Back to Courses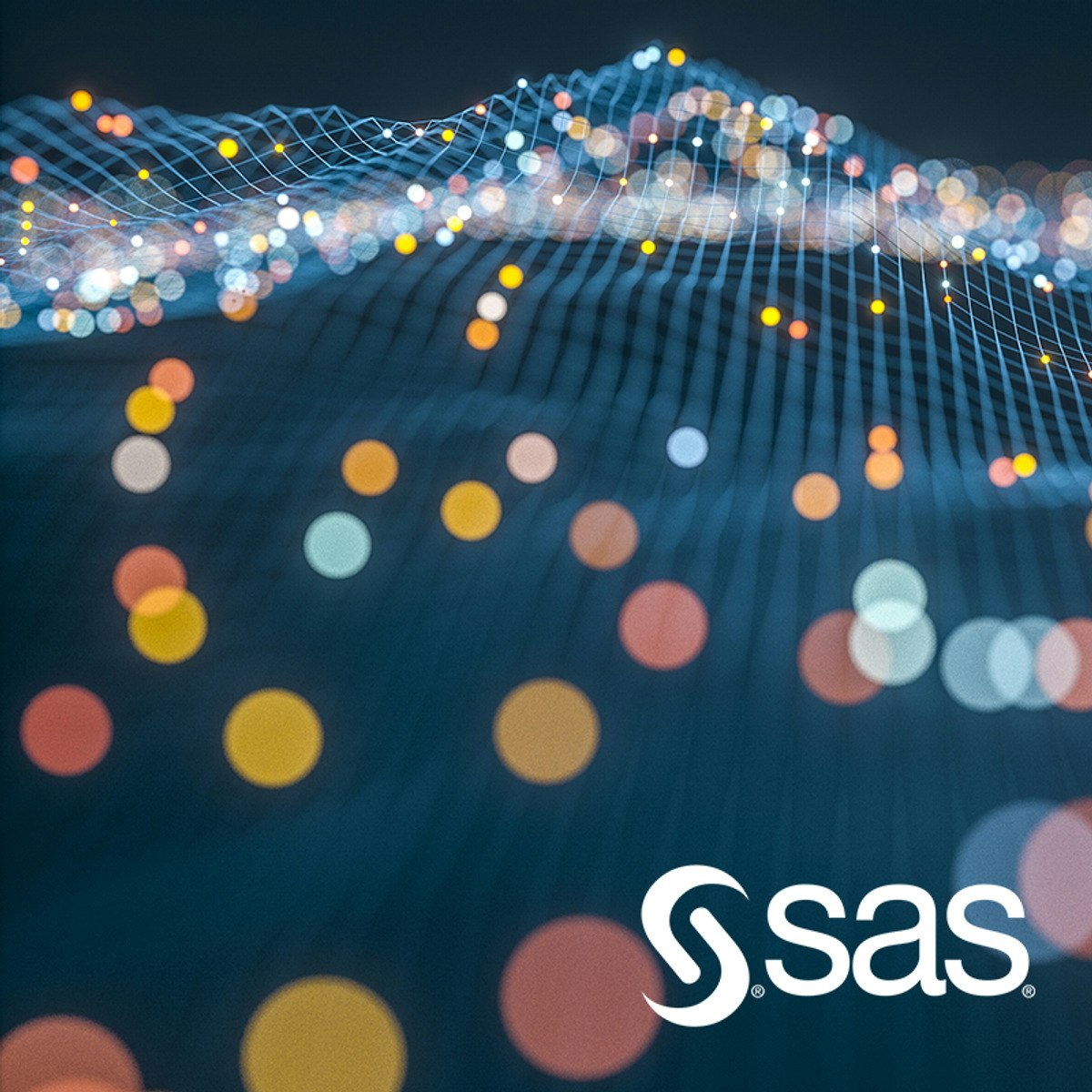
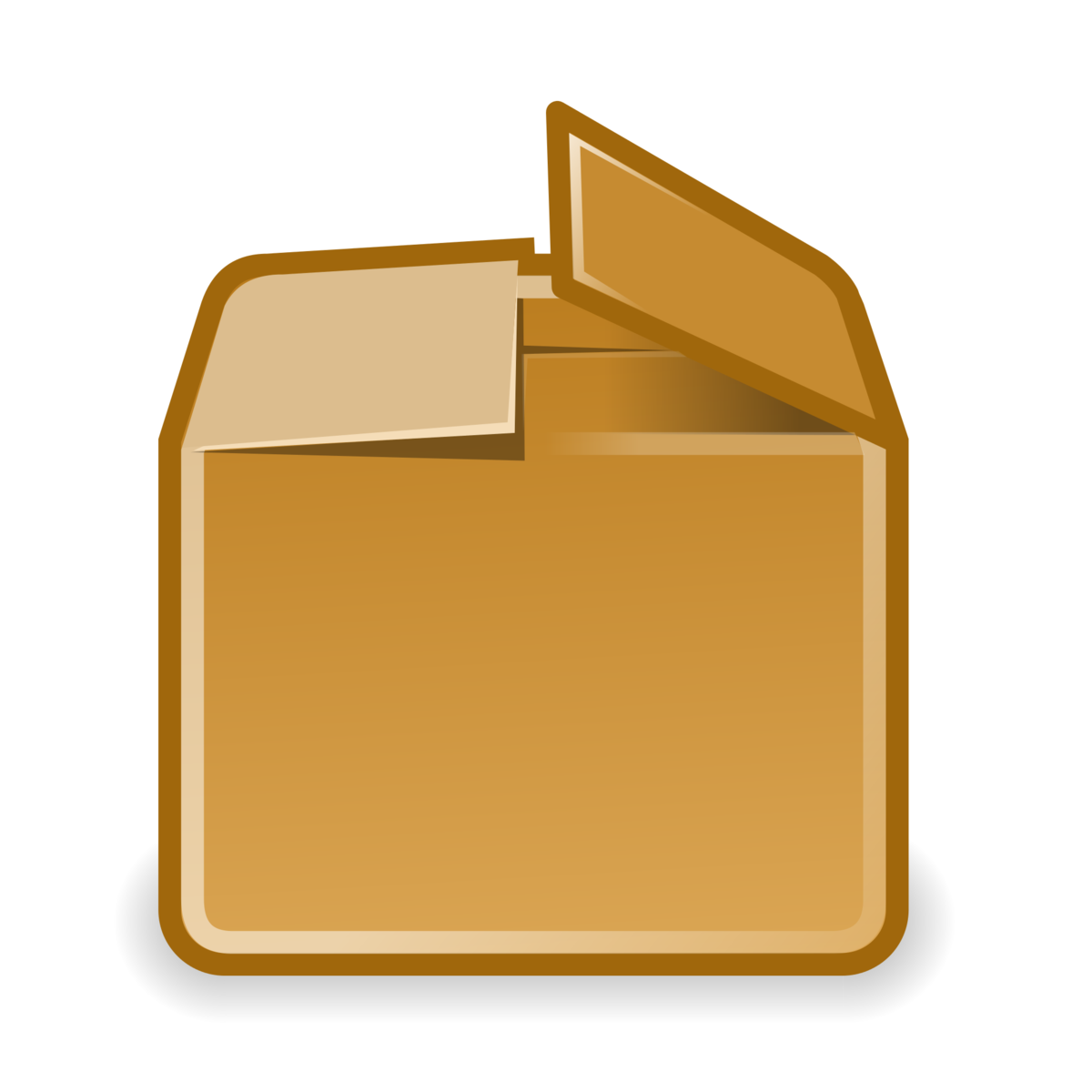
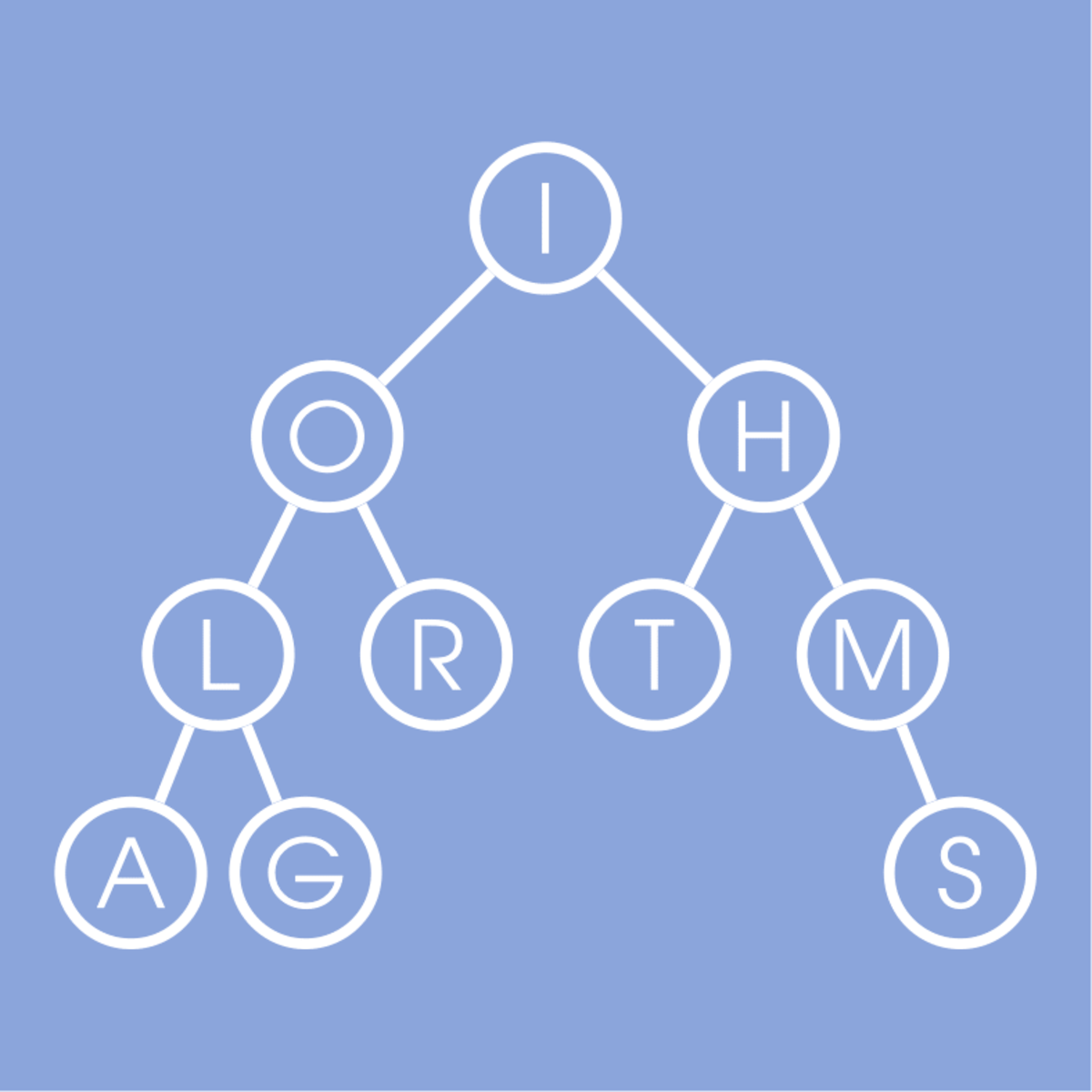
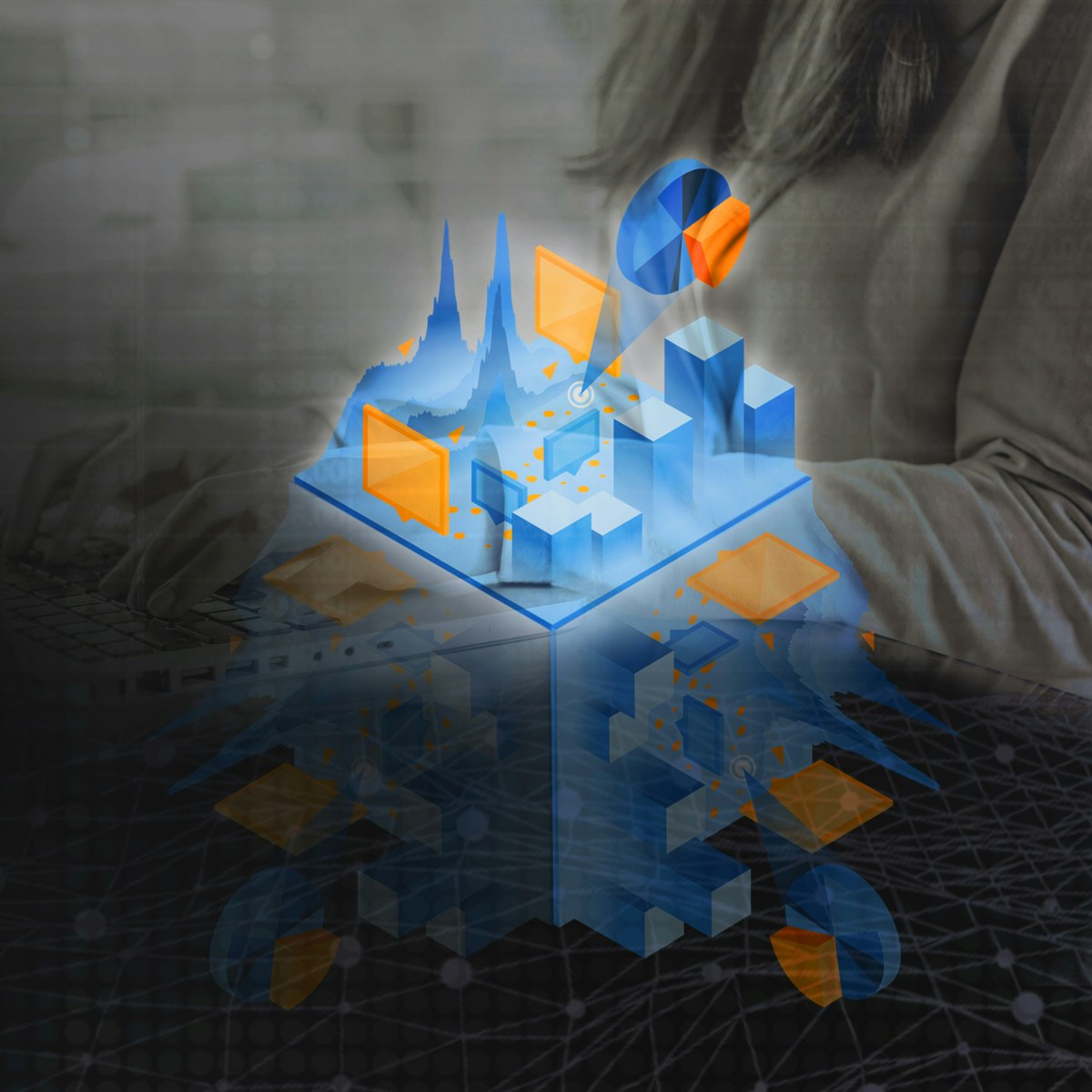
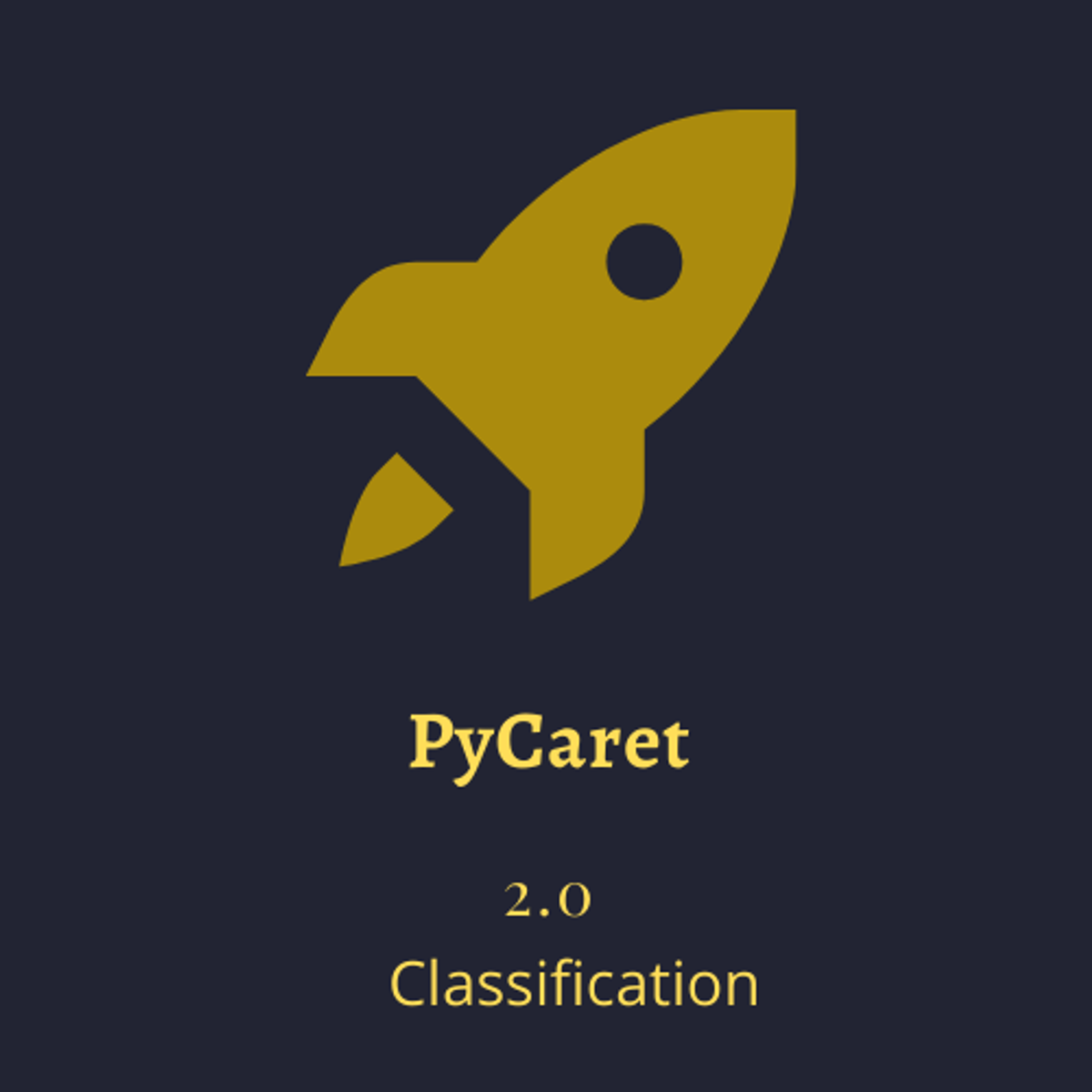
