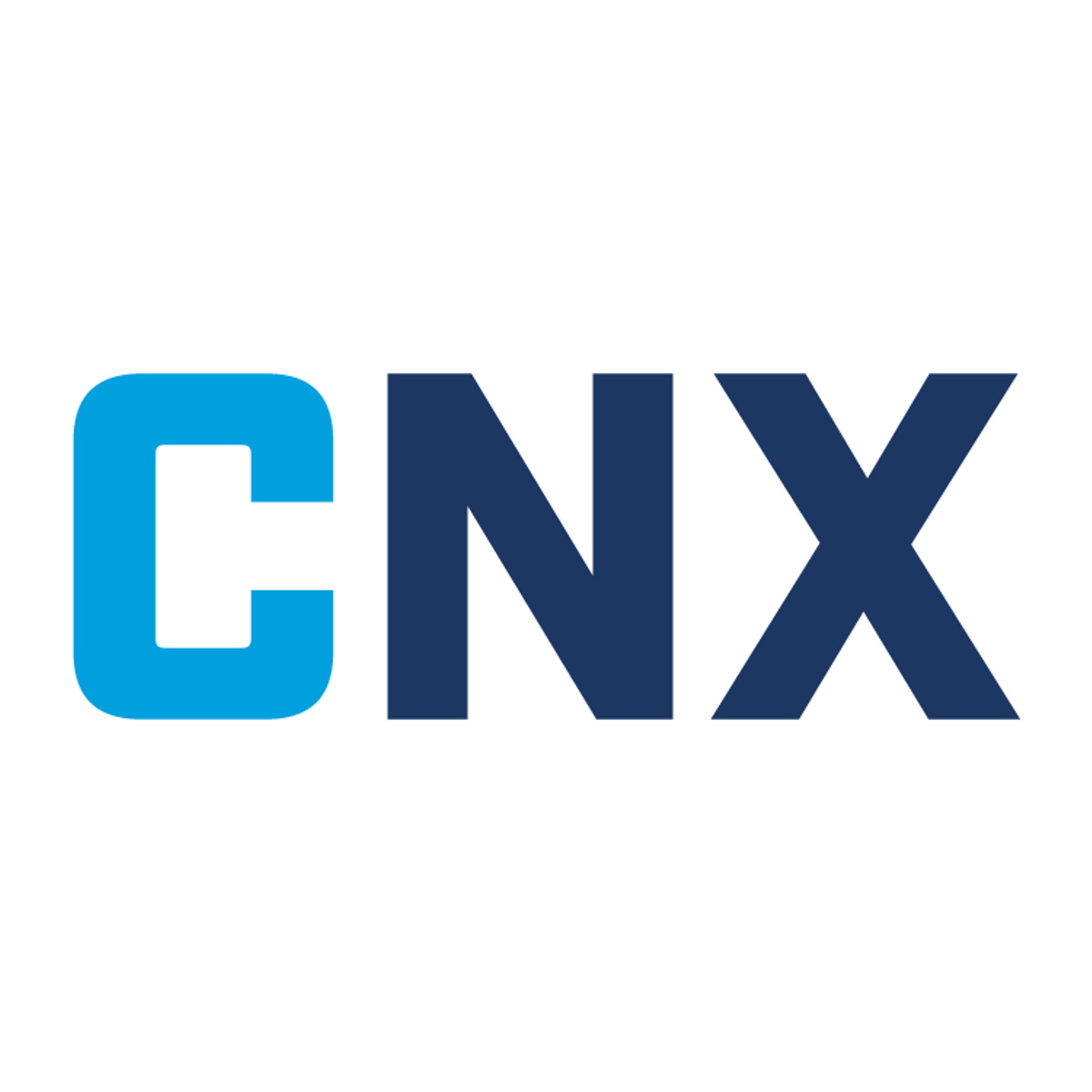
Preparing for Your CertNexus Certification Exam
What is a certification? How is it different than a certificate or credential? This mini-course will answer these questions and provide learners direction on how to prepare for a certification exam from CertNexus or an other certification vendor. It includes tips and tricks to succeed in your journey towards certification, as well as step by step instructions how to schedule and take your exam, whether in person or online. In addition we will provide next steps after your certification, including posting your badge to social posts and your organization.
