Back to Courses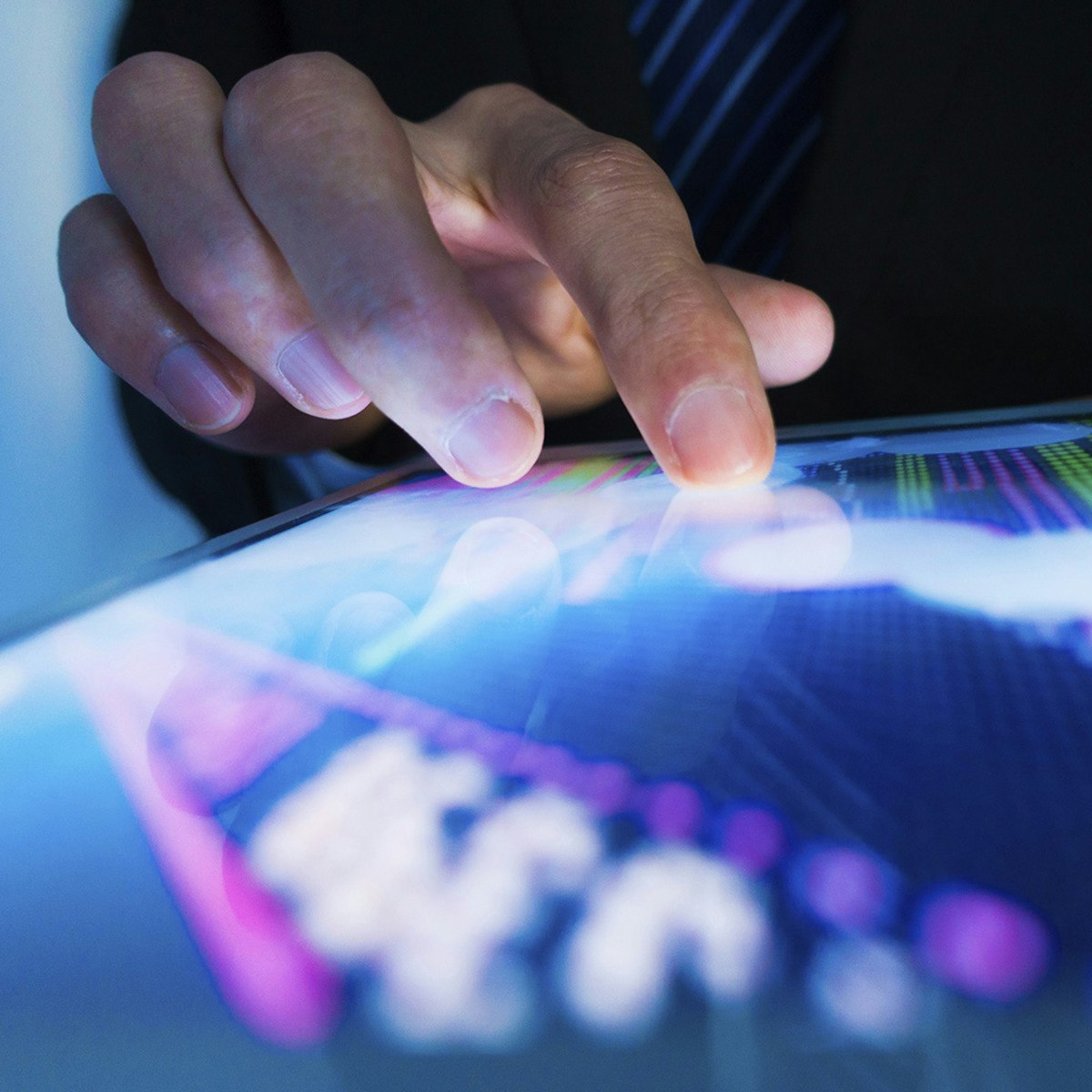
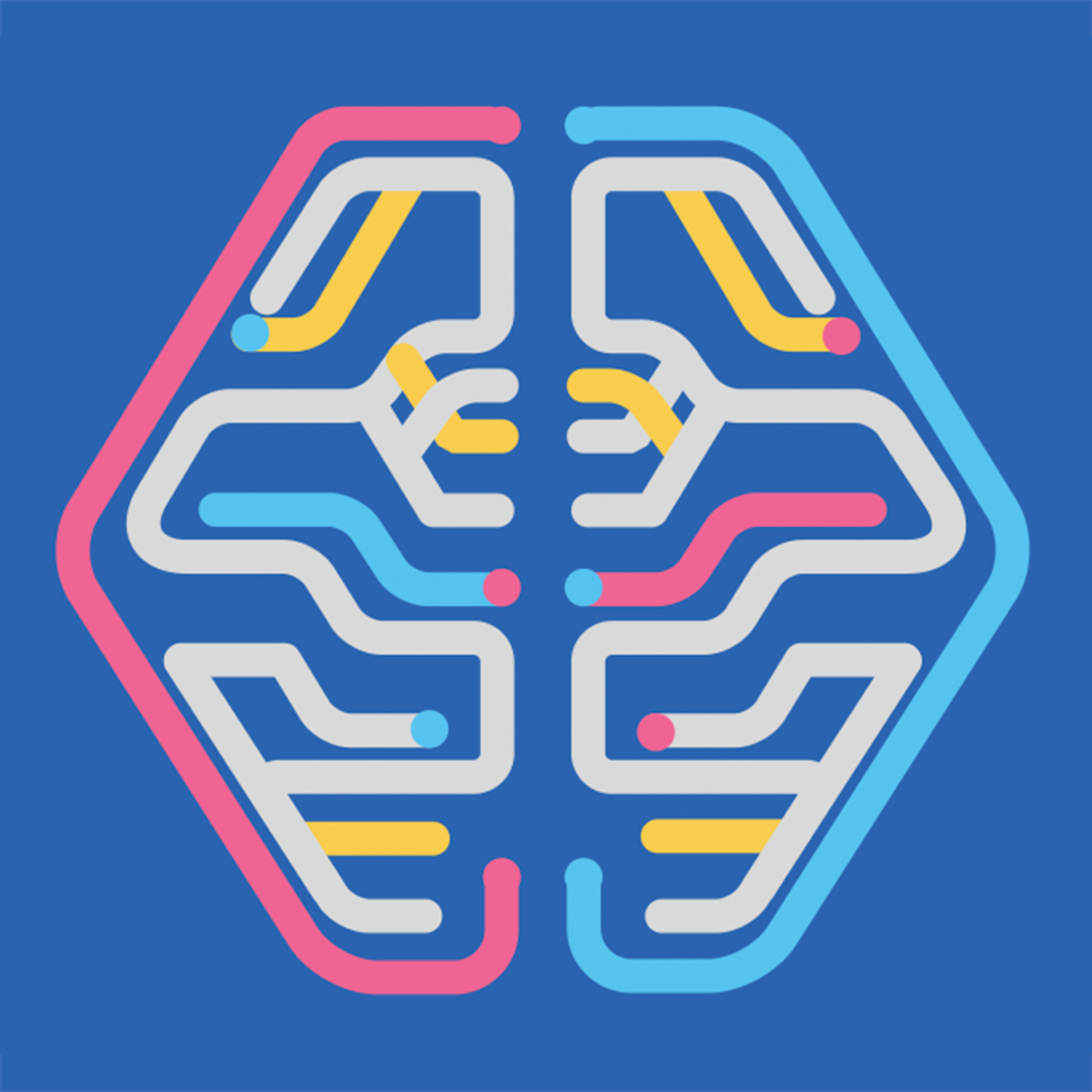
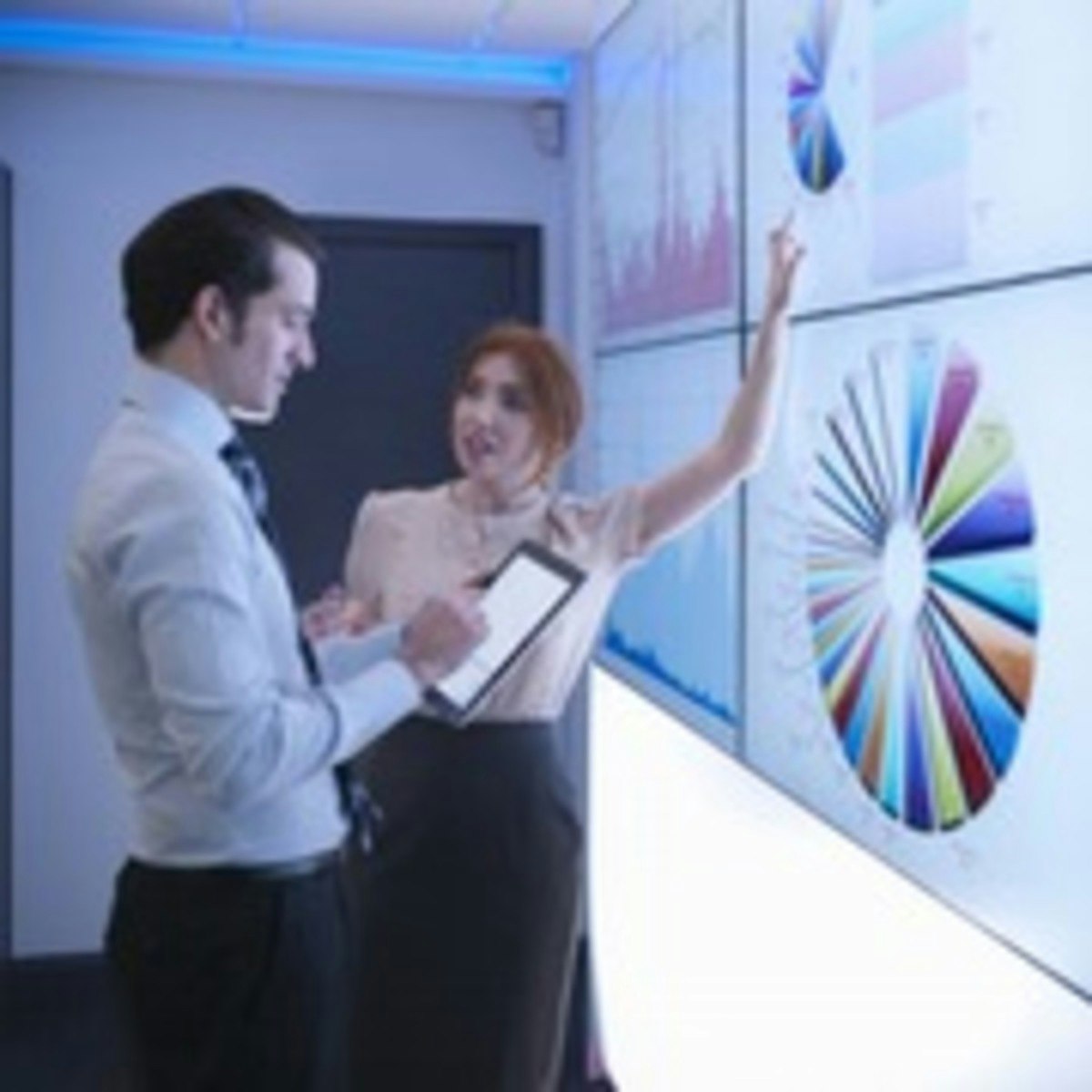
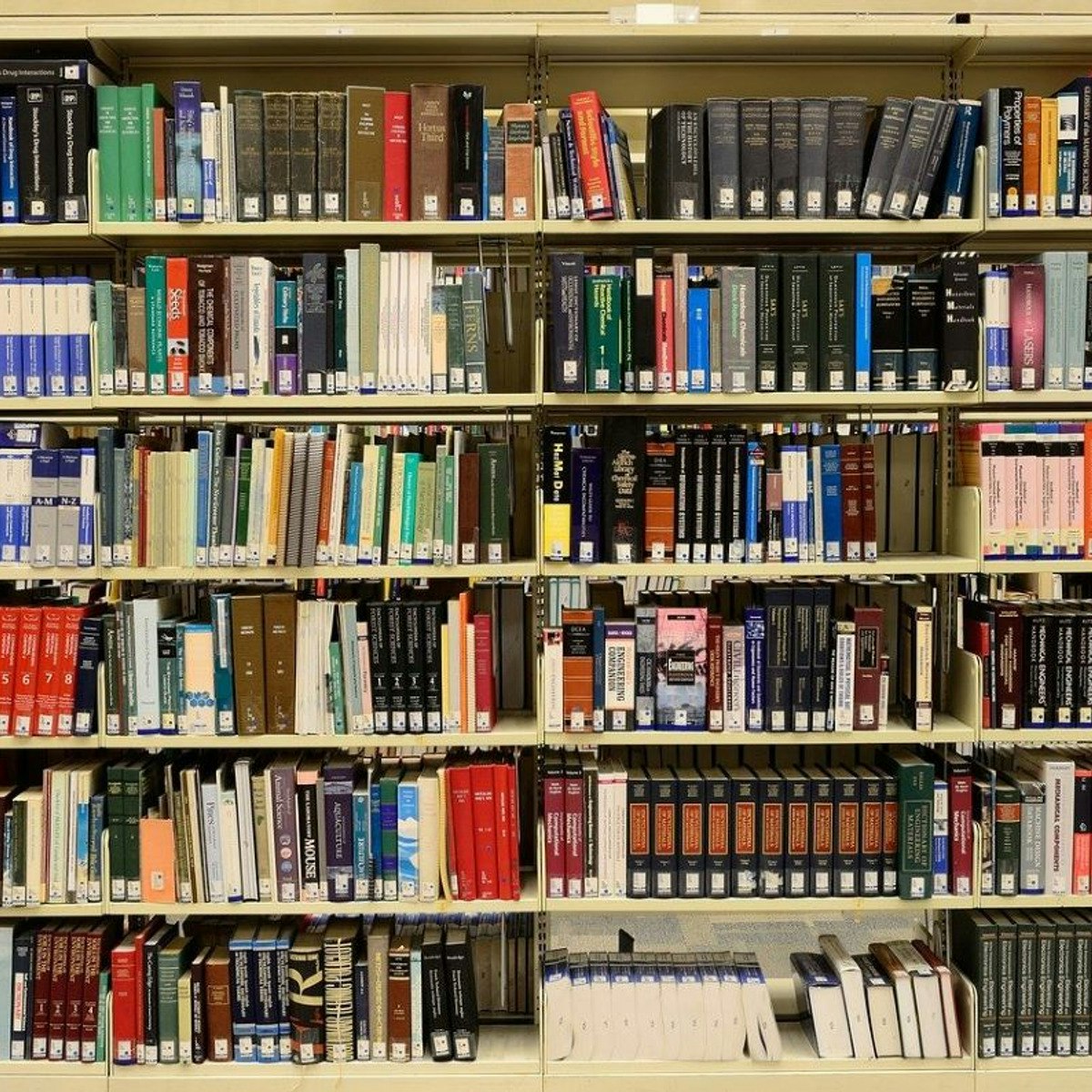
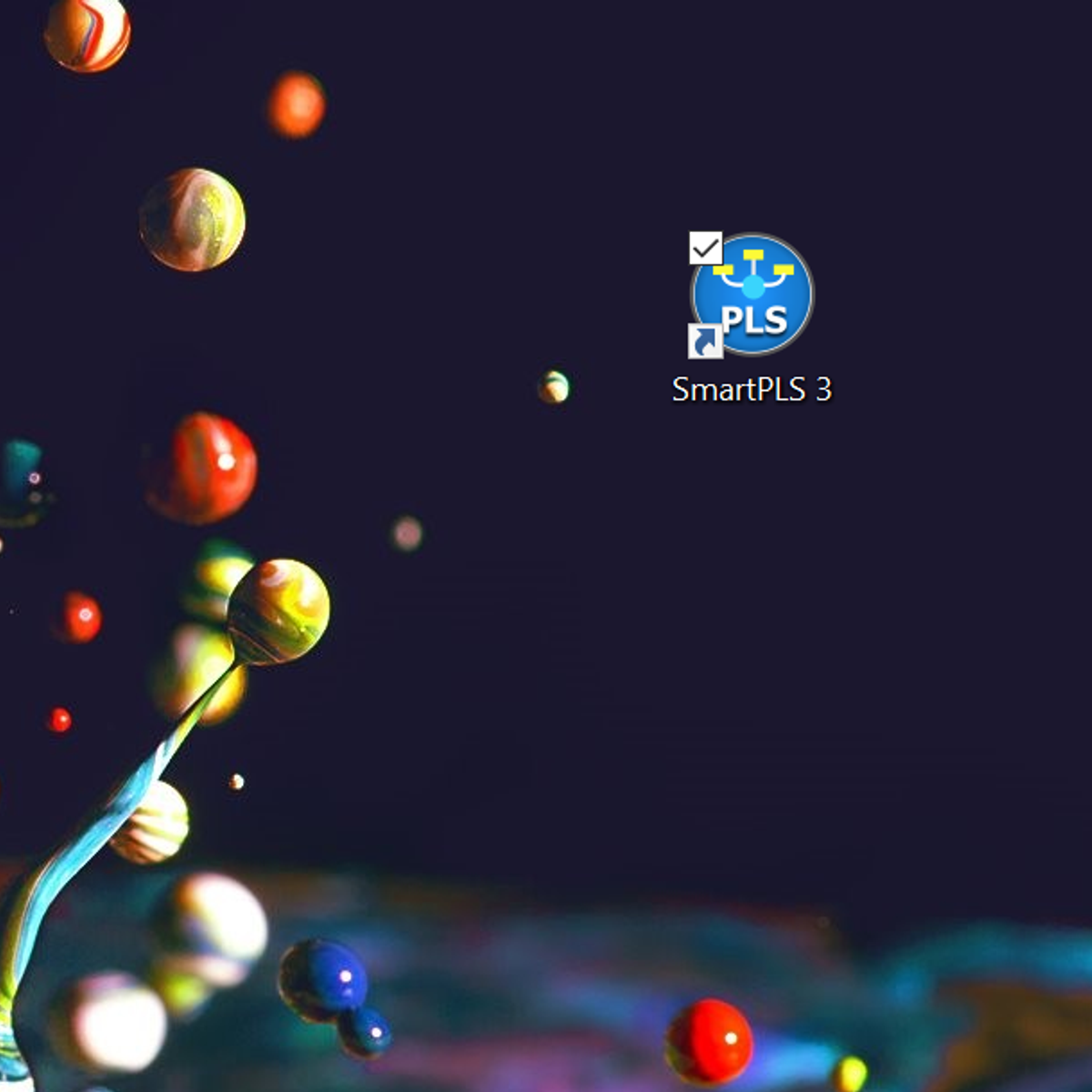
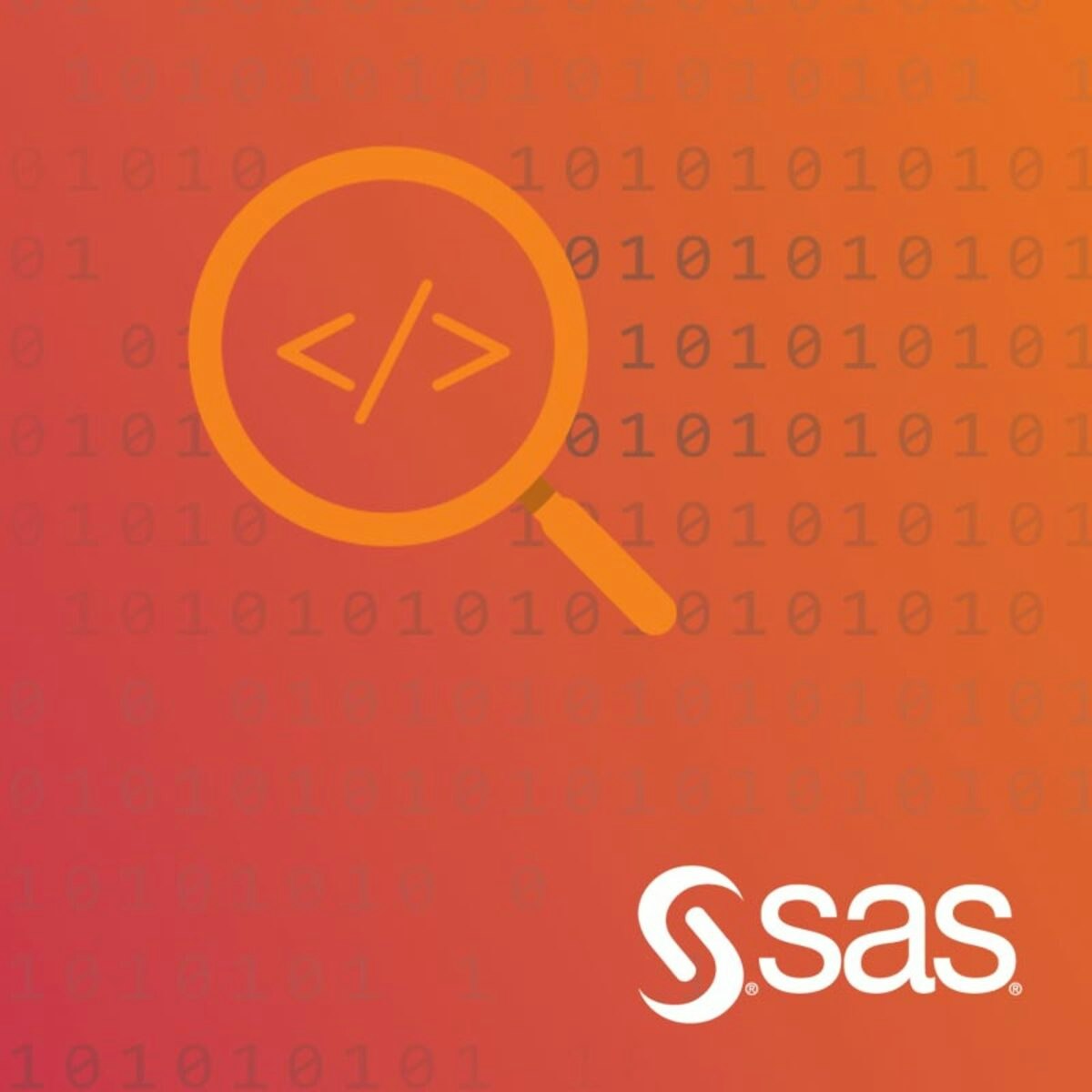
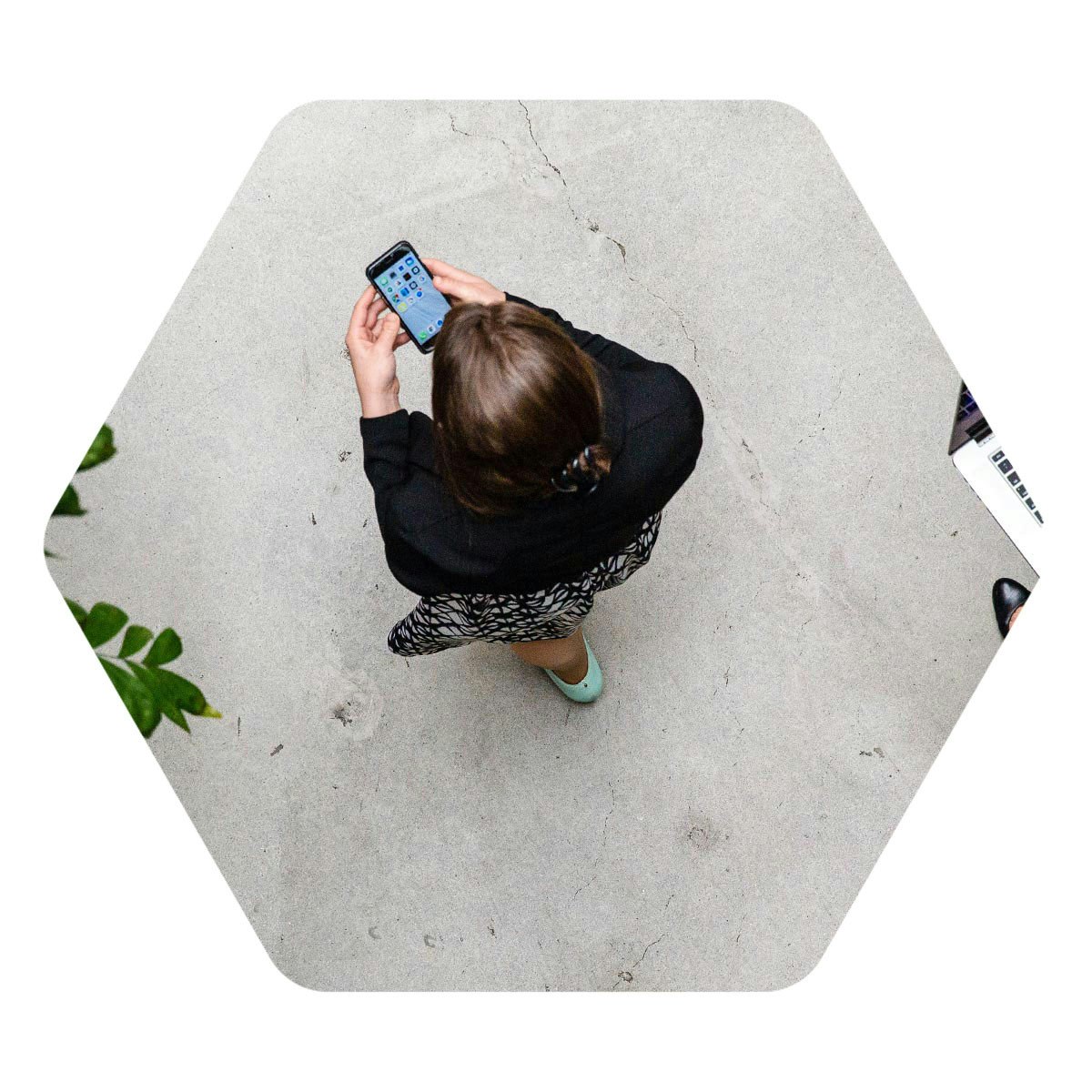
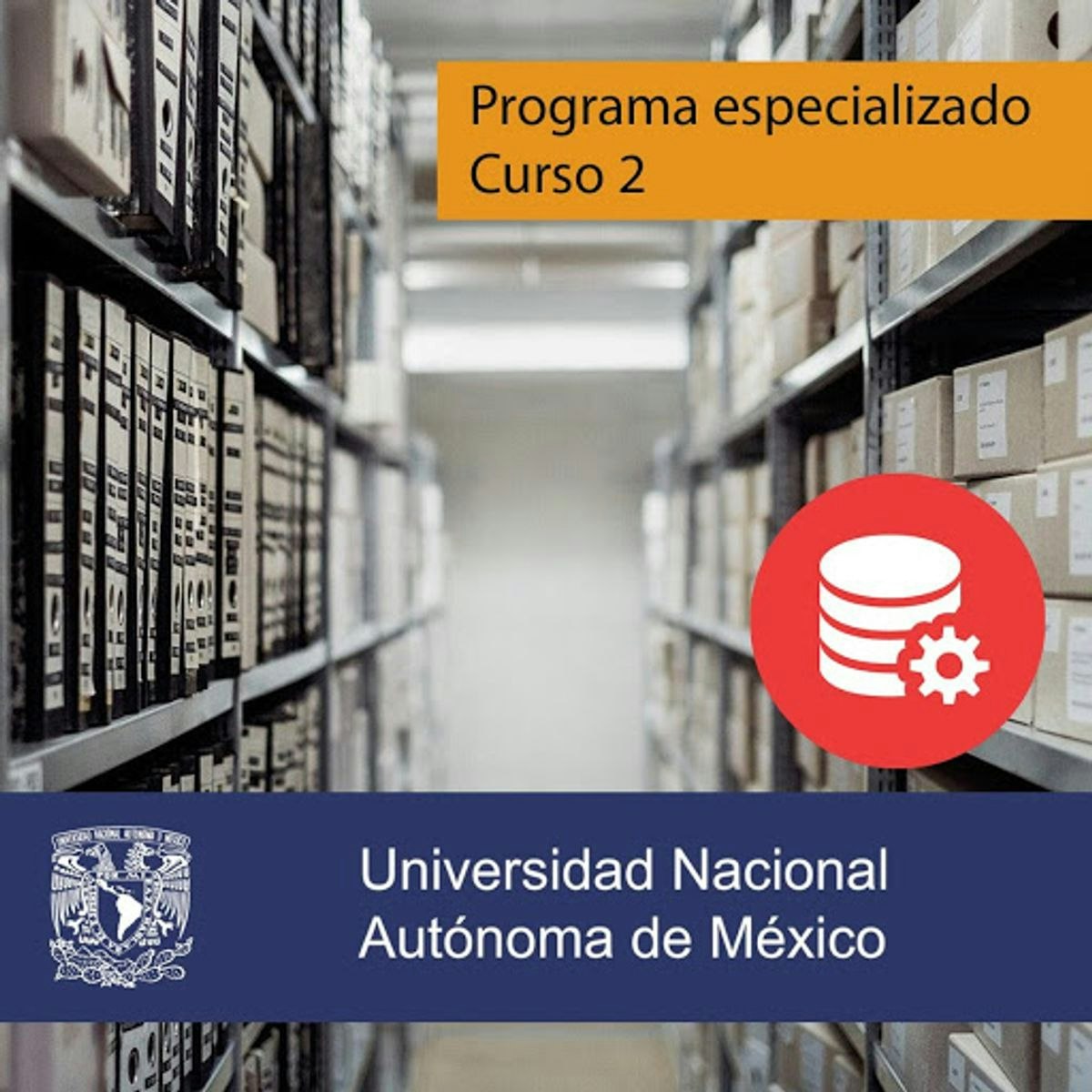
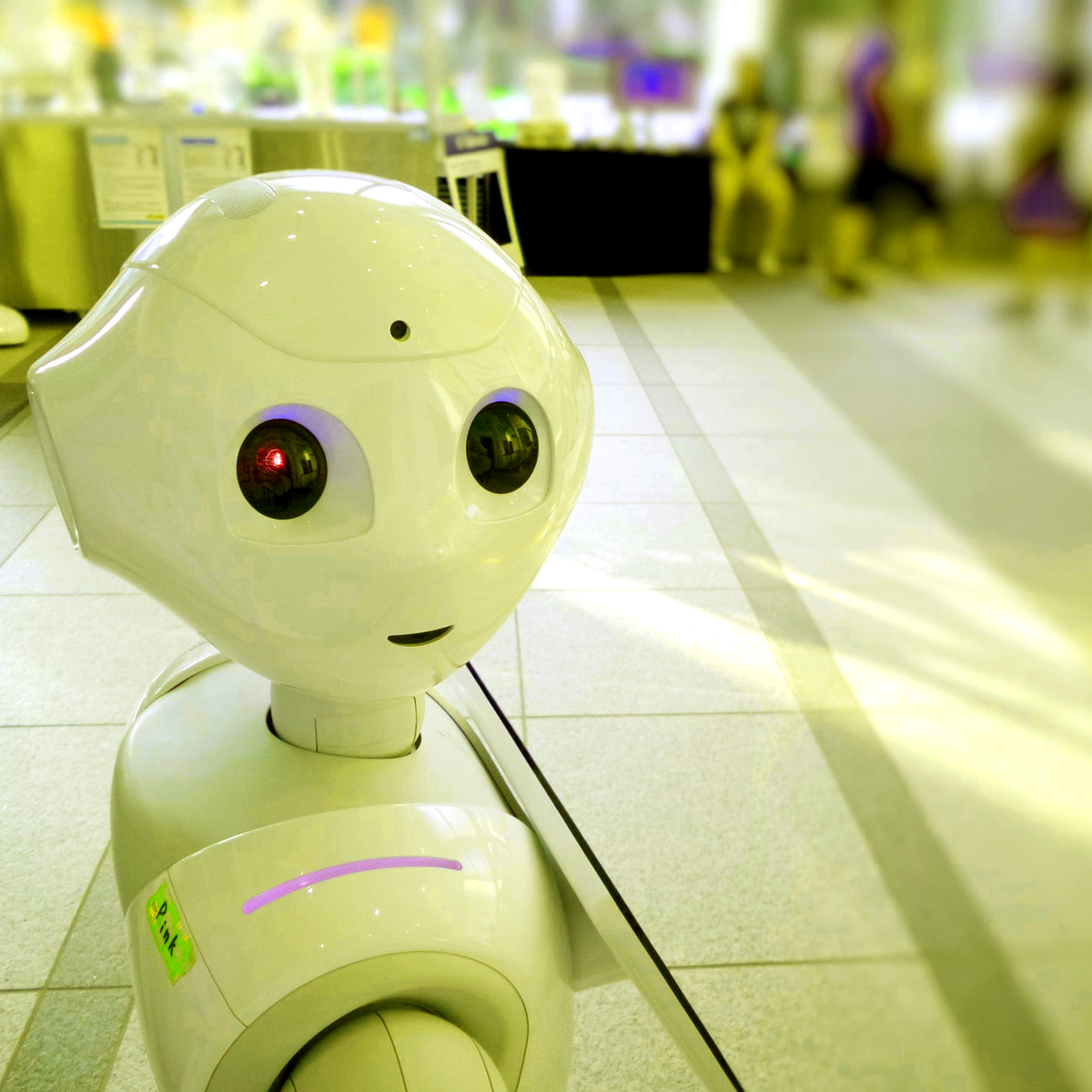
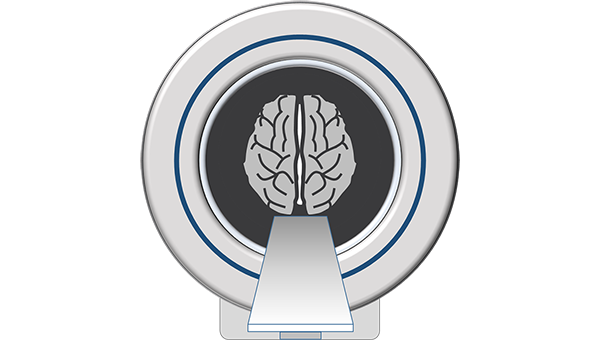
