Back to Courses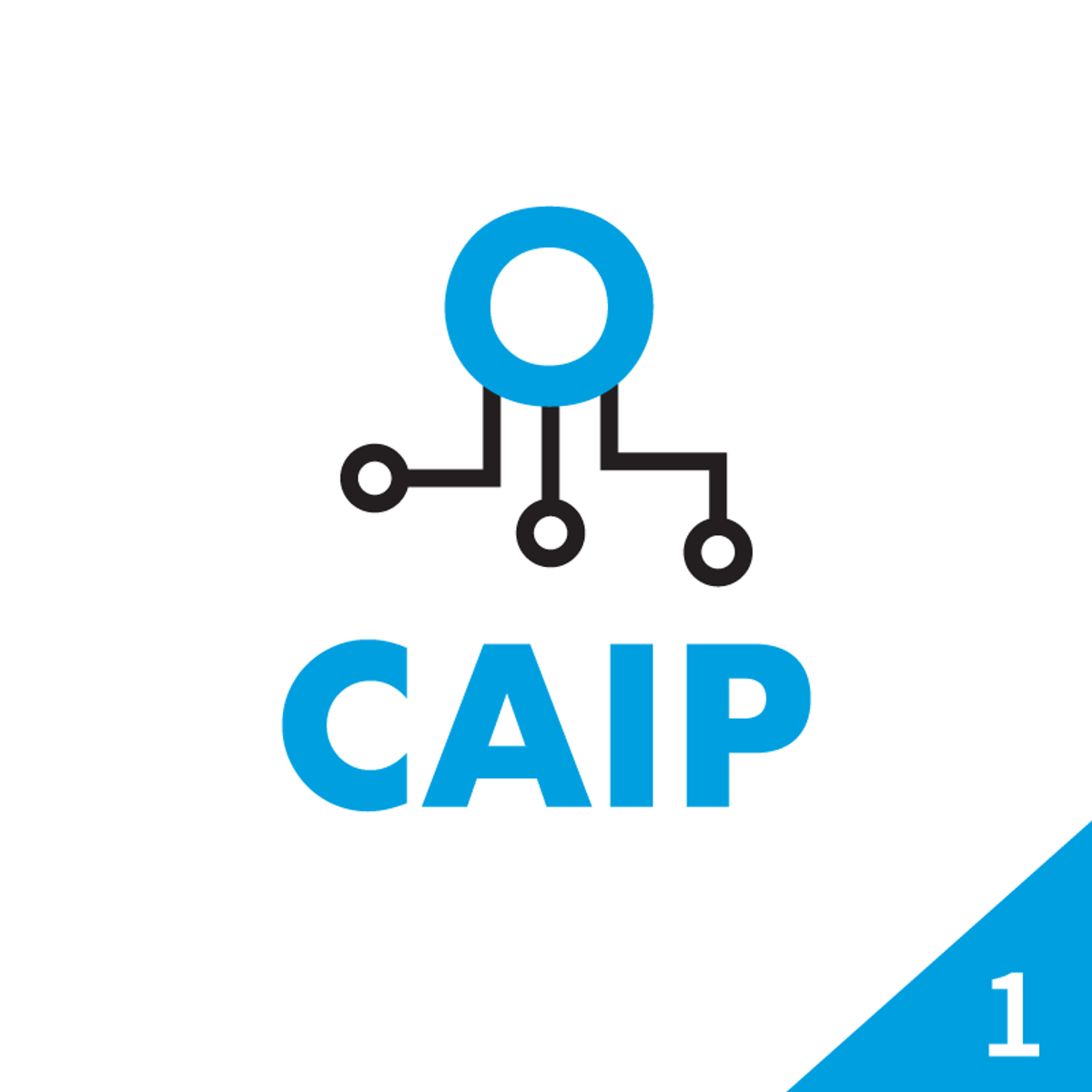
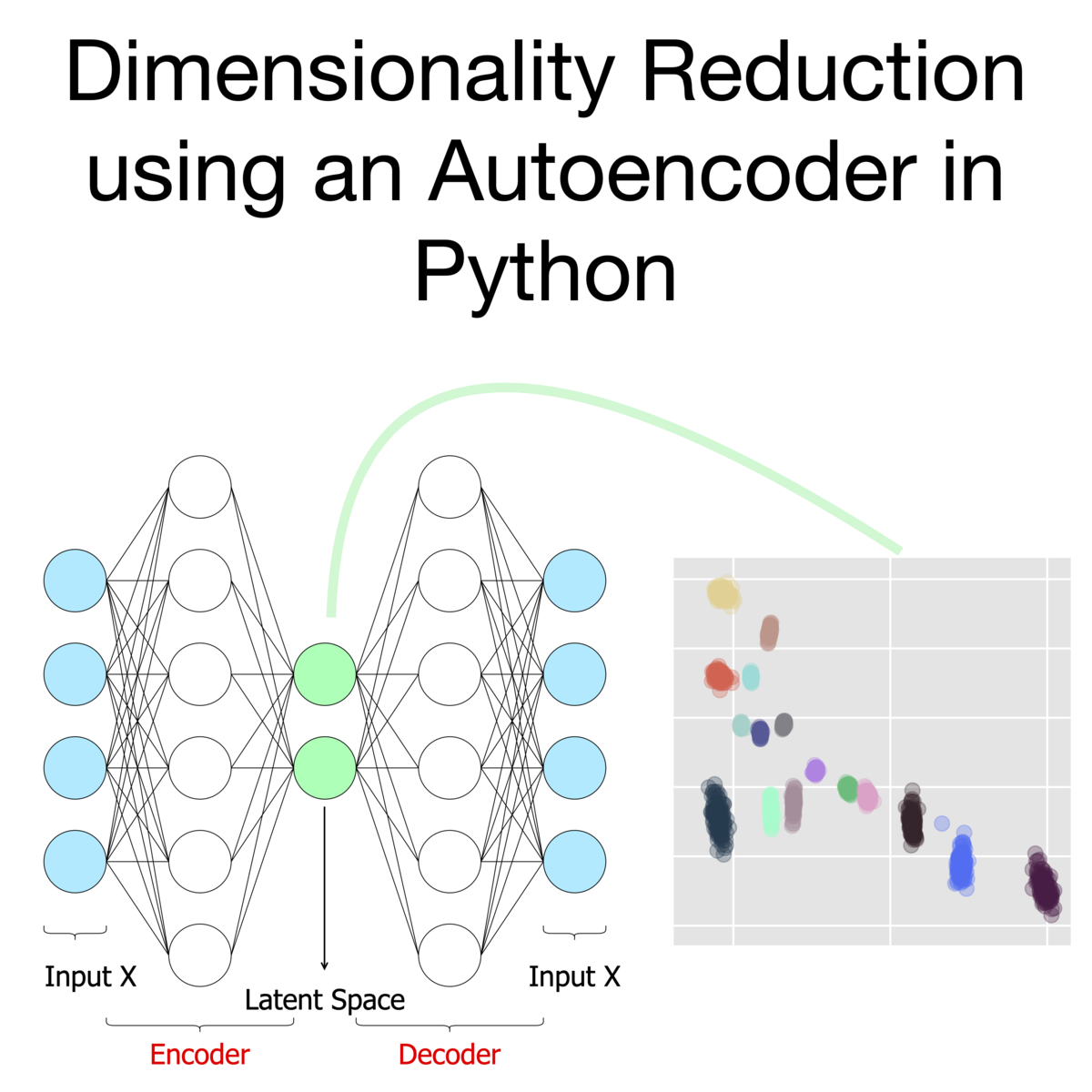
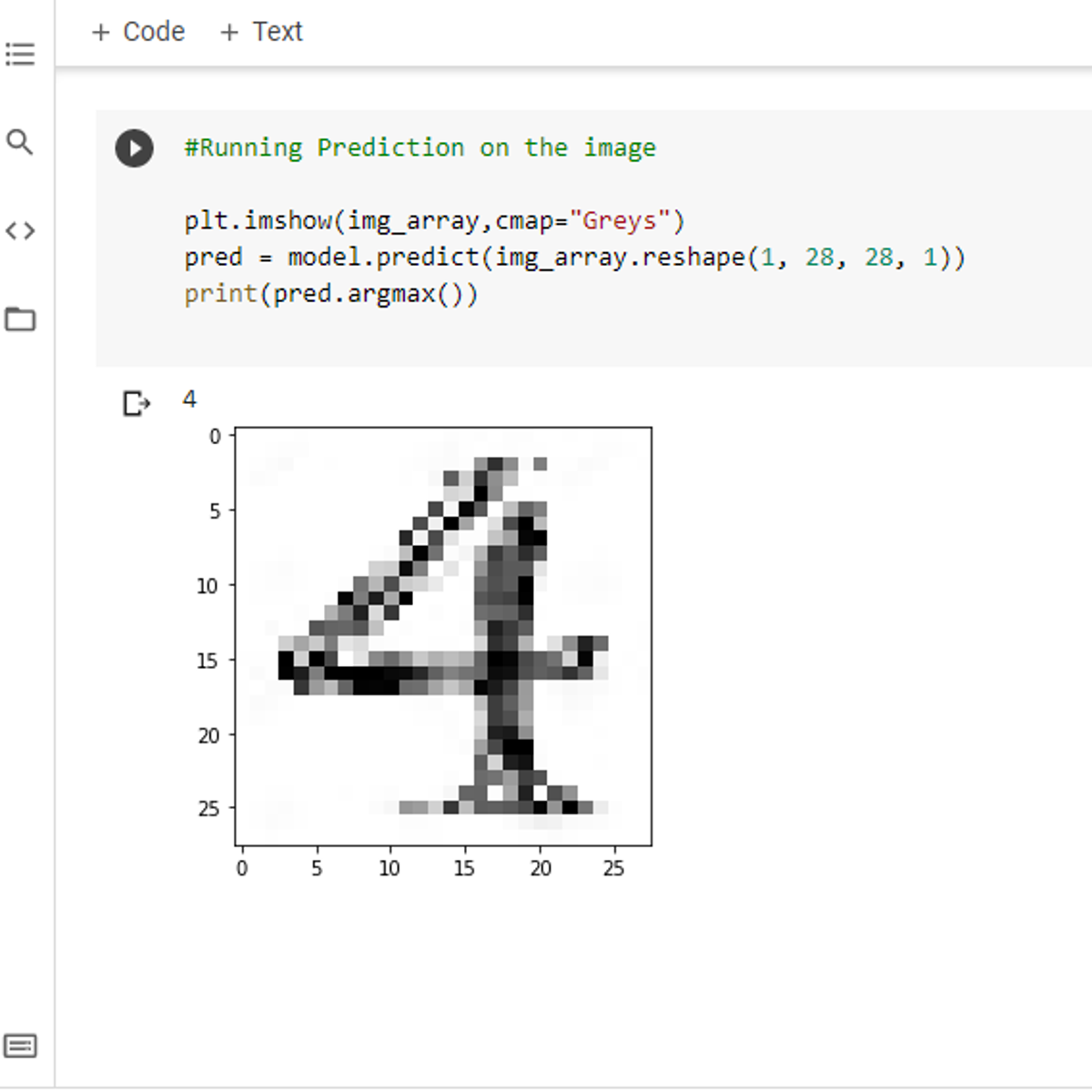
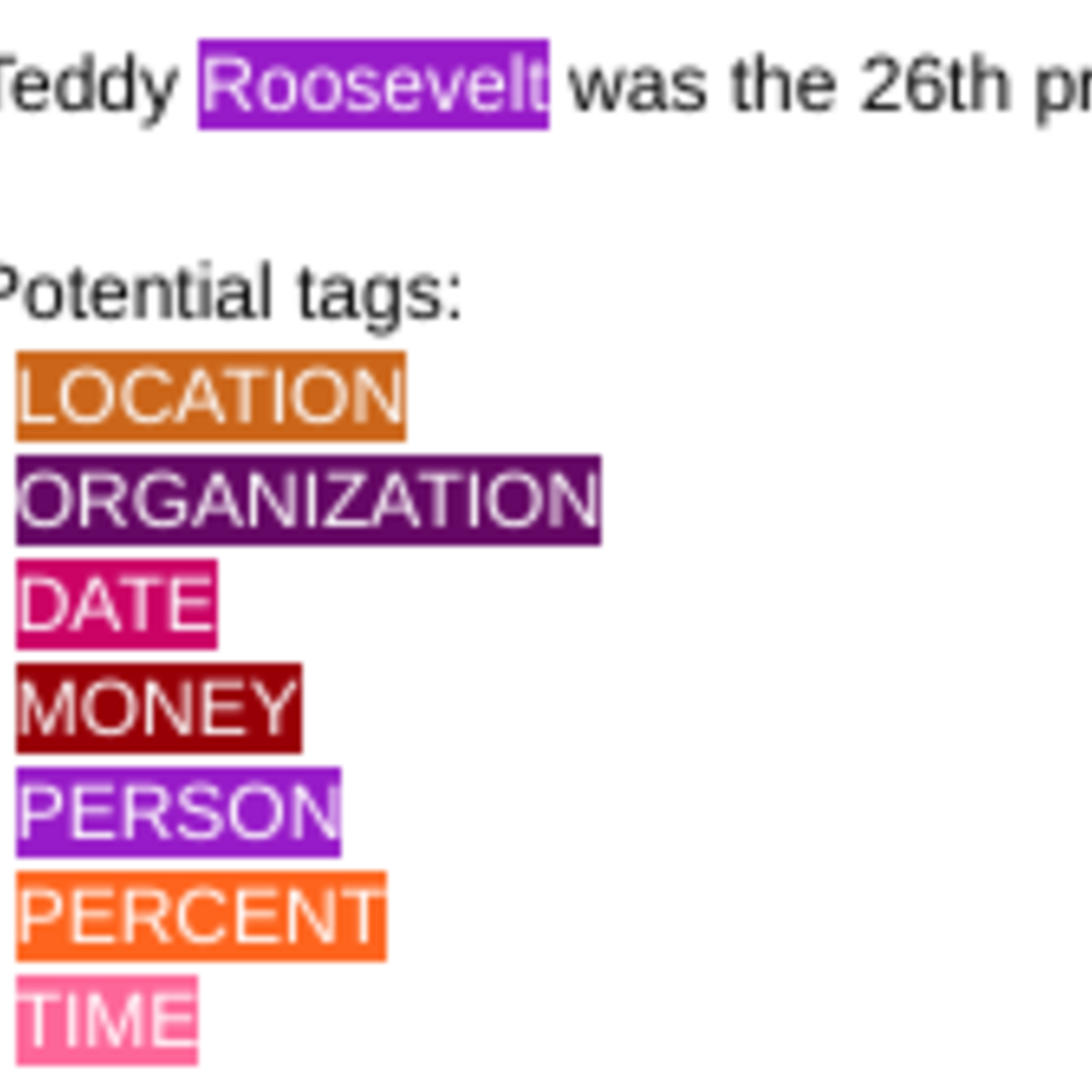
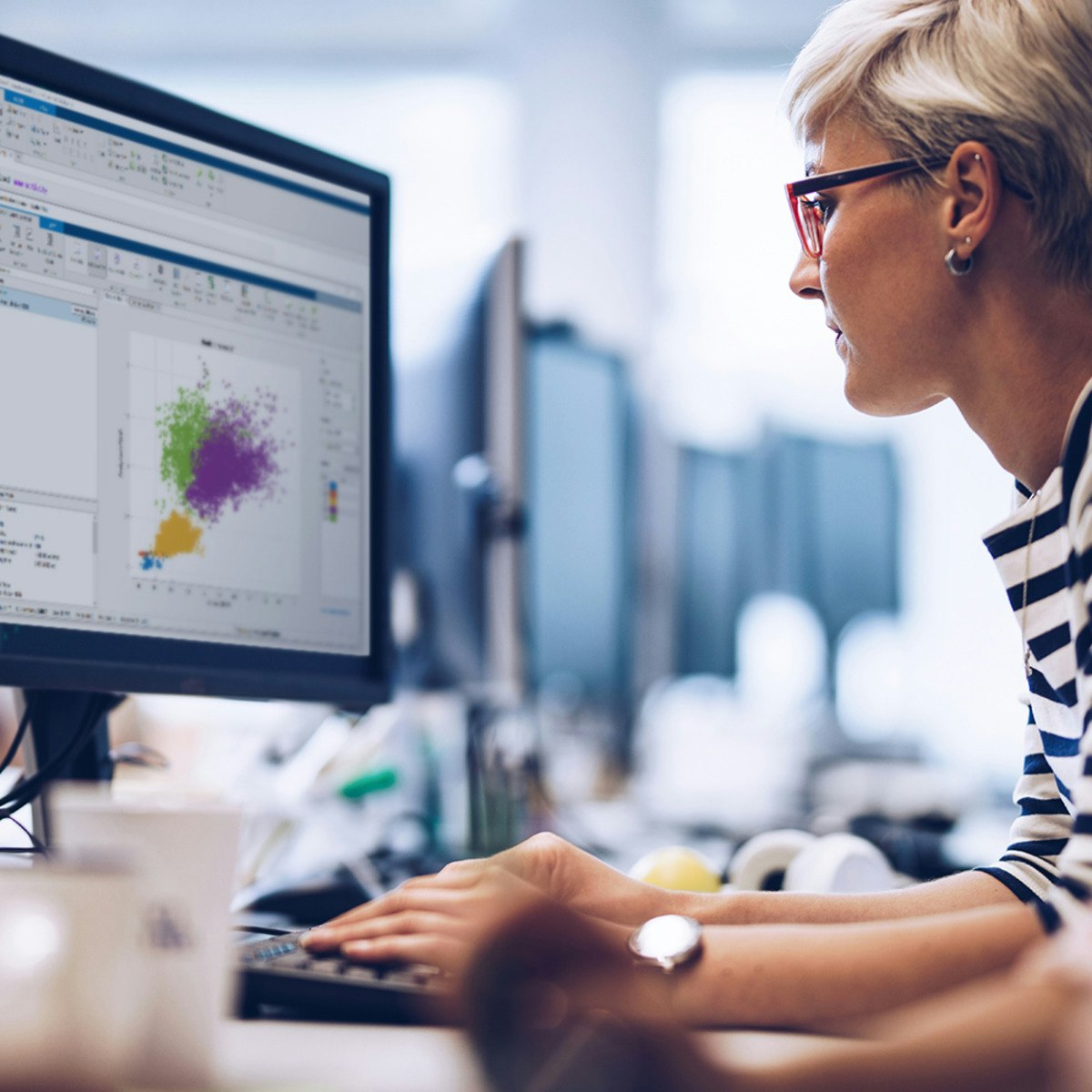