Candidates with industry recognized certifications can earn up to 25% more than candidates without a certification. Learn how to successfully prepare for, pass, and share your certification.
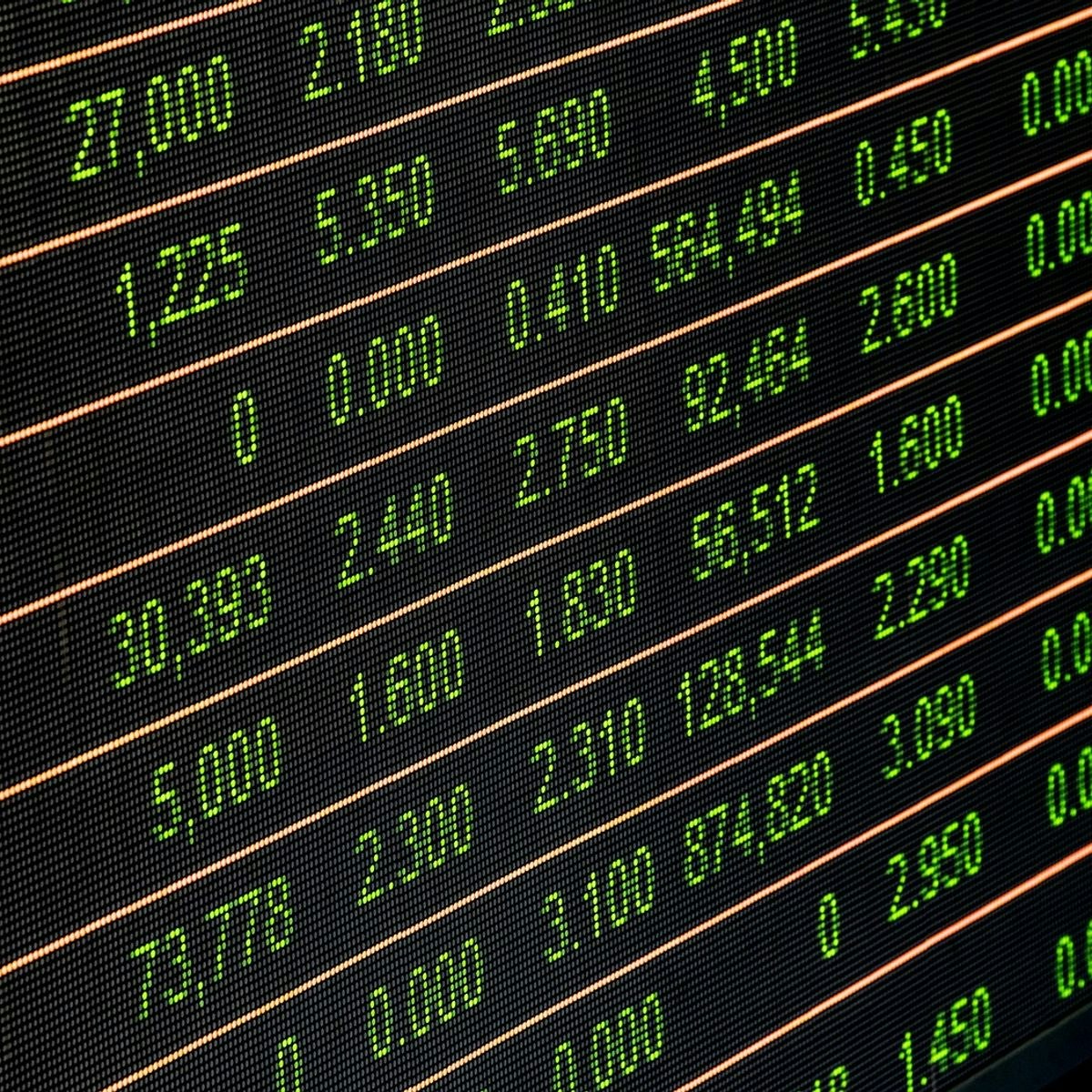
Linear Regression and Multiple Linear Regression in Julia
This guided project is for those who want to learn how to use Julia for linear regression and multiple linear regression. You will learn what linear regression is, how to build linear regression models in Julia and how to test the performance of your model.
