Back to Courses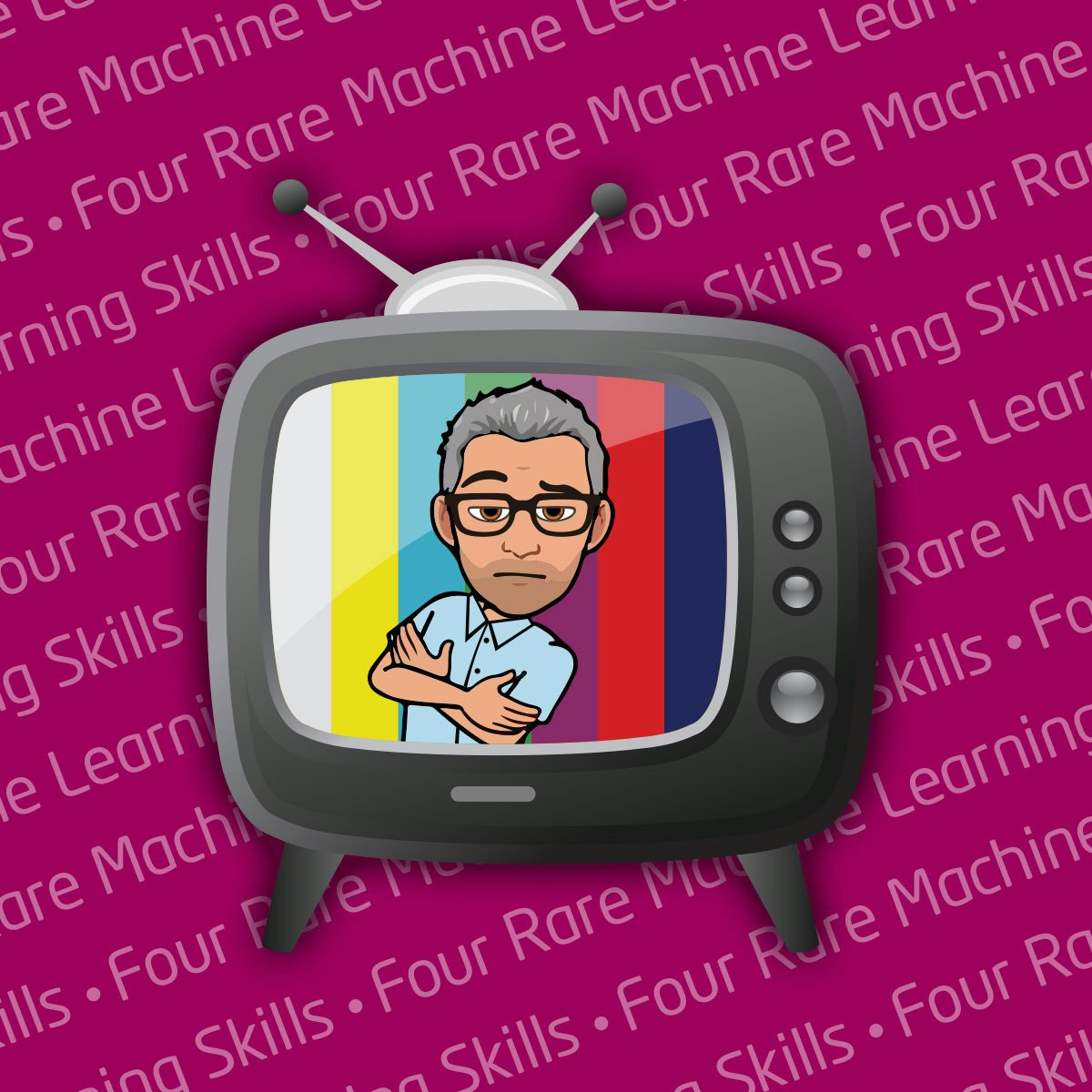
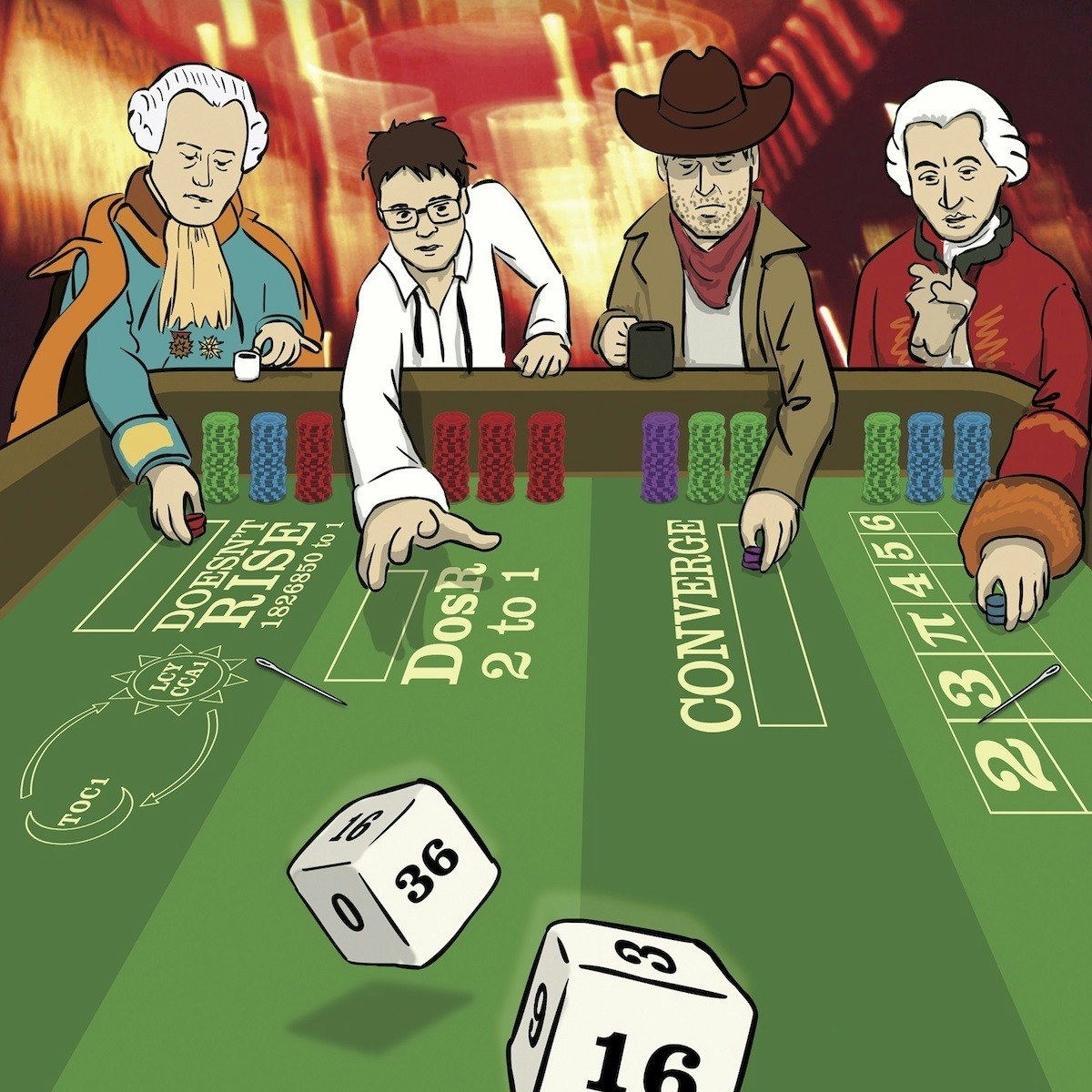
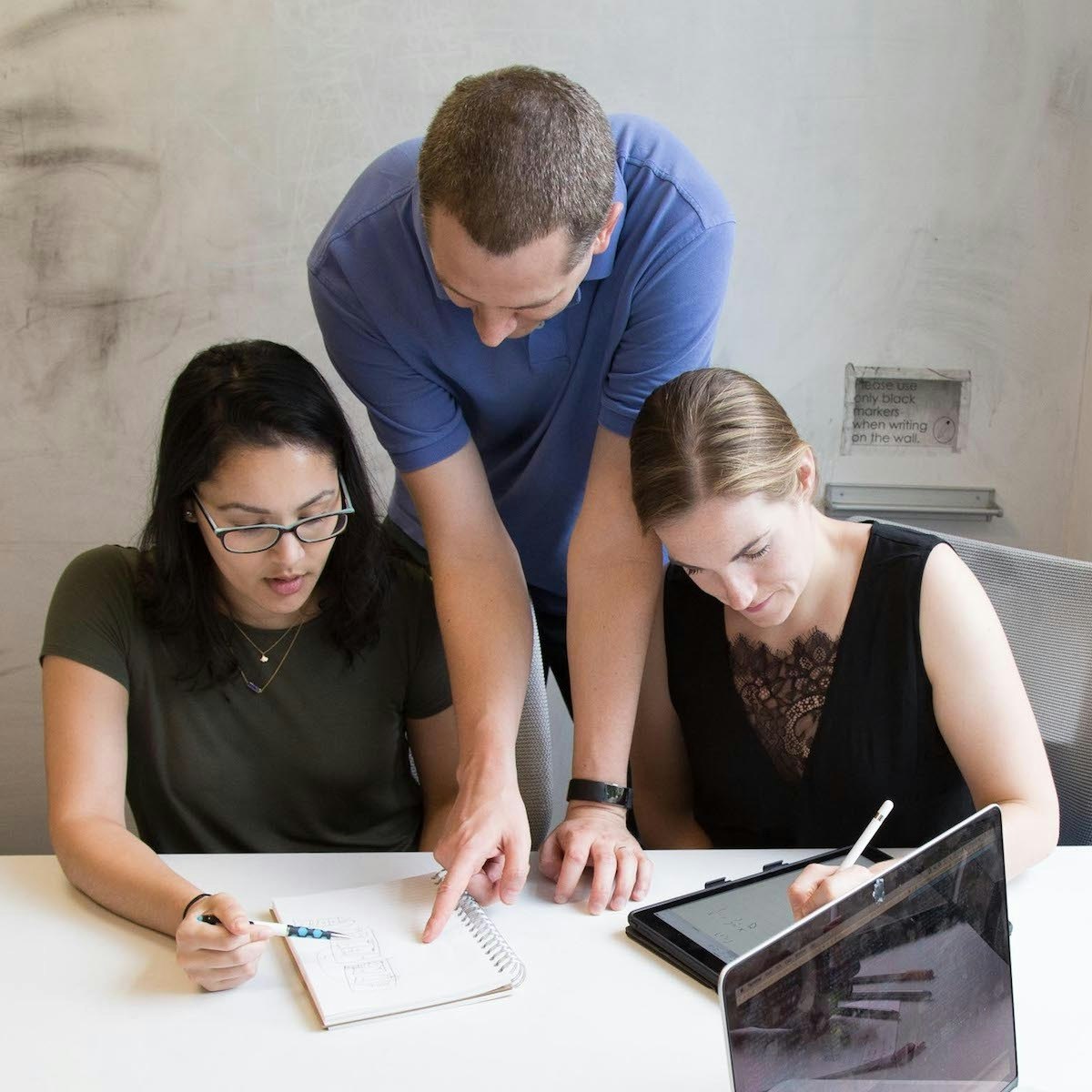
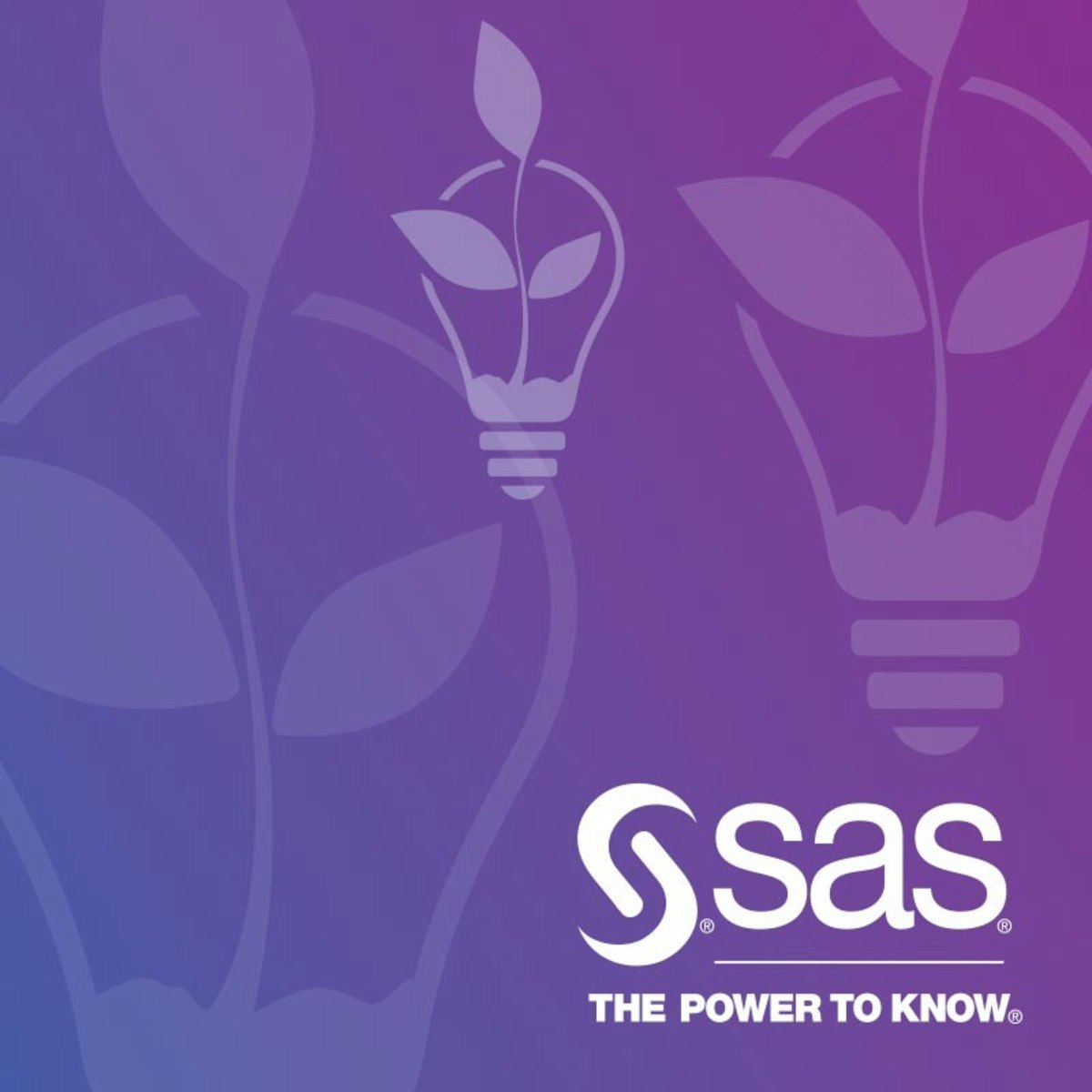
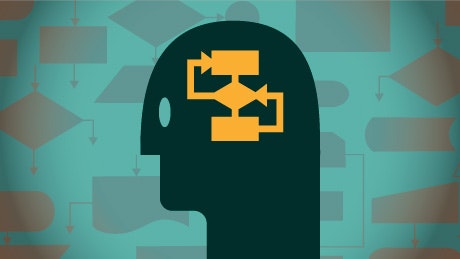