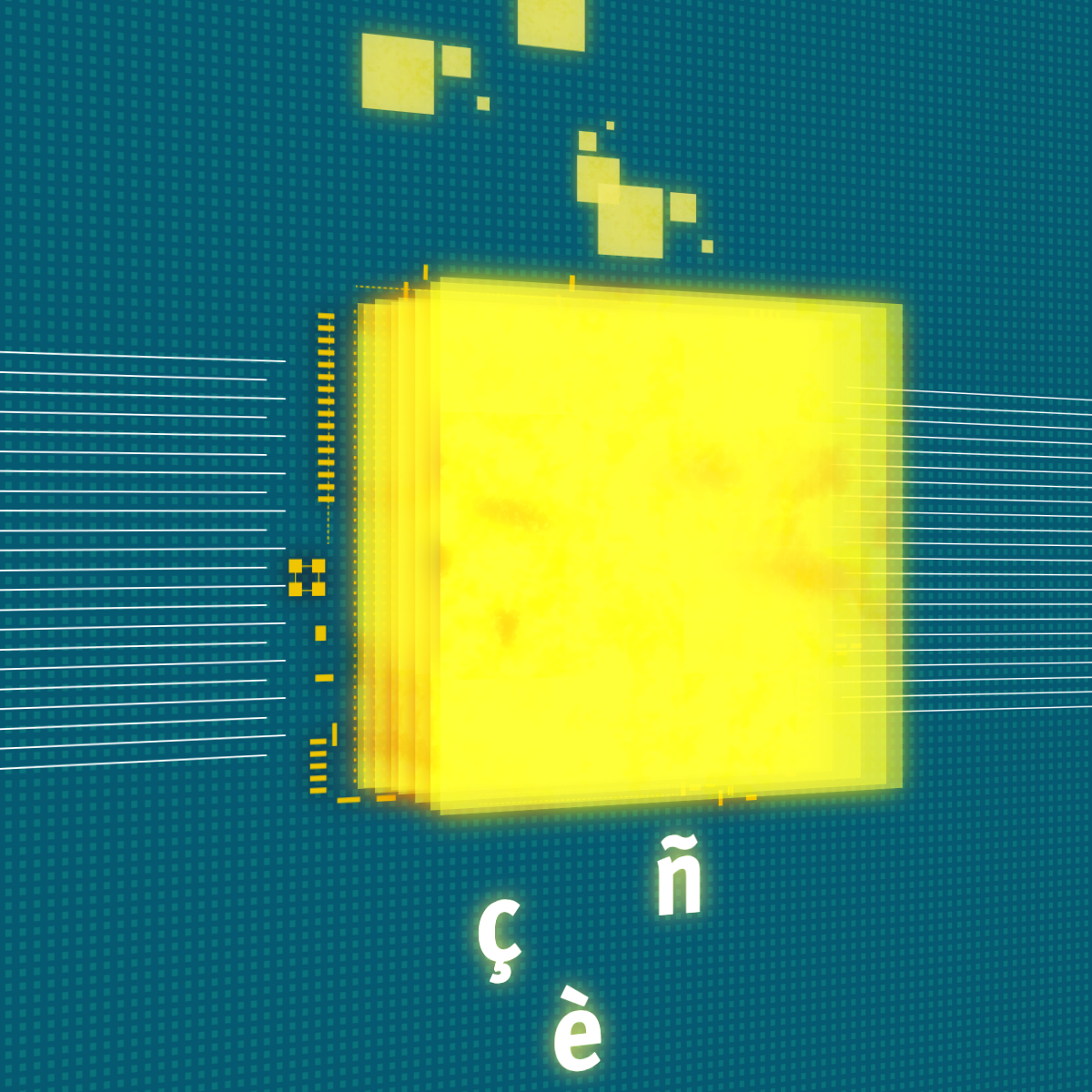
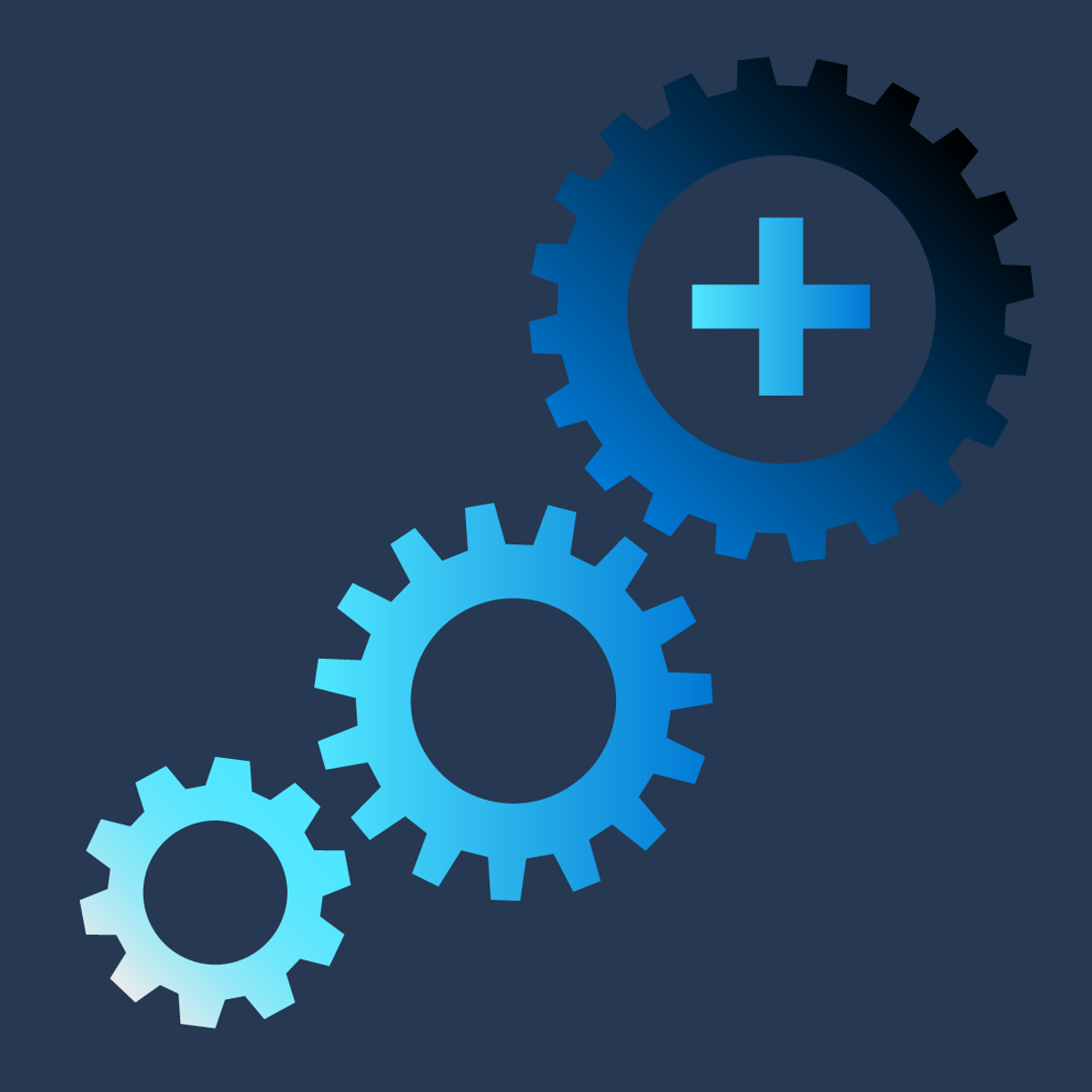
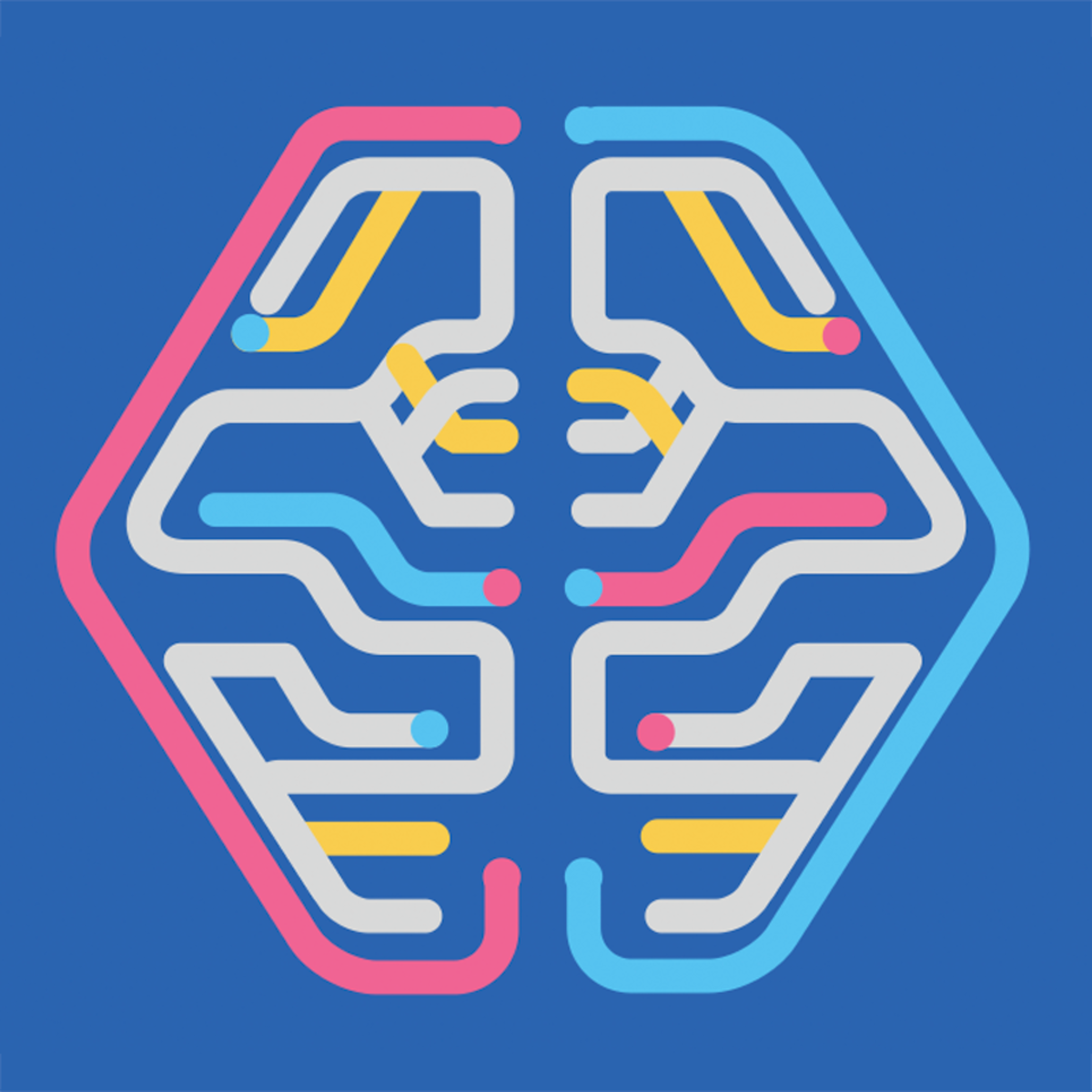
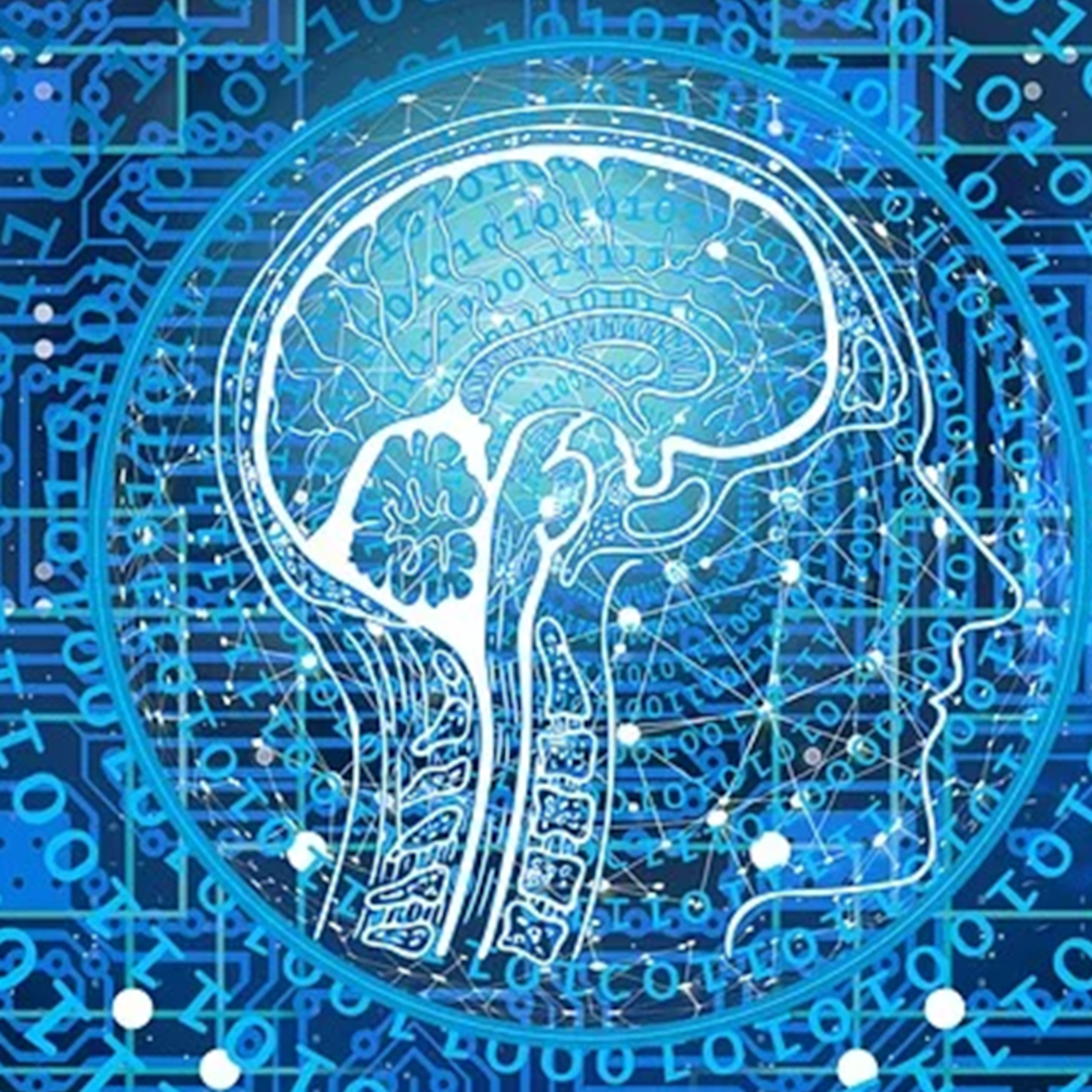
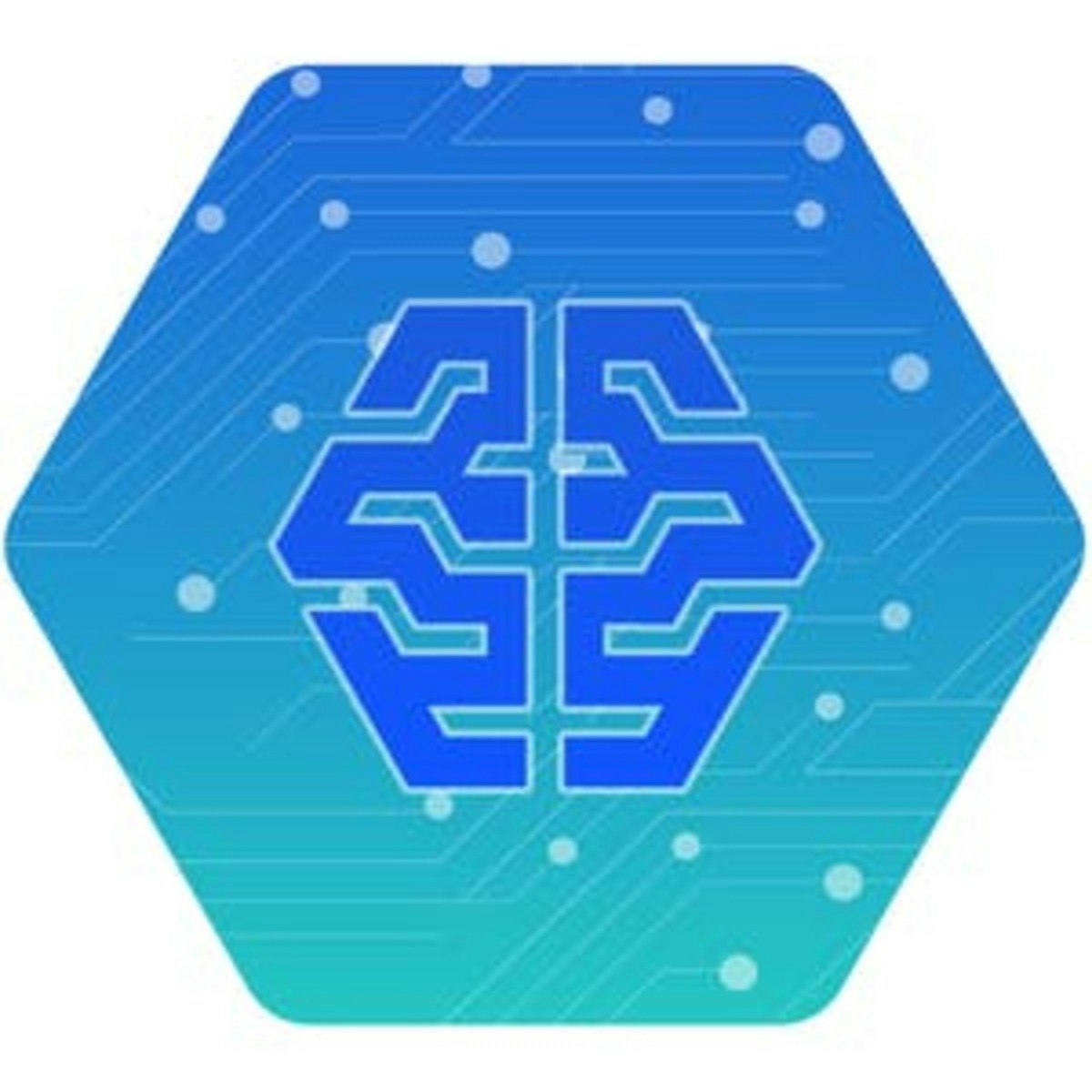
Machine Learning Courses - Page 6
