Back to Courses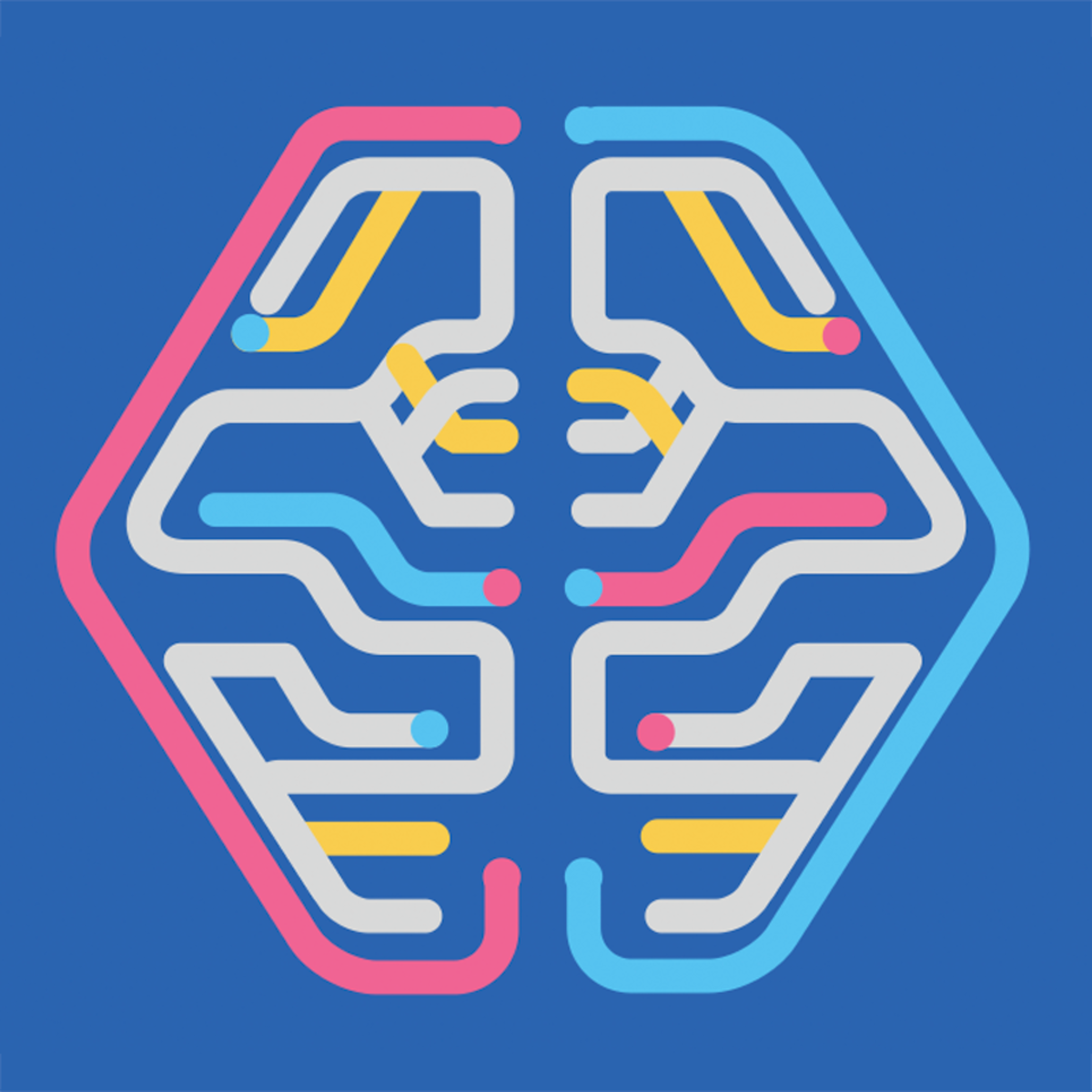
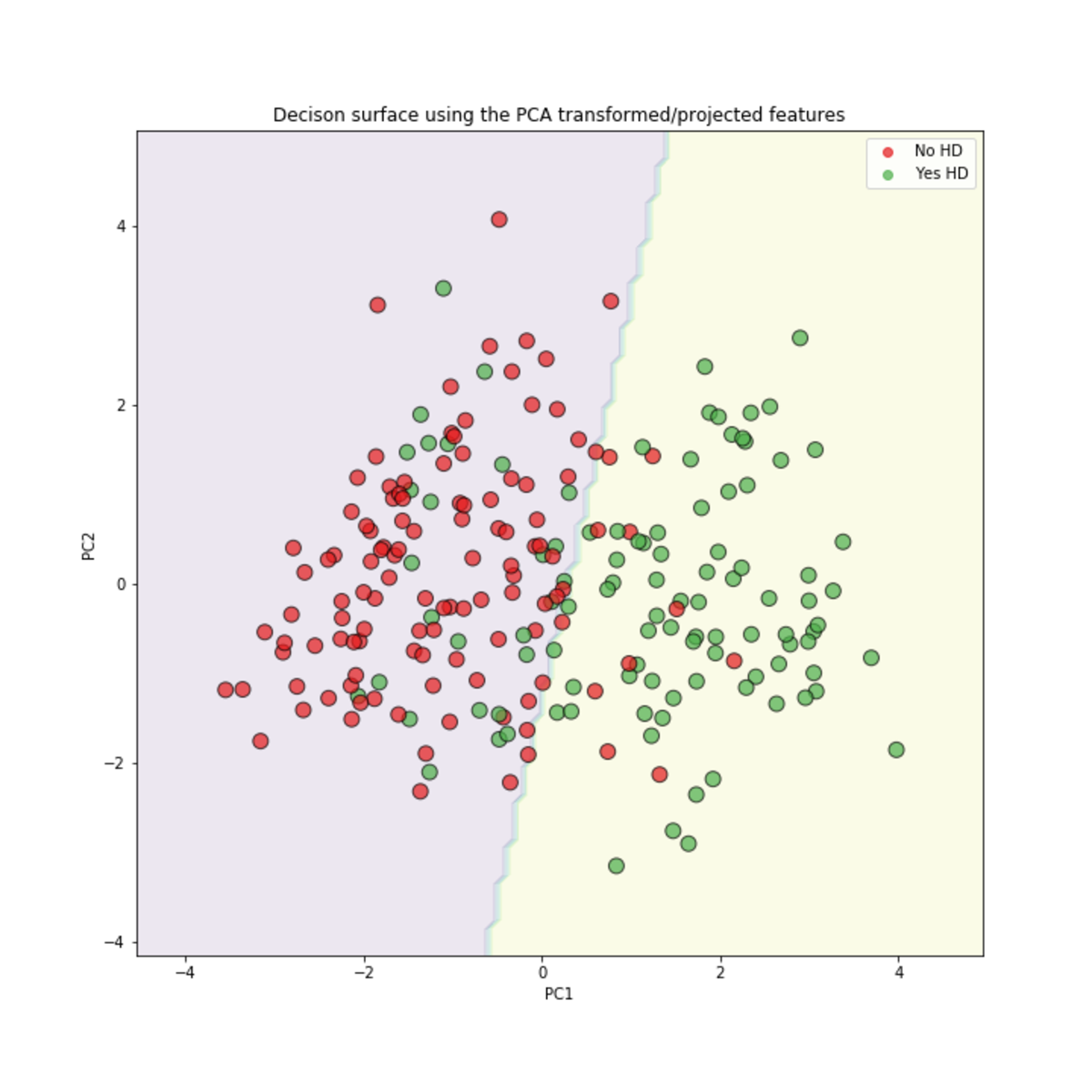
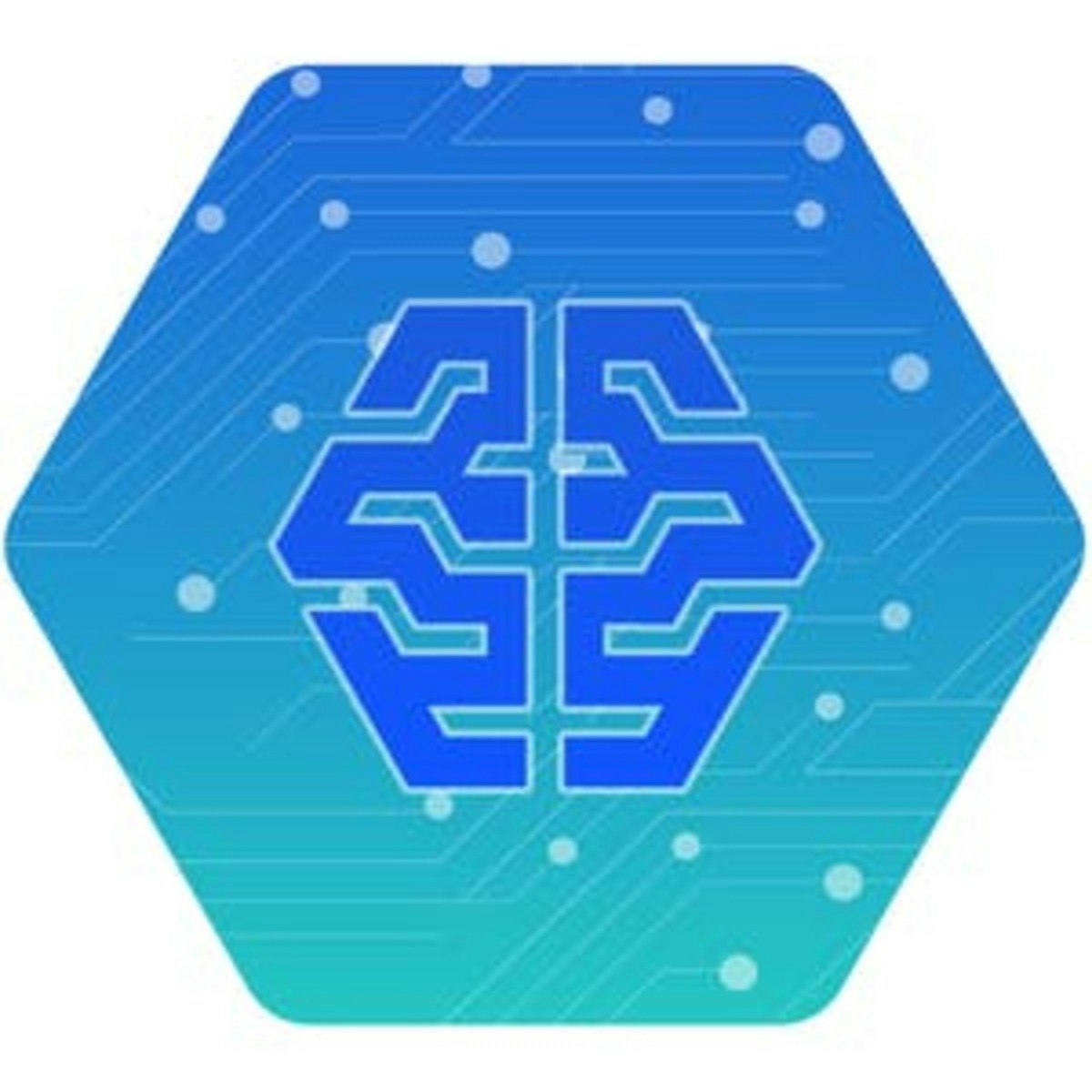
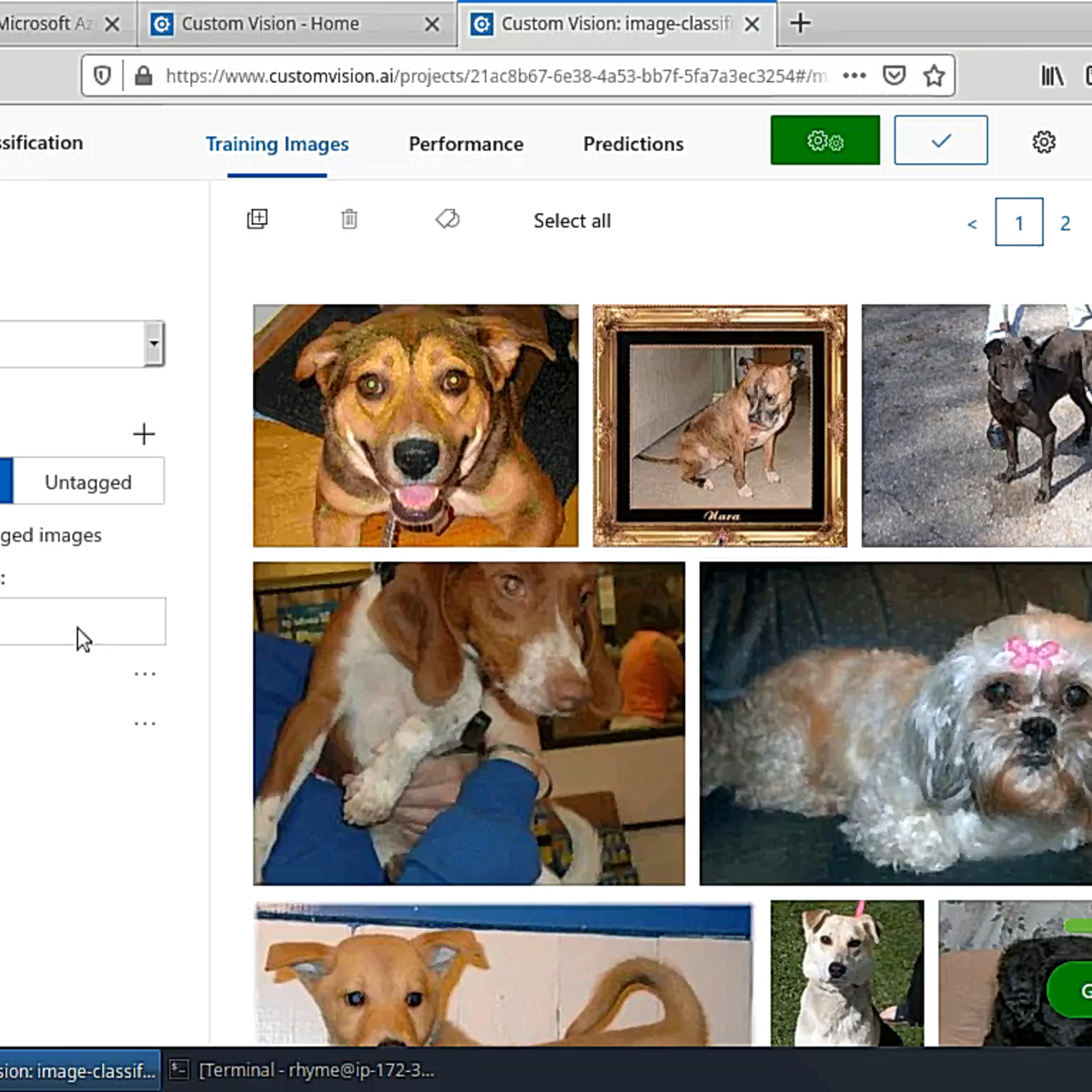
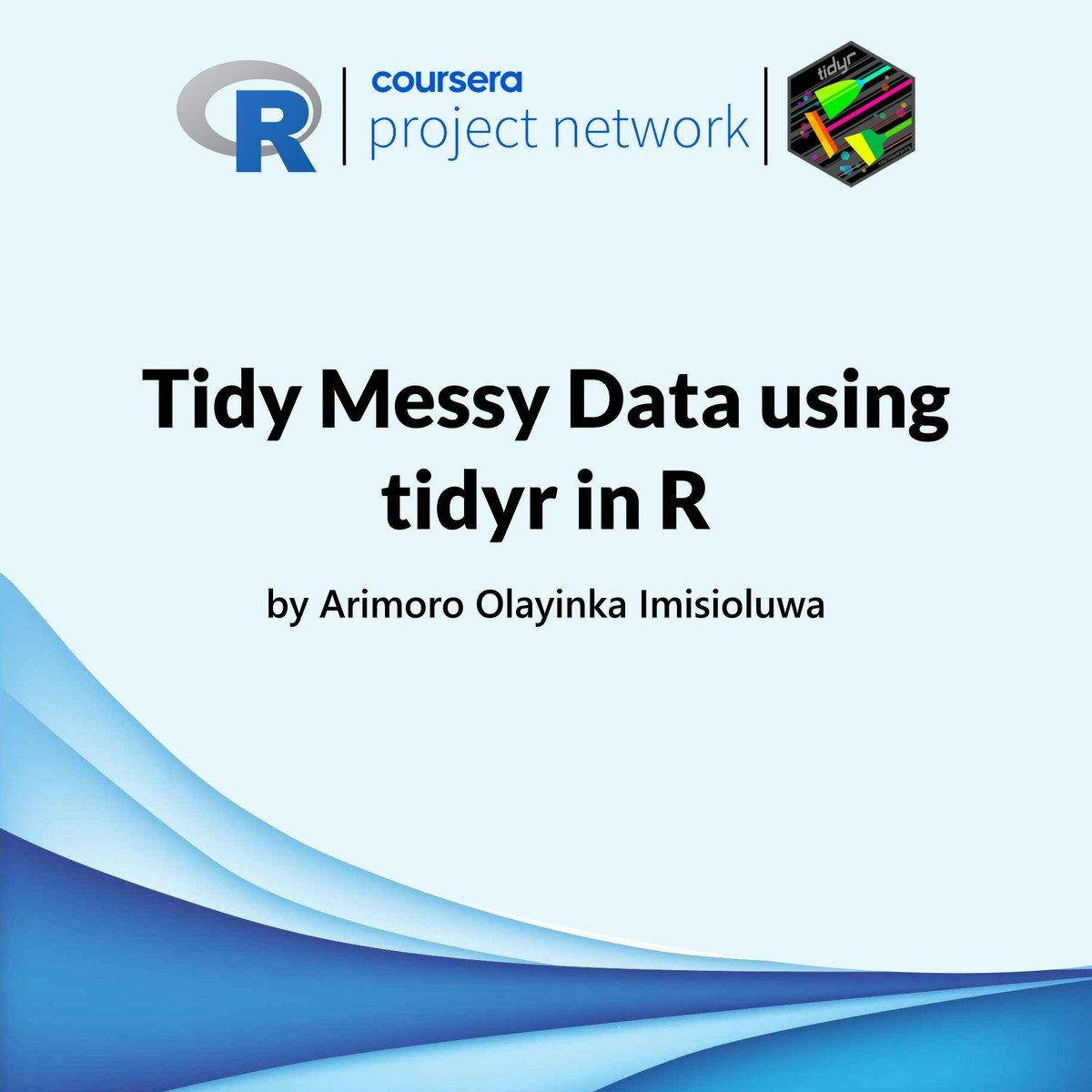
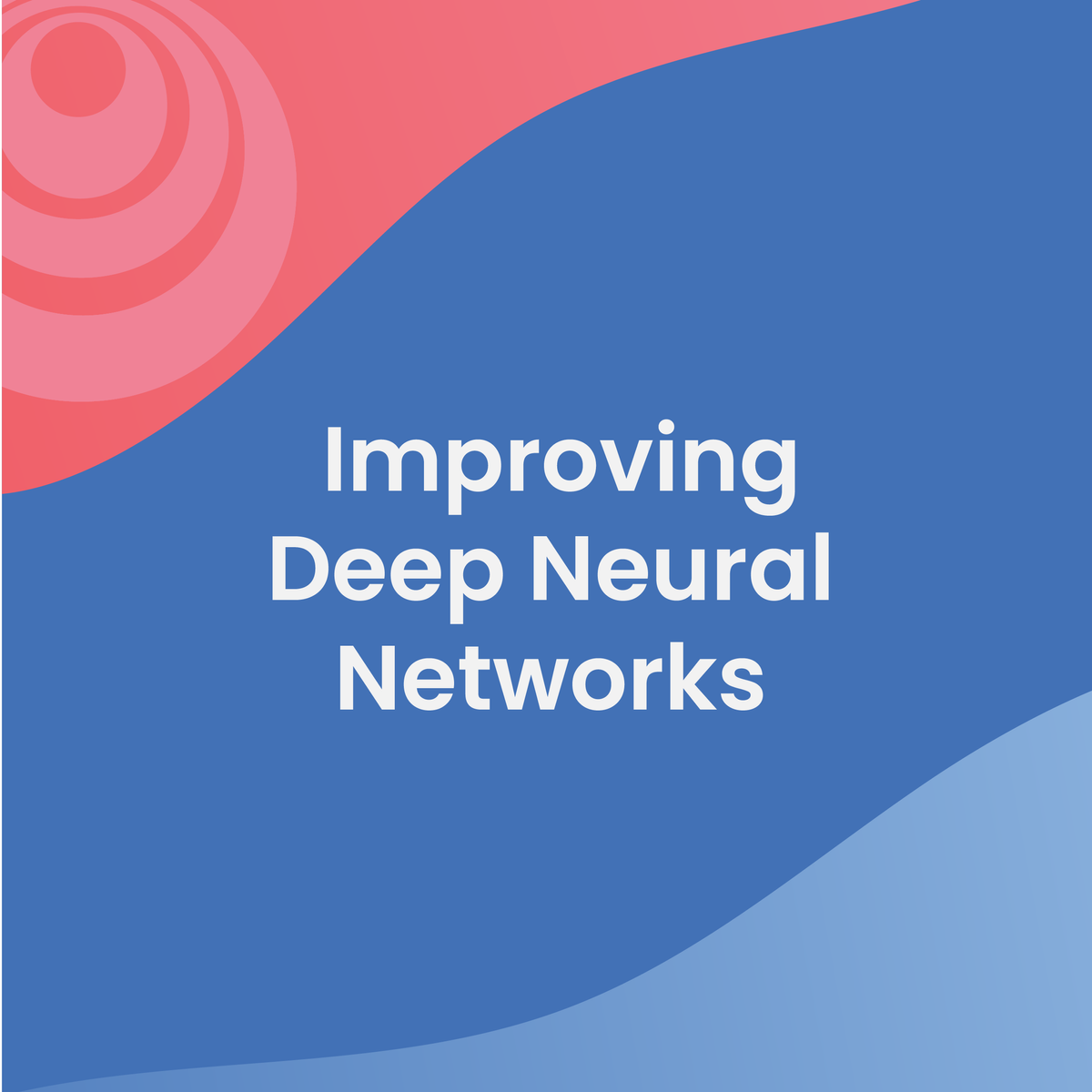
