Back to Courses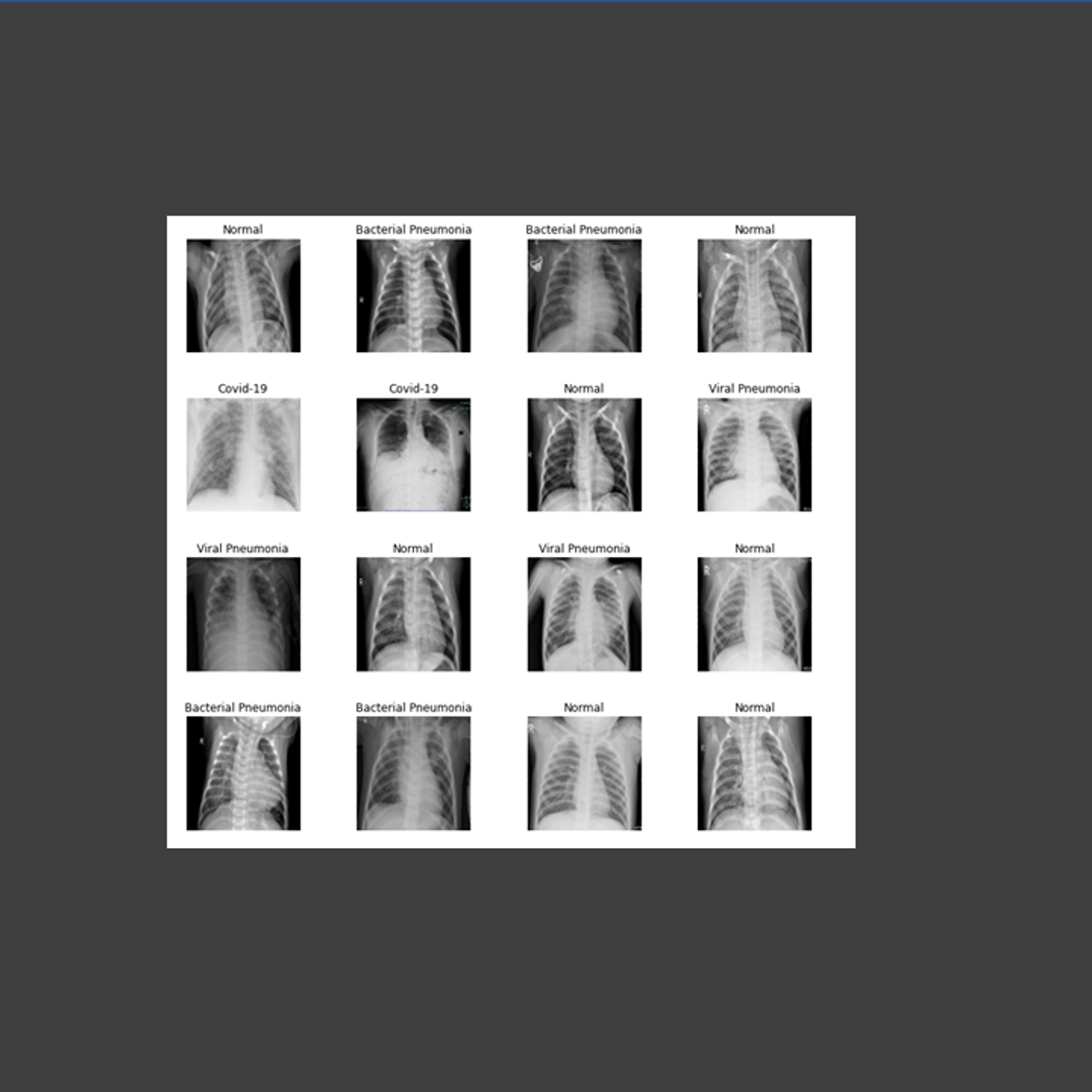