While you are watching me code, you will get a cloud desktop with all the required software pre-installed. This will allow you to code along with me. After all, we learn best with active, hands-on learning.
Special Features:
1) Work with real-world stock market data.
2) Best practices and tips are provided.
3) You get a copy of the jupyter notebook that you create which acts as a handy reference guide.
Please note that the version of Julia used is 1.0.4
Note: This project works best for learners who are based in the North America region. We’re currently working on providing the same experience in other regions.
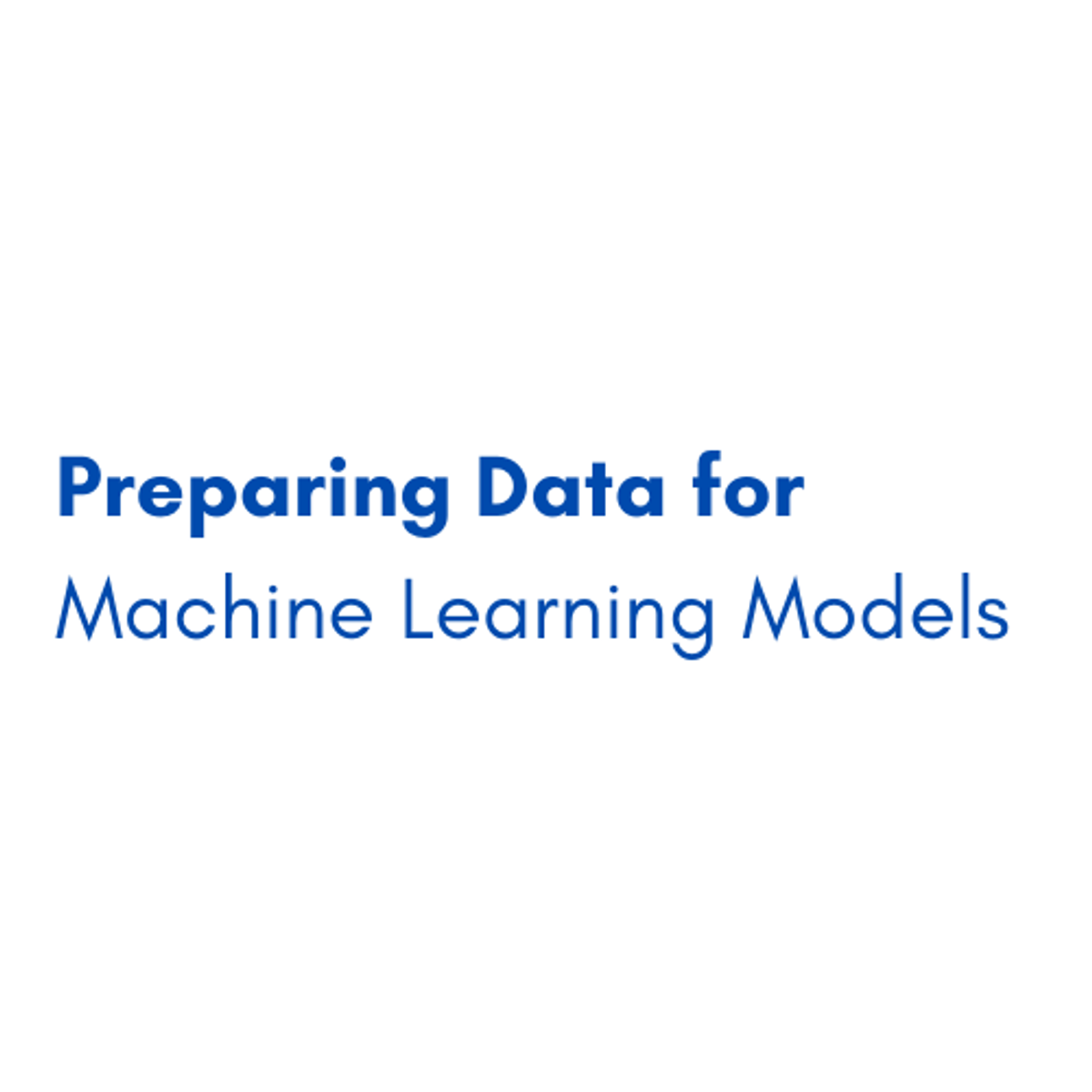
Preparing Data for Machine Learning Models
By the end of this project, you will extract colors pixels as training dataset into a form where you can feed it to your Machine Learning Model using numpy arrays.