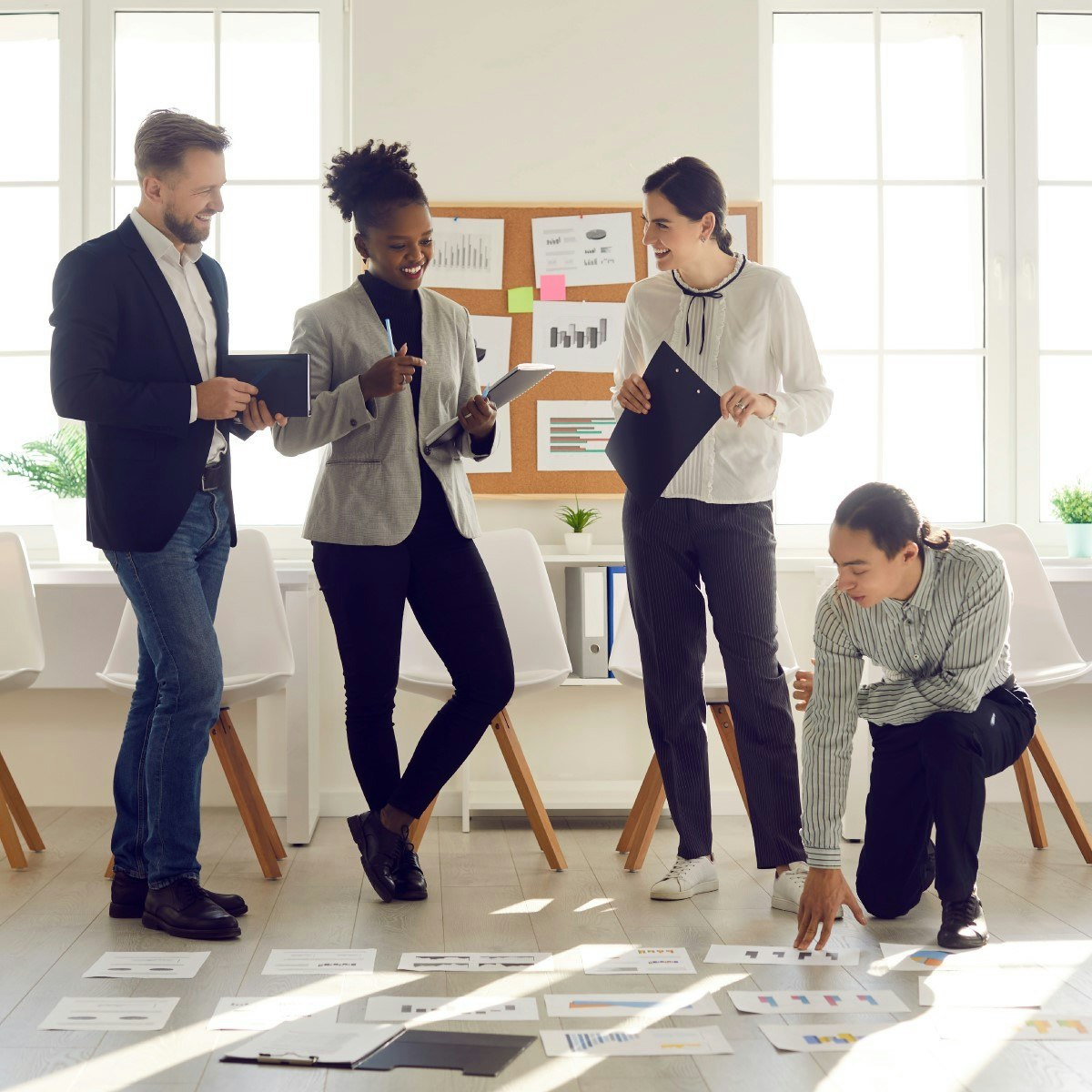
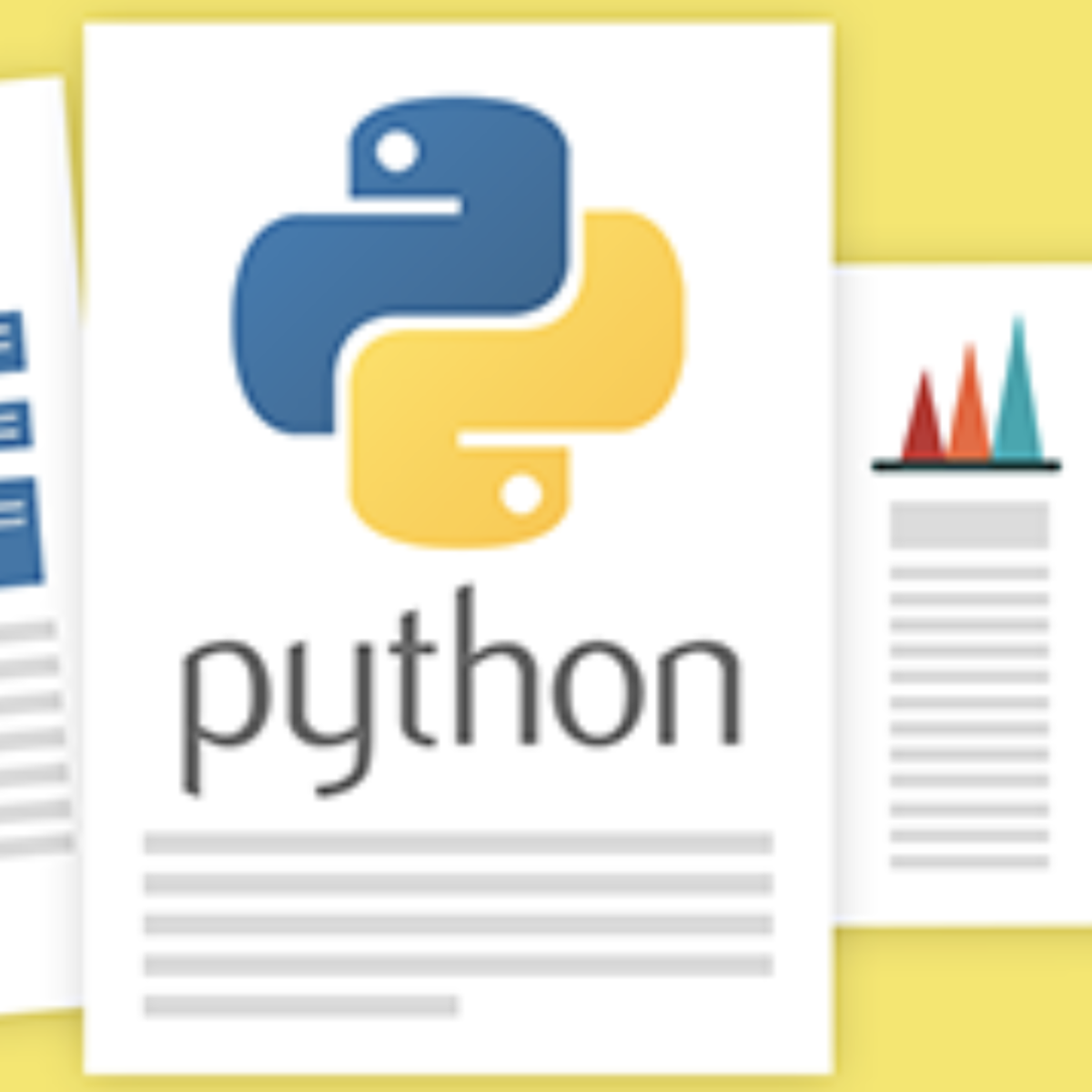
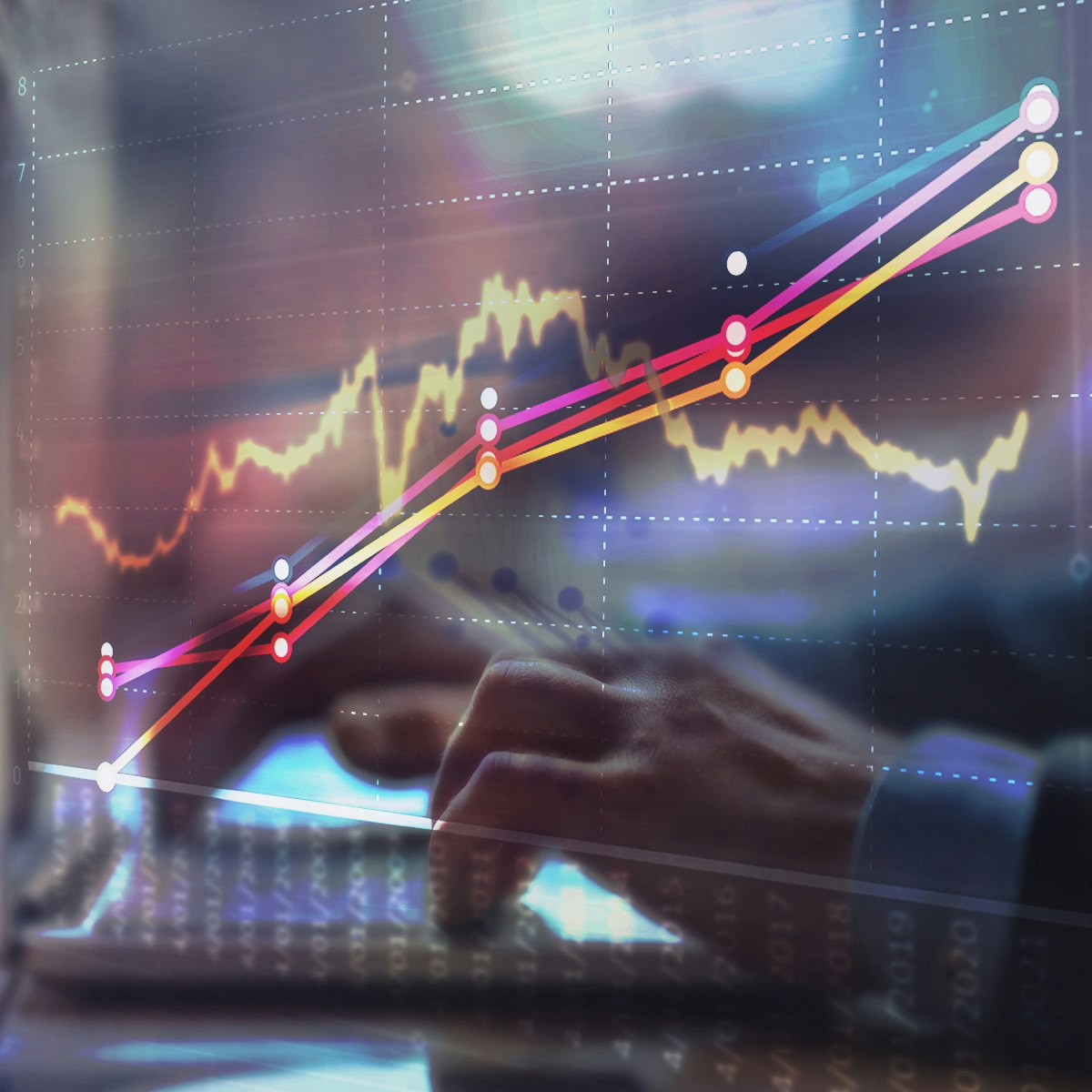
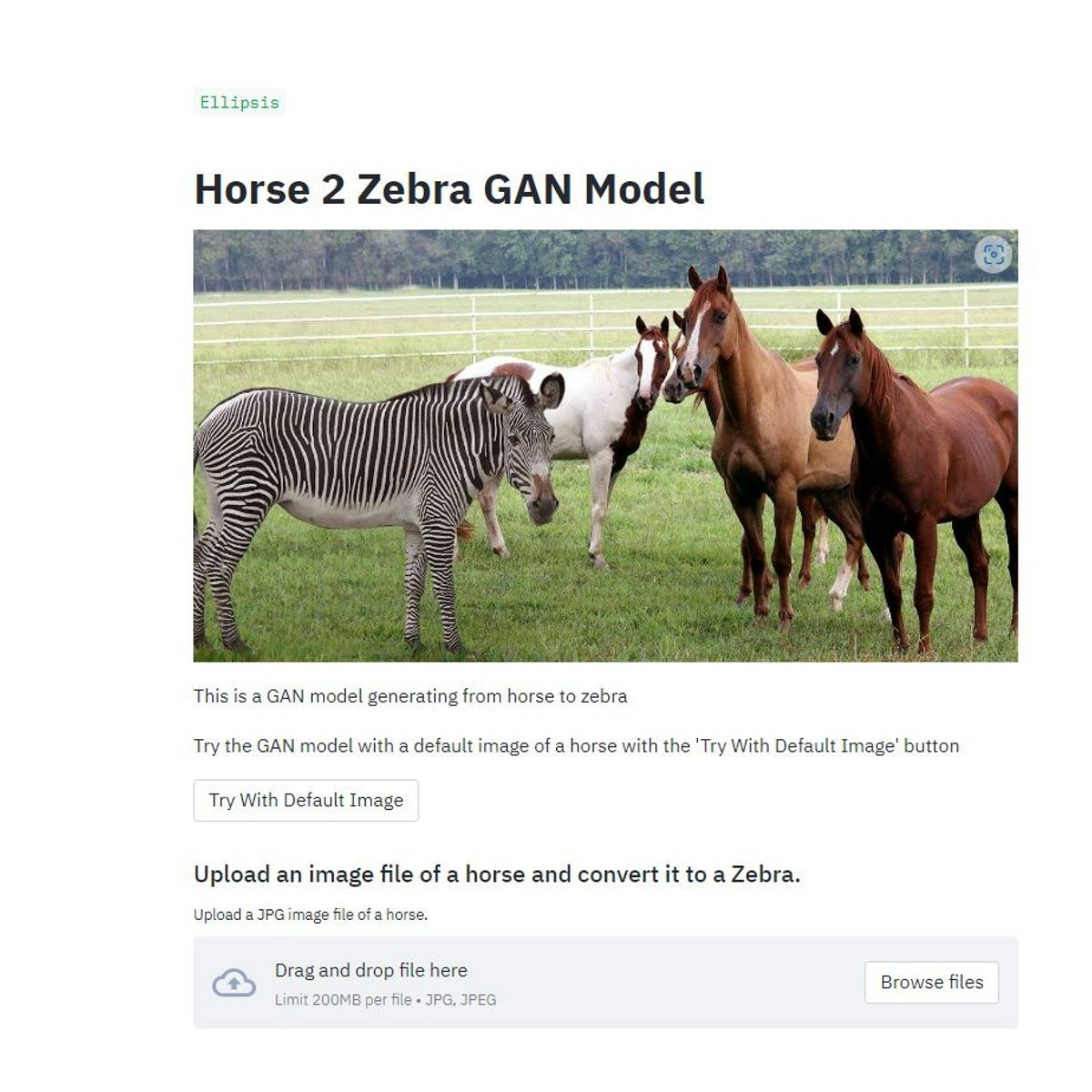
Data Science Courses - Page 16
Showing results 151-160 of 1407