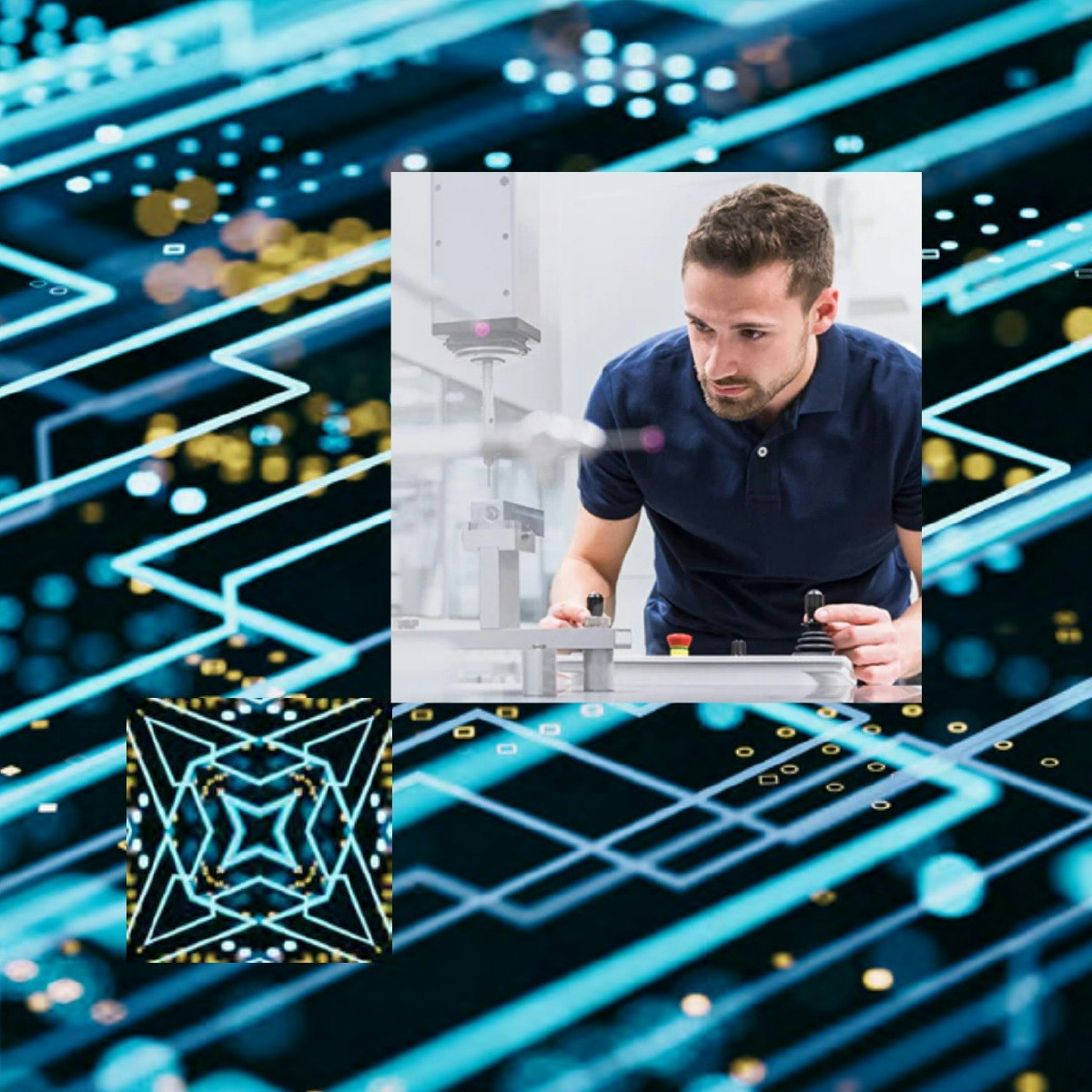