Data Science Courses - Page 45
Showing results 441-450 of 1407
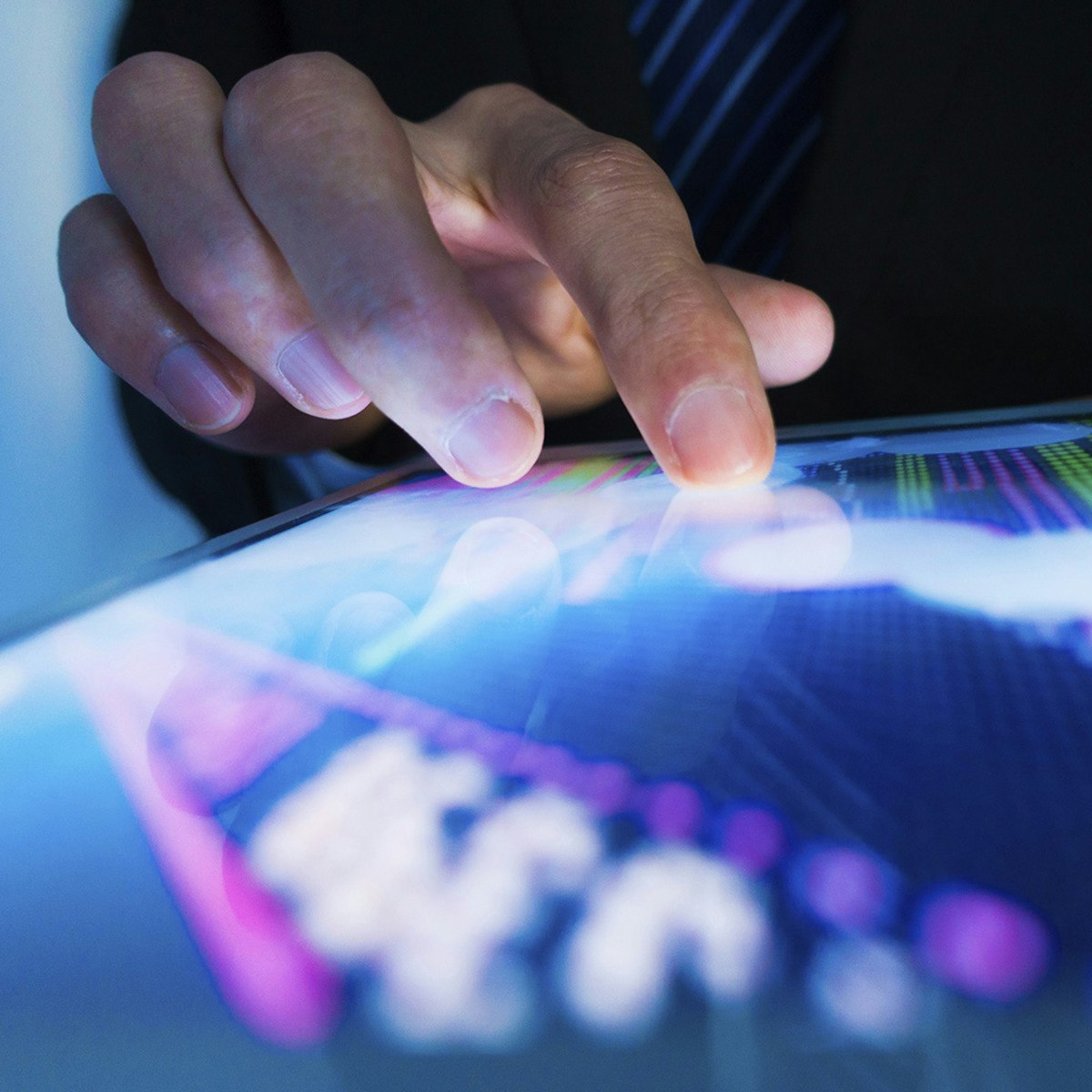
Business Applications of Hypothesis Testing and Confidence Interval Estimation
Confidence intervals and Hypothesis tests are very important tools in the Business Statistics toolbox. A mastery over these topics will help enhance your business decision making and allow you to understand and measure the extent of ‘risk’ or ‘uncertainty’ in various business processes.
This is the third course in the specialization "Business Statistics and Analysis" and the course advances your knowledge about Business Statistics by introducing you to Confidence Intervals and Hypothesis Testing. We first conceptually understand these tools and their business application. We then introduce various calculations to constructing confidence intervals and to conduct different kinds of Hypothesis Tests. These are done by easy to understand applications.
To successfully complete course assignments, students must have access to a Windows version of Microsoft Excel 2010 or later. Please note that earlier versions of Microsoft Excel (2007 and earlier) will not be compatible to some Excel functions covered in this course.