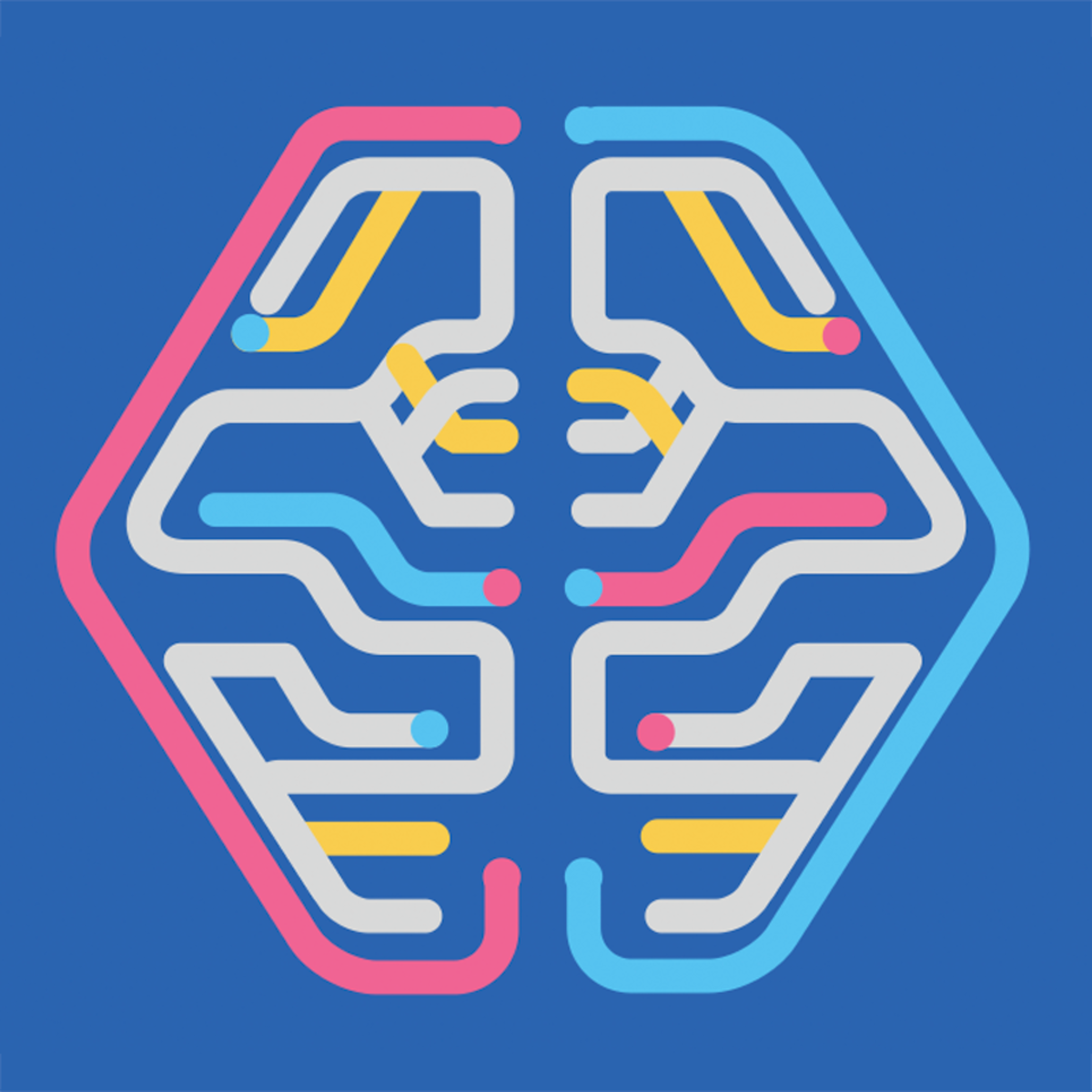
Launching into Machine Learning
The course begins with a discussion about data: how to improve data quality and perform exploratory data analysis. We describe Vertex AI AutoML and how to build, train, and deploy an ML model without writing a single line of code. You will understand the benefits of Big Query ML. We then discuss how to optimize a machine learning (ML) model and how generalization and sampling can help assess the quality of ML models for custom training.