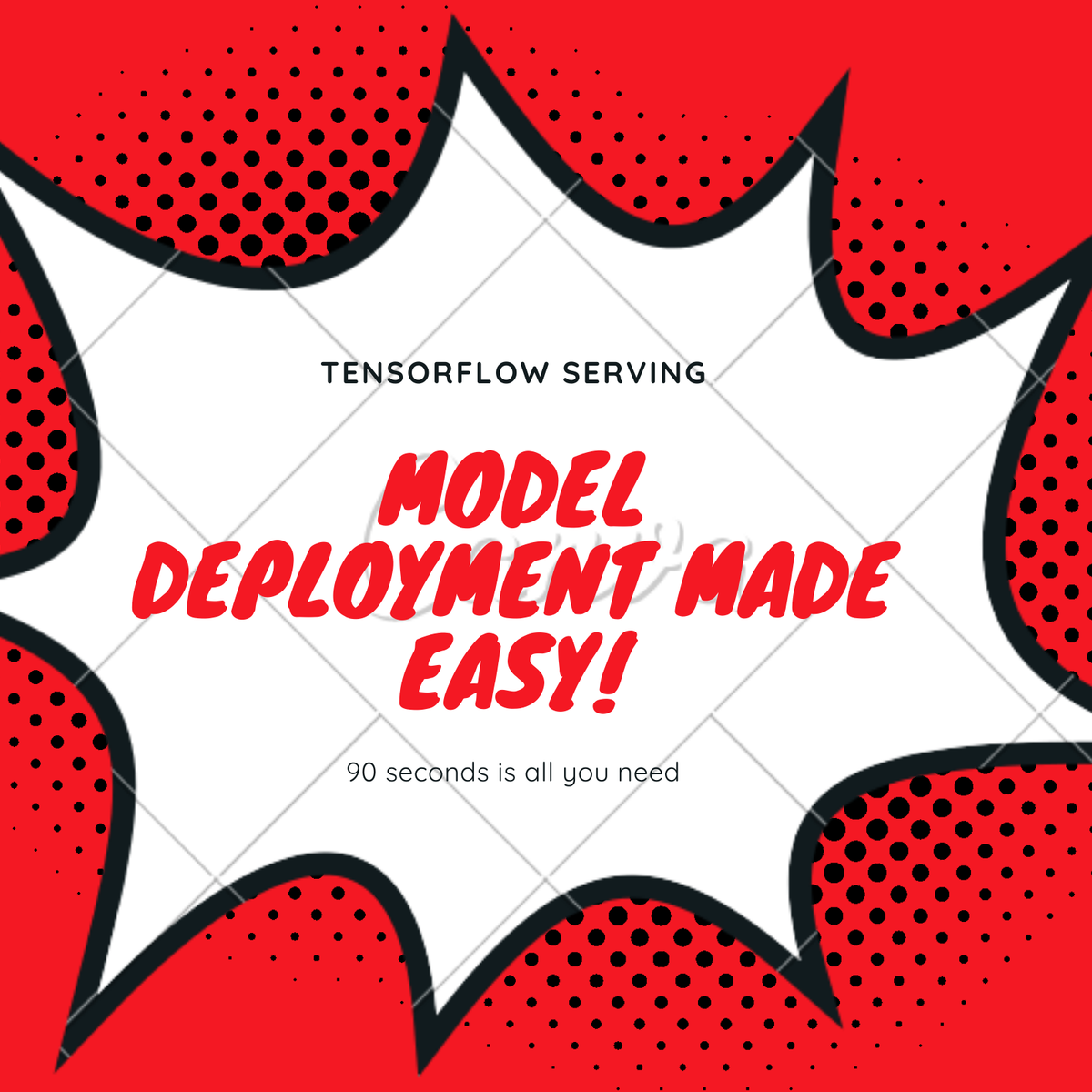