WEEK 1
Module 1: Confidence Interval - Introduction
In this module you will get to conceptually understand what a confidence interval is and how is its constructed. We will introduce the various building blocks for the confidence interval such as the t-distribution, the t-statistic, the z-statistic and their various excel formulas. We will then use these building blocks to construct confidence intervals.
Topics covered include:
• Introducing the t-distribution, the T.DIST and T.INV excel functions
• Conceptual understanding of a Confidence Interval
• The z-statistic and the t-statistic
• Constructing a Confidence Interval using z-statistic and t-statistic
WEEK 2
Module 2: Confidence Interval - Applications
This module presents various business applications of the confidence interval including an application where we use the confidence interval to calculate an appropriate sample size. We also introduce with an application, the confidence interval for a population proportion. Towards the close of module we start introducing the concept of Hypothesis Testing.
Topics covered include:
• Applications of Confidence Interval
• Confidence Interval for a Population Proportion
• Sample Size Calculation
• Hypothesis Testing, An Introduction
WEEK 3
Module 3: Hypothesis Testing
This module introduces Hypothesis Testing. You get to understand the logic behind hypothesis tests. The four steps for conducting a hypothesis test are introduced and you get to apply them for hypothesis tests for a population mean as well as population proportion. You will understand the difference between single tail hypothesis tests and two tail hypothesis tests and also the Type I and Type II errors associated with hypothesis tests and ways to reduce such errors.
Topics covered include:
• The Logic of Hypothesis Testing
• The Four Steps for conducting a Hypothesis Test
• Single Tail and Two Tail Hypothesis Tests
• Guidelines, Formulas and an Application of Hypothesis Test
• Hypothesis Test for a Population Proportion
• Type I and Type II Errors in a Hypothesis
WEEK 4
Module 4: Hypothesis Test - Differences in Mean
In this module, you'll apply Hypothesis Tests to test the difference between two different data, such hypothesis tests are called difference in means tests. We will introduce the three kinds of difference in means test and apply them to various business applications. We will also introduce the Excel dialog box to conduct such hypothesis tests.
Topics covered include:
• Introducing the Difference-In-Means Hypothesis Test
• Applications of the Difference-In-Means Hypothesis Test
• The Equal & Unequal Variance Assumption and the Paired t-test for difference in means.
• Some more applications
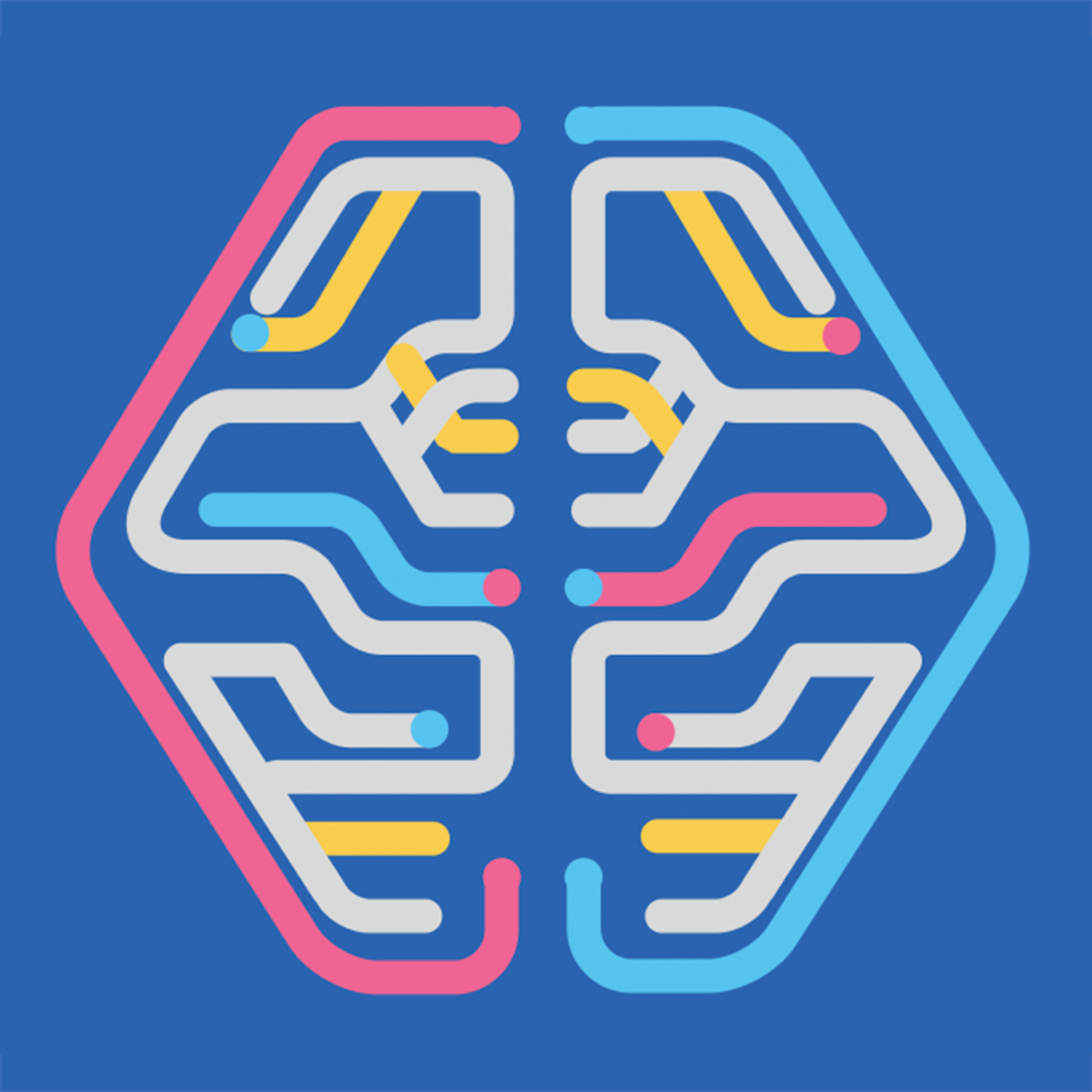
How Google does Machine Learning
What are best practices for implementing machine learning on Google Cloud? What is Vertex AI and how can you use the platform to quickly build, train, and deploy AutoML machine learning models without writing a single line of code? What is machine learning, and what kinds of problems can it solve?
Google thinks about machine learning slightly differently: it’s about providing a unified platform for managed datasets, a feature store, a way to build, train, and deploy machine learning models without writing a single line of code, providing the ability to label data, create Workbench notebooks using frameworks such as TensorFlow, SciKit Learn, Pytorch, R, and others. Our Vertex AI Platform also includes the ability to train custom models, build component pipelines, and perform both online and batch predictions. We also discuss the five phases of converting a candidate use case to be driven by machine learning, and consider why it is important to not skip the phases. We end with a recognition of the biases that machine learning can amplify and how to recognize them.
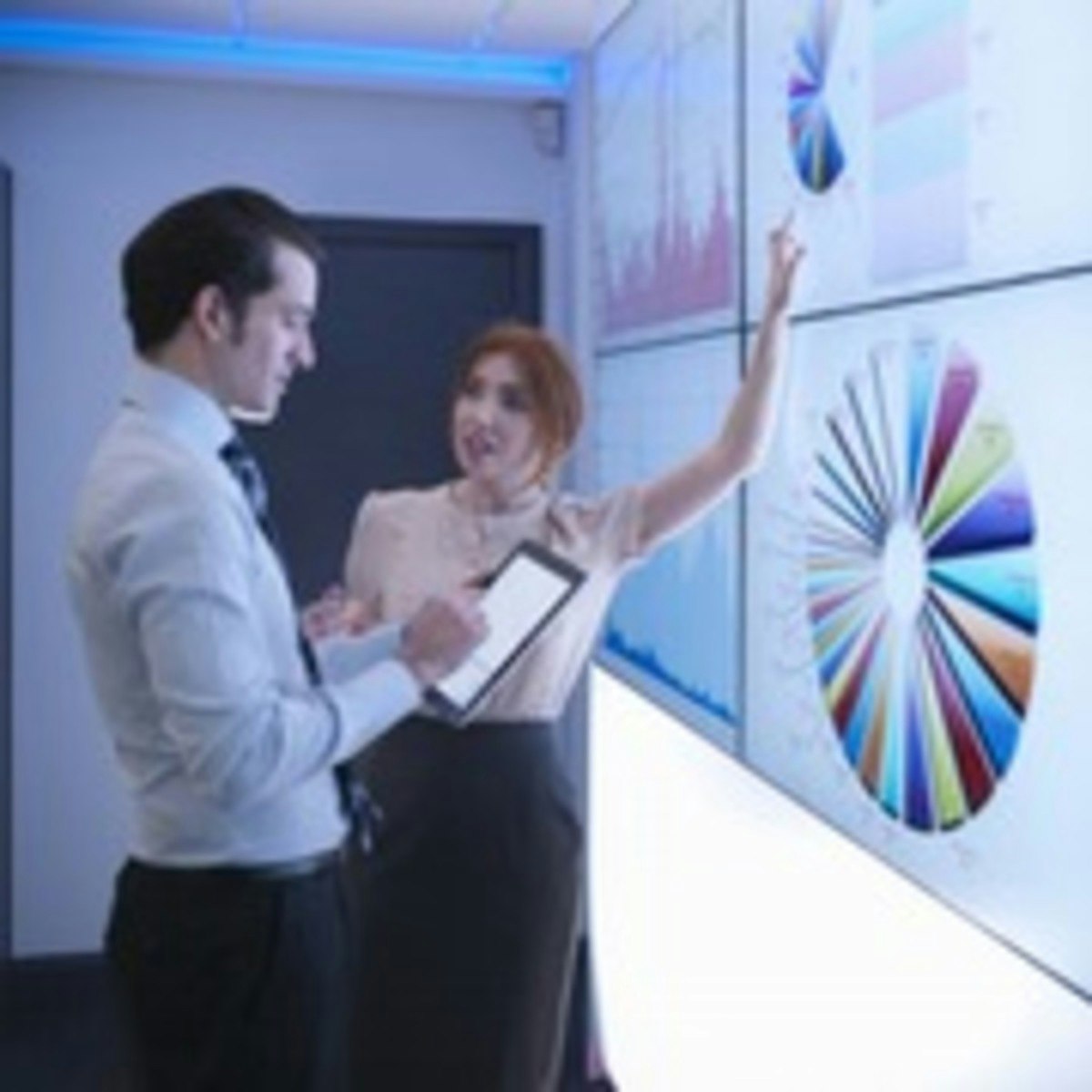
Visual Analytics with Tableau
In this third course of the specialization, we’ll drill deeper into the tools Tableau offers in the areas of charting, dates, table calculations and mapping. We’ll explore the best choices for charts, based on the type of data you are using. We’ll look at specific types of charts including scatter plots, Gantt charts, histograms, bullet charts and several others, and we’ll address charting guidelines. We’ll define discrete and continuous dates, and examine when to use each one to explain your data. You’ll learn how to create custom and quick table calculations and how to create parameters. We’ll also introduce mapping and explore how Tableau can use different types of geographic data, how to connect to multiple data sources and how to create custom maps.