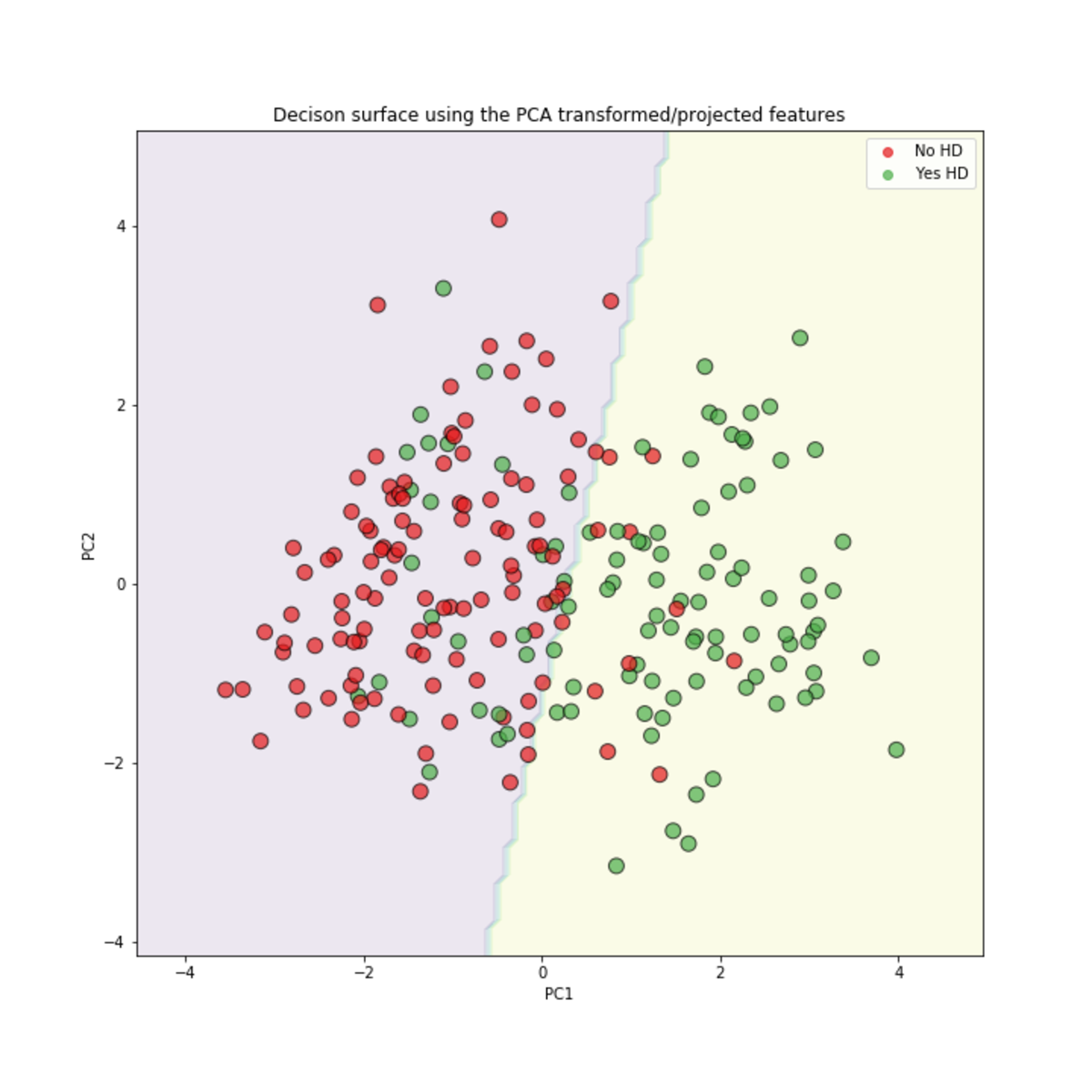
Support Vector Machines in Python, From Start to Finish
In this lesson we will built this Support Vector Machine for classification using scikit-learn and the Radial Basis Function (RBF) Kernel. Our training data set contains continuous and categorical data from the UCI Machine Learning Repository to predict whether or not a patient has heart disease.
This course runs on Coursera's hands-on project platform called Rhyme. On Rhyme, you do projects in a hands-on manner in your browser. You will get instant access to pre-configured cloud desktops containing all of the software and data you need for the project. Everything is already set up directly in your Internet browser so you can just focus on learning. For this project, you’ll get instant access to a cloud desktop with (e.g. Python, Jupyter, and Tensorflow) pre-installed.
Prerequisites:
In order to be successful in this project, you should be familiar with programming in Python and the concepts behind Support Vector Machines, the Radial Basis Function, Regularization, Cross Validation and Confusion Matrices.
Notes:
- You will be able to access the cloud desktop 5 times. However, you will be able to access instructions videos as many times as you want.
- This course works best for learners who are based in the North America region. We’re currently working on providing the same experience in other regions.
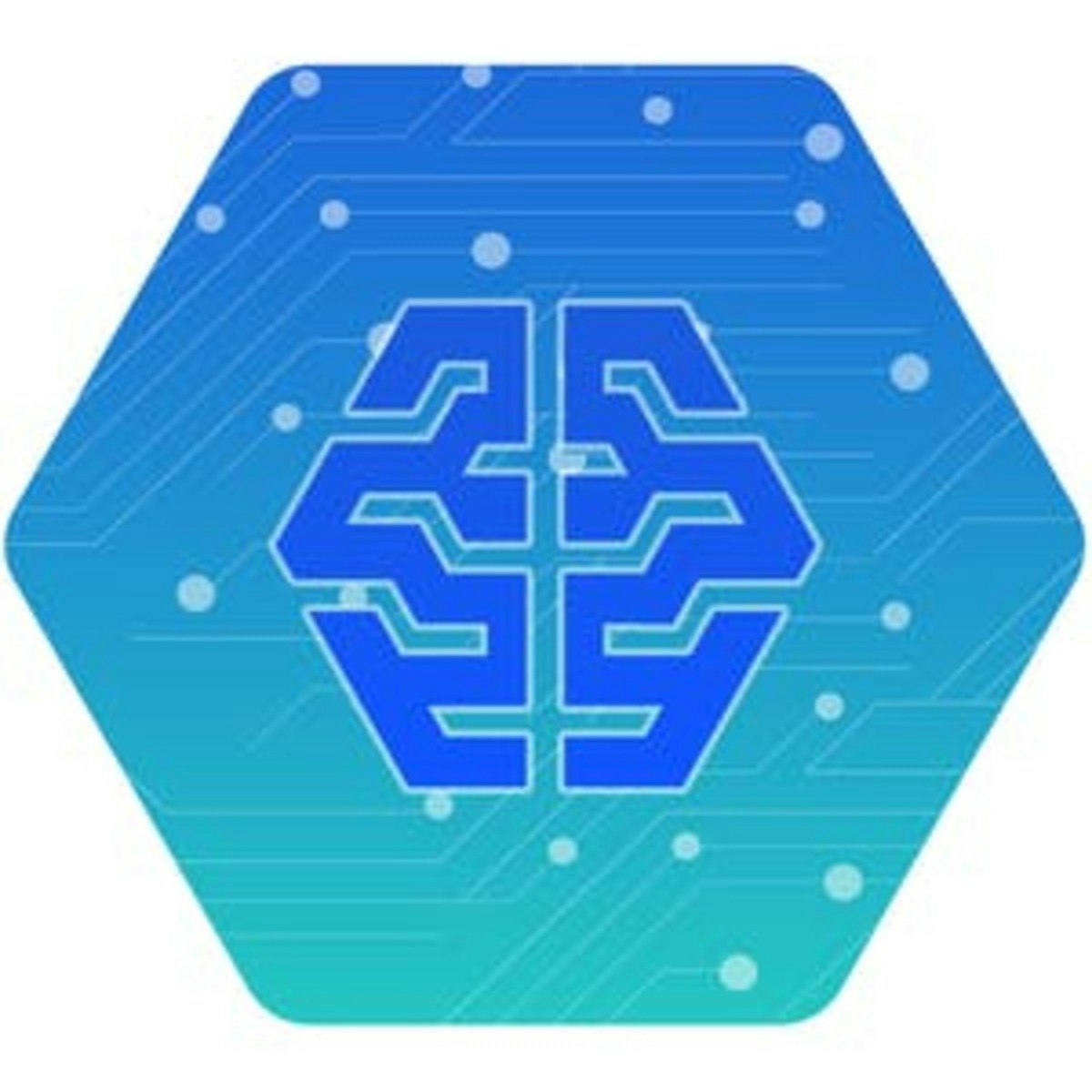
Smart Analytics, Machine Learning, and AI on GCP
Incorporating machine learning into data pipelines increases the ability of businesses to extract insights from their data. This course covers several ways machine learning can be included in data pipelines on Google Cloud depending on the level of customization required. For little to no customization, this course covers AutoML. For more tailored machine learning capabilities, this course introduces Notebooks and BigQuery machine learning (BigQuery ML). Also, this course covers how to productionalize machine learning solutions using Vertex AI. Learners will get hands-on experience building machine learning models on Google Cloud using QwikLabs.