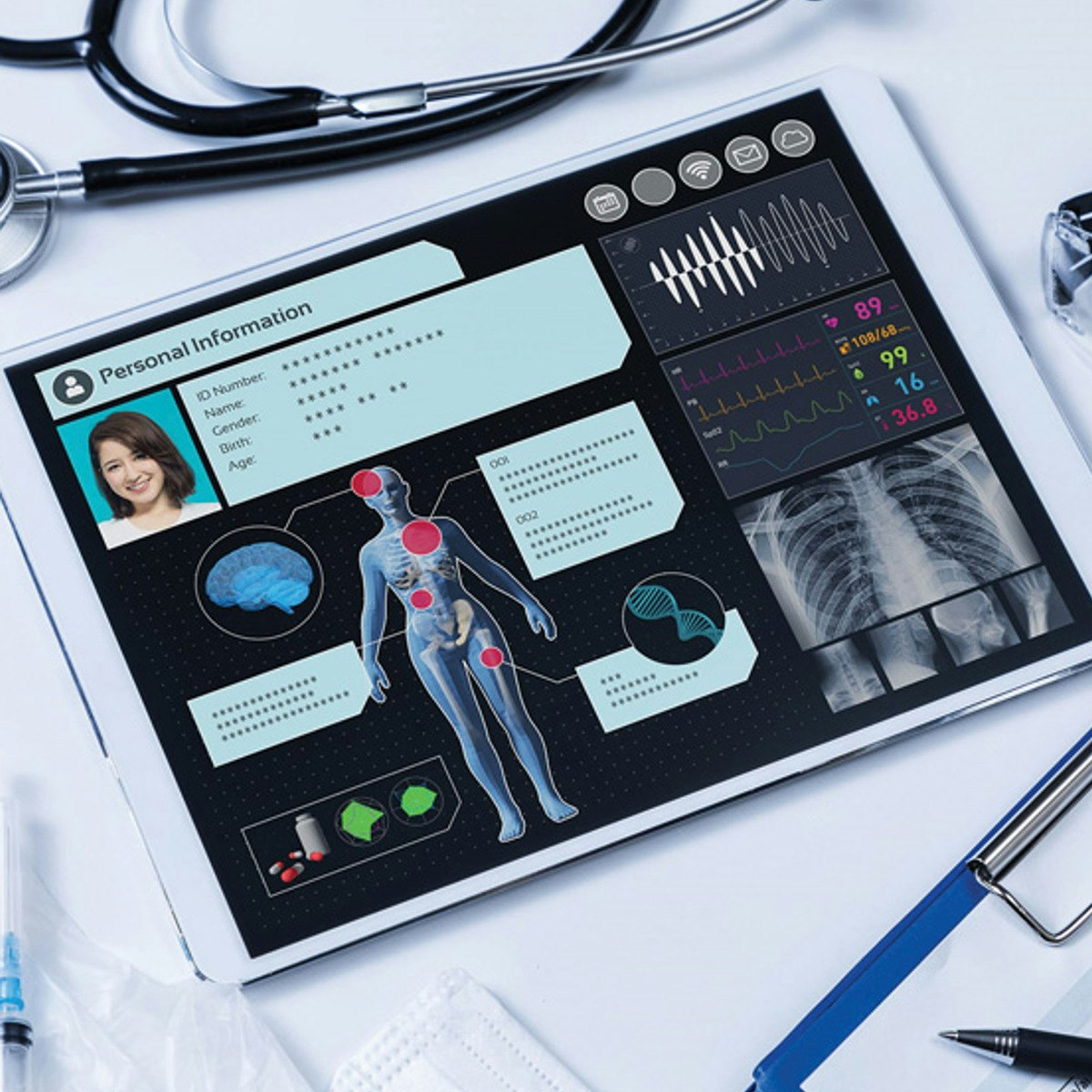