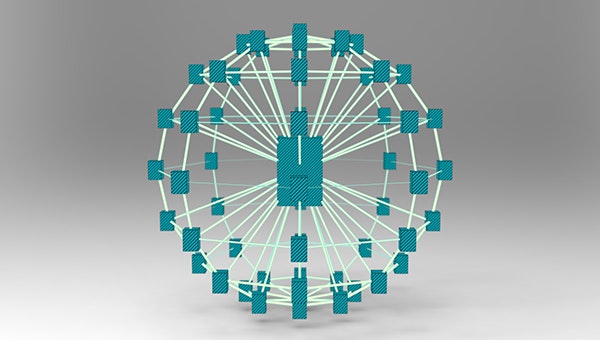
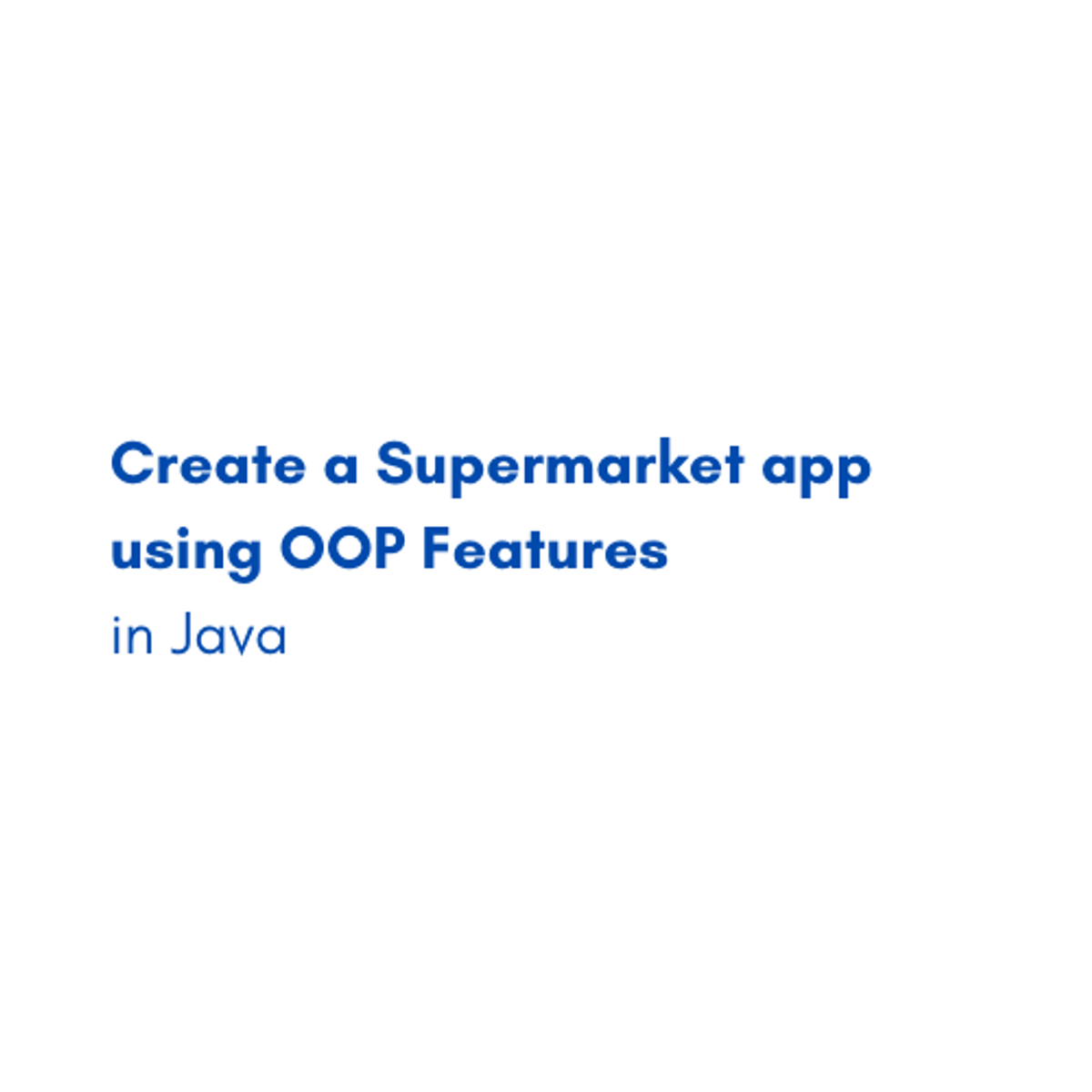
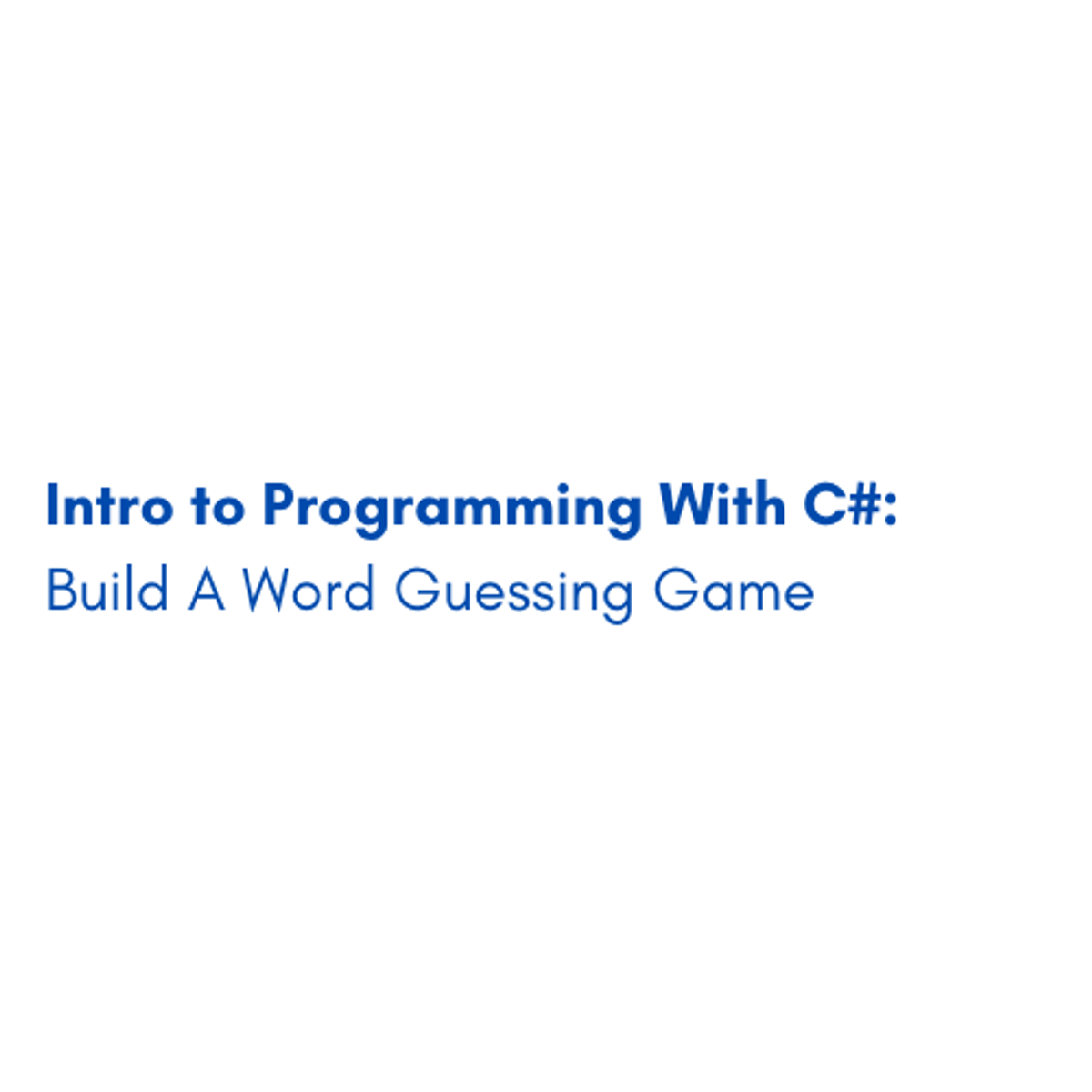
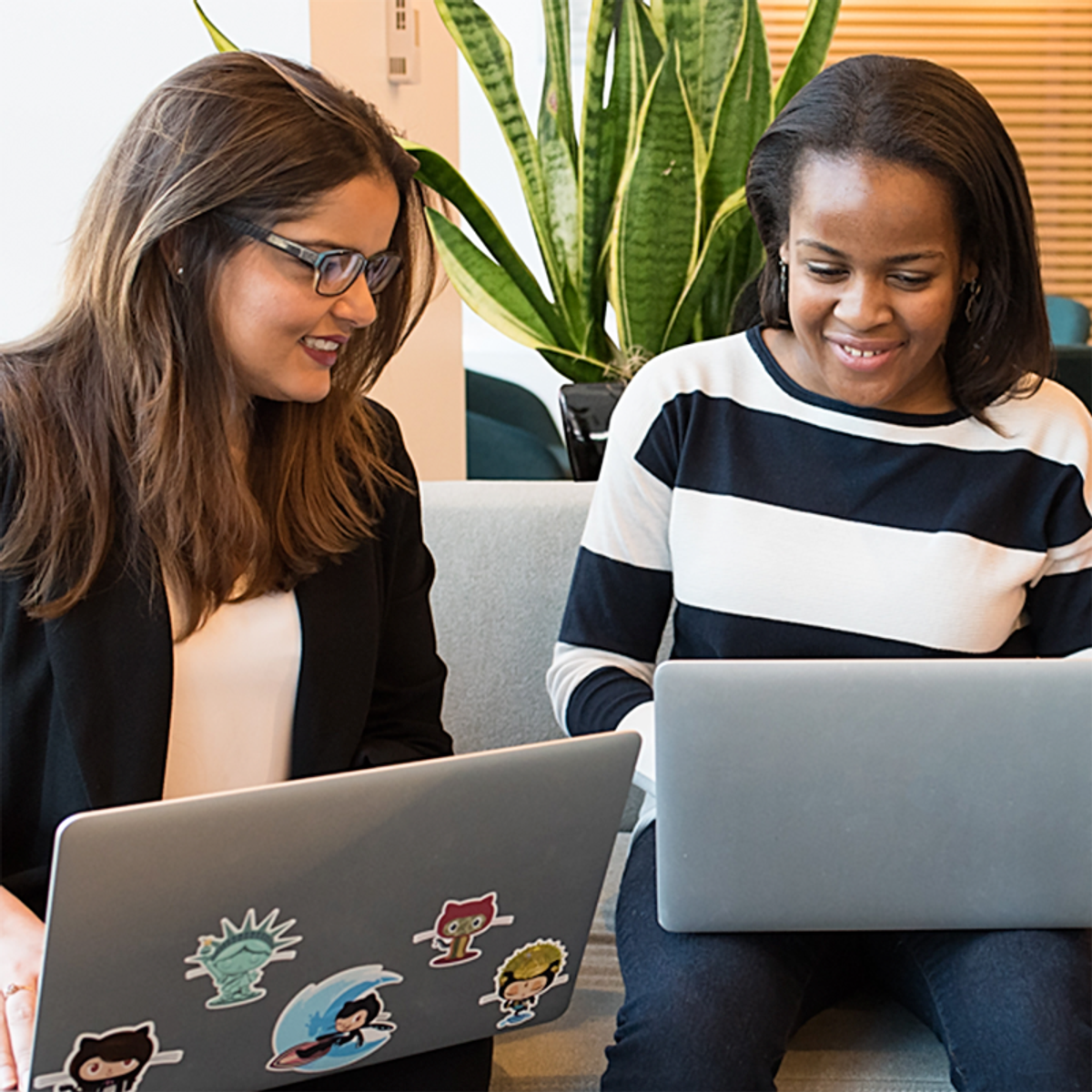
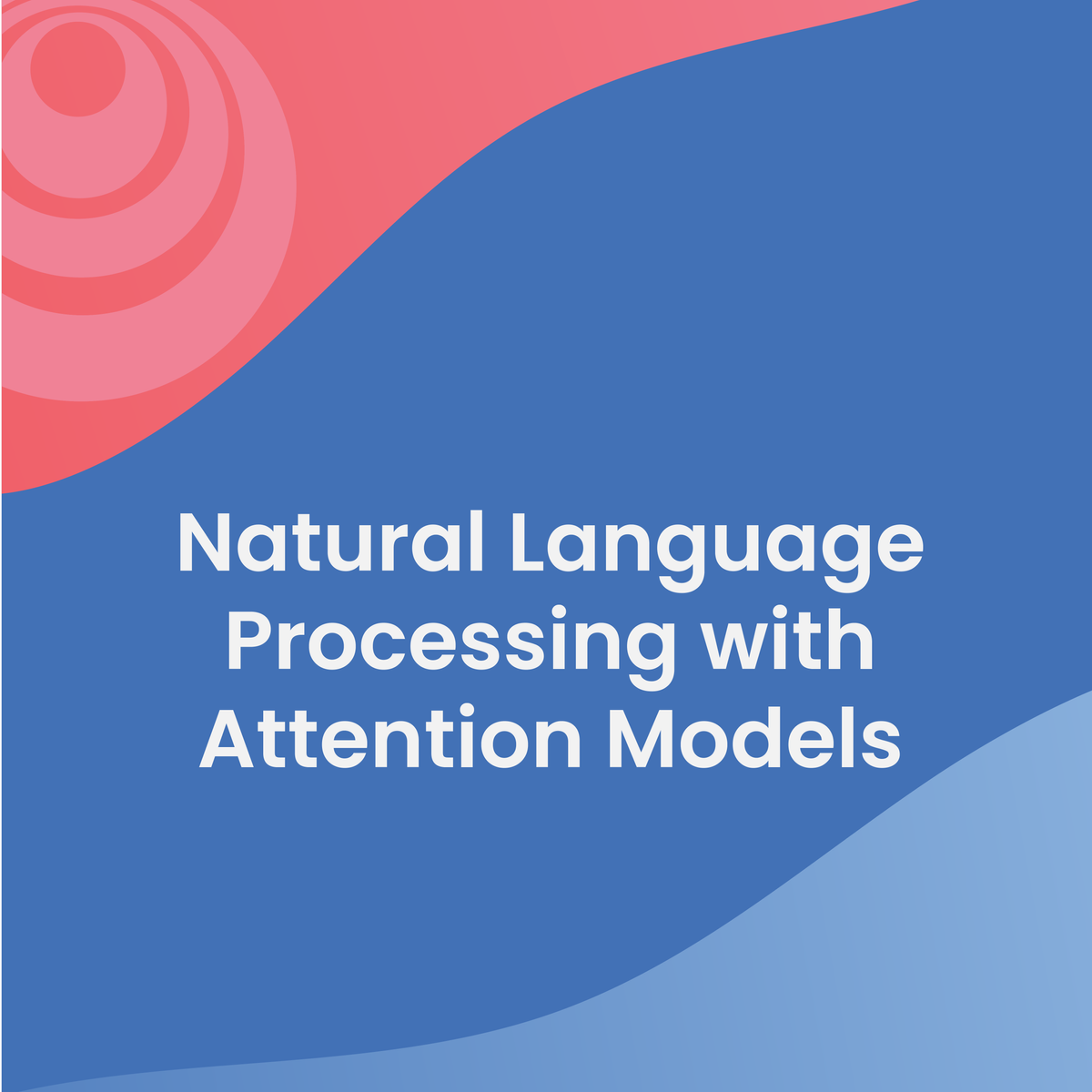