In this project we will work with images, you will get introduced to computer vision basic concepts.
Moreover, you will be able to properly handle arrays and preprocess your training dataset and label it.
Extracting features and preparing data is a very crucial task as it influences your model.
So you will start to learn the basics of handling the data into the format where it would be accepted by a Machine Learning algorithm as Training Dataset.
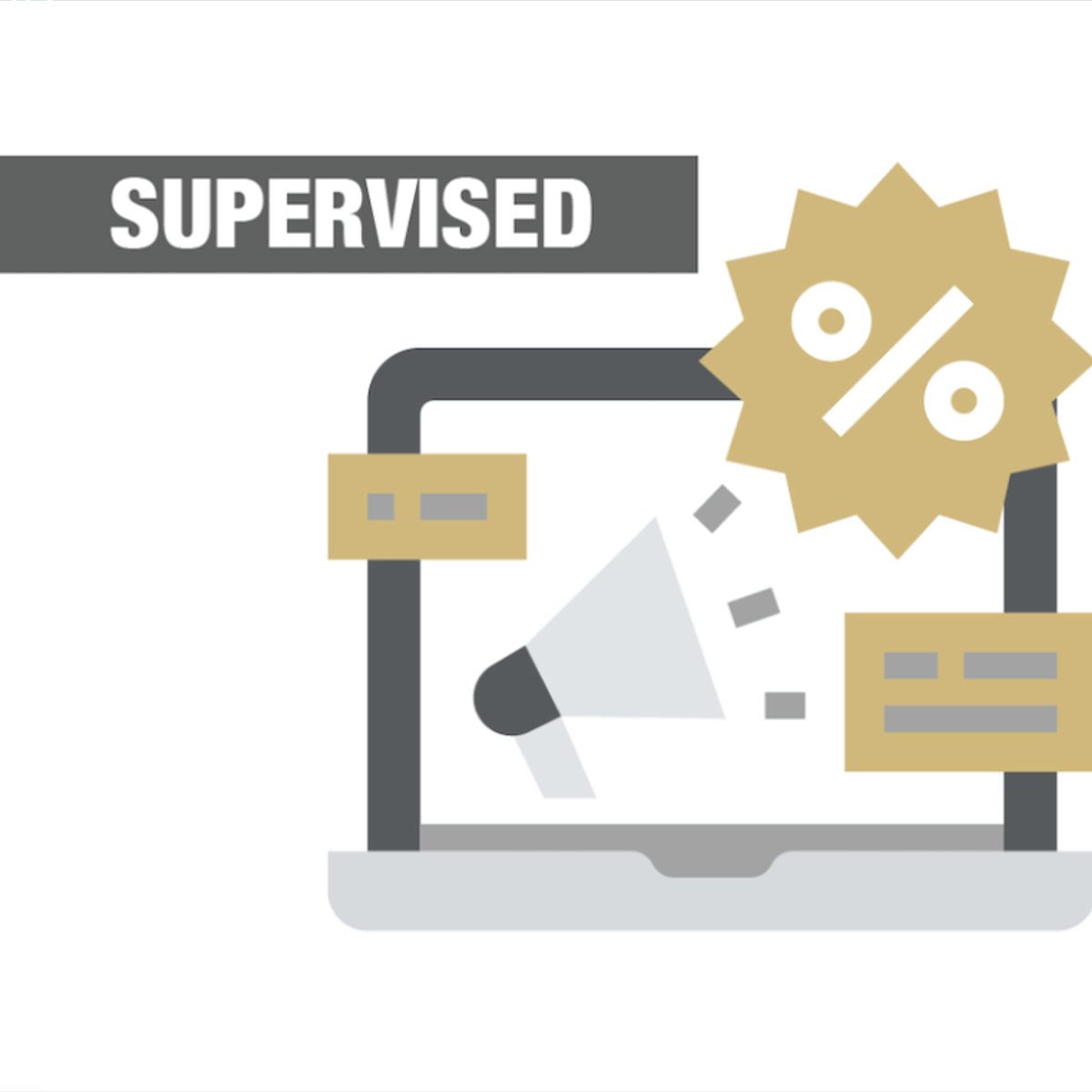
Supervised Text Classification for Marketing Analytics
Marketing data often requires categorization or labeling. In today’s age, marketing data can also be very big, or larger than what humans can reasonably tackle. In this course, students learn how to use supervised deep learning to train algorithms to tackle text classification tasks. Students walk through a conceptual overview of supervised machine learning and dive into real-world datasets through instructor-led tutorials in Python. The course concludes with a major project.