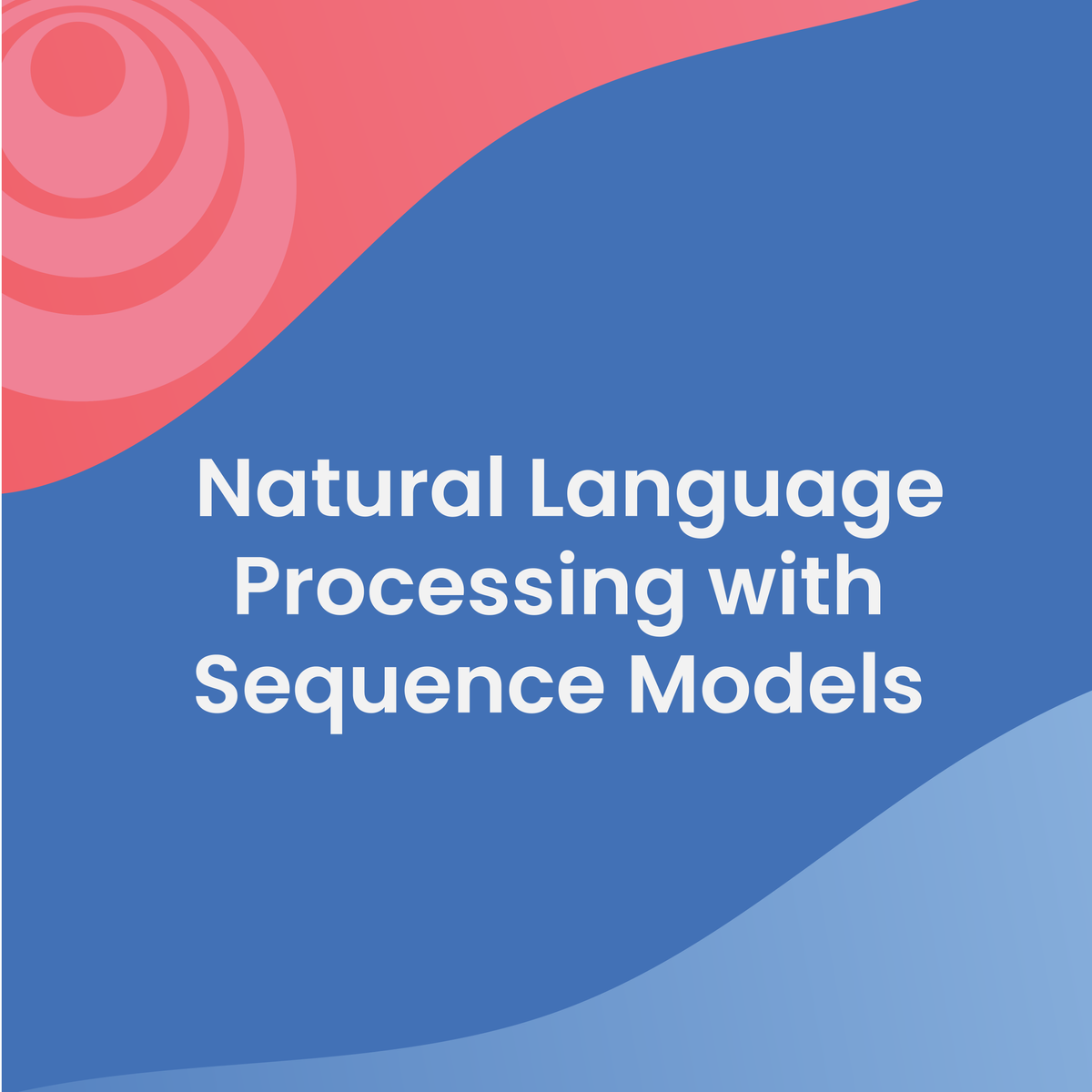