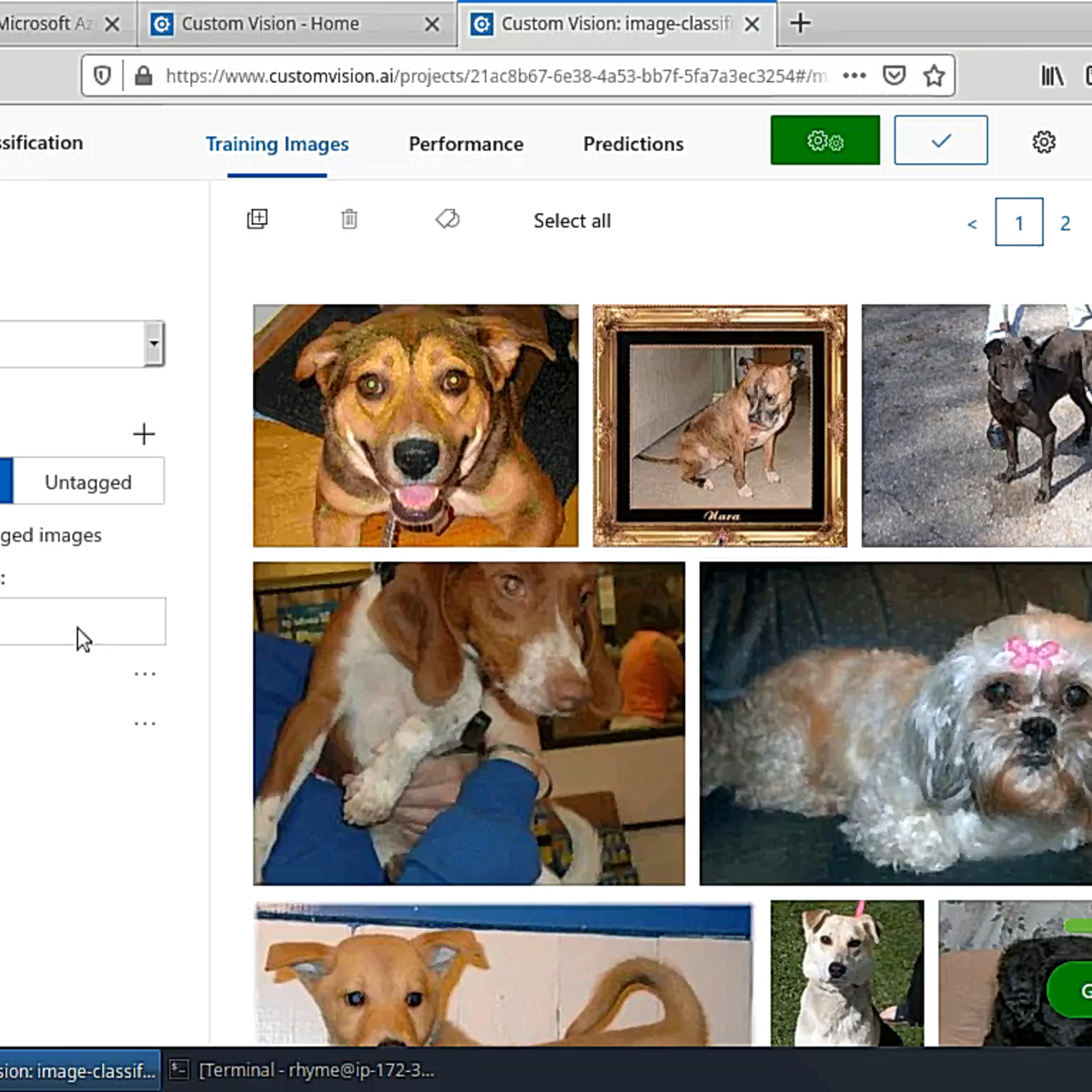
AutoML for Computer Vision with Microsoft Custom Vision
Welcome to this hands-on project on using Microsoft’s Custom Vision service for automated machine learning or AutoML as it’s popularly known. In this project, you are going to use Microsoft’s drag and drop tool to train your computer to recognize images of dogs and cats. We are going to do all of this without writing a single line of code! To take this guided-project, you do not need a background in computer science, machine learning or coding.
The only prerequisite for this project is that you have a Microsoft Azure account. If you don’t already have one, you will have to sign up for it.
Note: This course works best for learners who are based in the North America region. We’re currently working on providing the same experience in other regions.
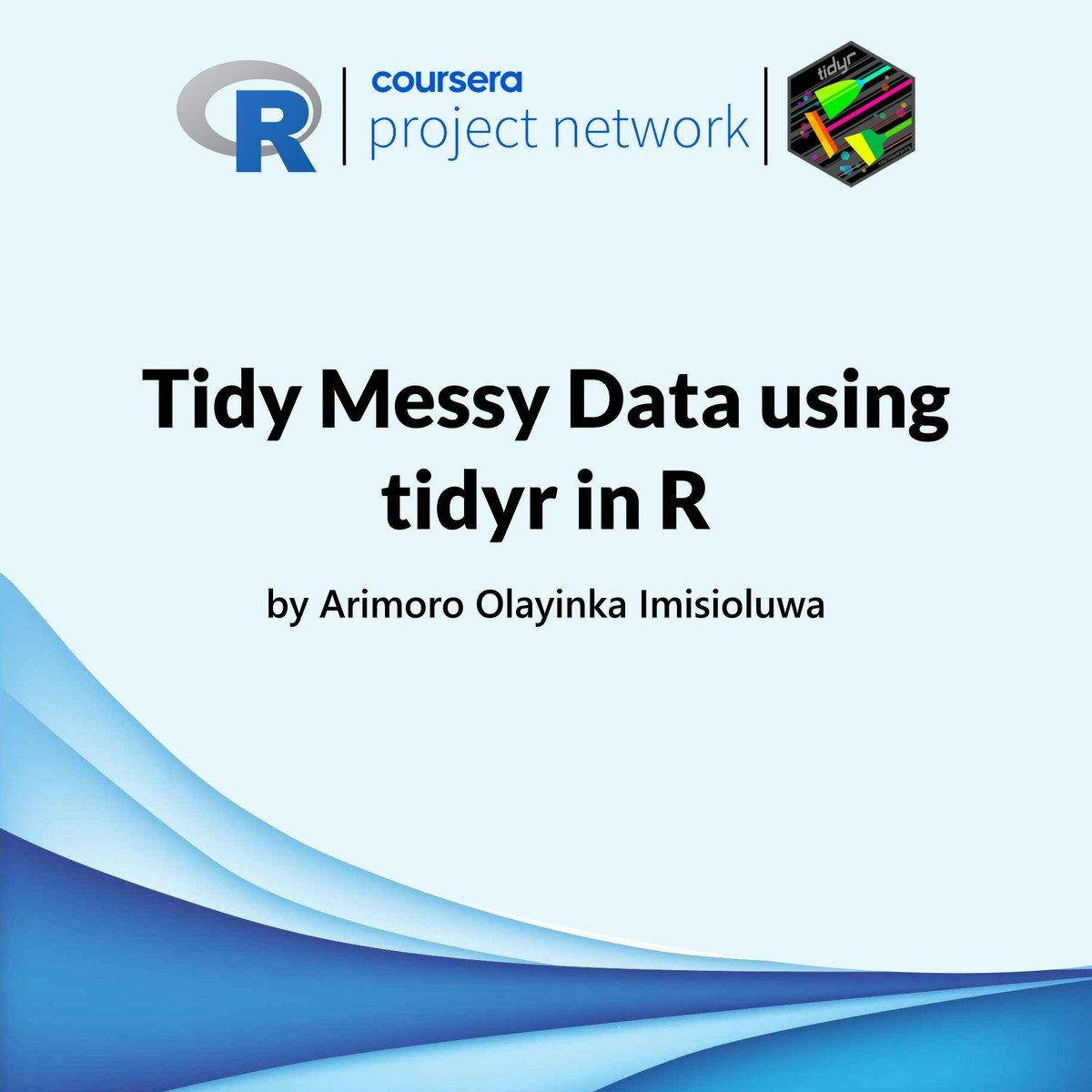
Tidy Messy Data using tidyr in R
As data enthusiasts and professionals, our work often requires dealing with data in different forms. In particular, messy data can be a big challenge because the quality of your analysis largely depends on the quality of the data. This project-based course, "Tidy Messy Data using tidyr in R," is intended for beginner and intermediate R users with related experiences who are willing to advance their knowledge and skills.
In this course, you will learn practical ways for data cleaning, reshaping, and transformation using R. You will learn how to use different tidyr functions like pivot_longer(), pivot_wider(), separate_rows(), separate(), and others to achieve the tidy data principles. By the end of this 2-hour-long project, you will get hands-on massaging data to put in the proper format. By extension, you will learn to create plots using ggplot().
This project-based course is a beginner to an intermediate-level course in R. Therefore, to get the most out of this project, it is essential to have a basic understanding of using R. Specifically, you should be able to load data into R and understand how the pipe function works. It will be helpful to complete my previous project titled "Data Manipulation with dplyr in R."