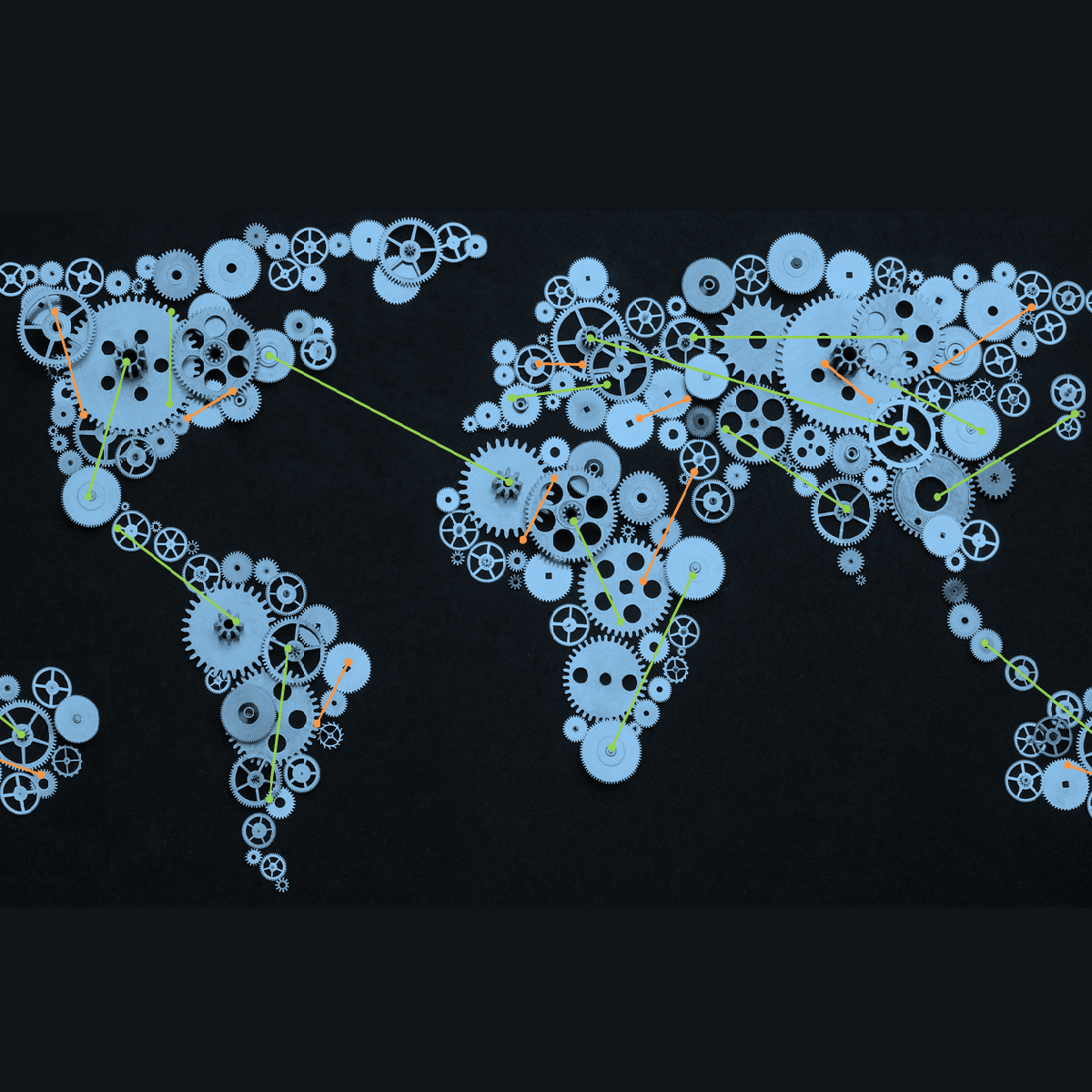
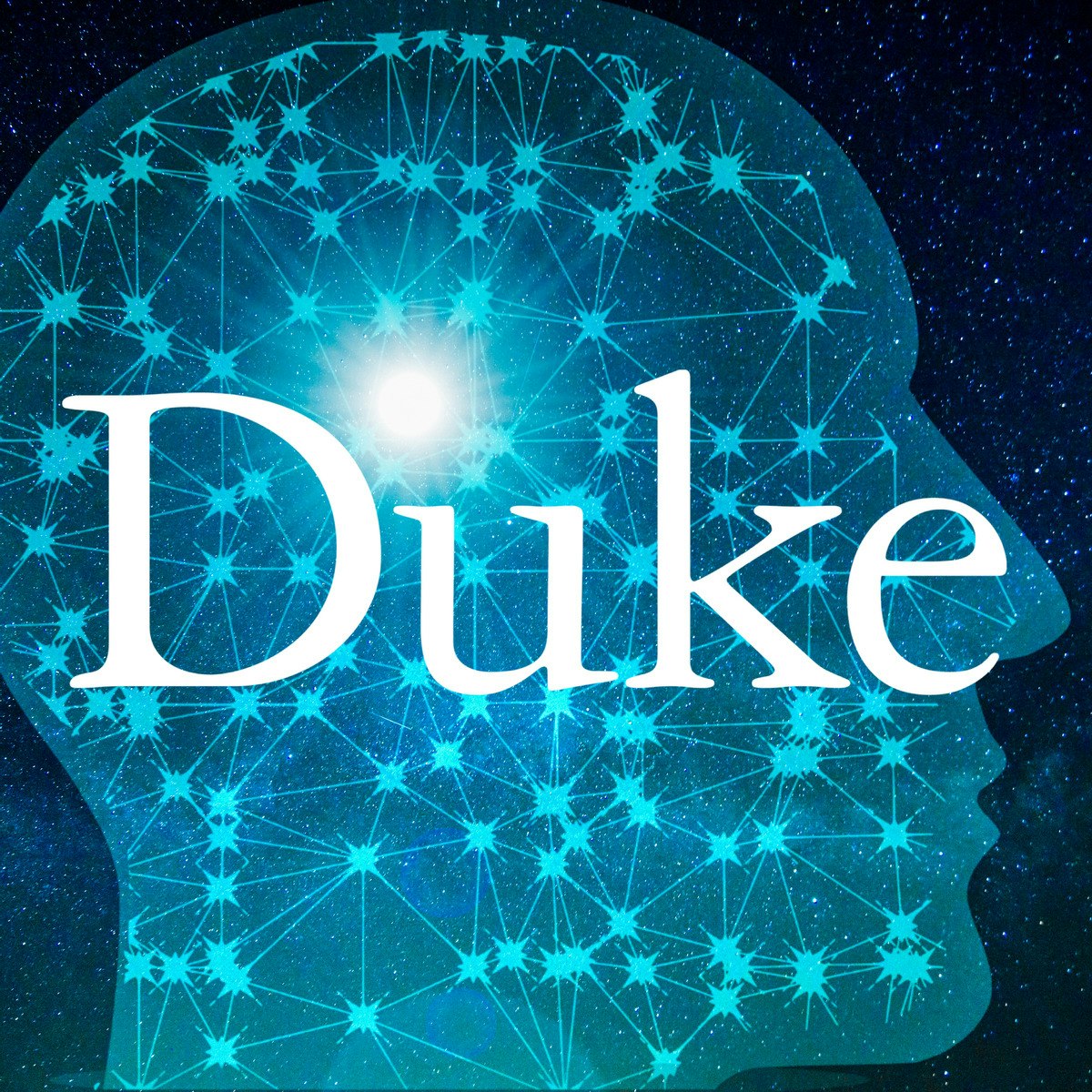
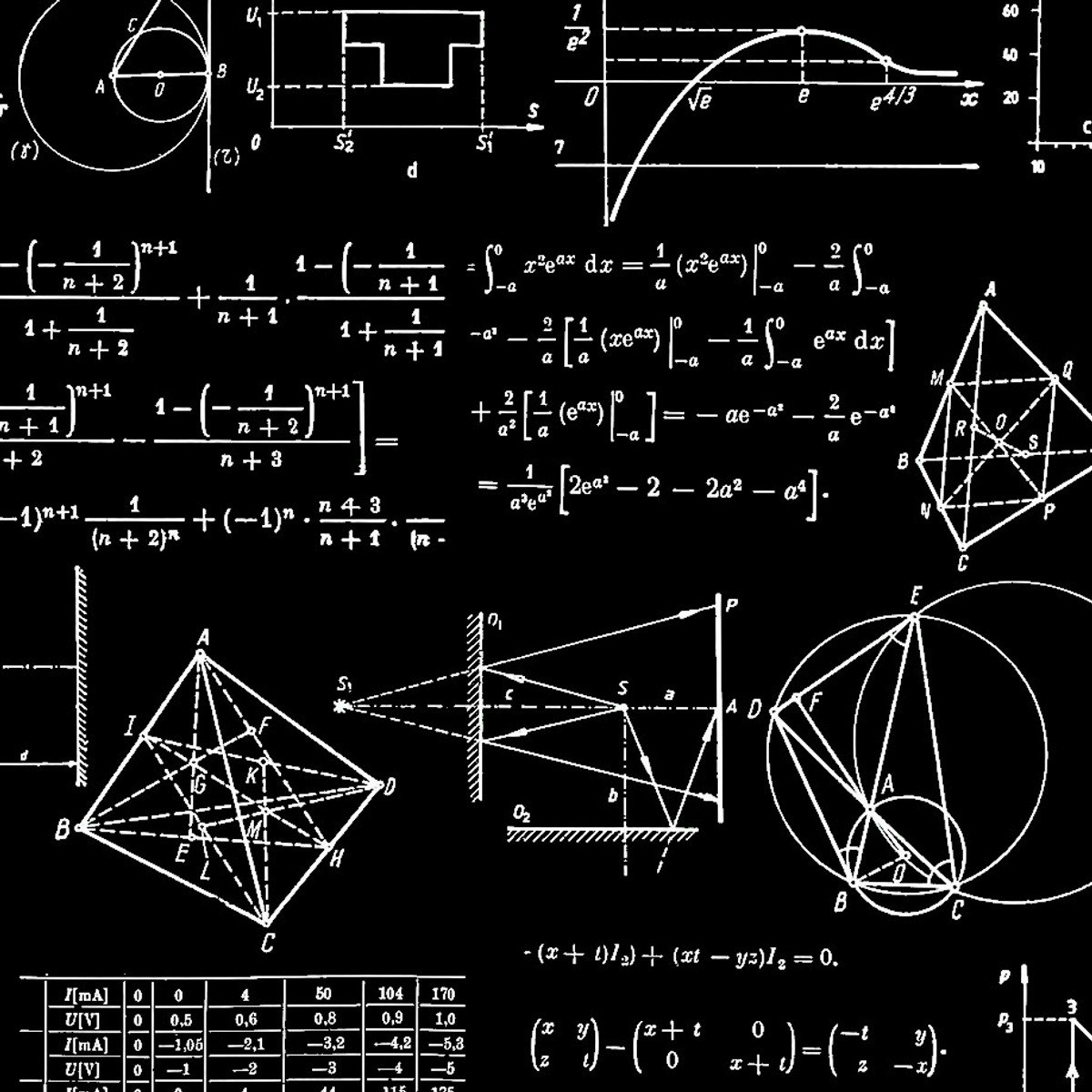
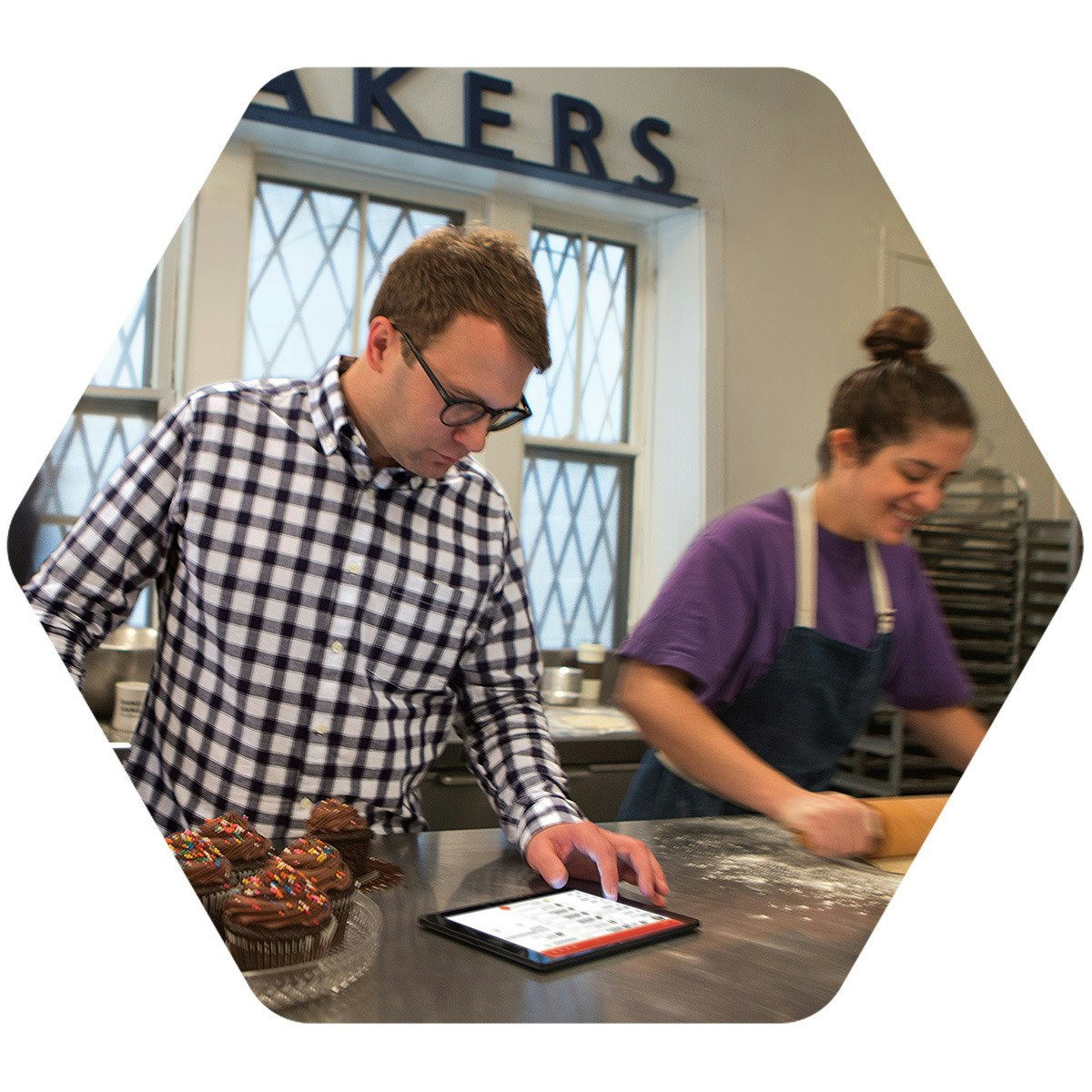
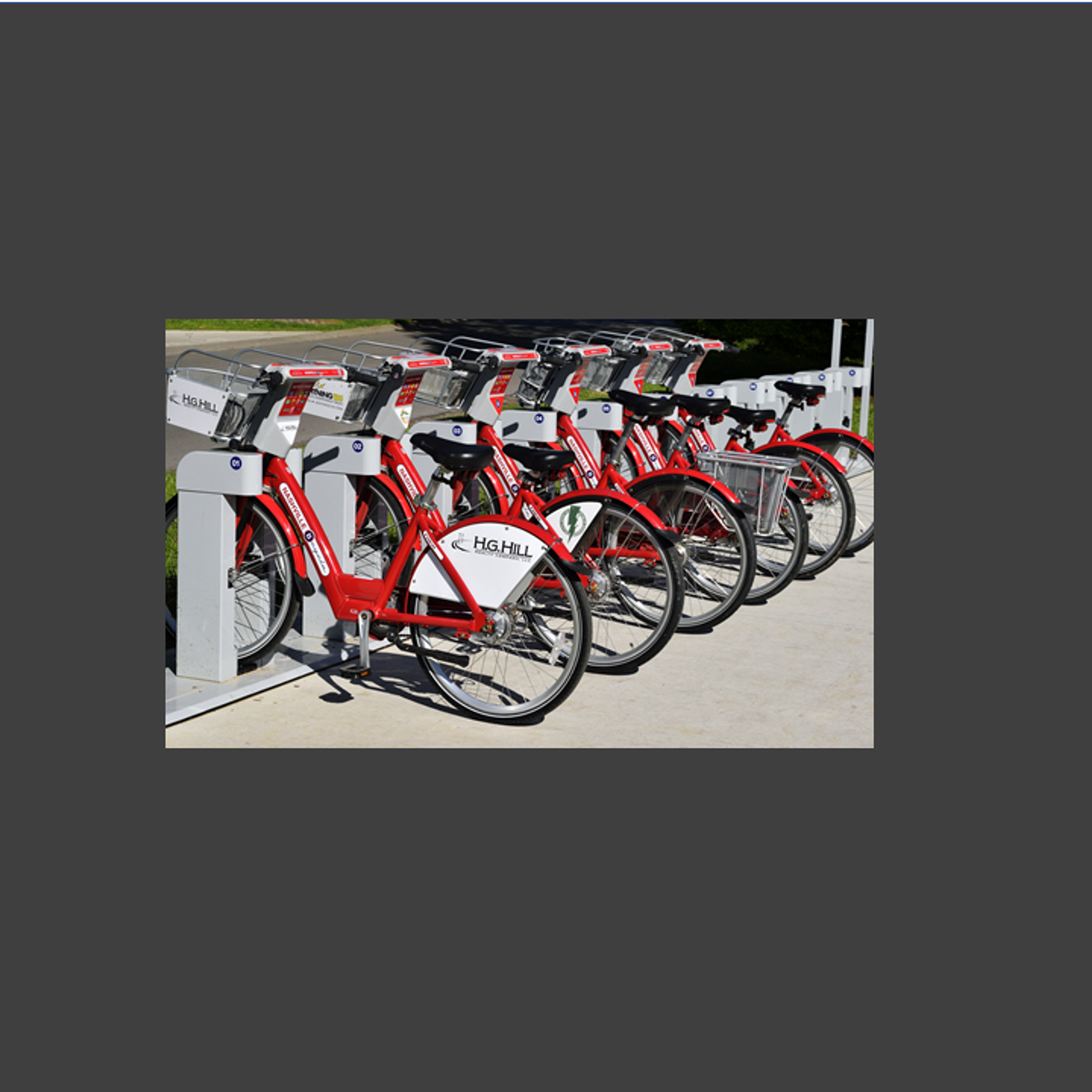
Data Science Courses - Page 93
Showing results 921-930 of 1407