This course can be taken for academic credit as part of CU Boulder’s Master of Science in Data Science (MS-DS) degree offered on the Coursera platform. The MS-DS is an interdisciplinary degree that brings together faculty from CU Boulder’s departments of Applied Mathematics, Computer Science, Information Science, and others. With performance-based admissions and no application process, the MS-DS is ideal for individuals with a broad range of undergraduate education and/or professional experience in computer science, information science, mathematics, and statistics. Learn more about the MS-DS program at https://www.coursera.org/degrees/master-of-science-data-science-boulder.
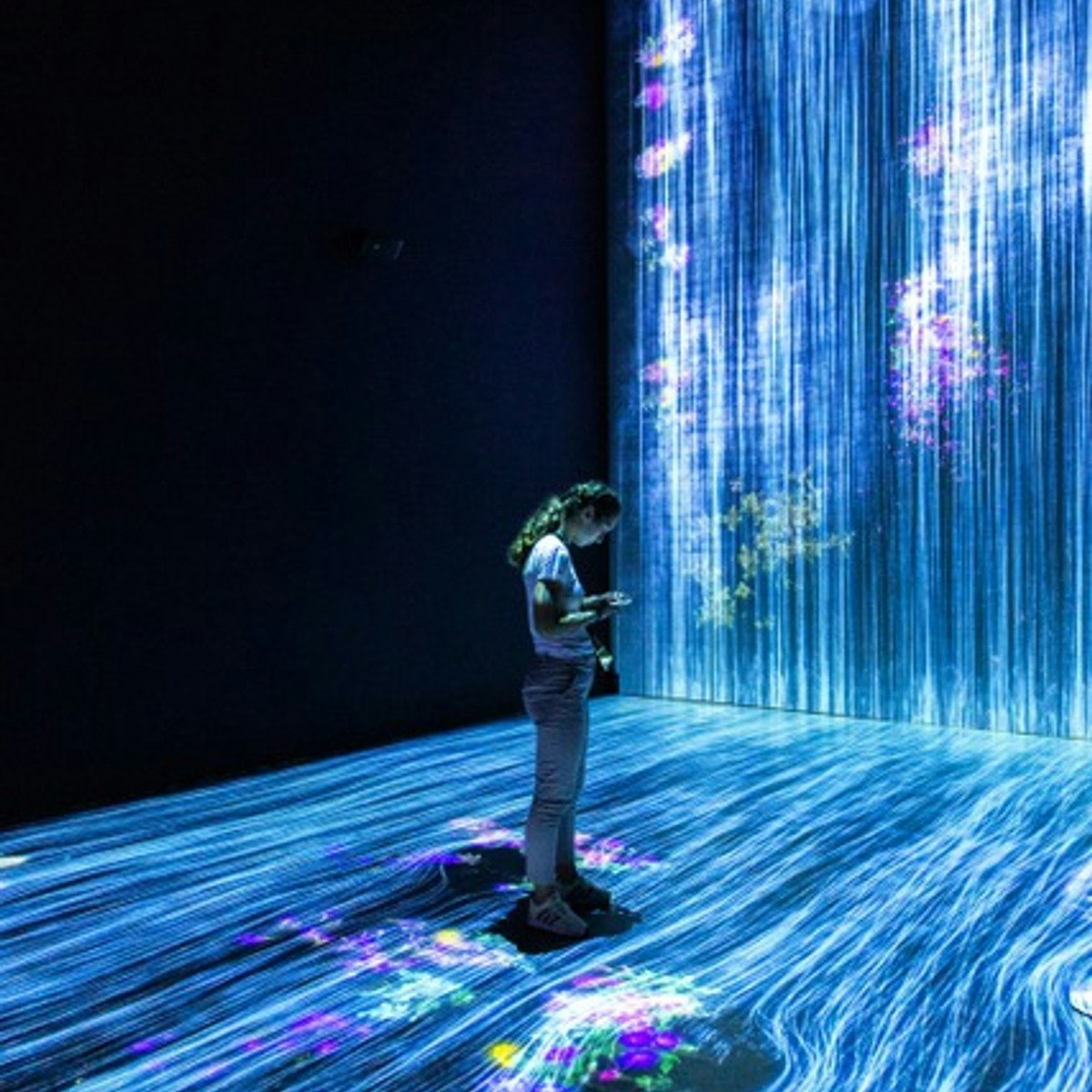
Introduction to Machine Learning: Supervised Learning
In this course, you’ll be learning various supervised ML algorithms and prediction tasks applied to different data. You’ll learn when to use which model and why, and how to improve the model performances. We will cover models such as linear and logistic regression, KNN, Decision trees and ensembling methods such as Random Forest and Boosting, kernel methods such as SVM.
Prior coding or scripting knowledge is required. We will be utilizing Python extensively throughout the course. In this course, you will need to have a solid foundation in Python or sufficient previous experience coding with other programming languages to pick up Python quickly.
We will be learning how to use data science libraries like NumPy, pandas, matplotlib, statsmodels, and sklearn. The course is designed for programmers beginning to work with those libraries. Prior experience with those libraries would be helpful but not necessary.
College-level math skills, including Calculus and Linear Algebra, are required. Our hope for this course is that the math will be understandable but not intimidating.
This course can be taken for academic credit as part of CU Boulder’s Master of Science in Data Science (MS-DS) degree offered on the Coursera platform. The MS-DS is an interdisciplinary degree that brings together faculty from CU Boulder’s departments of Applied Mathematics, Computer Science, Information Science, and others. With performance-based admissions and no application process, the MS-DS is ideal for individuals with a broad range of undergraduate education and/or professional experience in computer science, information science, mathematics, and statistics. Learn more about the MS-DS program at https://www.coursera.org/degrees/master-of-science-data-science-boulder.