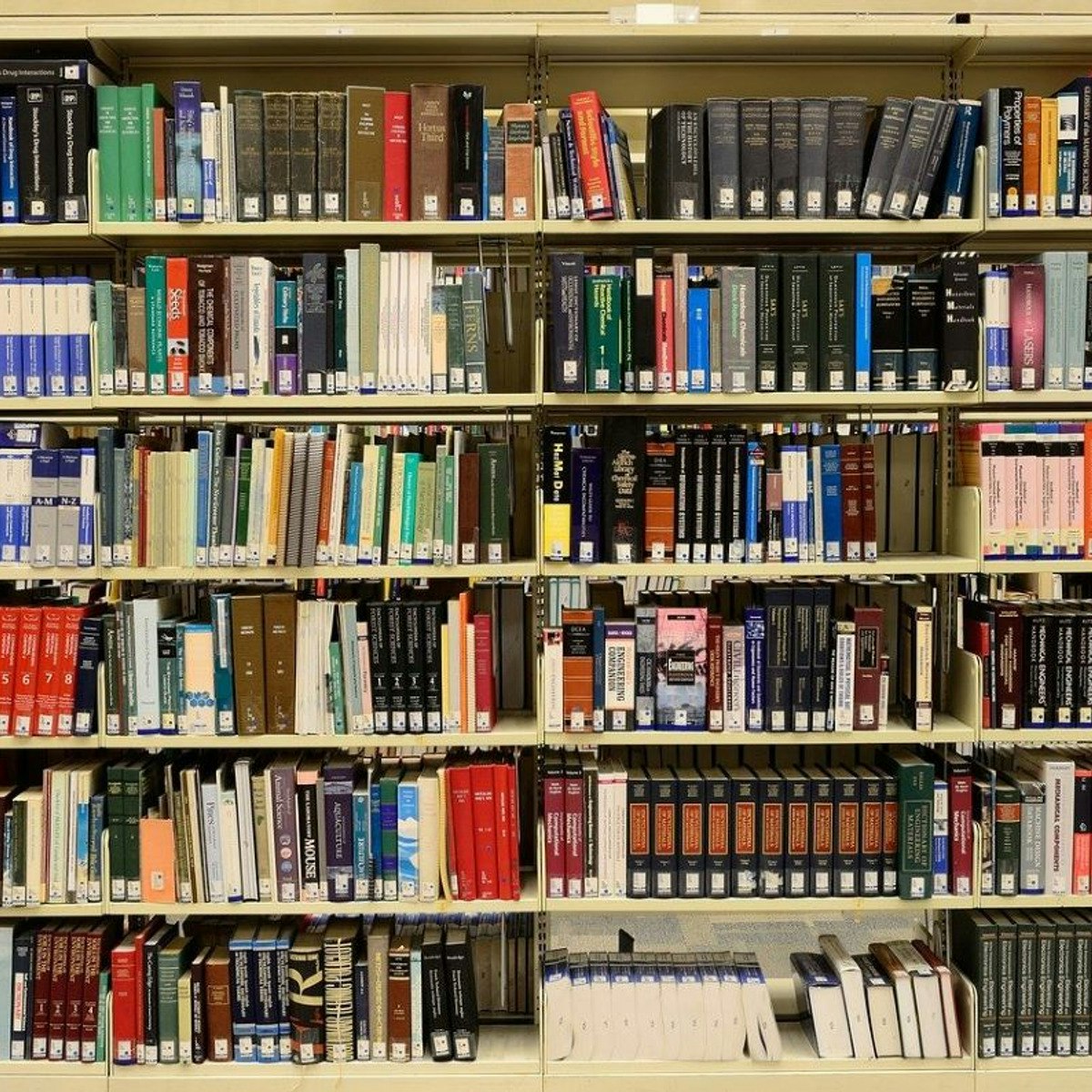
Quantitative Text Analysis and Measures of Readability in R
By the end of this project, you will be able to load textual data into R and turn it into a corpus object. You will also understand the concept of measures of readability in textual analysis. You will know how to estimate the level of readability of a text document or corpus of documents using a number of different readability metrics and how to plot the variation in readability levels in a text document corpus over time at the document and paragraph level.
This project is aimed at beginners who have a basic familiarity with the statistical programming language R and the RStudio environment, or people with a small amount of experience who would like to learn how to measure the readability of textual data.
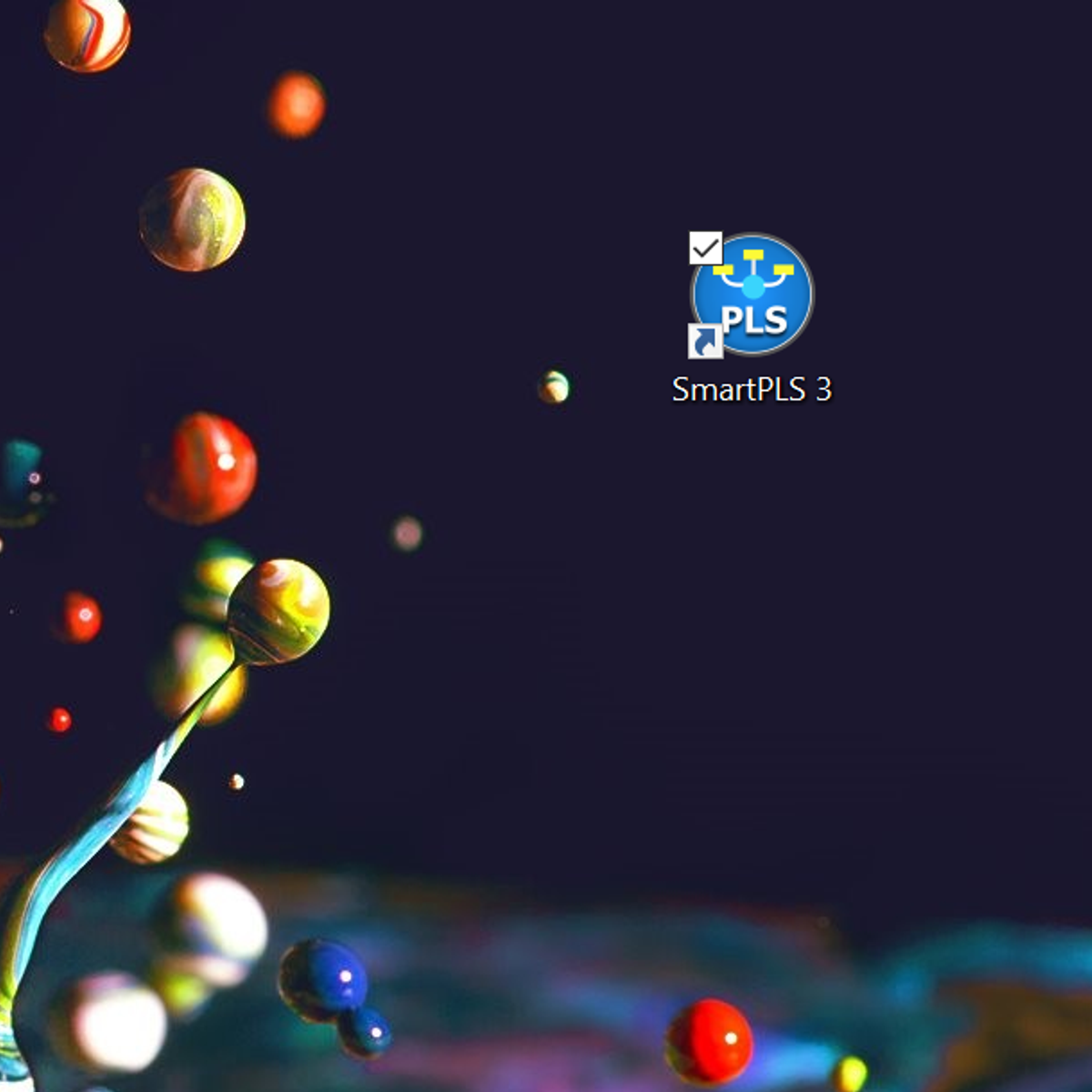
Advanced Models in Smartpls
In this 1-hour long project-based course, you will learn how to create path models using Smartpls. We will take a project on changing behavior and check if attitudes or subjective norms impact behavior the most.
We will learn how to launch this new software, create the model and run it. We will then show you how to interpret the same. We will also learn how to create models for different groups such as males and females and if there is a difference between them.