Showing results 51-60 of 485
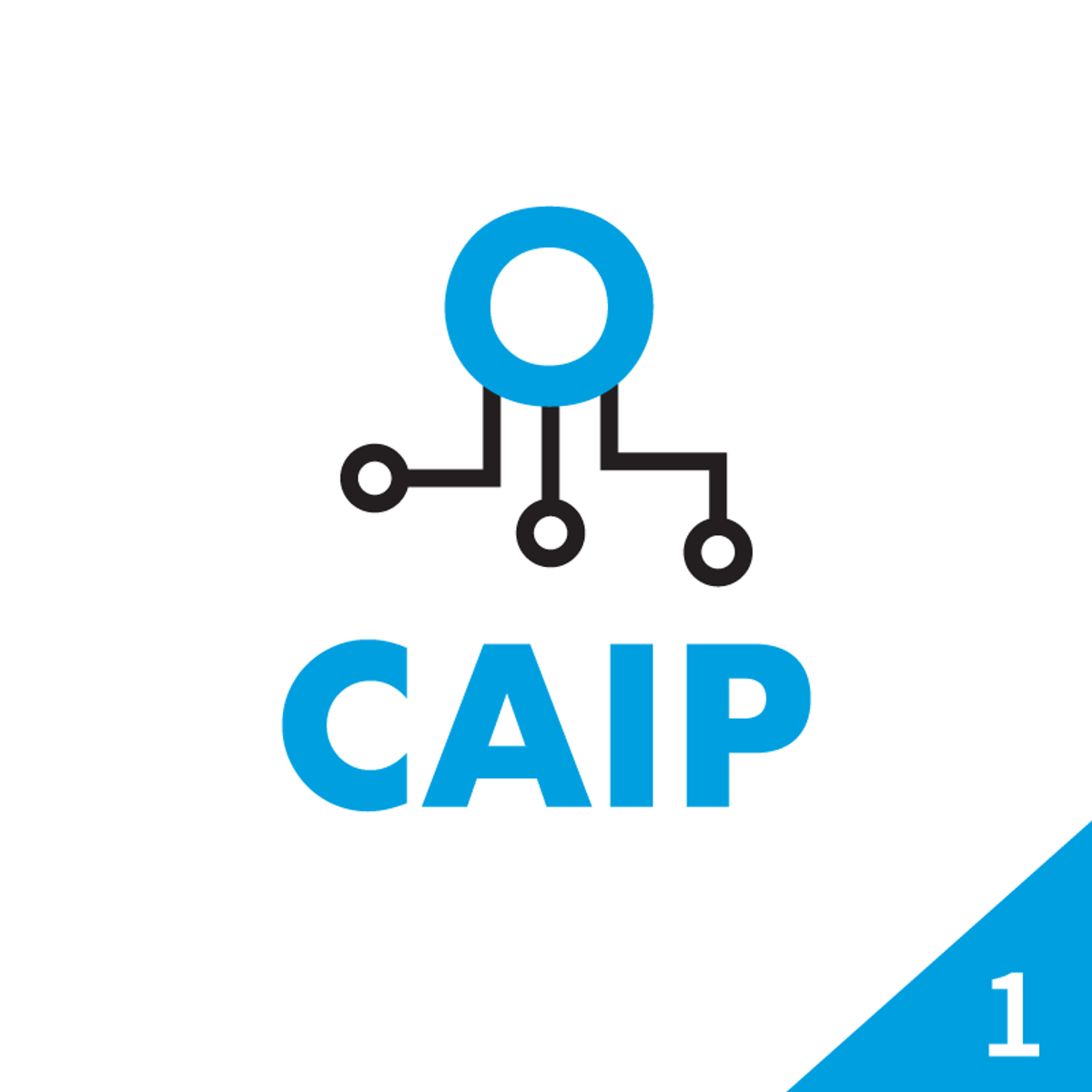
Solve Business Problems with AI and Machine Learning
Artificial intelligence (AI) and machine learning (ML) have become an essential part of the toolset for many organizations. When used effectively, these tools provide actionable insights that drive critical decisions and enable organizations to create exciting, new, and innovative products and services.