Algorithms Courses - Page 6
Showing results 51-60 of 326
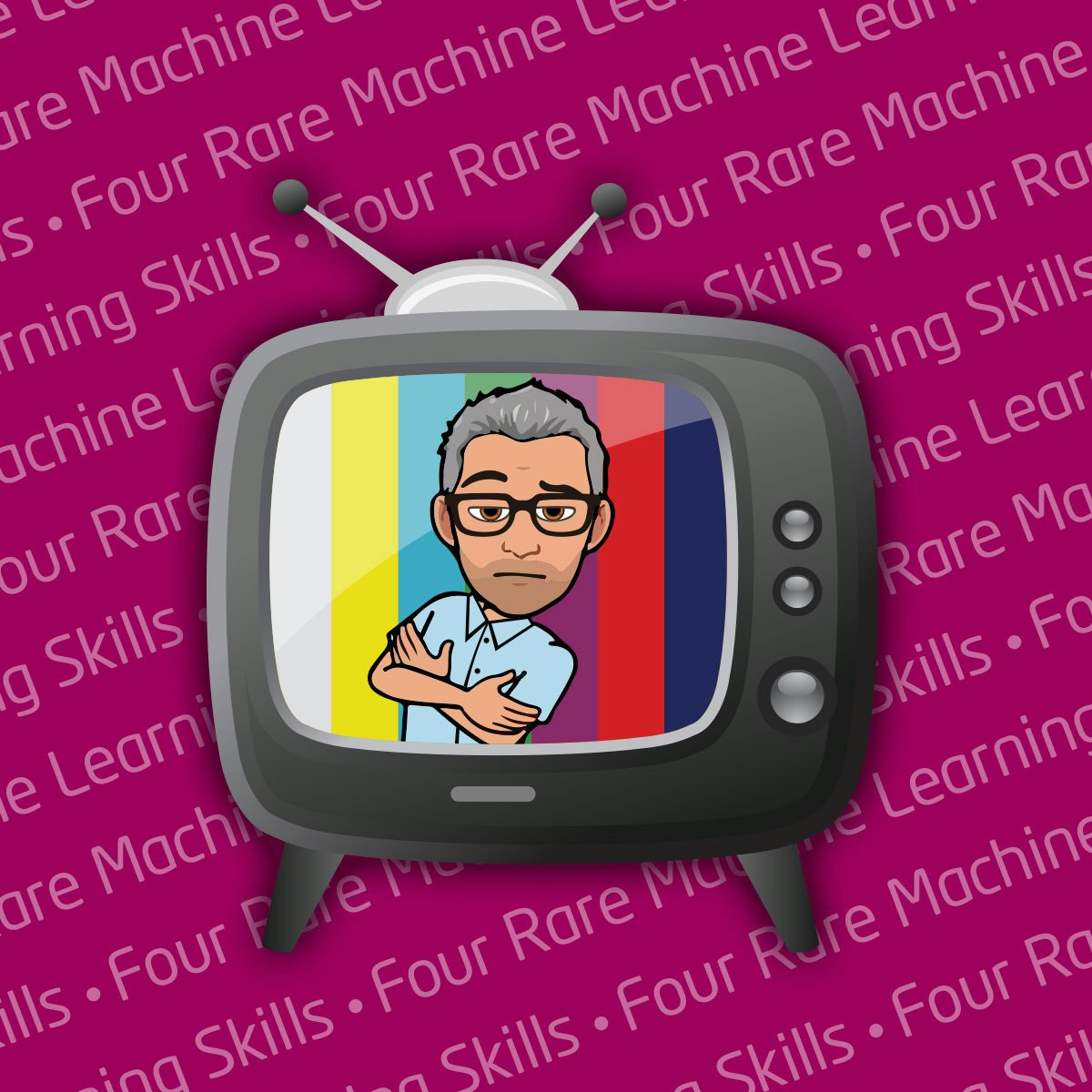
Four Rare Machine Learning Skills All Data Scientists Need
This course covers the most neglected yet critical skills in machine learning, four vital techniques that are very rarely covered – most courses and books omit them entirely.
1) UPLIFT MODELING (AKA PERSUASION MODELING): When you're modeling, are you even predicting the right thing?