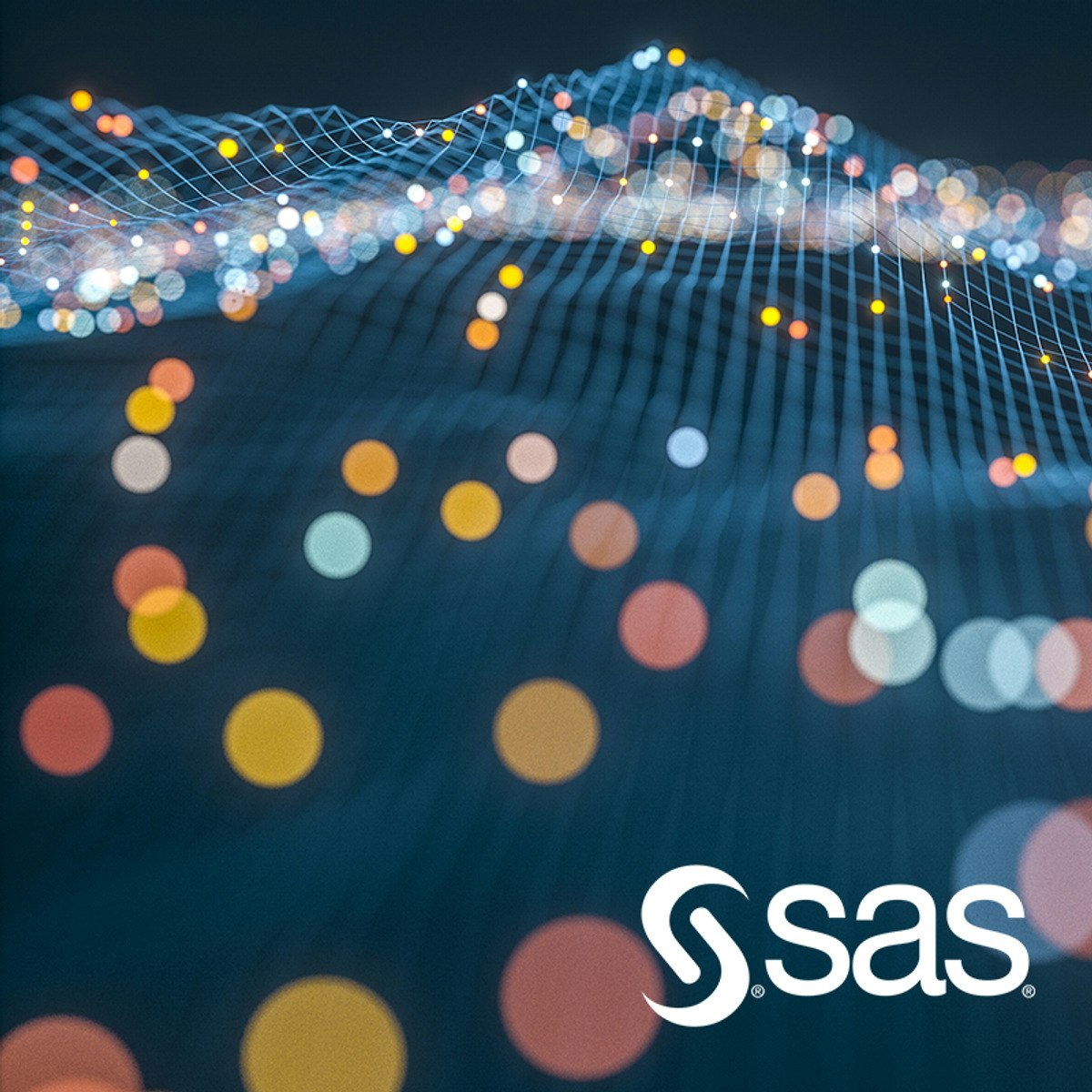
Building a Large-Scale, Automated Forecasting System
In this course you learn to develop and maintain a large-scale forecasting project using SAS Visual Forecasting tools. Emphasis is initially on selecting appropriate methods for data creation and variable transformations, model generation, and model selection. Then you learn how to improve overall baseline forecasting performance by modifying default processes in the system.