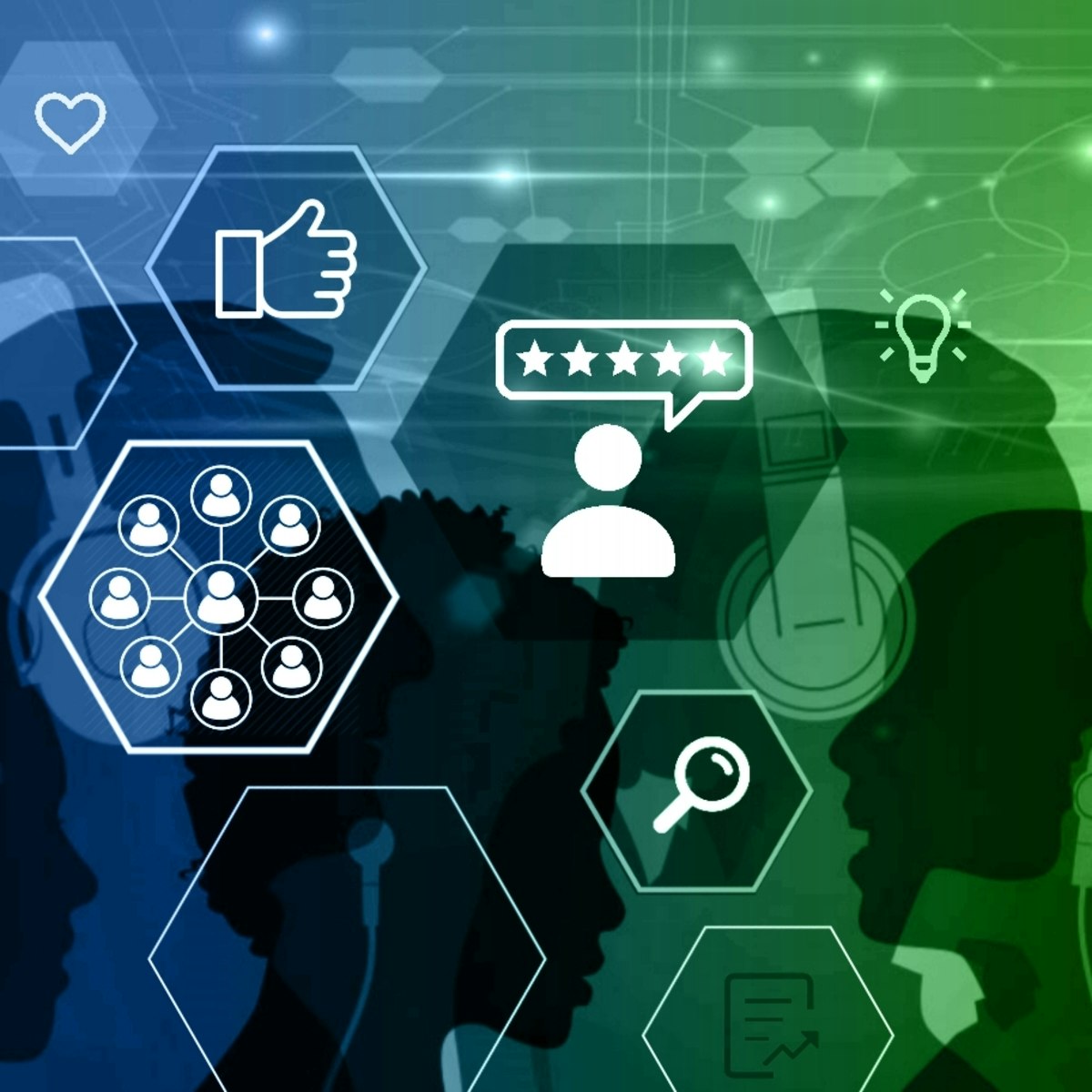
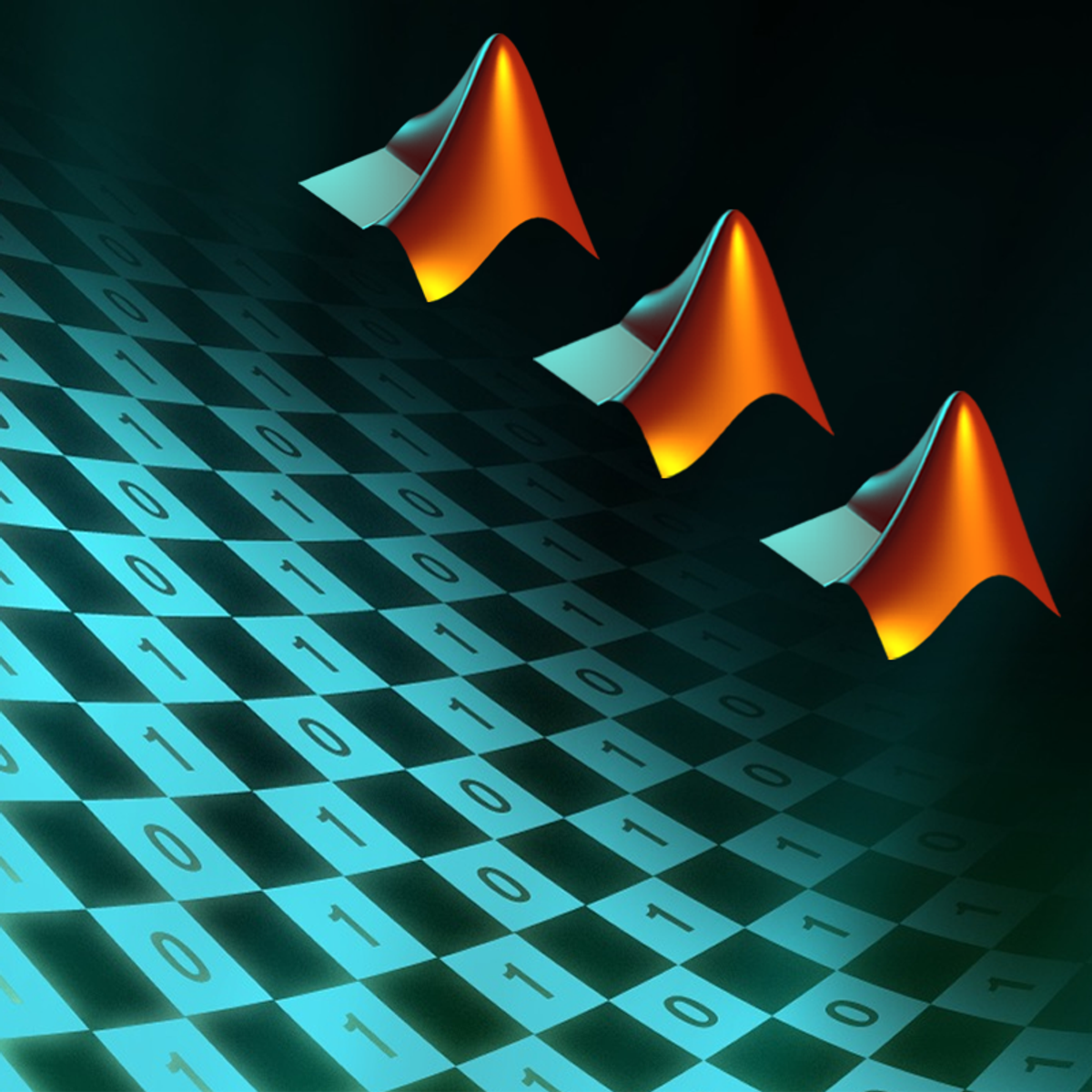