This is the first of four courses in the Certified Artificial Intelligence Practitioner (CAIP) professional certification. This course is meant as an entry point into the world of AI/ML. You'll learn about the business problems that AI/ML can solve, as well as the specific AI/ML technologies that can solve them. In addition, you'll get an overview of the general workflow involved in machine learning, as well as the tools and other resources that support it. This course also promotes the importance of ethics in AI/ML, and provides you with techniques for addressing ethical challenges.
Ultimately, this course will get you thinking about the "why?" of AI/ML, and it will ensure that your more technical work in later courses is done with clear business goals in mind.
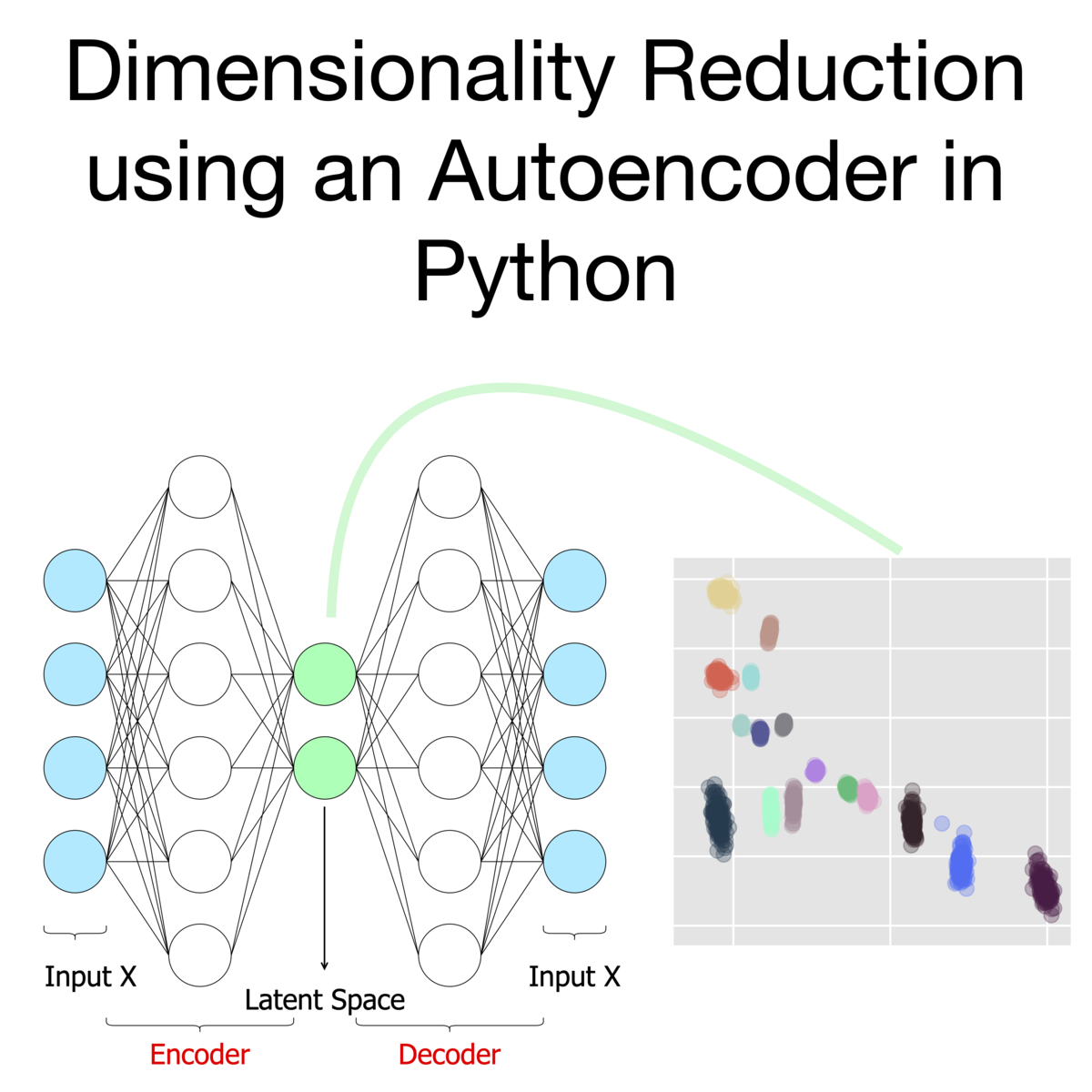
Dimensionality Reduction using an Autoencoder in Python
In this 1-hour long project, you will learn how to generate your own high-dimensional dummy dataset. You will then learn how to preprocess it effectively before training a baseline PCA model. You will learn the theory behind the autoencoder, and how to train one in scikit-learn. You will also learn how to extract the encoder portion of it to reduce dimensionality of your input data. In the course of this project, you will also be exposed to some basic clustering strength metrics.
Note: This course works best for learners who are based in the North America region. We’re currently working on providing the same experience in other regions.
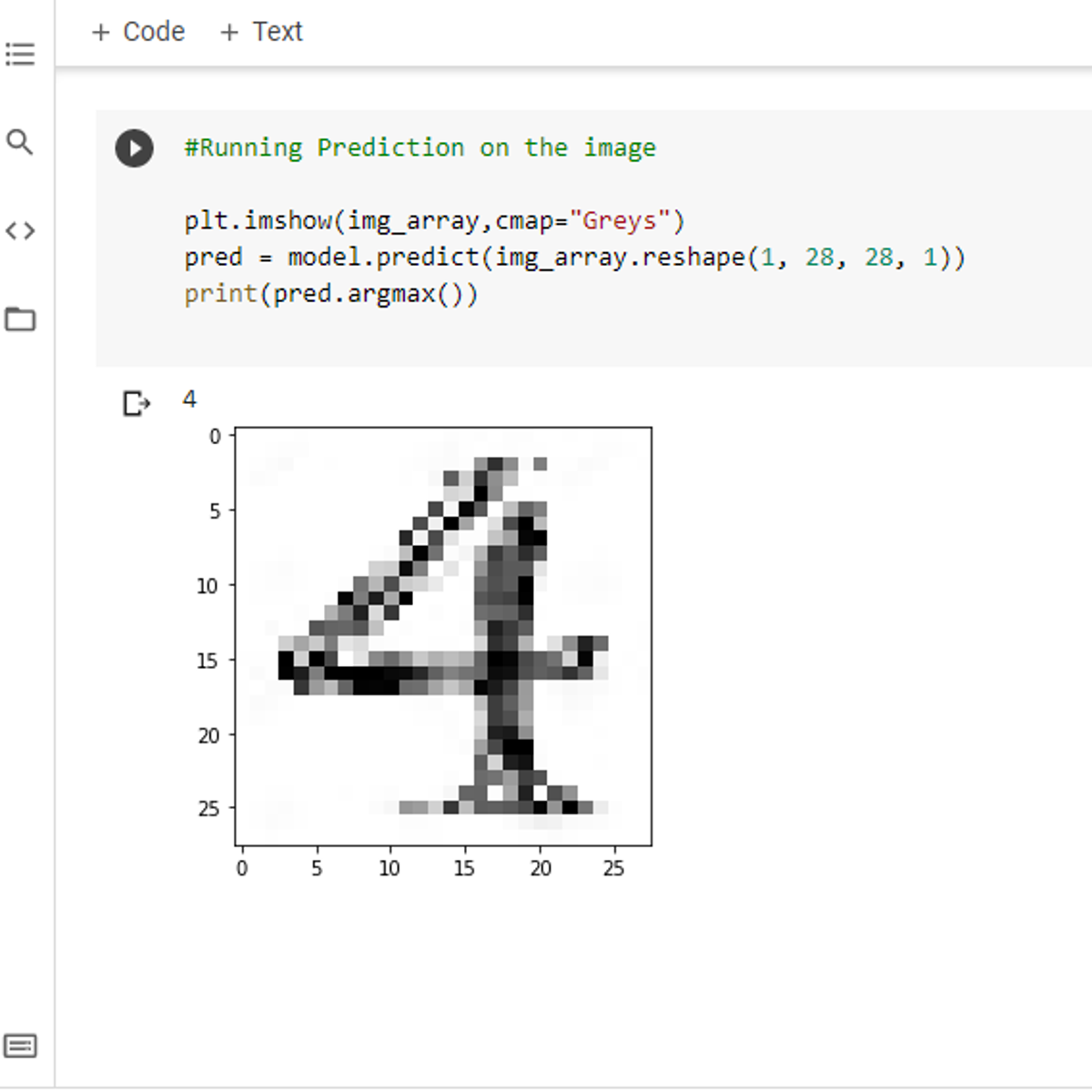
Build a Machine Learning Image Classifier with Python
In this 1-hour long project-based course, you will learn how to build your own Machine Learning Image Classifier using Python and Colab. You will be able to easily load the data, preview it, process and normalize it, then train and test your model! I hope you enjoy the experience!
Note: This course works best for learners who are based in the North America region. We’re currently working on providing the same experience in other regions.