This course is appropriate for analysts interested in augmenting their machine learning skills with analysis tools that are appropriate for assaying, modifying, modeling, forecasting, and managing data that consist of variables that are collected over time. The courses is primarily syntax based, so analysts taking this course need some familiarity with coding. Experience with an object-oriented language is helpful, as is familiarity with manipulating large tables.
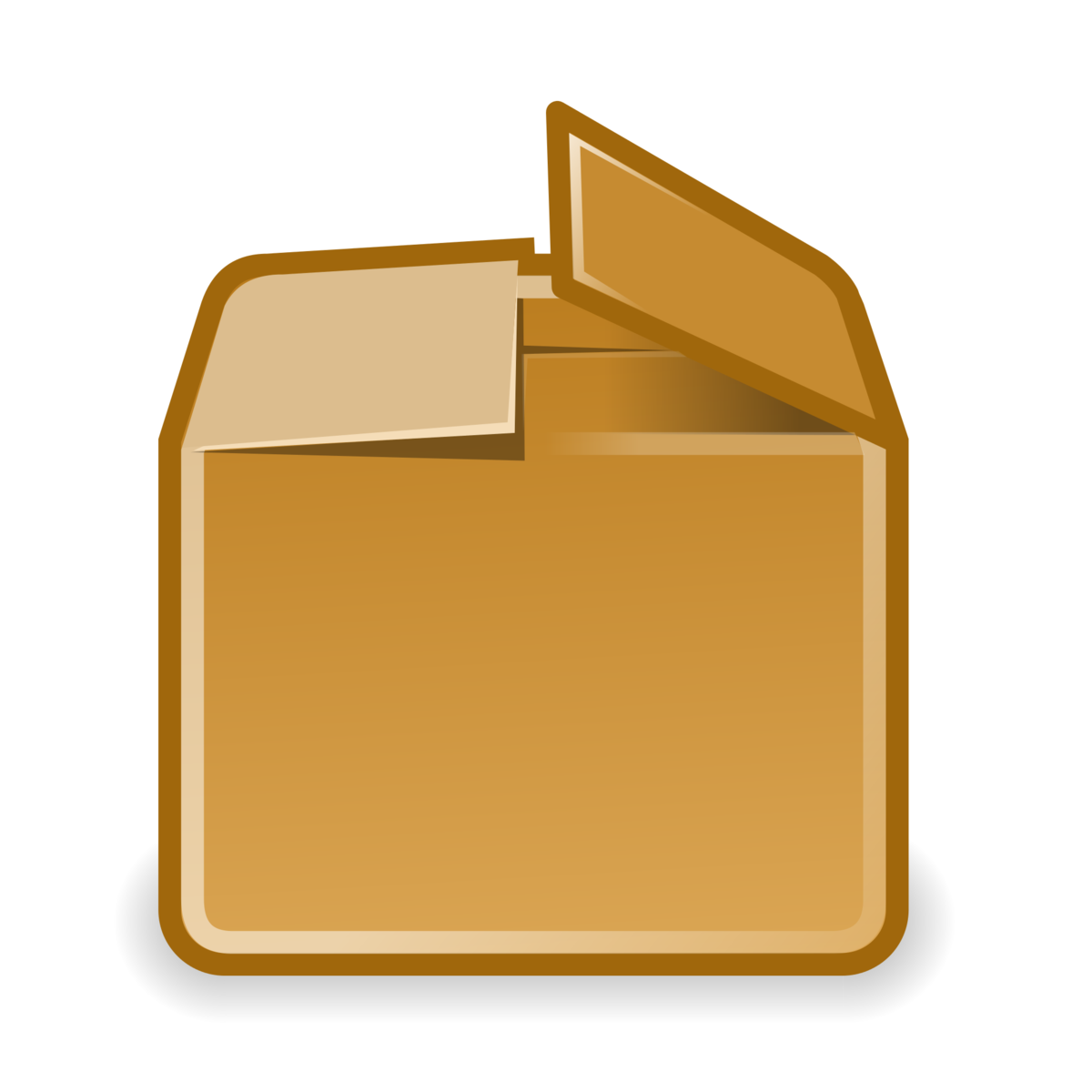
Building R Packages
Writing good code for data science is only part of the job. In order to maximizing the usefulness and reusability of data science software, code must be organized and distributed in a manner that adheres to community-based standards and provides a good user experience. This course covers the primary means by which R software is organized and distributed to others. We cover R package development, writing good documentation and vignettes, writing robust software, cross-platform development, continuous integration tools, and distributing packages via CRAN and GitHub. Learners will produce R packages that satisfy the criteria for submission to CRAN.
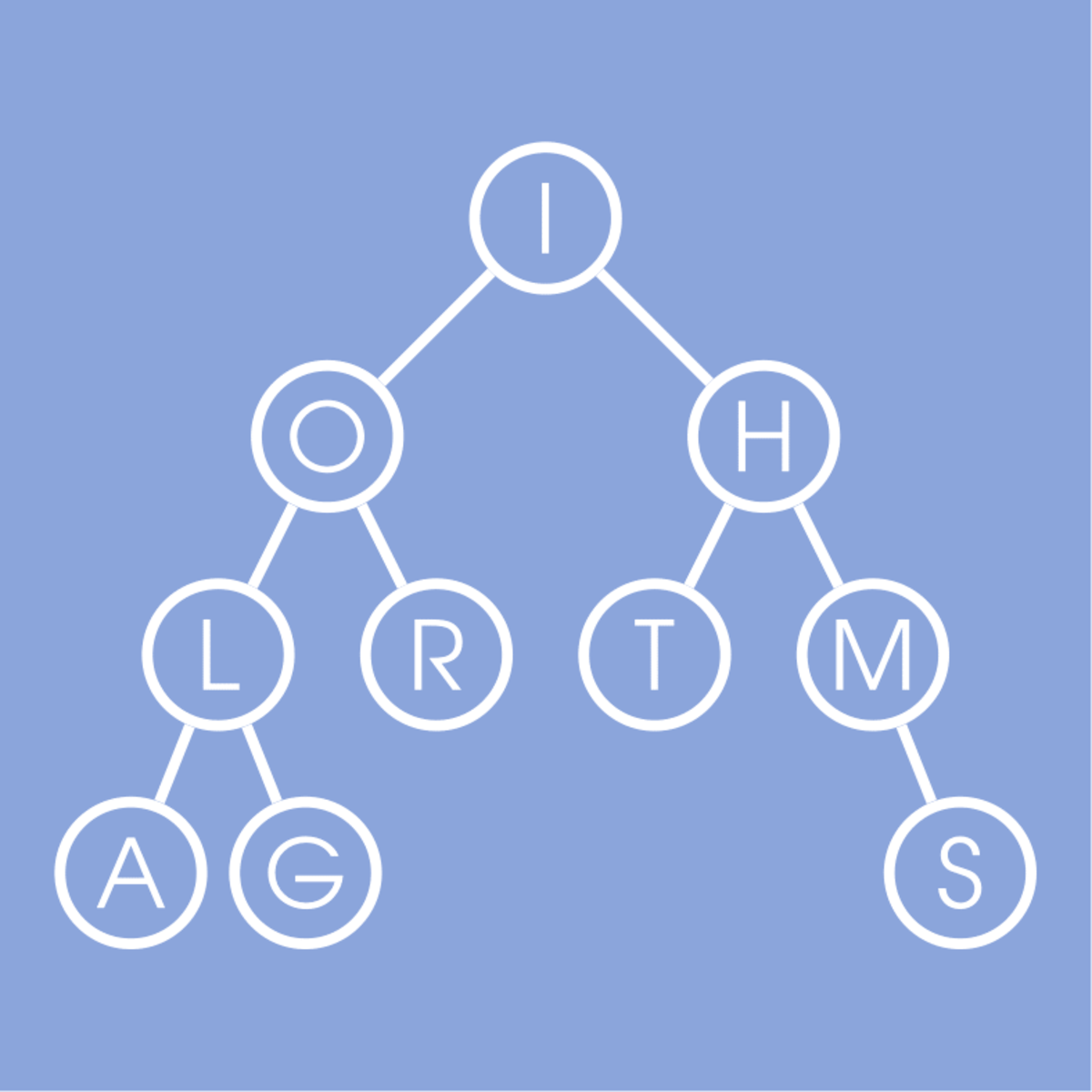
Data Structures
A good algorithm usually comes together with a set of good data structures that allow the algorithm to manipulate the data efficiently. In this online course, we consider the common data structures that are used in various computational problems. You will learn how these data structures are implemented in different programming languages and will practice implementing them in our programming assignments. This will help you to understand what is going on inside a particular built-in implementation of a data structure and what to expect from it. You will also learn typical use cases for these data structures.
A few examples of questions that we are going to cover in this class are the following:
1. What is a good strategy of resizing a dynamic array?
2. How priority queues are implemented in C++, Java, and Python?
3. How to implement a hash table so that the amortized running time of all operations is O(1) on average?
4. What are good strategies to keep a binary tree balanced?
You will also learn how services like Dropbox manage to upload some large files instantly and to save a lot of storage space!