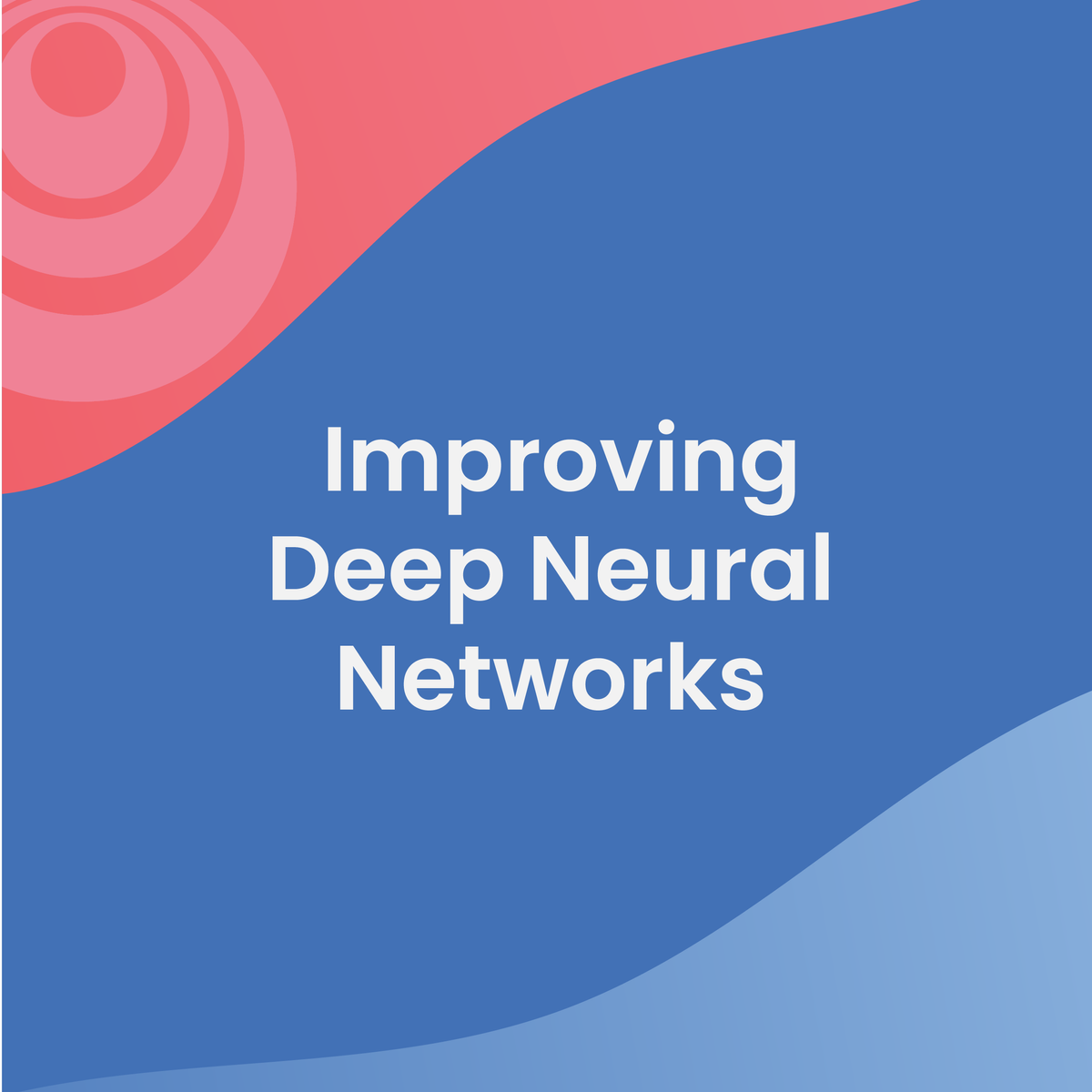
Improving Deep Neural Networks: Hyperparameter Tuning, Regularization and Optimization
In the second course of the Deep Learning Specialization, you will open the deep learning black box to understand the processes that drive performance and generate good results systematically.
By the end, you will learn the best practices to train and develop test sets and analyze bias/variance for building deep learning applications; be able to use standard neural network techniques such as initialization, L2 and dropout regularization, hyperparameter tuning, batch normalization, and gradient checking; implement and apply a variety of optimization algorithms, such as mini-batch gradient descent, Momentum, RMSprop and Adam, and check for their convergence; and implement a neural network in TensorFlow.
The Deep Learning Specialization is our foundational program that will help you understand the capabilities, challenges, and consequences of deep learning and prepare you to participate in the development of leading-edge AI technology. It provides a pathway for you to gain the knowledge and skills to apply machine learning to your work, level up your technical career, and take the definitive step in the world of AI.
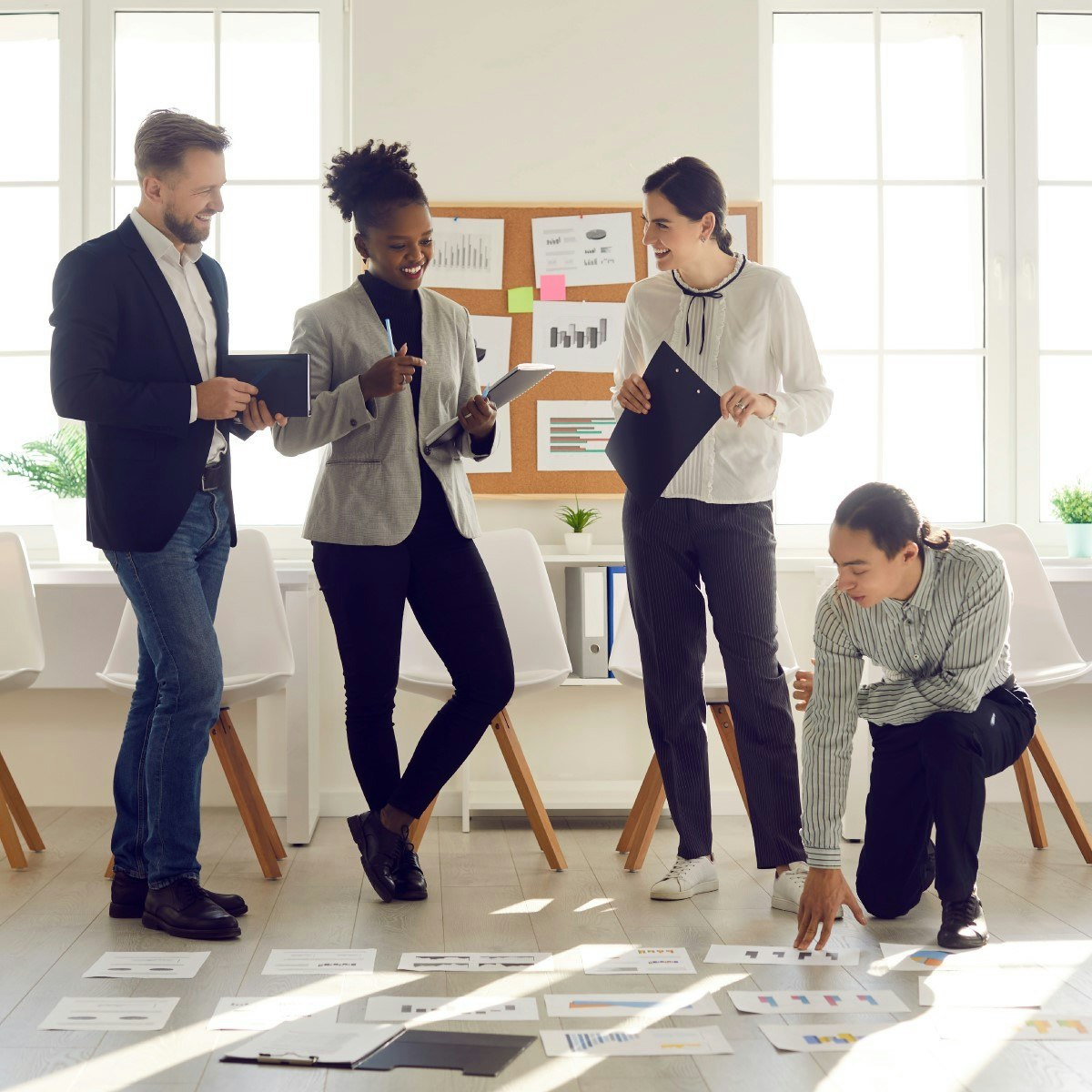
Data Analyst Career Guide and Interview Preparation
This course is designed to prepare you to enter the job market as a data analyst. It provides guidance about the regular functions and tasks of data analysts and their place in the data ecosystem, as well as the opportunities of the profession and some options for career development. It explains practical techniques for creating essential job-seeking materials such as a resume and a portfolio, as well as auxiliary tools like a cover letter and an elevator pitch. You will learn how to find and assess prospective job positions, apply to them, and lay the groundwork for interviewing. You will also get inside tips and steps you can use to perform professionally and effectively at interviews. Let seasoned professionals share their experience to help you get ahead of the competition.
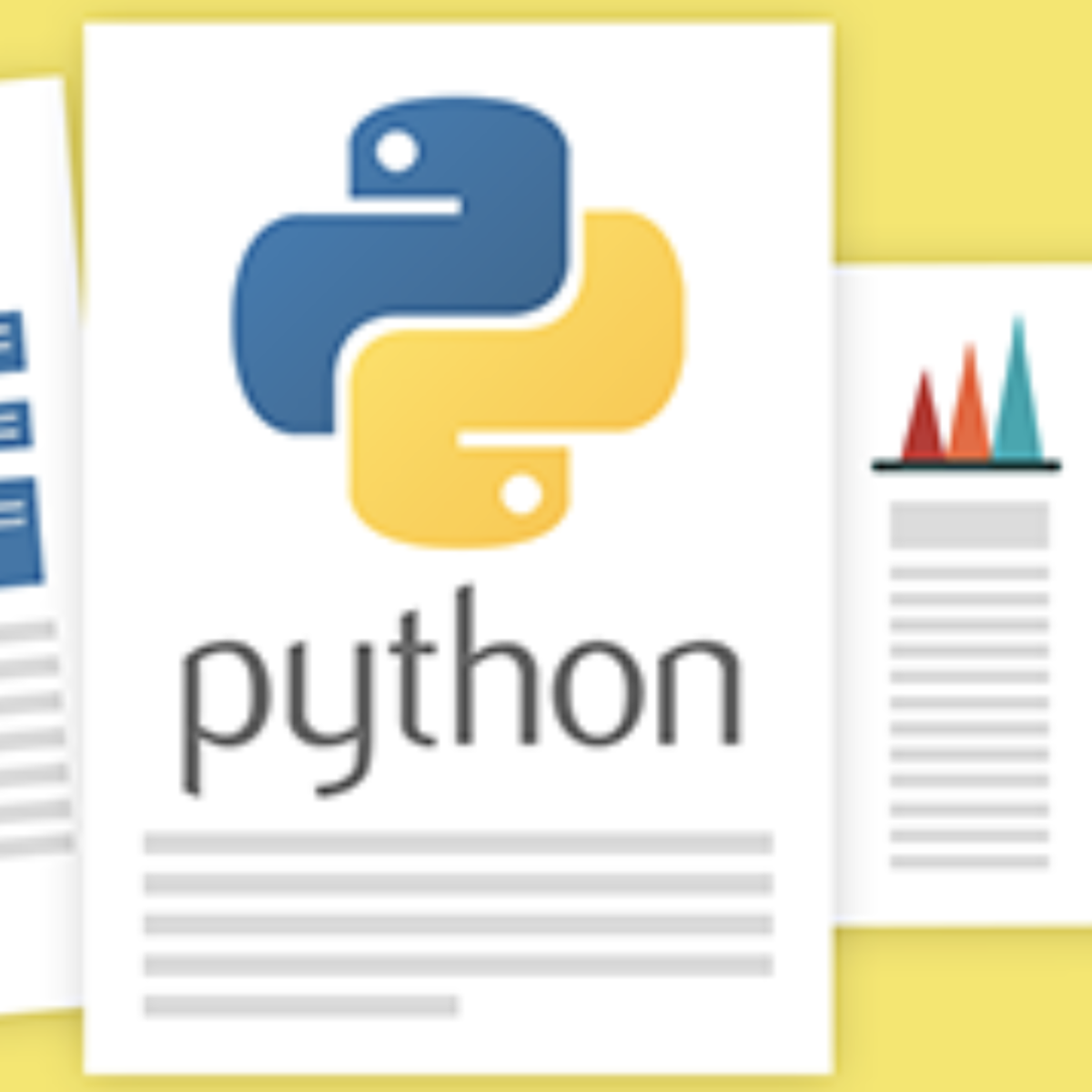
Data Analysis with Python
Analyzing data with Python is an essential skill for Data Scientists and Data Analysts. This course will take you from the basics of data analysis with Python to building and evaluating data models.