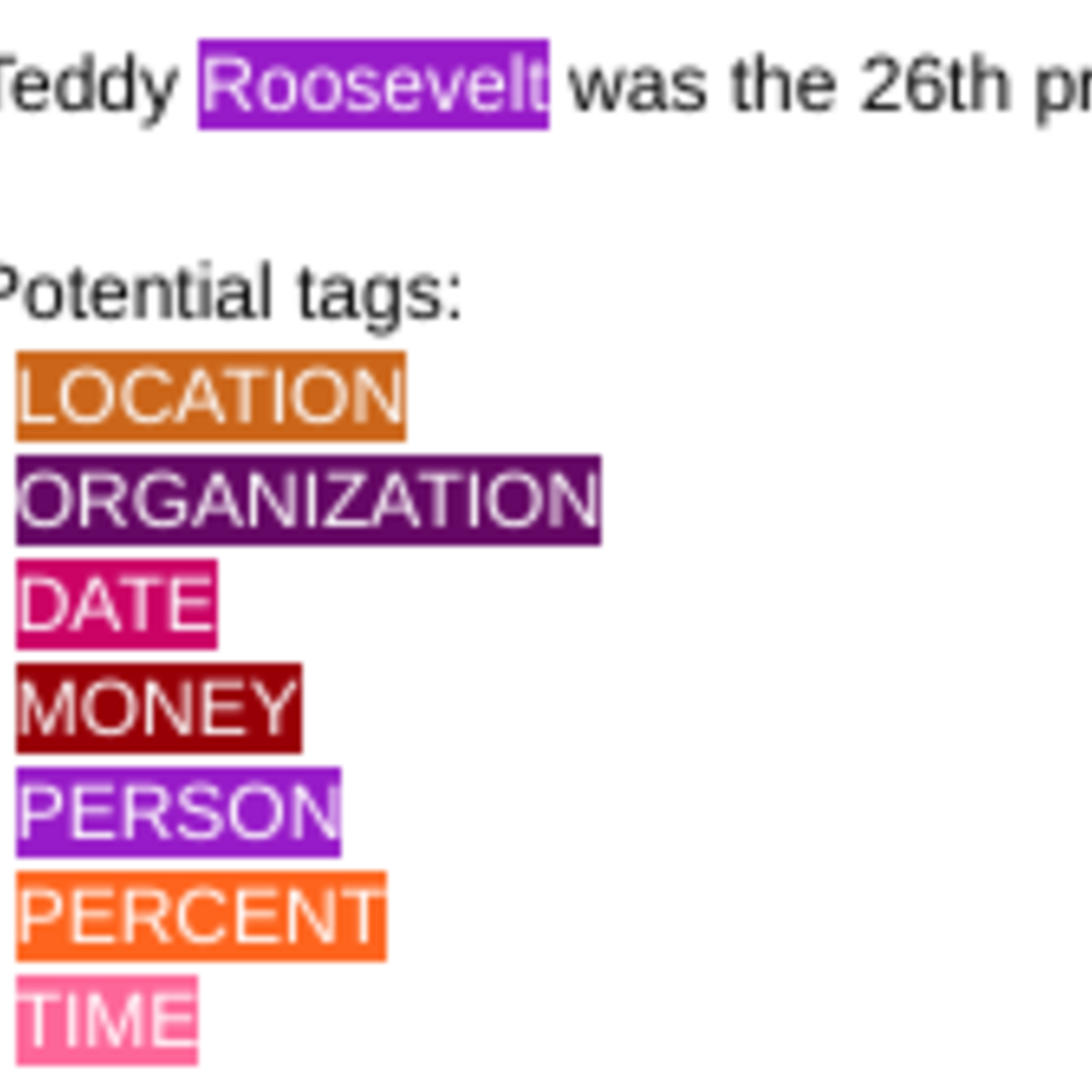
Named Entity Recognition using LSTMs with Keras
In this 1-hour long project-based course, you will use the Keras API with TensorFlow as its backend to build and train a bidirectional LSTM neural network model to recognize named entities in text data. Named entity recognition models can be used to identify mentions of people, locations, organizations, etc. Named entity recognition is not only a standalone tool for information extraction, but it also an invaluable preprocessing step for many downstream natural language processing applications like machine translation, question answering, and text summarization.
This course runs on Coursera's hands-on project platform called Rhyme. On Rhyme, you do projects in a hands-on manner in your browser. You will get instant access to pre-configured cloud desktops containing all of the software and data you need for the project. Everything is already set up directly in your internet browser so you can just focus on learning. For this project, you’ll get instant access to a cloud desktop with Python, Jupyter, and Keras pre-installed.
Notes:
- You will be able to access the cloud desktop 5 times. However, you will be able to access instructions videos as many times as you want.
- This course works best for learners who are based in the North America region. We’re currently working on providing the same experience in other regions.
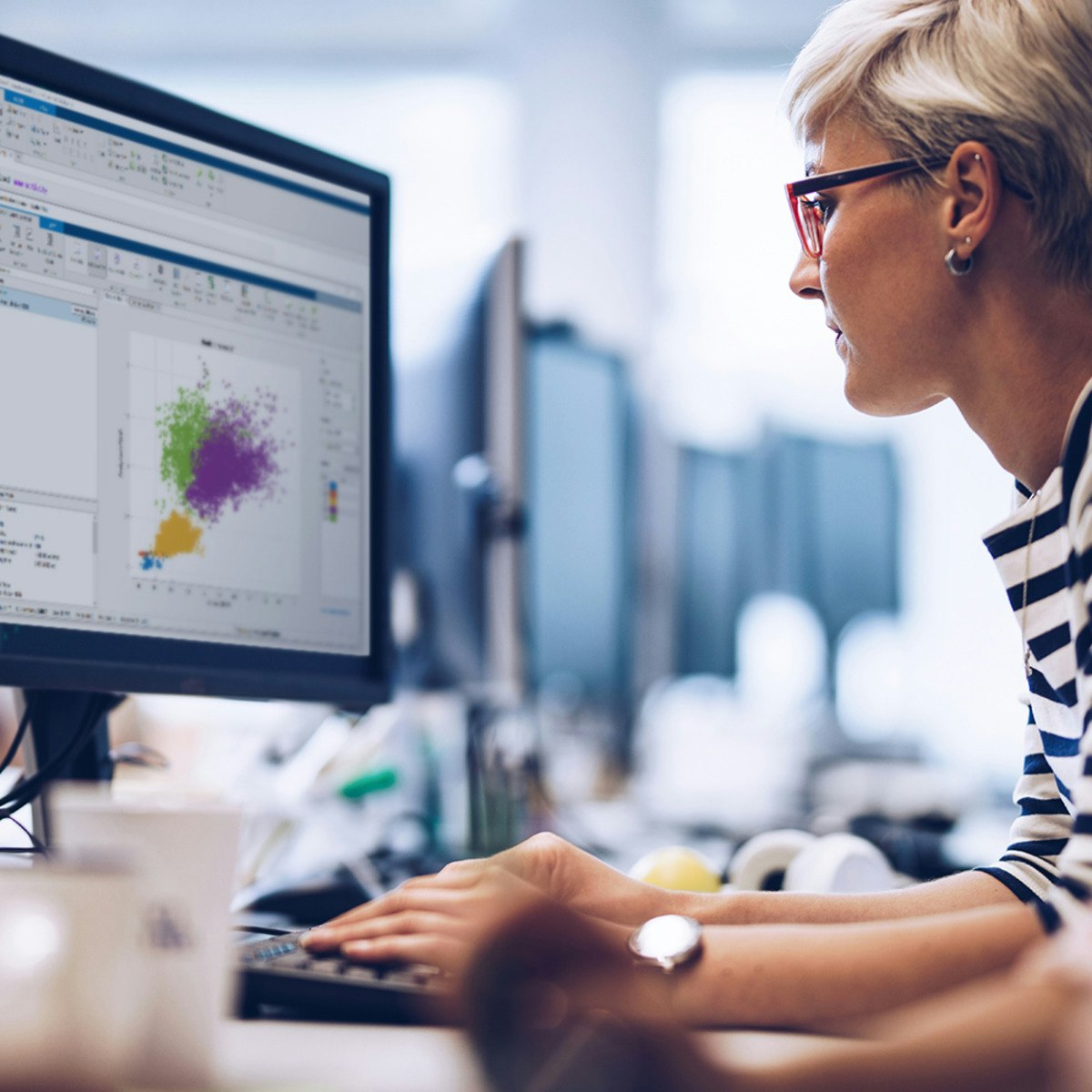
Predictive Modeling and Machine Learning with MATLAB
In this course, you will build on the skills learned in Exploratory Data Analysis with MATLAB and Data Processing and Feature Engineering with MATLAB to increase your ability to harness the power of MATLAB to analyze data relevant to the work you do.
These skills are valuable for those who have domain knowledge and some exposure to computational tools, but no programming background. To be successful in this course, you should have some background in basic statistics (histograms, averages, standard deviation, curve fitting, interpolation) and have completed courses 1 through 2 of this specialization.
By the end of this course, you will use MATLAB to identify the best machine learning model for obtaining answers from your data. You will prepare your data, train a predictive model, evaluate and improve your model, and understand how to get the most out of your models.
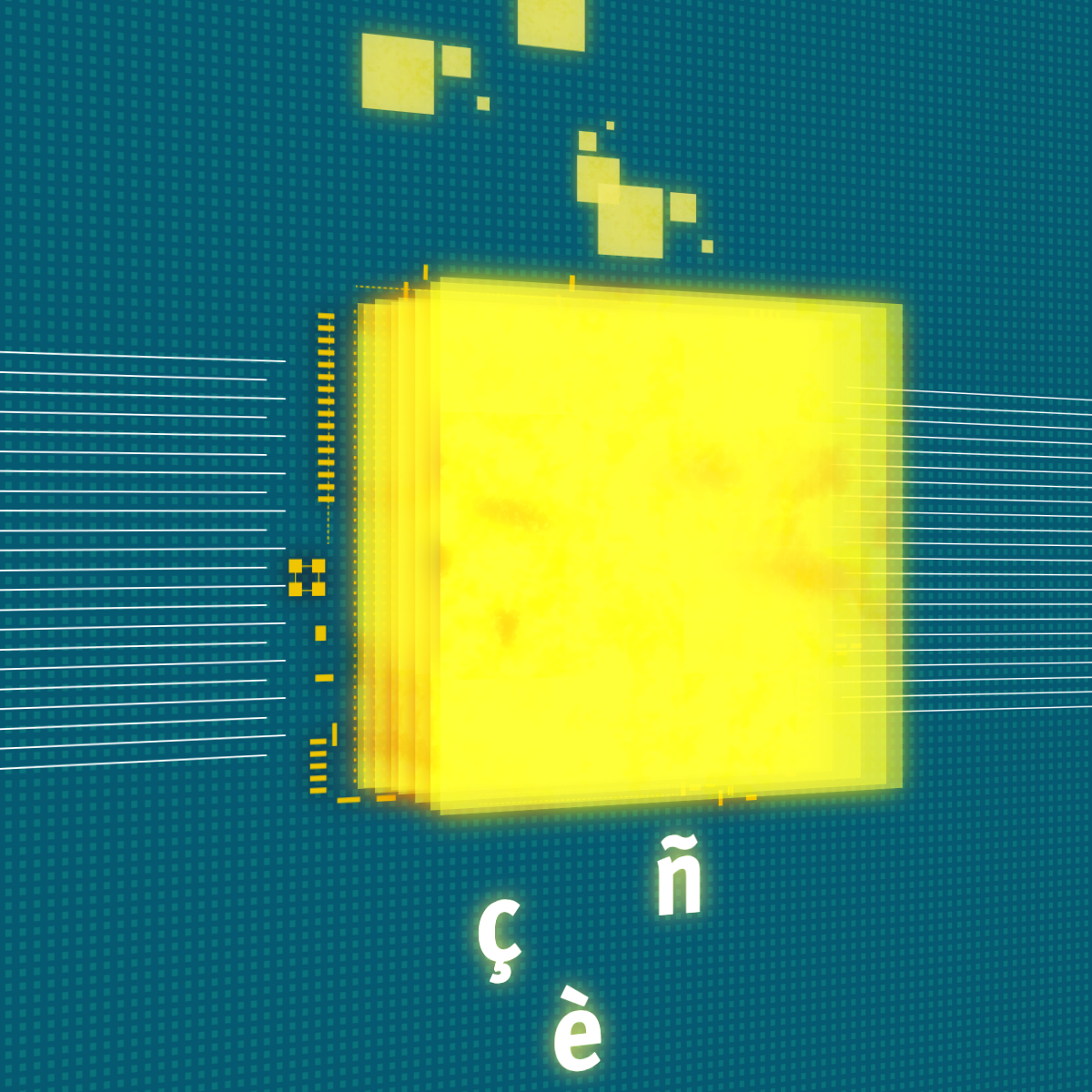
Customising your models with TensorFlow 2
Welcome to this course on Customising your models with TensorFlow 2!