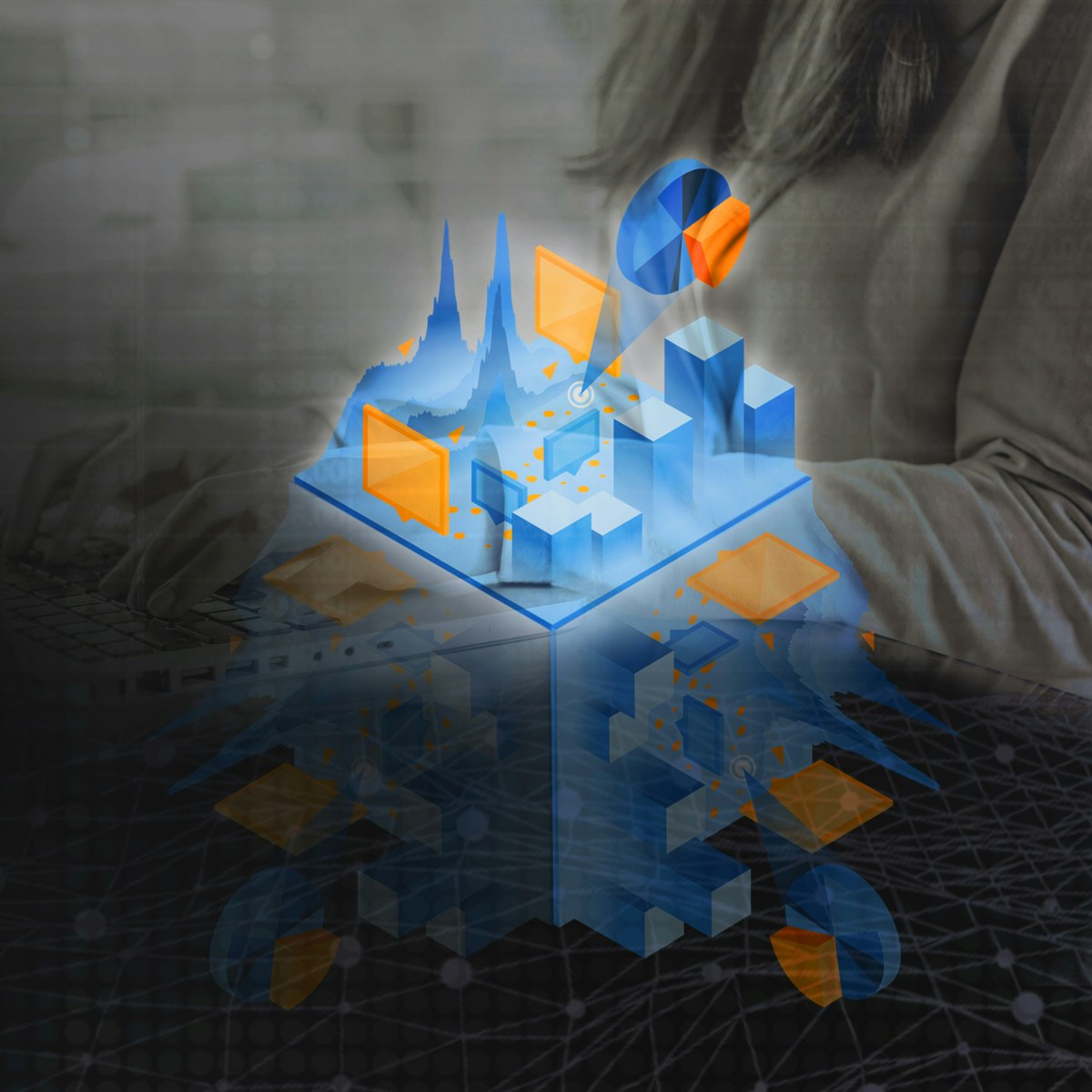
Excel Skills for Business: Advanced
Spreadsheet software remains one of the most ubiquitous pieces of software used in workplaces around the world. Learning to confidently operate this software means adding a highly valuable asset to your employability portfolio. Across the globe, millions of job advertisements requiring Excel skills are posted every day. At a time when digital skills jobs are growing much faster than non-digital jobs, completing this course will position you ahead of others, so keep reading.
In this last course of our Specialization Excel Skills for Business you will build on the strong foundations of the first three courses: Essentials, Intermediate I + II. In the Advanced course, we will prepare you to become a power user of Excel - this is your last step before specializing at a professional level. The topics we have prepared will challenge you as you learn how to use advanced formula techniques and sophisticated lookups. You will clean and prepare data for analysis, and learn how to work with dates and financial functions. An in-depth look at spreadsheet design and documentation will prepare you for our big finale, where you will learn how to build professional dashboards in Excel.
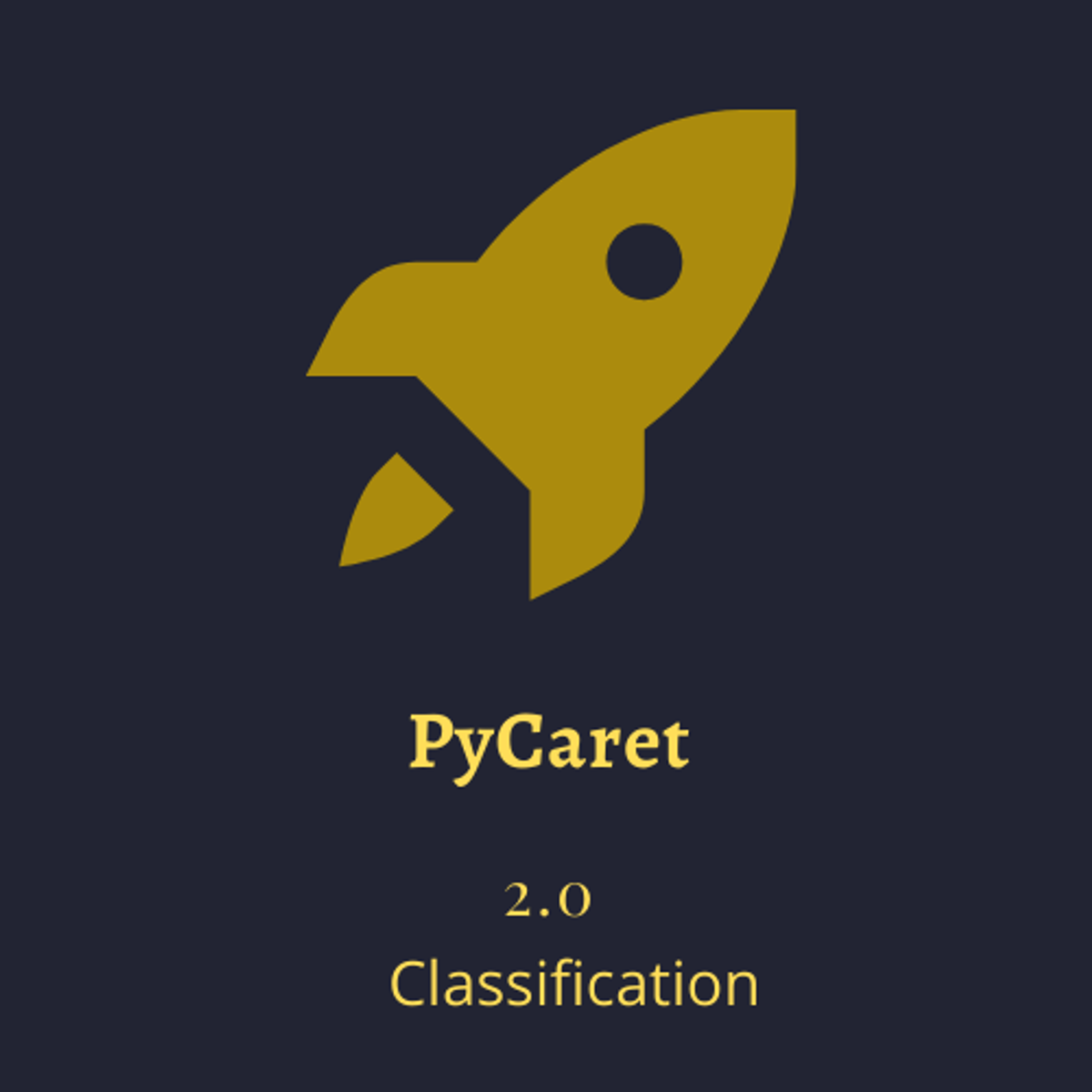
PyCaret: Anatomy of Classification
In this 2 hour 10 mins long project-based course, you will learn how to set up PyCaret Environment and become familiar with the variety of data preparing tasks done during setup, be able to create, see and compare performance of several models, learn how to tune your model without doing an exhaustive search, create impressive visuals of models, feature importance and much more.
Note: This course works best for learners who are based in the North America region. We’re currently working on providing the same experience in other regions.
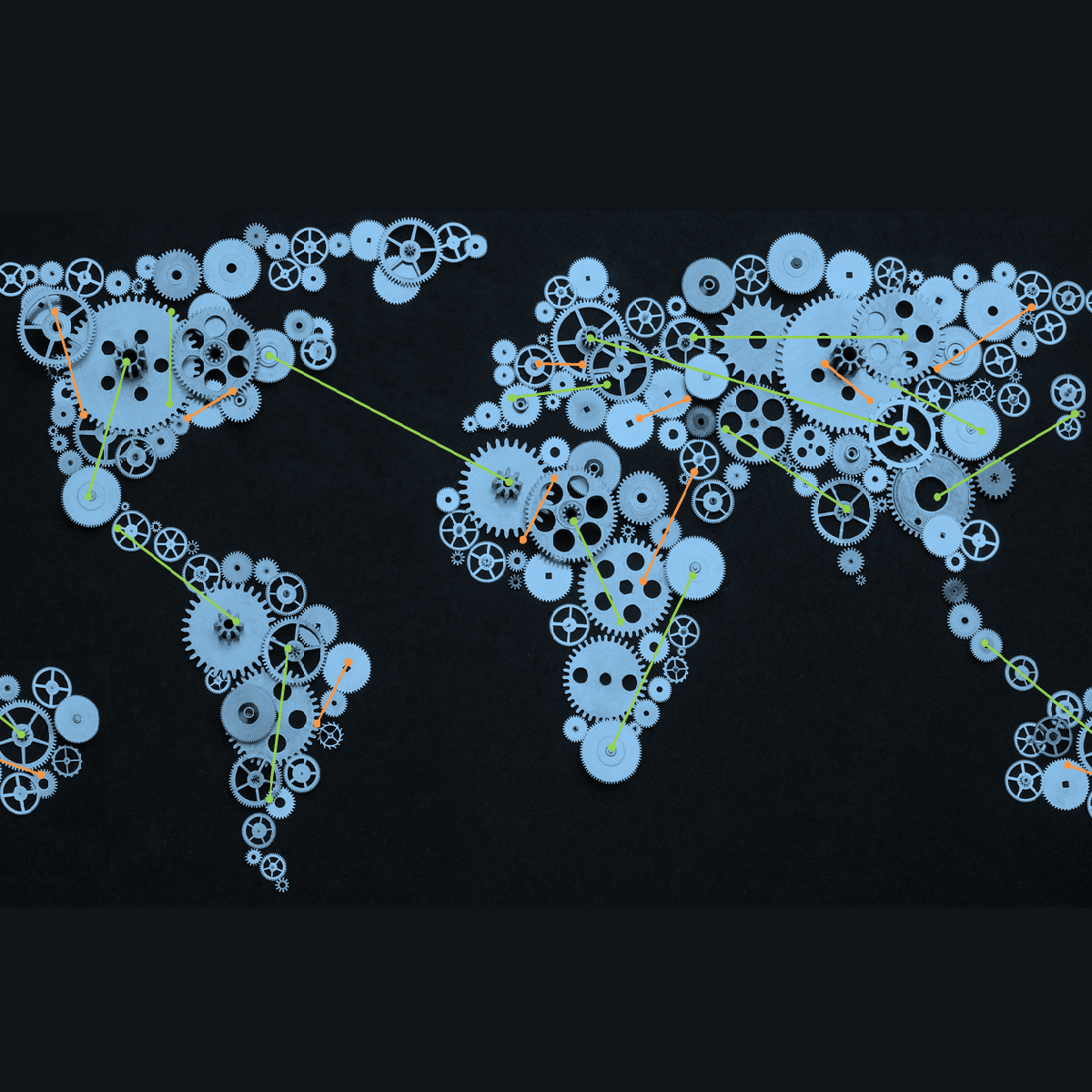
Advanced Manufacturing Enterprise
Enterprises that seek to become proficient in advanced manufacturing must incorporate manufacturing management tools and integrate data throughout the supply chain to be successful. This course will make students aware of what a digitally connected enterprise is, as they learn about the operational complexity of enterprises, business process optimization and the concept of an integrated product-process-value chain.