2) THE ACCURACY FALLACY: When evaluating how well a model works, are you even reporting on the right thing?
3) P-HACKING: Are your simplest discoveries from data even real?
4) THE PARADOX OF ENSEMBLE MODELS: Do you understand how they work, even though they seem to defy Occam's Razor?
>> WHY THESE ADVANCED METHODS ARE ESSENTIAL: Each one addresses a question that is fundamental to machine learning (above). For many projects, success hinges on these particular skills.
>> NO HANDS-ON – BUT FOR TECHNICAL LEARNERS: This course has no coding and no use of machine learning software. Instead, it lays the conceptual groundwork before you take on the hands-on practice. When it comes to these state-of-the-art techniques and prevalent pitfalls, there's a foundation of conceptual knowledge to build before going hands-on – and you'll be glad you did.
>> VENDOR-NEUTRAL: This course includes illuminating software demos of machine learning in action using SAS products. However, the curriculum is vendor-neutral and universally-applicable. The contents and learning objectives apply, regardless of which machine learning software tools you end up choosing to work with.
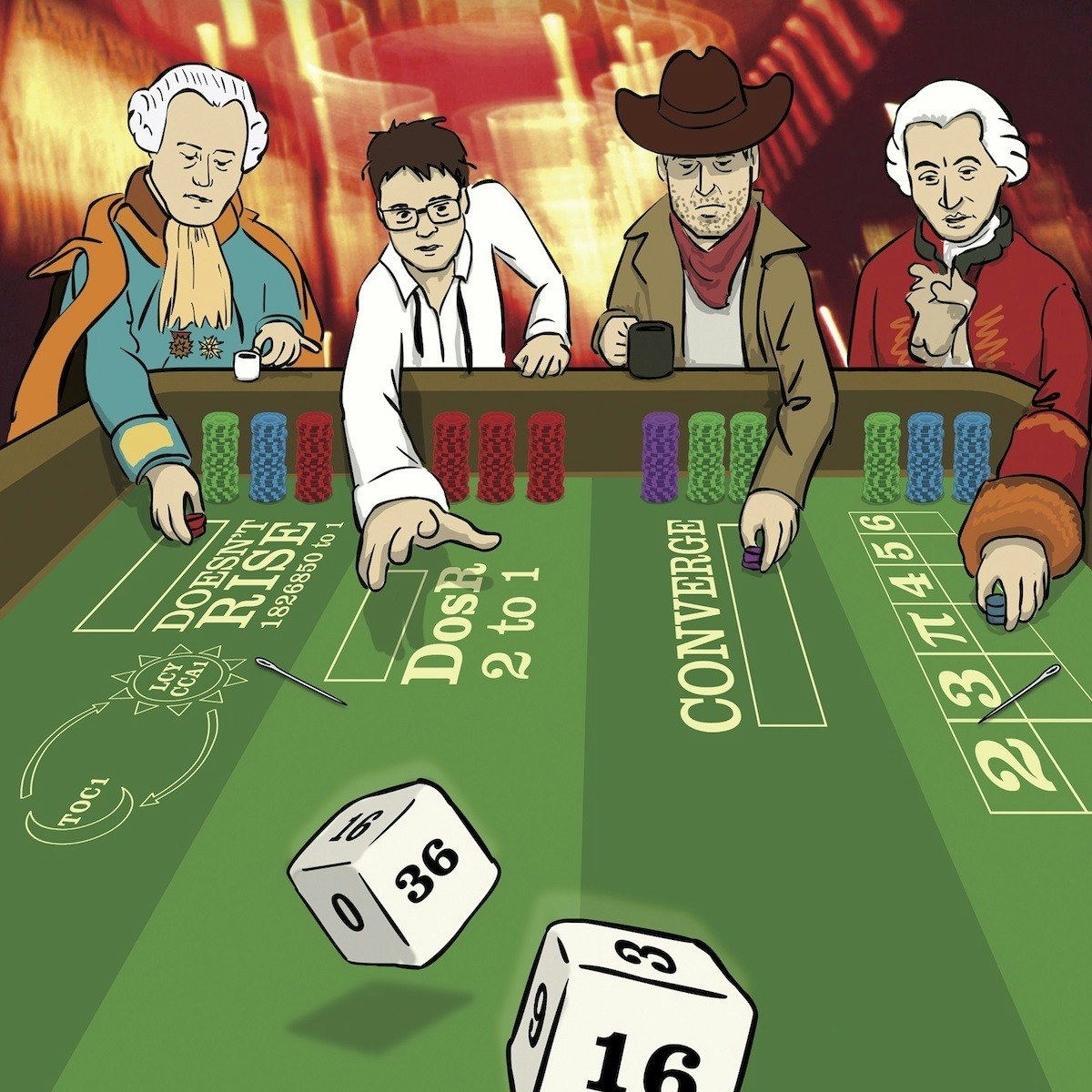
Finding Hidden Messages in DNA (Bioinformatics I)
Named a top 50 MOOC of all time by Class Central!
This course begins a series of classes illustrating the power of computing in modern biology. Please join us on the frontier of bioinformatics to look for hidden messages in DNA without ever needing to put on a lab coat.
In the first half of the course, we investigate DNA replication, and ask the question, where in the genome does DNA replication begin? We will see that we can answer this question for many bacteria using only some straightforward algorithms to look for hidden messages in the genome.
In the second half of the course, we examine a different biological question, when we ask which DNA patterns play the role of molecular clocks. The cells in your body manage to maintain a circadian rhythm, but how is this achieved on the level of DNA? Once again, we will see that by knowing which hidden messages to look for, we can start to understand the amazingly complex language of DNA. Perhaps surprisingly, we will apply randomized algorithms, which roll dice and flip coins in order to solve problems.
Finally, you will get your hands dirty and apply existing software tools to find recurring biological motifs within genes that are responsible for helping Mycobacterium tuberculosis go "dormant" within a host for many years before causing an active infection.
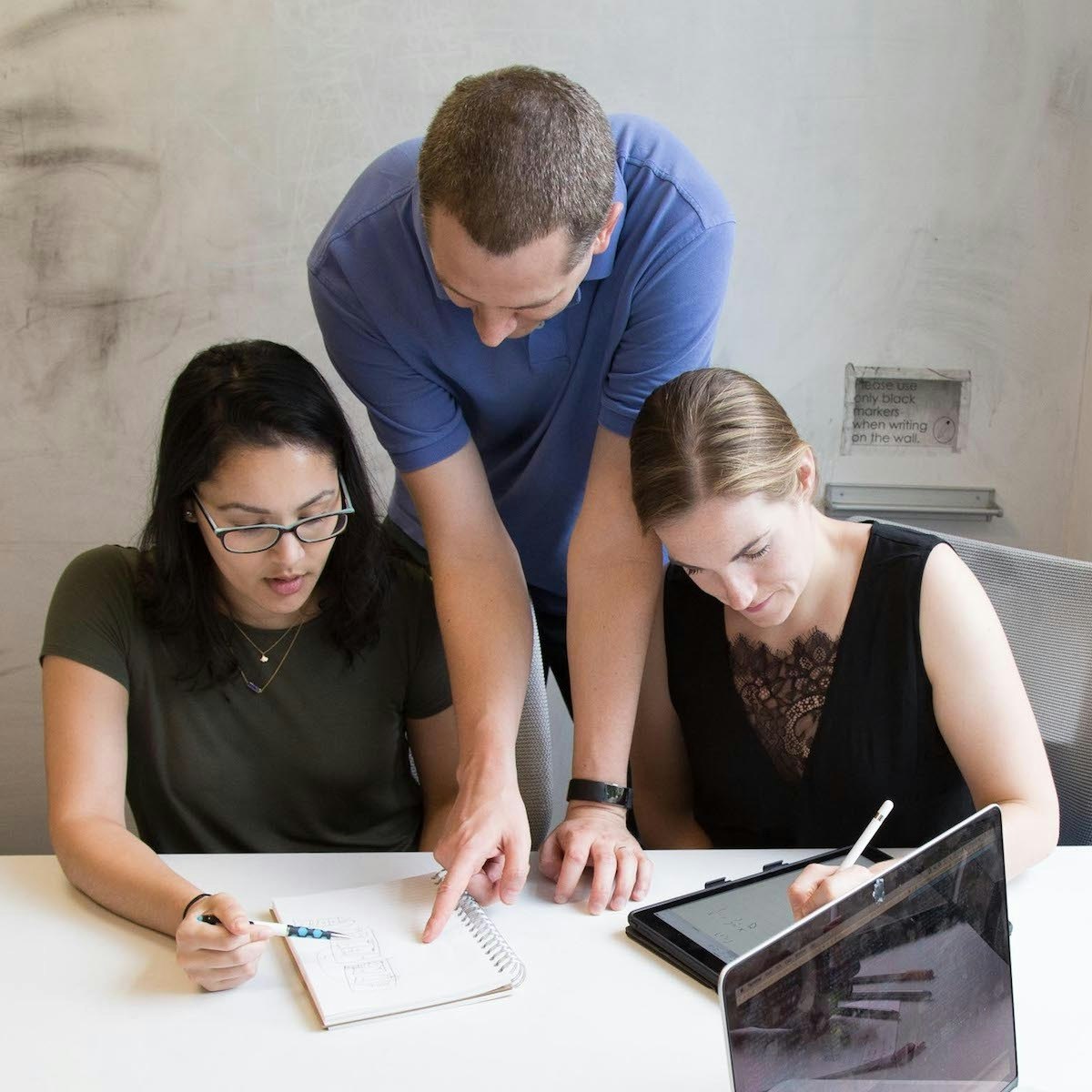
Pointers, Arrays, and Recursion
The third course in the specialization Introduction to Programming in C introduces the programming constructs pointers, arrays, and recursion. Pointers provide control and flexibility when programming in C by giving you a way to refer to the location of other data. Arrays provide a way to bundle data by guaranteeing sequences of data are grouped together. Finally, recursive functions—functions that call themselves—provide an alternative to iteration that are very useful for implementing certain algorithms.