In this course you will deepen your knowledge and skills with TensorFlow, in order to develop fully customised deep learning models and workflows for any application. You will use lower level APIs in TensorFlow to develop complex model architectures, fully customised layers, and a flexible data workflow. You will also expand your knowledge of the TensorFlow APIs to include sequence models.
You will put concepts that you learn about into practice straight away in practical, hands-on coding tutorials, which you will be guided through by a graduate teaching assistant. In addition there is a series of automatically graded programming assignments for you to consolidate your skills.
At the end of the course, you will bring many of the concepts together in a Capstone Project, where you will develop a custom neural translation model from scratch.
TensorFlow is an open source machine library, and is one of the most widely used frameworks for deep learning. The release of TensorFlow 2 marks a step change in the product development, with a central focus on ease of use for all users, from beginner to advanced level.
This course follows on directly from the previous course Getting Started with TensorFlow 2. The additional prerequisite knowledge required in order to be successful in this course is proficiency in the python programming language, (this course uses python 3), knowledge of general machine learning concepts (such as overfitting/underfitting, supervised learning tasks, validation, regularisation and model selection), and a working knowledge of the field of deep learning, including typical model architectures (MLP, CNN, RNN, ResNet), and concepts such as transfer learning, data augmentation and word embeddings.
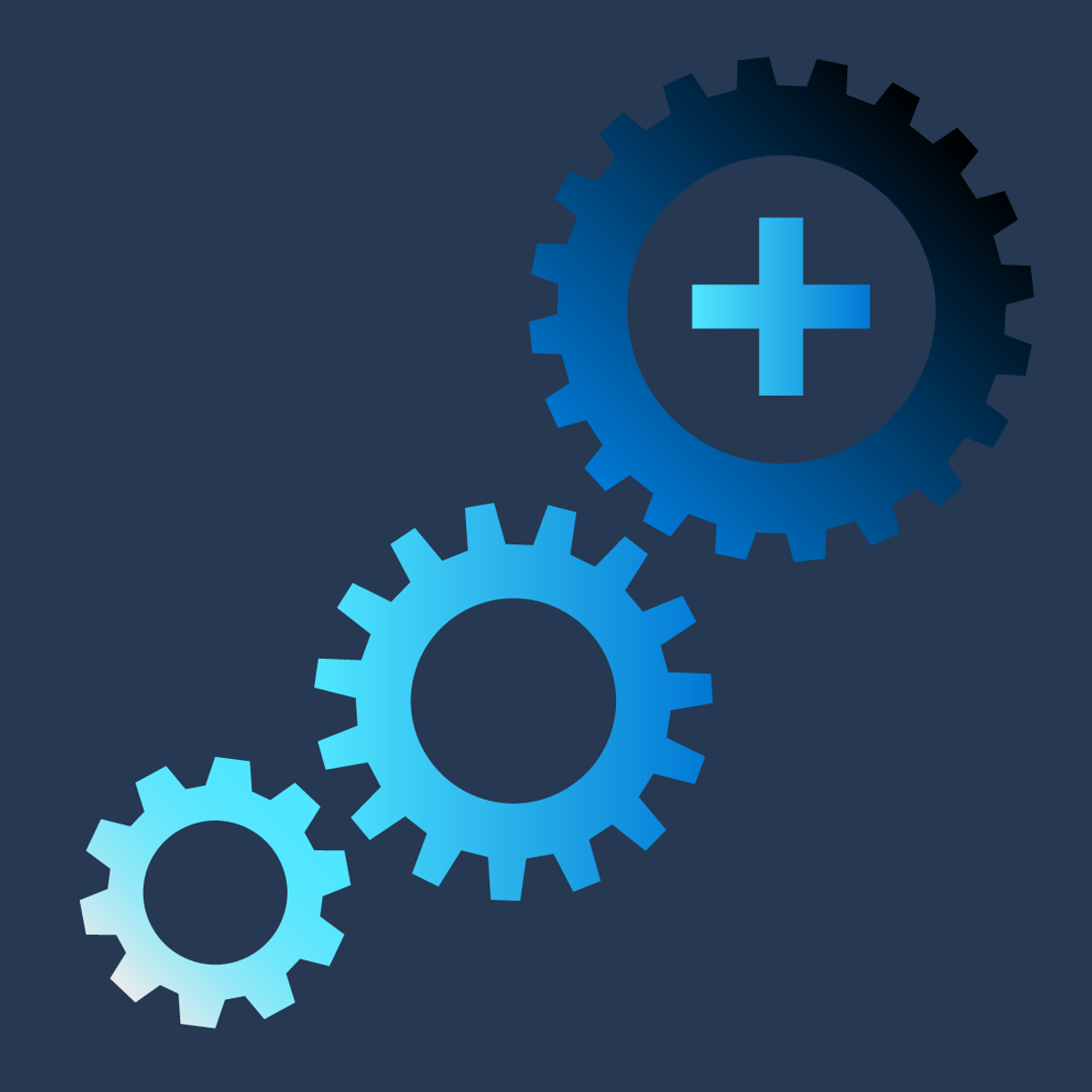
Microsoft Azure Machine Learning
Machine learning is at the core of artificial intelligence, and many modern applications and services depend on predictive machine learning models. Training a machine learning model is an iterative process that requires time and compute resources. Automated machine learning can help make it easier. In this course, you will learn how to use Azure Machine Learning to create and publish models without writing code.
This course will help you prepare for Exam AI-900: Microsoft Azure AI Fundamentals. This is the second course in a five-course program that prepares you to take the AI-900 certification exam. This course teaches you the core concepts and skills that are assessed in the AI fundamentals exam domains. This beginner course is suitable for IT personnel who are just beginning to work with Microsoft Azure and want to learn about Microsoft Azure offerings and get hands-on experience with the product. Microsoft Azure AI Fundamentals can be used to prepare for other Azure role-based certifications like Microsoft Azure Data Scientist Associate or Microsoft Azure AI Engineer Associate, but it is not a prerequisite for any of them.
This course is intended for candidates with both technical and non-technical backgrounds. Data science and software engineering experience is not required; however, some general programming knowledge or experience would be beneficial. To be successful in this course, you need to have basic computer literacy and proficiency in the English language. You should be familiar with basic computing concepts and terminology, general technology concepts, including concepts of machine learning and artificial intelligence.
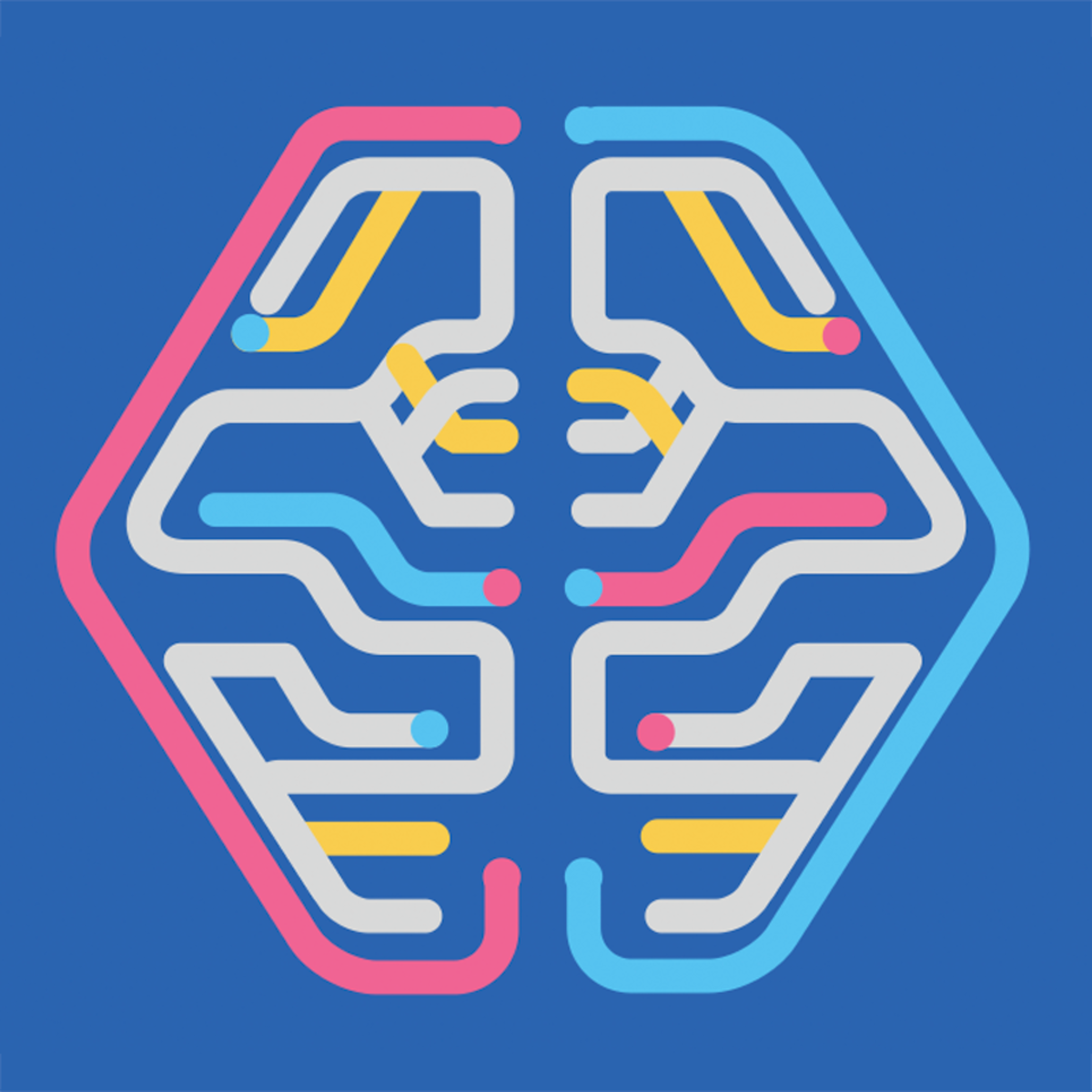
Launching into Machine Learning
The course begins with a discussion about data: how to improve data quality and perform exploratory data analysis. We describe Vertex AI AutoML and how to build, train, and deploy an ML model without writing a single line of code. You will understand the benefits of Big Query ML. We then discuss how to optimize a machine learning (ML) model and how generalization and sampling can help assess the quality of ML models for custom training.