Students will become acquainted with the available tools, technologies and techniques for aggregation and integration of data throughout the manufacturing supply chain and entire product life-cycle. They will receive foundational knowledge to assist in efforts to facilitate design, planning, and production scheduling of goods and services by applying product life cycle data.
Main concepts of this course will be delivered through lectures, readings, discussions and various videos.
This is the sixth course in the Digital Manufacturing & Design Technology specialization that explores the many facets of manufacturing’s “Fourth Revolution,” aka Industry 4.0, and features a culminating project involving creation of a roadmap to achieve a self-established DMD-related professional goal. To learn more about the Digital Manufacturing and Design Technology specialization, please watch the overview video by copying and pasting the following link into your web browser: https://youtu.be/wETK1O9c-CA
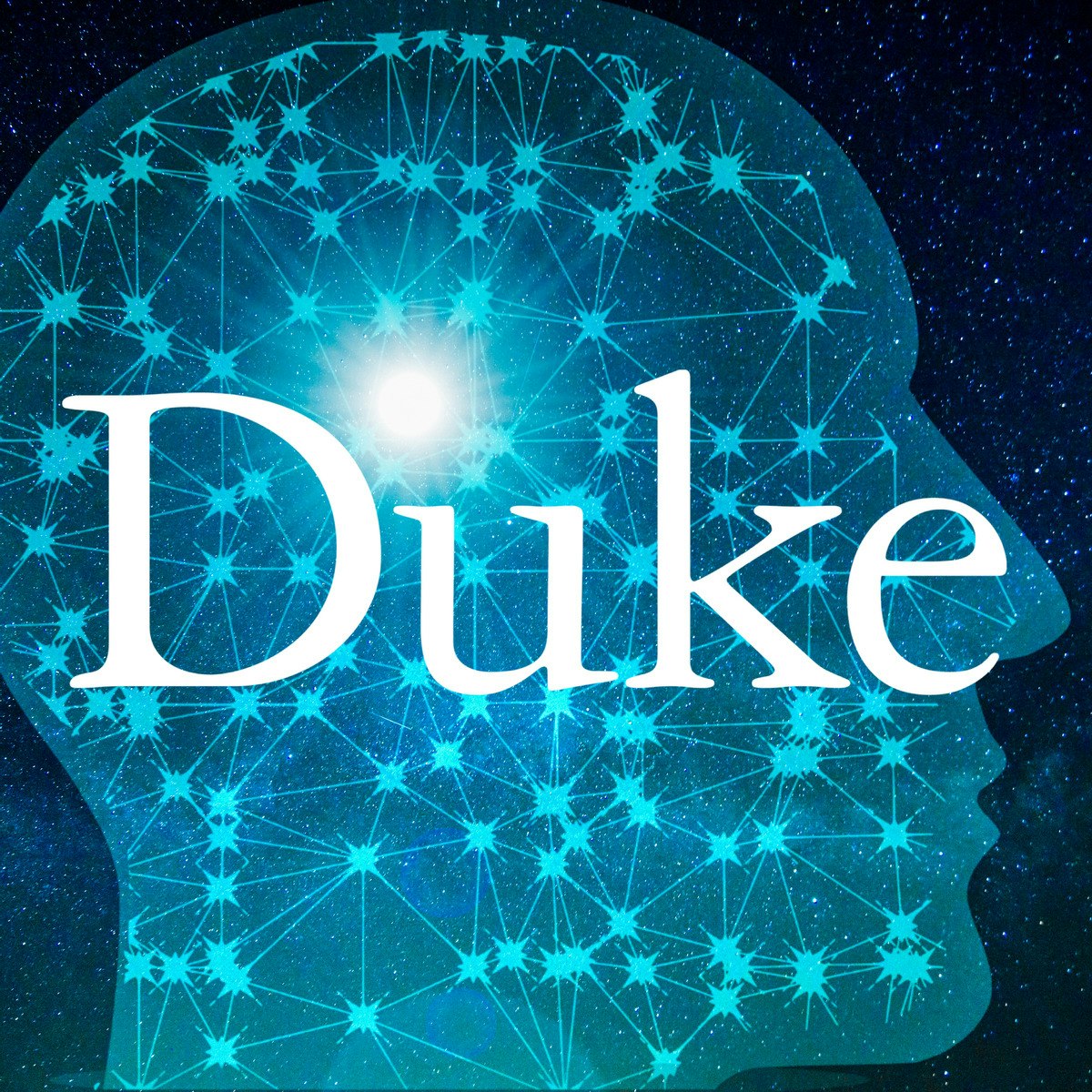
Managing Machine Learning Projects
This second course of the AI Product Management Specialization by Duke University's Pratt School of Engineering focuses on the practical aspects of managing machine learning projects. The course walks through the keys steps of a ML project from how to identify good opportunities for ML through data collection, model building, deployment, and monitoring and maintenance of production systems. Participants will learn about the data science process and how to apply the process to organize ML efforts, as well as the key considerations and decisions in designing ML systems.
At the conclusion of this course, you should be able to:
1) Identify opportunities to apply ML to solve problems for users
2) Apply the data science process to organize ML projects
3) Evaluate the key technology decisions to make in ML system design
4) Lead ML projects from ideation through production using best practices
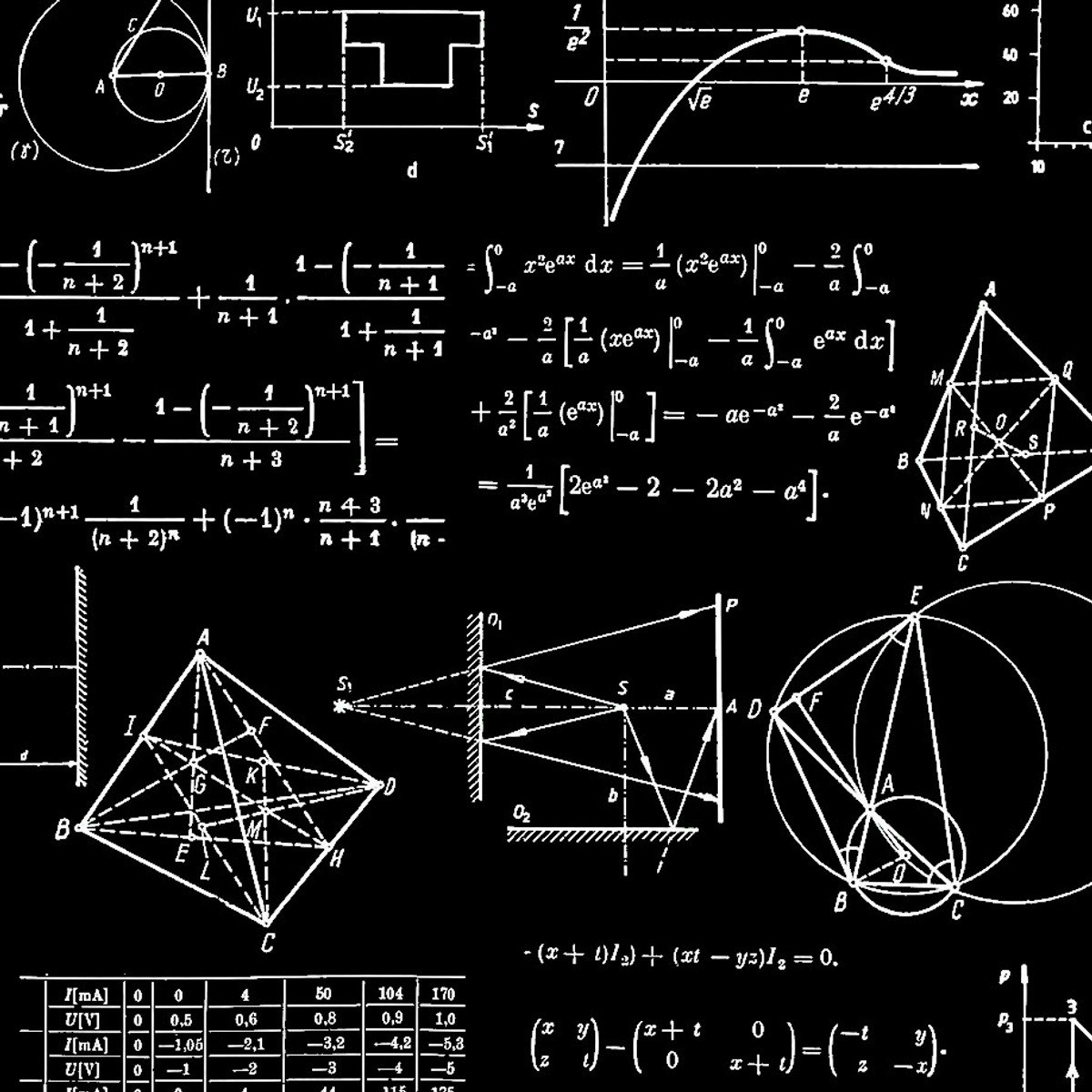
Essential Linear Algebra for Data Science
Are you interested in Data Science but lack the math background for it? Has math always been a tough subject that you tend to avoid? This course will teach you the most fundamental Linear Algebra that you will need for a career in Data Science without a ton of unnecessary proofs and concepts that you may never use. Consider this an expressway to Data Science with approachable methods and friendly concepts that will guide you to truly understanding the most important ideas in Linear Algebra.