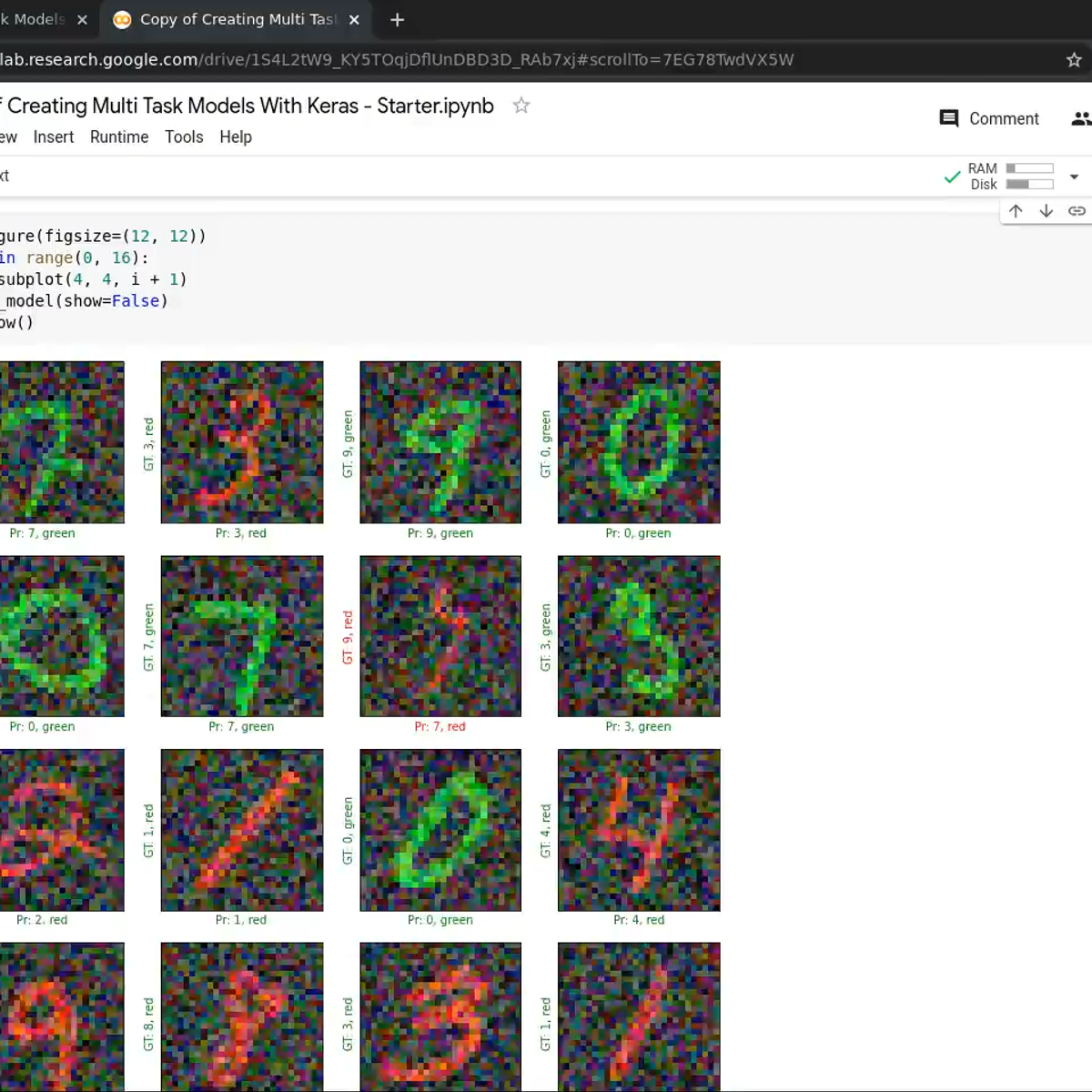
Creating Multi Task Models With Keras
In this 1 hour long guided project, you will learn to create and train multi-task, multi-output models with Keras. You will learn to use Keras' functional API to create a multi output model which will be trained to learn two different labels given the same input example. The model will have one input but two outputs. A few of the shallow layers will be shared between the two outputs, you will also use a ResNet style skip connection in the model. If you are familiar with Keras, you have probably come across examples of models that are trained to perform multiple tasks. For example, an object detection model where a CNN is trained to find all class instances in the input images as well as give a regression output to localize the detected class instances in the input. Being able to use Keras' functional API is a first step towards building complex, multi-output models like object detection models.
We will be using TensorFlow as our machine learning framework. The project uses the Google Colab environment. You will need prior programming experience in Python. You will also need prior experience with Keras. Consider this to be an intermediate level Keras project. This is a practical, hands on guided project for learners who already have theoretical understanding of Neural Networks, Convolutional Neural Networks, and optimization algorithms like gradient descent but want to understand how to use use Keras to write custom, more complex models than just plain sequential neural networks.
Note: This course works best for learners who are based in the North America region. We’re currently working on providing the same experience in other regions.
Popular Internships and Jobs by Categories
Find Jobs & Internships
Browse
© 2024 BoostGrad | All rights reserved