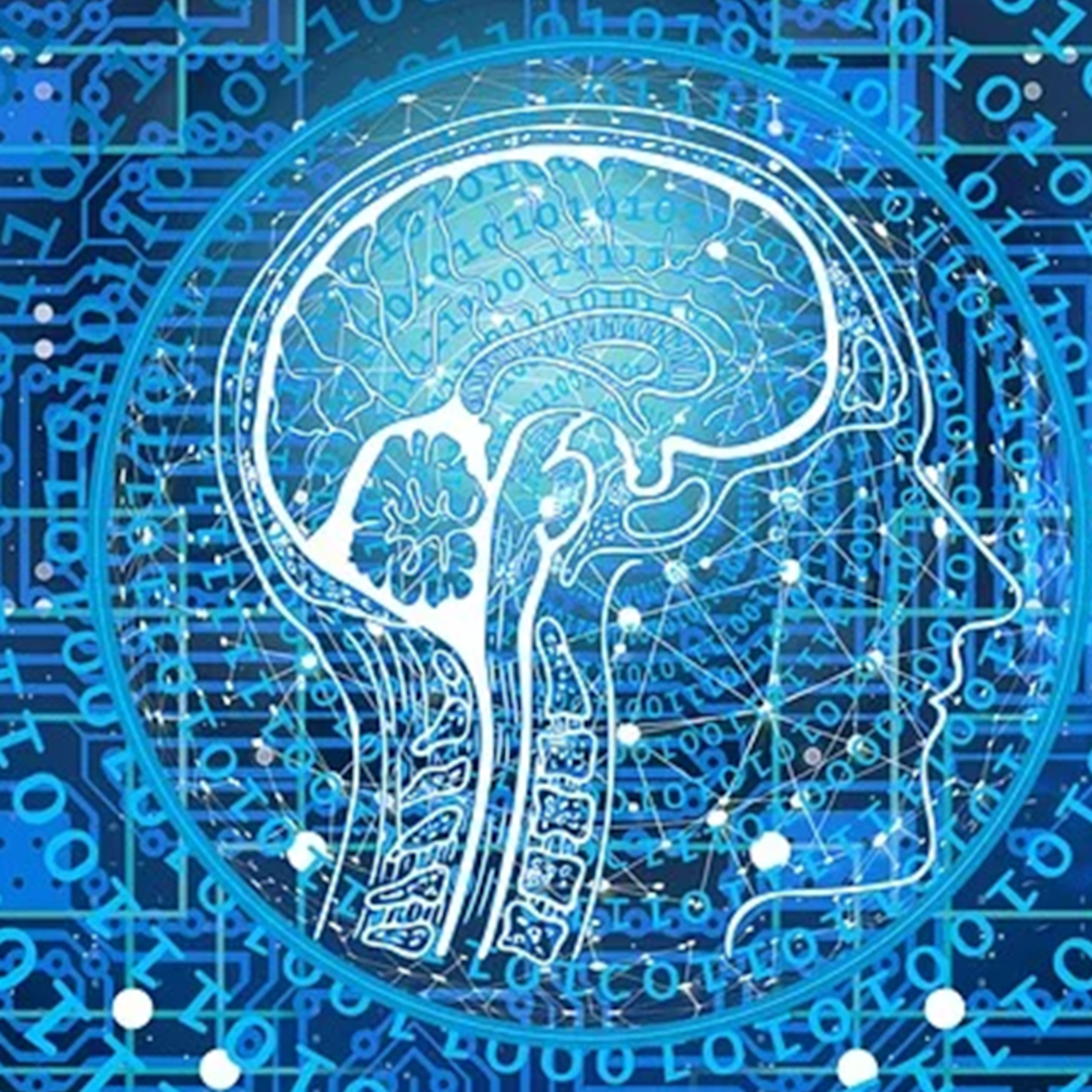
Exploratory Data Analysis for Machine Learning
This first course in the IBM Machine Learning Professional Certificate introduces you to Machine Learning and the content of the professional certificate. In this course you will realize the importance of good, quality data. You will learn common techniques to retrieve your data, clean it, apply feature engineering, and have it ready for preliminary analysis and hypothesis testing.
By the end of this course you should be able to:
Retrieve data from multiple data sources: SQL, NoSQL databases, APIs, Cloud
Describe and use common feature selection and feature engineering techniques
Handle categorical and ordinal features, as well as missing values
Use a variety of techniques for detecting and dealing with outliers
Articulate why feature scaling is important and use a variety of scaling techniques
Who should take this course?
This course targets aspiring data scientists interested in acquiring hands-on experience with Machine Learning and Artificial Intelligence in a business setting.
What skills should you have?
To make the most out of this course, you should have familiarity with programming on a Python development environment, as well as fundamental understanding of Calculus, Linear Algebra, Probability, and Statistics.
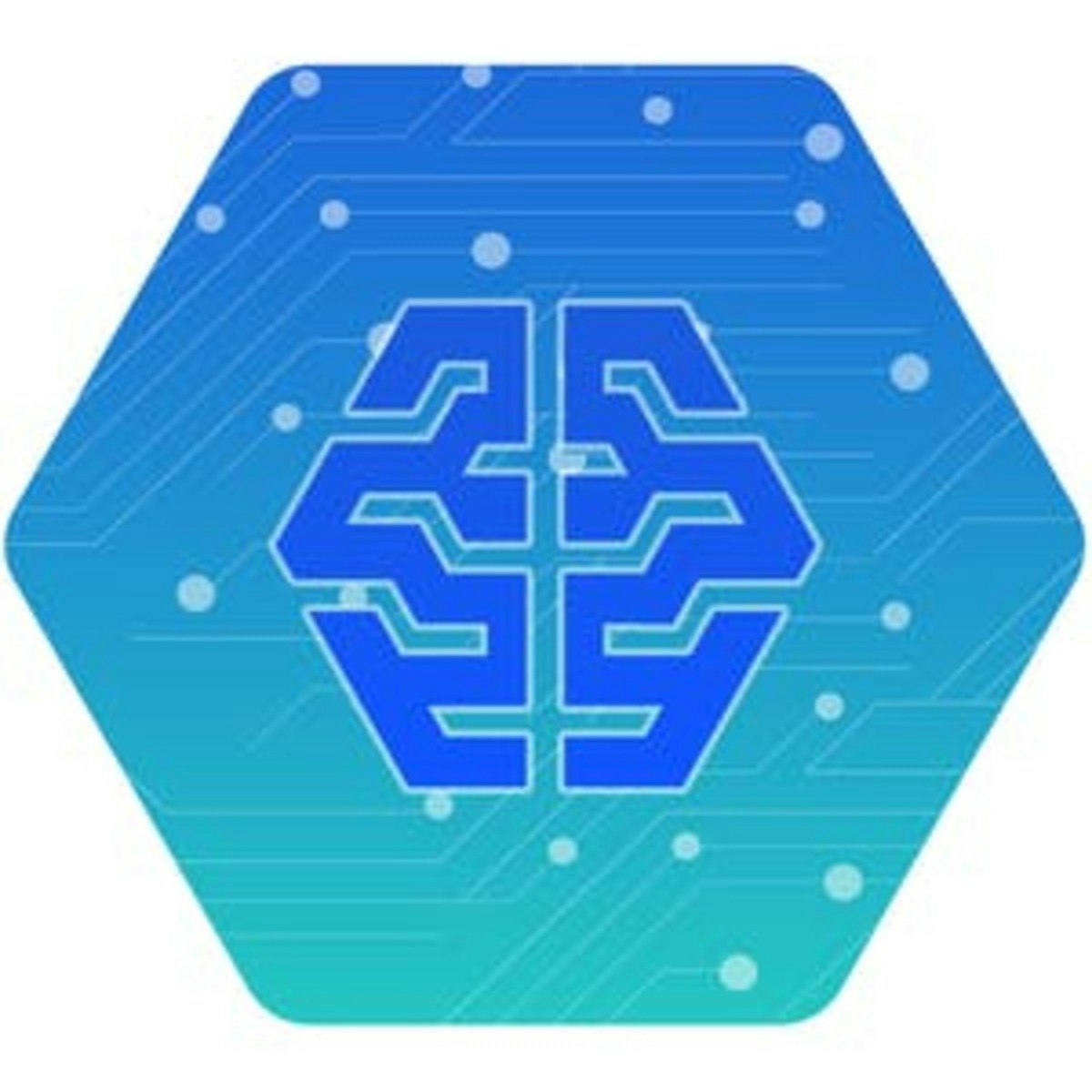
Smart Analytics, Machine Learning, and AI on GCP
Incorporating machine learning into data pipelines increases the ability of businesses to extract insights from their data. This course covers several ways machine learning can be included in data pipelines on Google Cloud depending on the level of customization required. For little to no customization, this course covers AutoML. For more tailored machine learning capabilities, this course introduces Notebooks and BigQuery machine learning (BigQuery ML). Also, this course covers how to productionalize machine learning solutions using Vertex AI. Learners will get hands-on experience building machine learning models on Google Cloud using QwikLabs.
Popular Internships and Jobs by Categories
Browse
© 2024 BoostGrad | All rights reserved