Topics covered include:
- collecting and importing data
- cleaning, preparing & formatting data
- data frame manipulation
- summarizing data,
- building machine learning regression models
- model refinement
- creating data pipelines
You will learn how to import data from multiple sources, clean and wrangle data, perform exploratory data analysis (EDA), and create meaningful data visualizations. You will then predict future trends from data by developing linear, multiple, polynomial regression models & pipelines and learn how to evaluate them.
In addition to video lectures you will learn and practice using hands-on labs and projects. You will work with several open source Python libraries, including Pandas and Numpy to load, manipulate, analyze, and visualize cool datasets. You will also work with scipy and scikit-learn, to build machine learning models and make predictions.
If you choose to take this course and earn the Coursera course certificate, you will also earn an IBM digital badge.
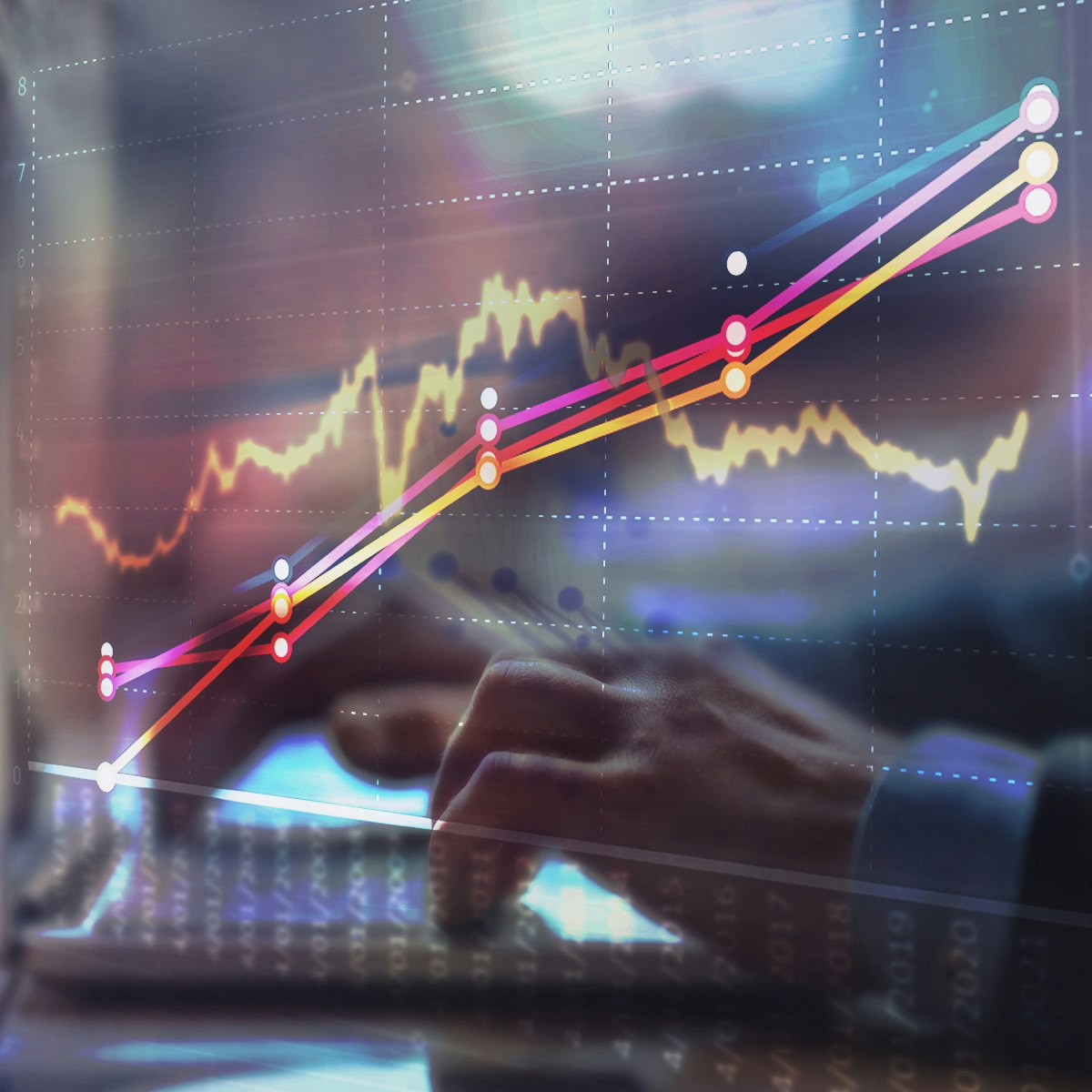
Excel Time Series Models for Business Forecasting
This course explores different time series business forecasting methods. The course covers a variety of business forecasting methods for different types of components present in time series data — level, trending, and seasonal. We will learn about the theoretical methods and apply these methods to business data using Microsoft Excel. These forecasting methods will be programmed into Microsoft Excel, displayed graphically, and we will optimise these models to produce accurate forecasts. We will compare different models and their forecasts to decide which model best suits our business' needs.
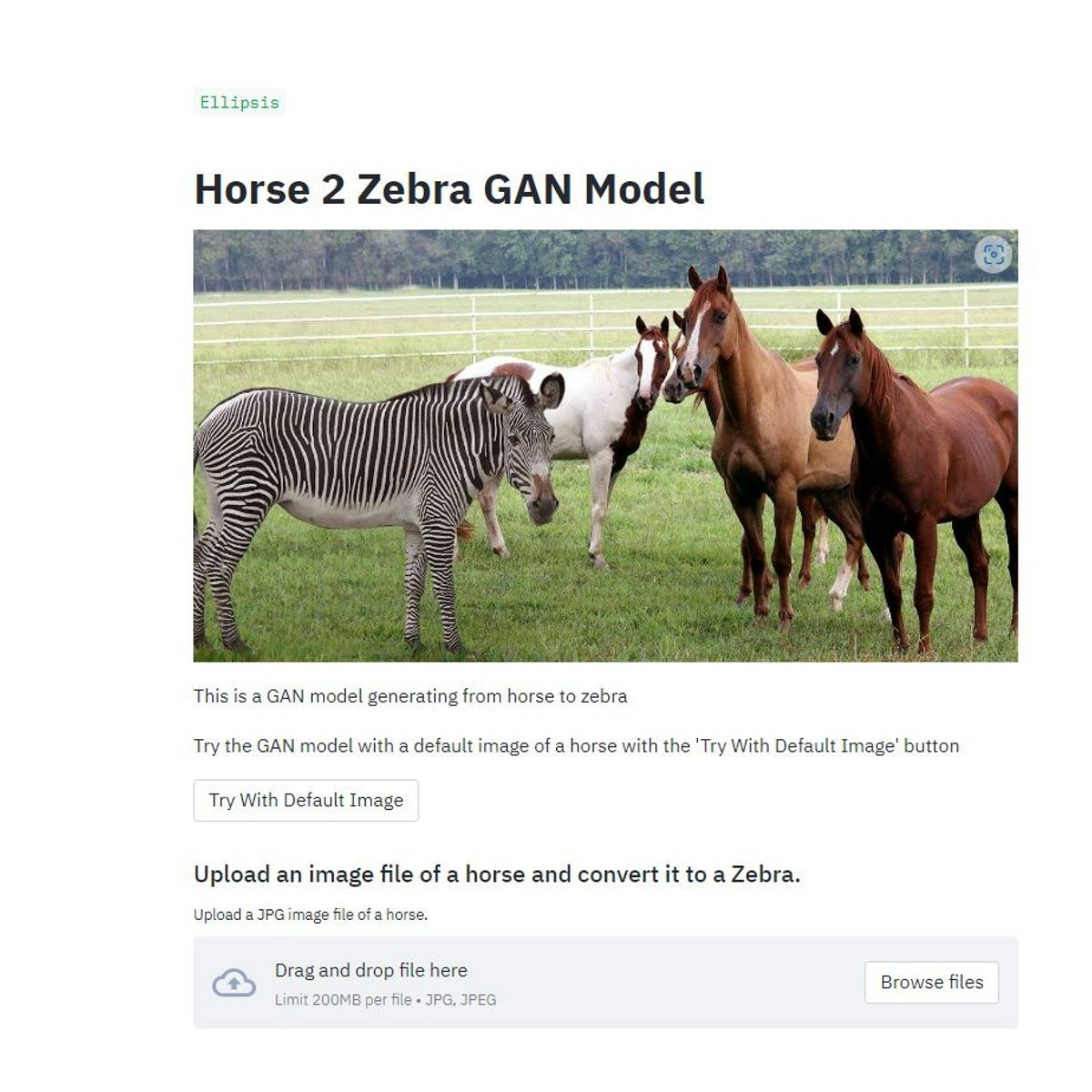
Building a Keras Horse Zebra CycleGAN Webapp with Streamlit
Welcome to the “Building a Keras Horse Zebra CycleGAN Webapp with Streamlit” guided project.
In this project, we will build a Streamlit web app of a keras trained computer vision CycleGAN for horse images to zebra and vice versa. This project will take a jpg image of a horse and transform it into a zebra and take a picture of a zebra and transform it to a horse.
This project is an intermediate python project for anyone interested in learning about how to productionize computer vision models or more specifically a beginner GAN model with Streamlit and Python.
It requires preliminary knowledge on how to build and train GAN models (as we will not be building or training models) but we will be using a model that has already been trained and provided in the workspace.