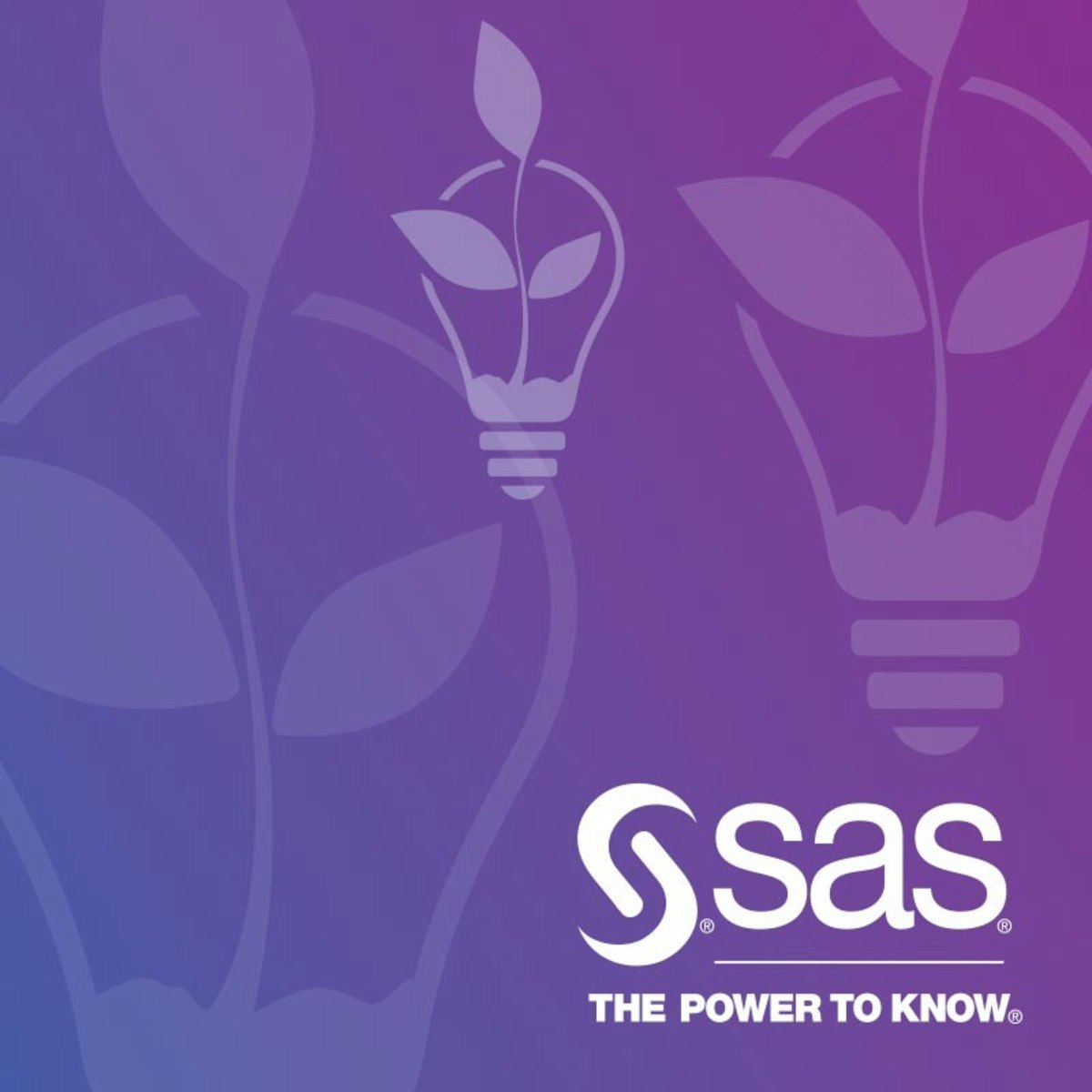
Practical SAS Programming and Certification Review
In this course you have the opportunity to use the skills you acquired in the two SAS programming courses to solve realistic problems. This course is also designed to give you a thorough review of SAS programming concepts so you are prepared to take the SAS Certified Specialist: Base Programming Using SAS 9.4 Exam.
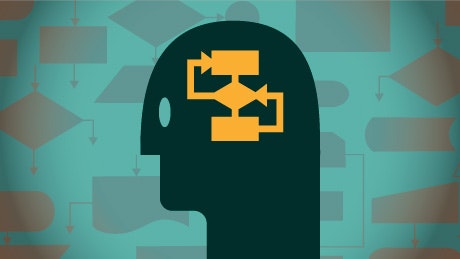
Algorithmic Thinking (Part 1)
Experienced Computer Scientists analyze and solve computational problems at a level of abstraction that is beyond that of any particular programming language. This two-part course builds on the principles that you learned in our Principles of Computing course and is designed to train students in the mathematical concepts and process of "Algorithmic Thinking", allowing them to build simpler, more efficient solutions to real-world computational problems.
In part 1 of this course, we will study the notion of algorithmic efficiency and consider its application to several problems from graph theory. As the central part of the course, students will implement several important graph algorithms in Python and then use these algorithms to analyze two large real-world data sets. The main focus of these tasks is to understand interaction between the algorithms and the structure of the data sets being analyzed by these algorithms.
Recommended Background - Students should be comfortable writing intermediate size (300+ line) programs in Python and have a basic understanding of searching, sorting, and recursion. Students should also have a solid math background that includes algebra, pre-calculus and a familiarity with the math concepts covered in "Principles of Computing".
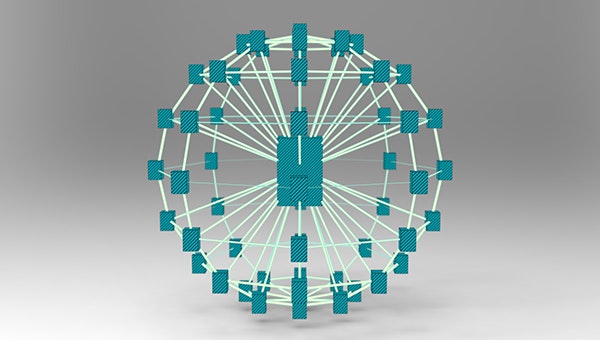
Cloud Computing Concepts, Part 1
Cloud computing systems today, whether open-source or used inside companies, are built using a common set of core techniques, algorithms, and design philosophies – all centered around distributed systems. Learn about such fundamental distributed computing "concepts" for cloud computing.
Some of these concepts include: clouds, MapReduce, key-value/NoSQL stores, classical distributed algorithms, widely-used distributed algorithms, scalability, trending areas, and much, much more!
Know how these systems work from the inside out. Get your hands dirty using these concepts with provided homework exercises. In the programming assignments, implement some of these concepts in template code (programs) provided in the C++ programming language. Prior experience with C++ is required.
The course also features interviews with leading researchers and managers, from both industry and academia.