Note: This course works best for learners who are based in the North America region. We’re currently working on providing the same experience in other regions.
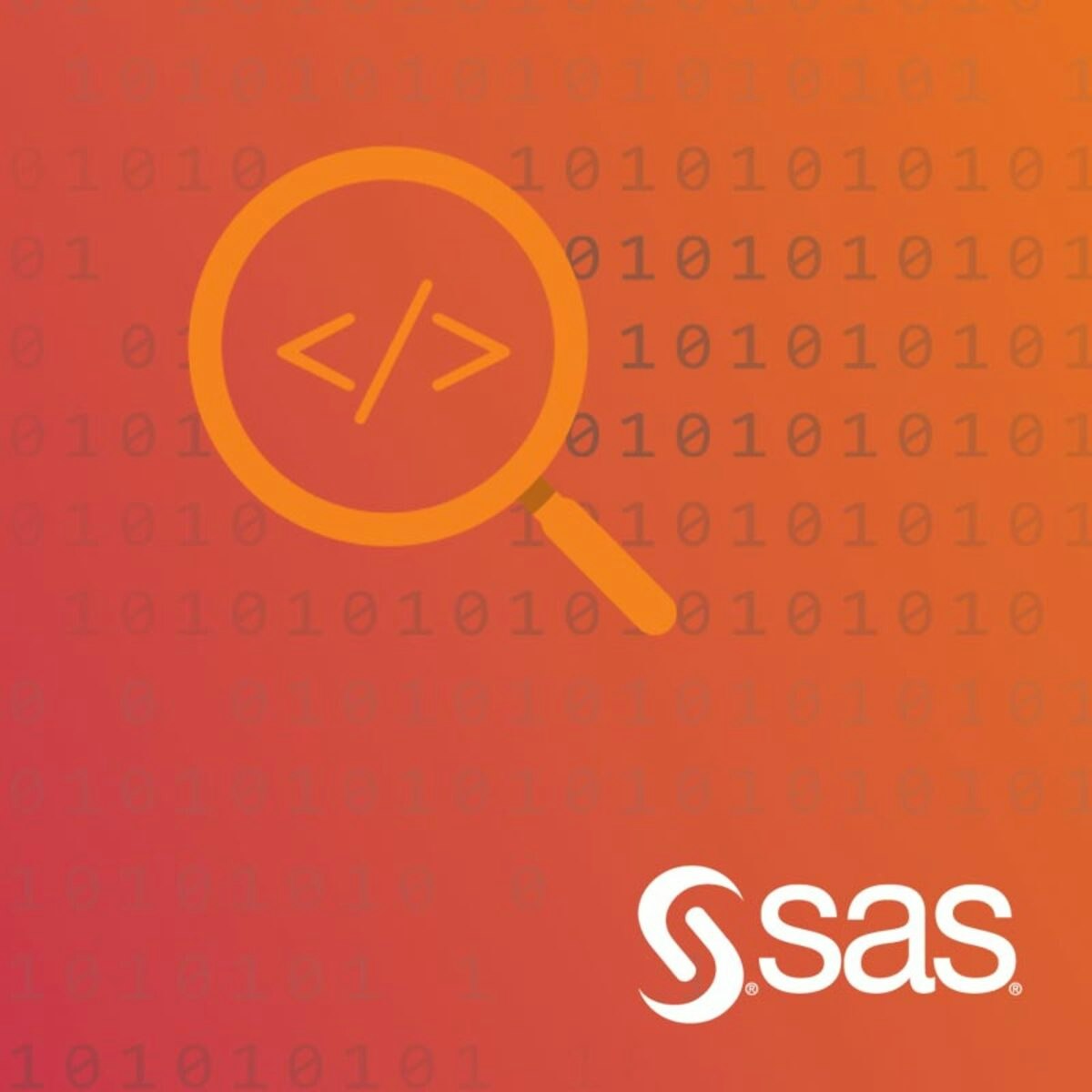
Advanced SAS Programming Techniques
In this course, you learn advanced techniques within the DATA step and procedures to manipulate data.
“By the end of this course, a learner will be able to…”
● Use additional functions (LAG, FINDC/FINDW, and COUNT/COUNTC/COUNTW).
● Perform pattern matching using PRX functions.
● Process repetitive code, rotate data, and perform table lookups using arrays.
● Perform table lookups and sort data using hash and hash iterator objects.
● Create numeric templates using the FORMAT procedure.
● Create custom functions using the FCMP procedure.
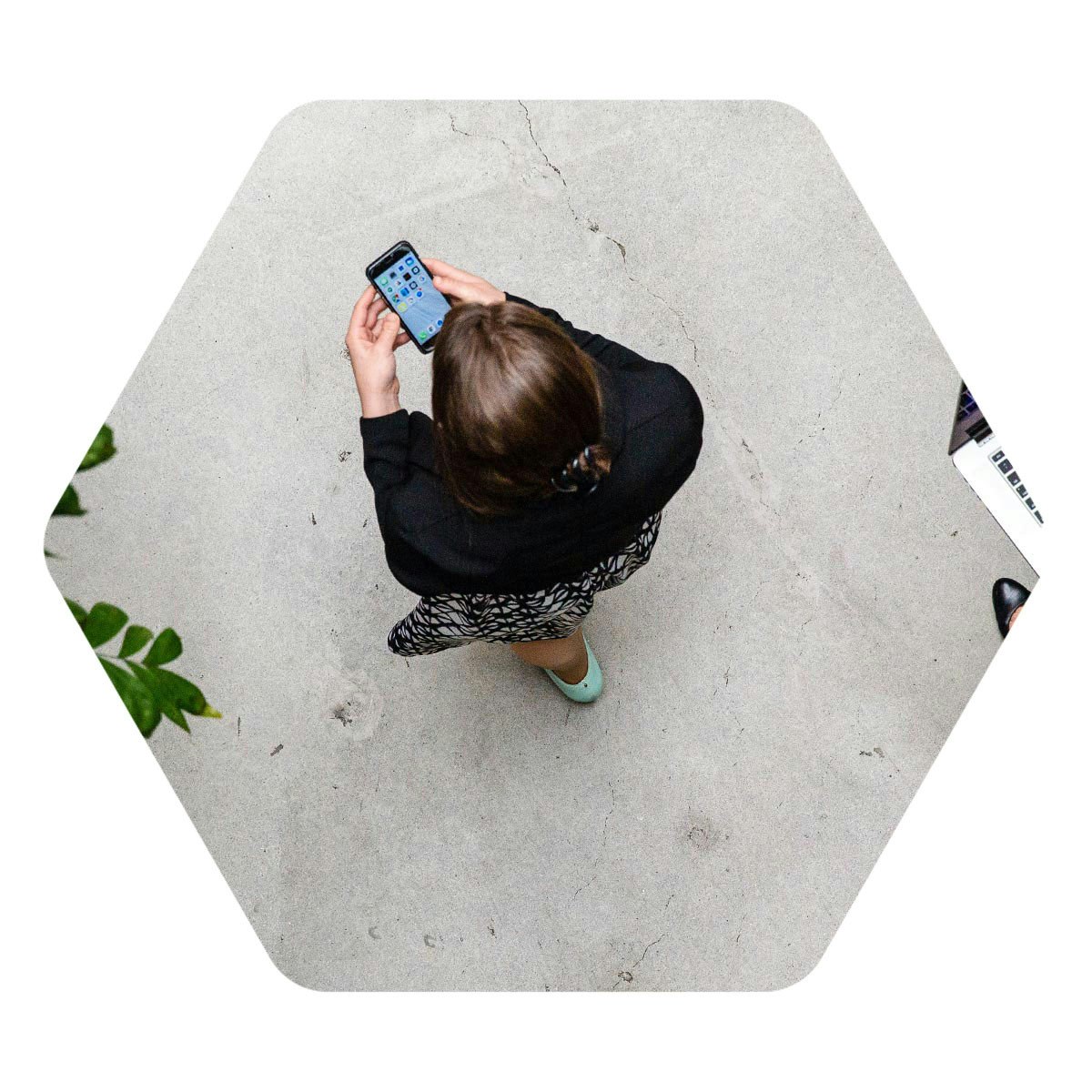
Achieving Advanced Insights with BigQuery
The third course in this course series is Achieving Advanced Insights with BigQuery. Here we will build on your growing knowledge of SQL as we dive into advanced functions and how to break apart a complex query into manageable steps.
We will cover the internal architecture of BigQuery (column-based sharded storage) and advanced SQL topics like nested and repeated fields through the use of Arrays and Structs. Lastly we will dive into optimizing your queries for performance and how you can secure your data through authorized views.
After completing this course, enroll in the Applying Machine Learning to your Data with Google Cloud course.