This course is designed to prepare learners to successfully complete Statistical Modeling for Data Science Application, which is part of CU Boulder's Master of Science in Data Science (MS-DS) program.
Logo courtesy of Dan-Cristian Pădureț on Unsplash.com
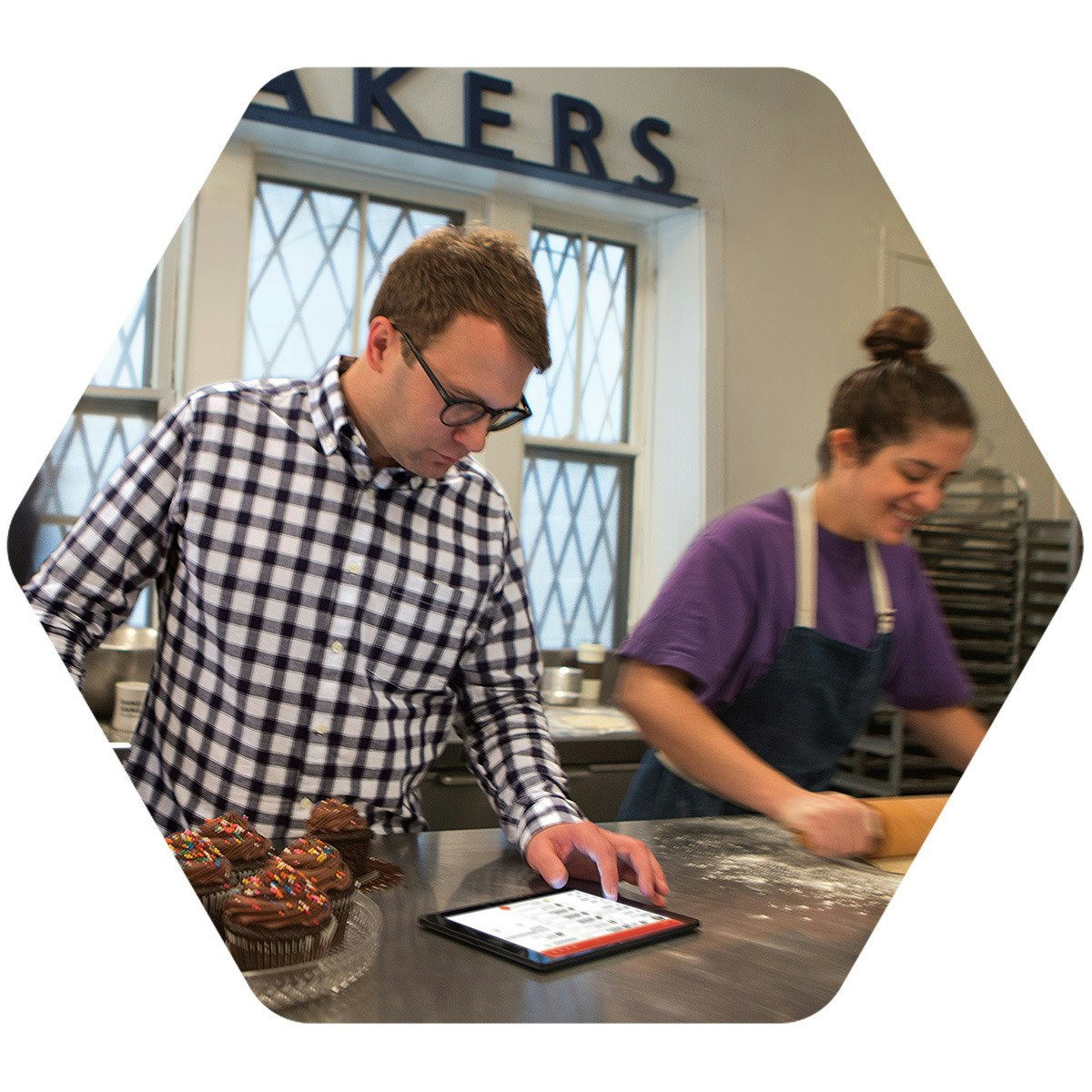
Building Conversational Experiences with Dialogflow
PLEASE NOTE: This course will close for new learner enrollment on January 22nd, 2021.
If you would prefer to engage with our latest courses related to this material, please see the following courses:
Contact Center AI: Conversational Design Fundamentals: https://www.coursera.org/learn/contact-center-ai-conversational-design-fundamentals/home/welcome.
Contact Center AI: Building a Dynamic Virtual Agent: https://www.coursera.org/learn/contact-center-ai-building-a-dynamic-virtual-agent/home/welcome
The Contact Center AI: Conversational Design Fundamentals and Contact Center AI: Building a Dynamic Virtual Agent courses have similar learning objectives to the ones in the course being deprecated in this notice. They walk learners through the process of identifying a use case, creating intents and entities for conversational agents, and using context and fulfillment for conversation awareness and third party system integrations. Since many use cases for virtual agents happen in the context of contact centers, we invite you to extend your expertise by taking additional courses in the Contact Center AI Specialization, although this is not required if your interest is solely on building automated conversational agents.
Thanks, and happy learning,
The Google Cloud Learning Services team
Course Description:
This course provides a deep dive into how to create a chatbot using Dialogflow, augment it with Cloud Natural Language API, and operationalize it using Google Cloud tools.
>>> By enrolling in this course you agree to the Qwiklabs Terms of Service as set out in the FAQ and located at: https://qwiklabs.com/terms_of_service <<<
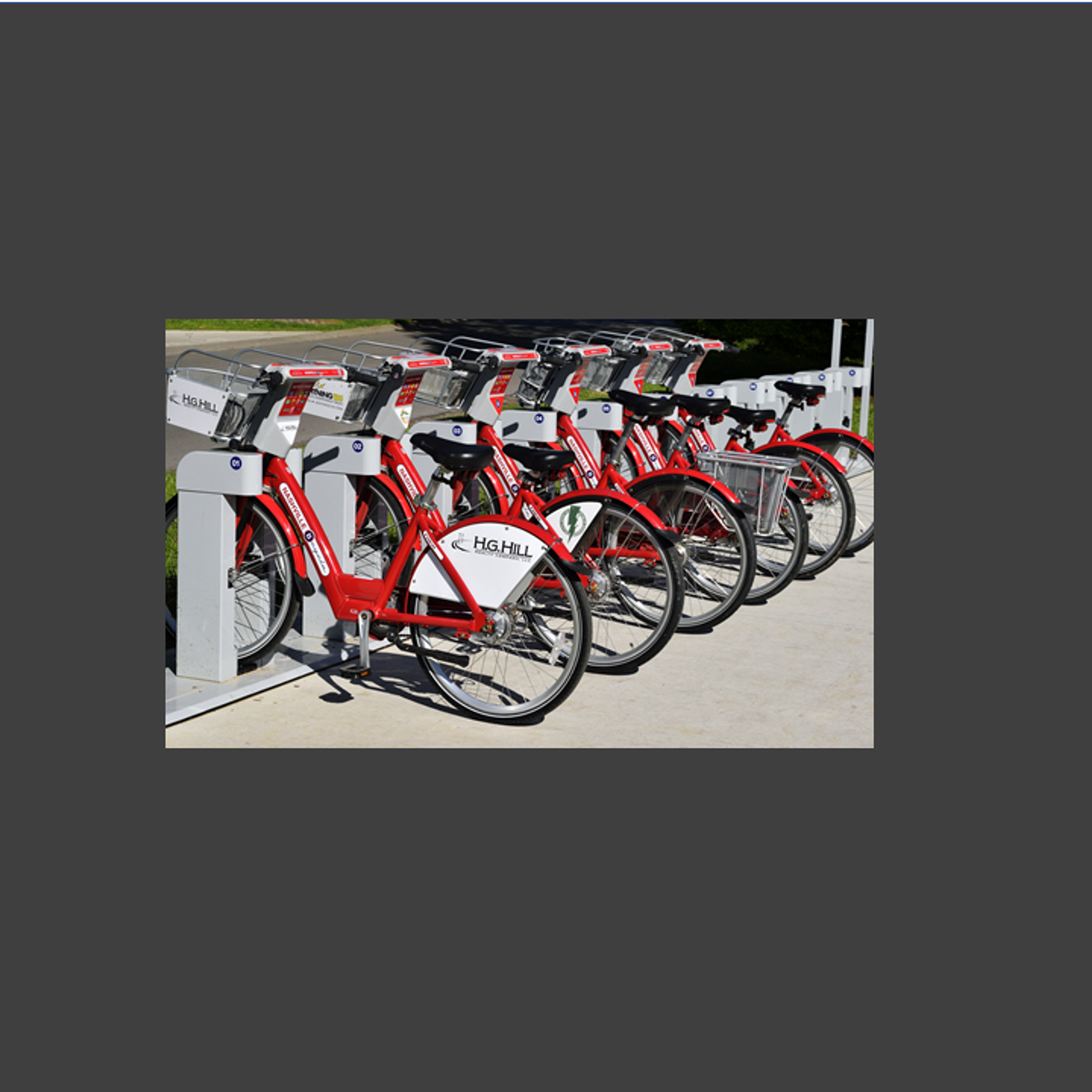
Bike Rental Sharing Demand Prediction with Machine Learning
In this 1-hour long project-based course, you will learn how to predict bike sharing demand with machine learning. Bike sharing services enable people to rent a bike from one location and drop it off at another location on an as-needed basis. The objective of this guided project is to predict bike sharing rental usage based on inputs such as temperature, season, humidity, wind speed.
Note: This course works best for learners who are based in the North America region. We’re currently working on providing the same experience in other regions.
Popular Internships and Jobs by Categories
Browse
© 2024 BoostGrad | All rights reserved