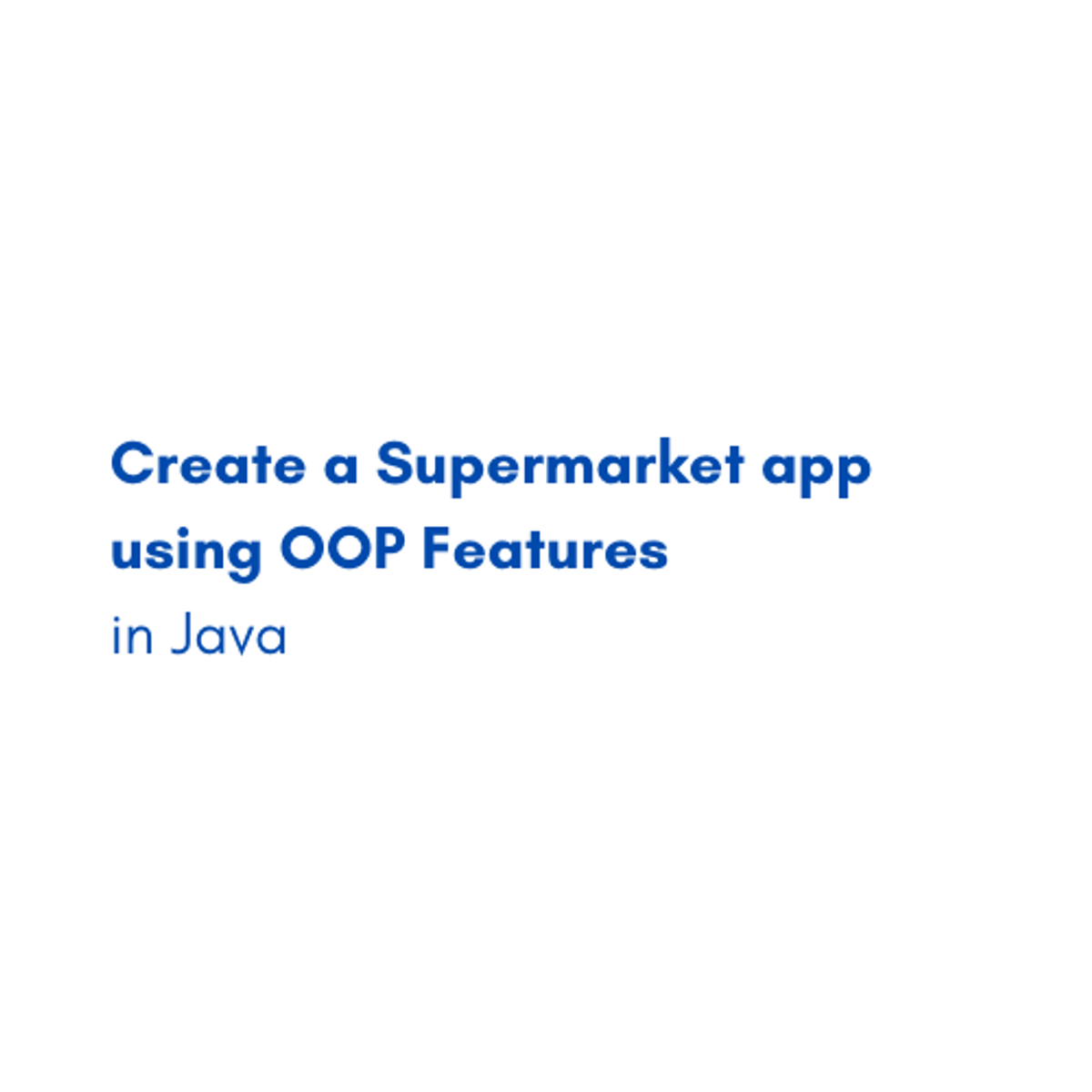
Create a Supermarket app using OOP Features in Java
In this 1-hour long project-based course, you will create a supermarket app using OOP Features in Java. You will learn how to code using the main Java OOP Features including Encapsulation, Polymorphism, Inheritance and Abstraction. You will learn how to create a main method to test your code and finalize your application.
Note: This course works best for learners who are based in the North America region. We’re currently working on providing the same experience in other regions.
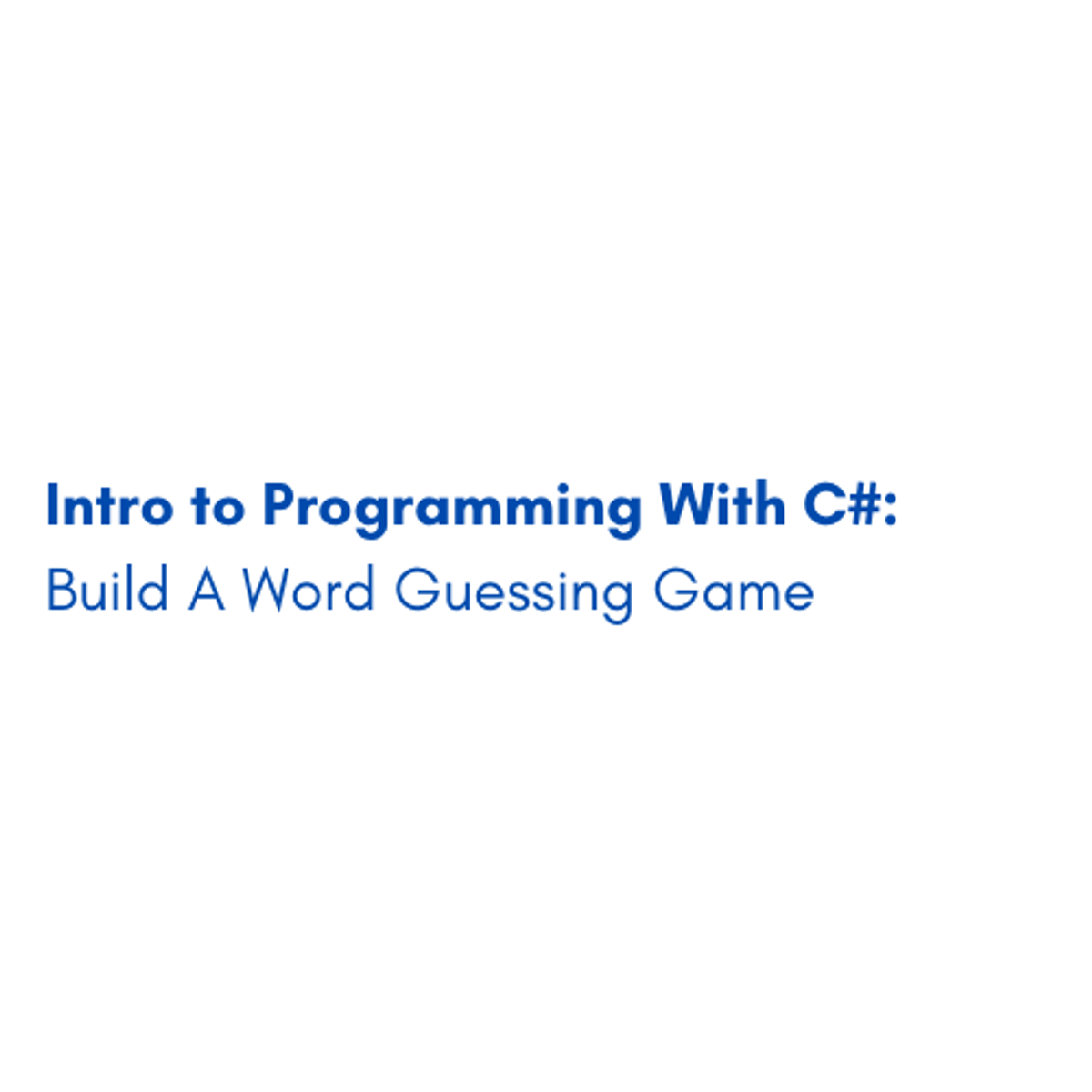
Intro to Programming With C#: Build A Word Guessing Game
By the end of this project, you will create a fully functioning Word Guessing game using C#. In this project, you will be able to identify and apply some important coding concepts, such as “variables,” “if-conditions,” “for-loops,” as well as learning how to display output and take inputs from the user.
In each task, you’ll be able to apply one of these concepts and at the end, tie them all together into the Word Guessing game we will be building.
You’ll have the knowledge and skills needed to start writing your very own programs. You’ll also be able to apply what you learn here using other languages such as C++, Java, Python, etc.
Note: This course works best for learners who are based in the North America region. We’re currently working on providing the same experience in other regions.