>>> By enrolling in this specialization you agree to the Qwiklabs Terms of Service as set out in the FAQ and located at: https://qwiklabs.com/terms_of_service <<<
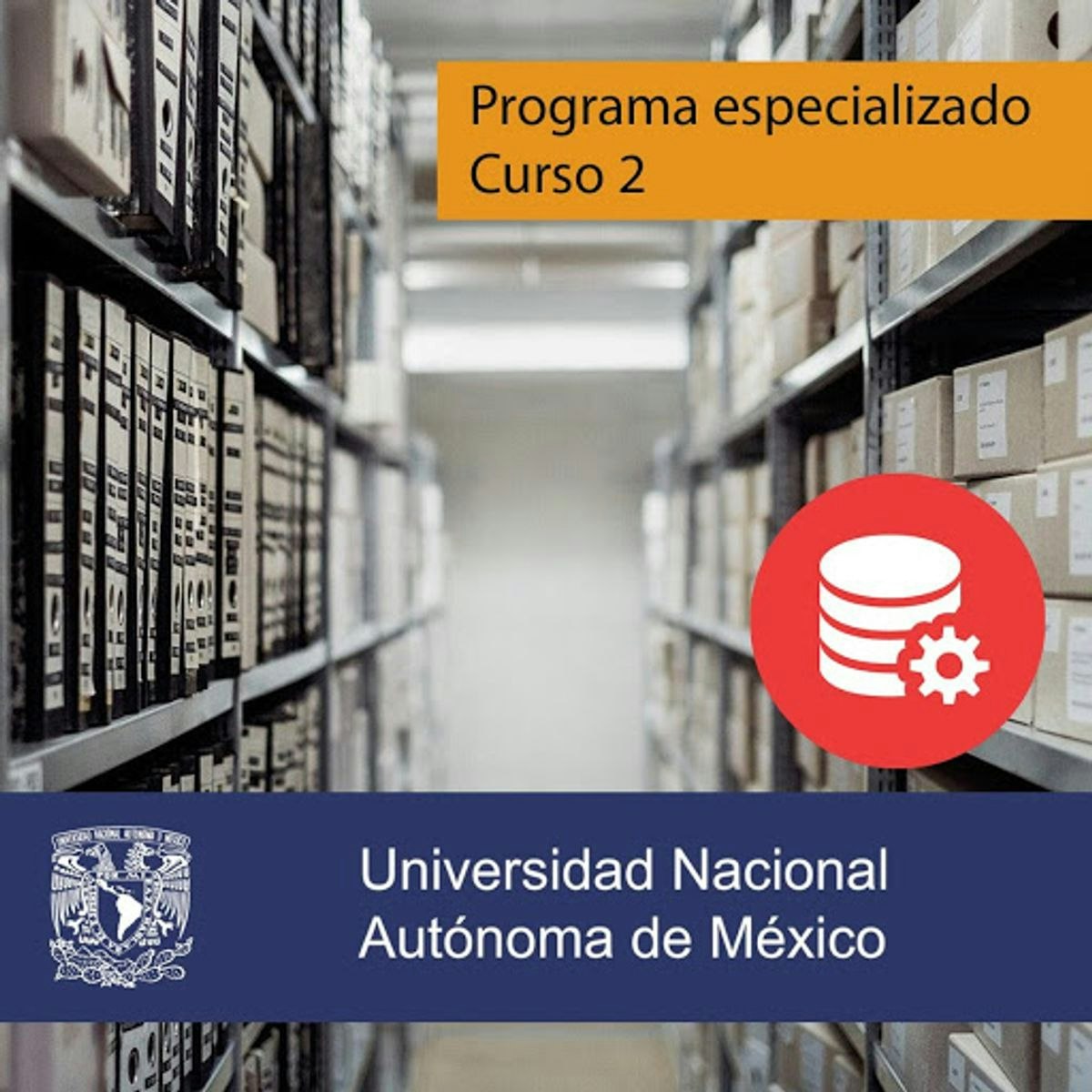
Business intelligence and data warehousing
Welcome to the specialization course Business Intelligence and Data Warehousing. This course will be completed on six weeks, it will be supported with videos and various documents that will allow you to learn in a very simple way how to identify, design and develop analytical information systems, such as Business Intelligence with a descriptive analysis on data warehouses. You will be able to understand the problem of integration and predictive analysis of high volume of unstructured data (big data) with data mining and the Hadoop framework.
After completing this course, a learner will be able to
● Create a Star o Snowflake data model Diagram through the Multidimensional Design from analytical business requirements and OLTP system
● Create a physical database system
● Extract, Transform and load data to a data-warehouse.
● Program analytical queries with SQL using MySQL
● Predictive analysis with RapidMiner
● Load relational or unstructured data to Hortonworks HDFS
● Execute Map-Reduce jobs to query data on HDFS for analytical purposes
Programming languages:
For course 2 you will use the MYSQL language.
Software to download:
Rapidminer
MYSQL
Excel
Hortonworks Hadoop framework
In case you have a Mac / IOS operating system you will need to use a virtual Machine (VirtualBox, Vmware).
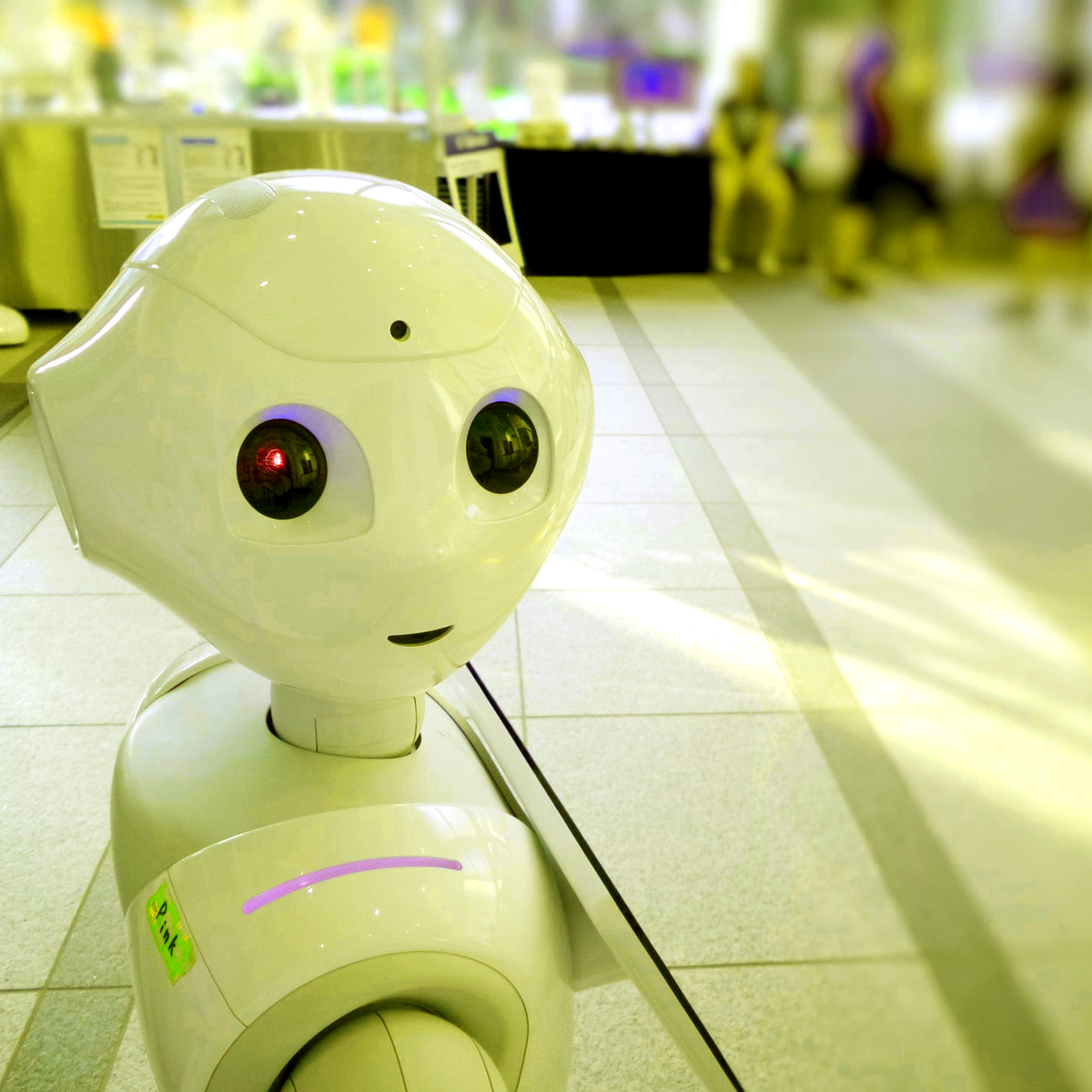
Automatic Machine Learning with H2O AutoML and Python
This is a hands-on, guided project on Automatic Machine Learning with H2O AutoML and Python. By the end of this project, you will be able to describe what AutoML is and apply automatic machine learning to a business analytics problem with the H2O AutoML interface in Python. H2O's AutoML automates the process of training and tuning a large selection of models, allowing the user to focus on other aspects of the data science and machine learning pipeline such as data pre-processing, feature engineering and model deployment.
To successfully complete the project, we recommend that you have prior experience in Python programming, basic machine learning theory, and have trained ML models with a library such as scikit-learn. We will not be exploring how any particular model works nor dive into the math behind them. Instead, we assume you have this foundational knowledge and want to learn to use H2O's AutoML interface for automatic machine learning.
Note: This course works best for learners who are based in the North America region. We’re currently working on providing the same experience in other regions.
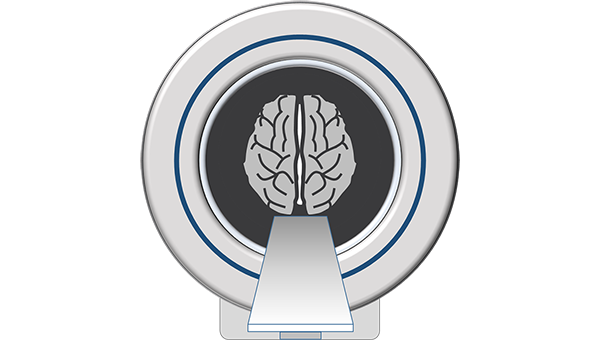
MRI Fundamentals
Welcome! In this course learners will develop expertise in basic magnetic resonance imaging (MRI) physics and principles and gain knowledge of many different data acquisition strategies in MRI. In particular, learners will get to know what is magnetic resonance phenomenon, how magnetic resonance signals are generated, how an image can be formulated using MRI, how soft tissue contrast can change with imaging parameters. Also introduced will be MR imaging sequences of spin echo, gradient echo, fast spin echo, echo planar imaging, inversion recovery, etc.
Popular Internships and Jobs by Categories
Browse
© 2024 BoostGrad | All rights reserved