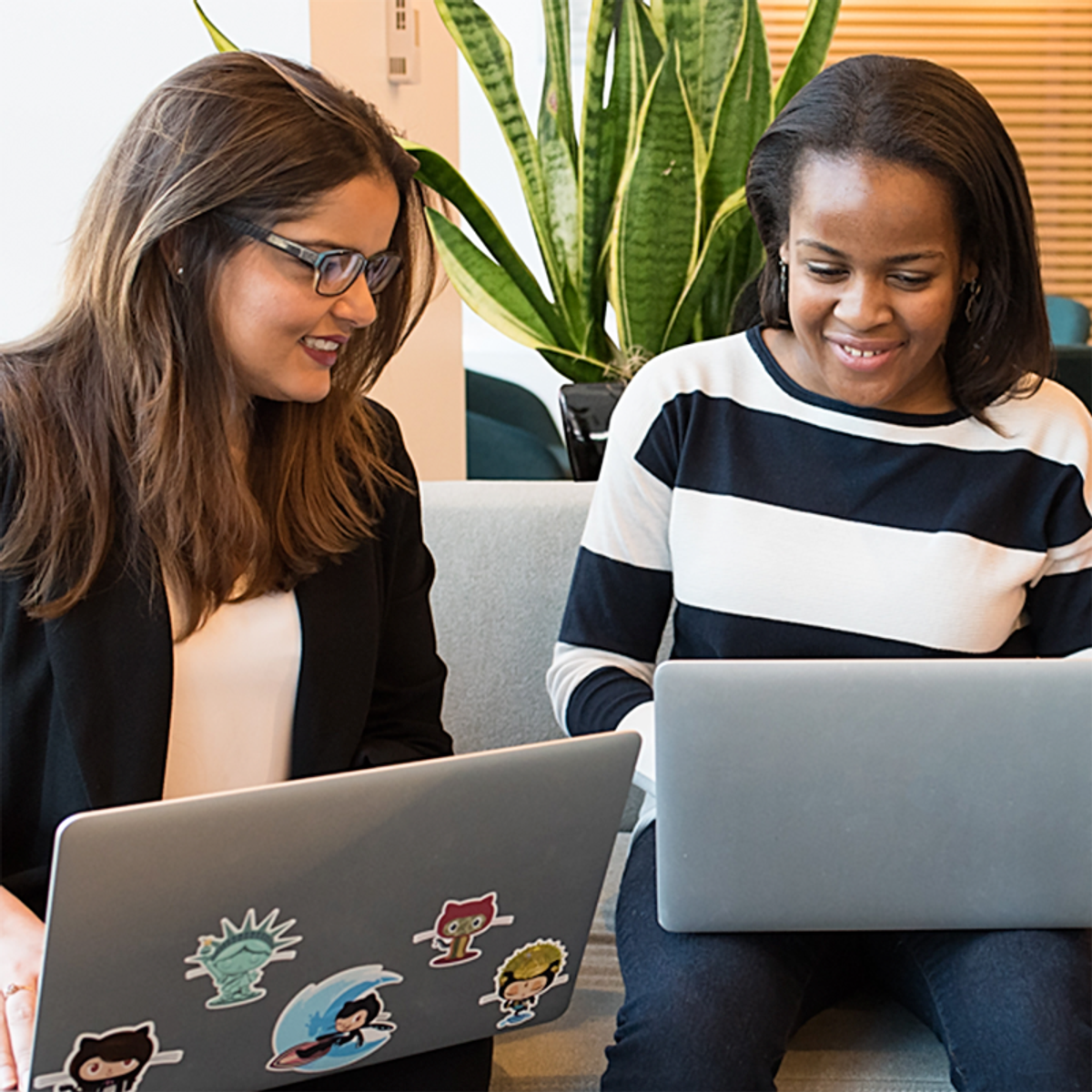
C Programming: Getting Started - 1
Start learning one of the most powerful and widely used programming languages: C. Within moments you will be coding hands-on in a browser tool that will provide instant feedback on your code.
The C programming language is one of the most stable and popular programming languages in the world. It helps to power your smartphone, your car's navigation system, robots, drones, trains, and almost all electronic devices. C is used in any circumstances where speed and flexibility are important, such as in embedded systems or high-performance computing.
In this course, you will get started with C and learn how to write your first programs, how to make simple computations and print the results to the screen, how to store values in variables and how to repeat instructions using loops.
Beginners, even those without any programming experience, will be able to immediately start coding in C with the help of powerful yet simple coding tools right within the web browser. No need to install anything!
Why learn C and not another programming language? Did you know that smartphones, your car’s navigation system, robots, drones, trains, and almost all electronic devices have some C-code running under the hood? C is used in any circumstance where speed and flexibility are important, such as in embedded systems or high-performance computing.
C is a foundational programming language taught at engineering schools around the world, and represents one of the building blocks of modern computer information technology. Invented in the 1970’s. It is still one of the most stable and popular programming languages in the world.
We are excited to introduce you to the world of coding and launch you along your path to becoming a skilled C programmer!
This is the first course in the C Programming with Linux Specialization. This series of seven courses will establish your programming skills and unlock doors to careers in computer engineering.
This course has received financial support from the Patrick & Lina Drahi Foundation.
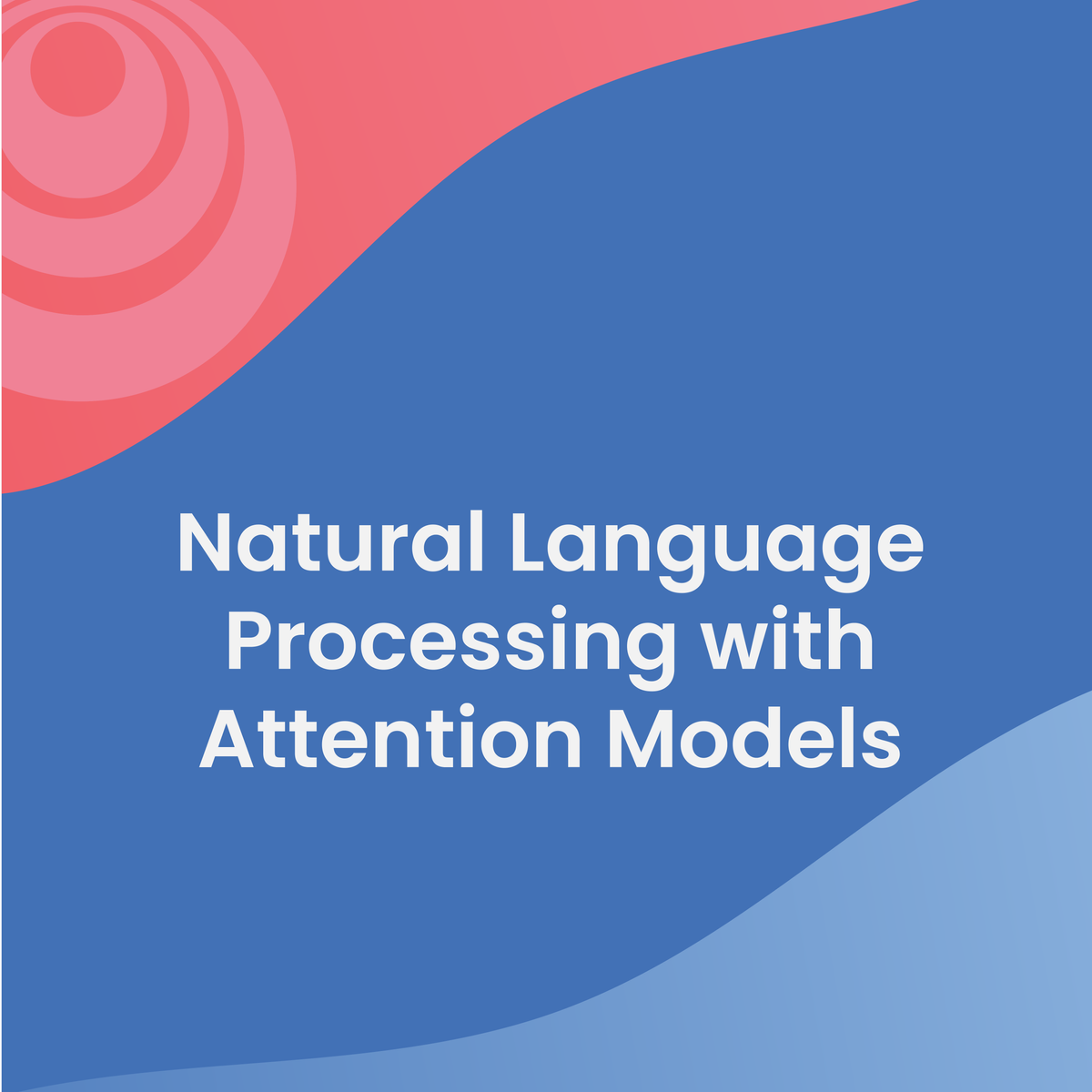
Natural Language Processing with Attention Models
In Course 4 of the Natural Language Processing Specialization, you will:
a) Translate complete English sentences into German using an encoder-decoder attention model,
b) Build a Transformer model to summarize text,
c) Use T5 and BERT models to perform question-answering, and
d) Build a chatbot using a Reformer model.
By the end of this Specialization, you will have designed NLP applications that perform question-answering and sentiment analysis, created tools to translate languages and summarize text, and even built a chatbot!
Learners should have a working knowledge of machine learning, intermediate Python including experience with a deep learning framework (e.g., TensorFlow, Keras), as well as proficiency in calculus, linear algebra, and statistics. Please make sure that you’ve completed course 3 - Natural Language Processing with Sequence Models - before starting this course.
This Specialization is designed and taught by two experts in NLP, machine learning, and deep learning. Younes Bensouda Mourri is an Instructor of AI at Stanford University who also helped build the Deep Learning Specialization. Łukasz Kaiser is a Staff Research Scientist at Google Brain and the co-author of Tensorflow, the Tensor2Tensor and Trax libraries, and the Transformer paper.