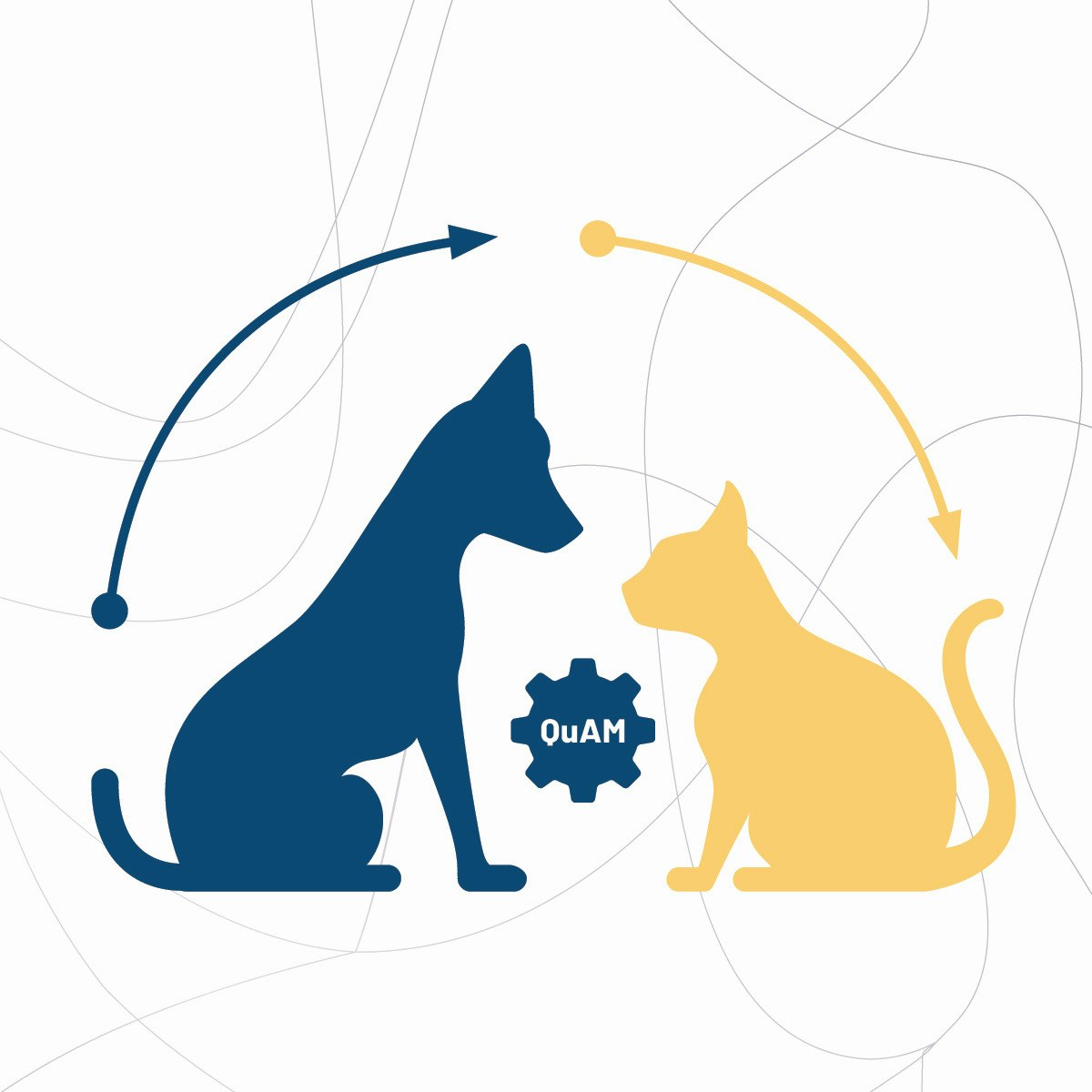
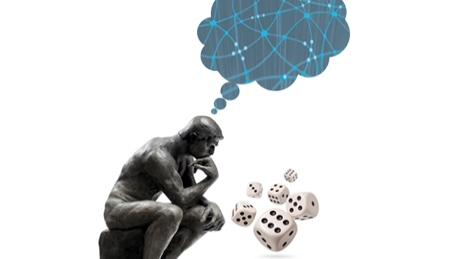
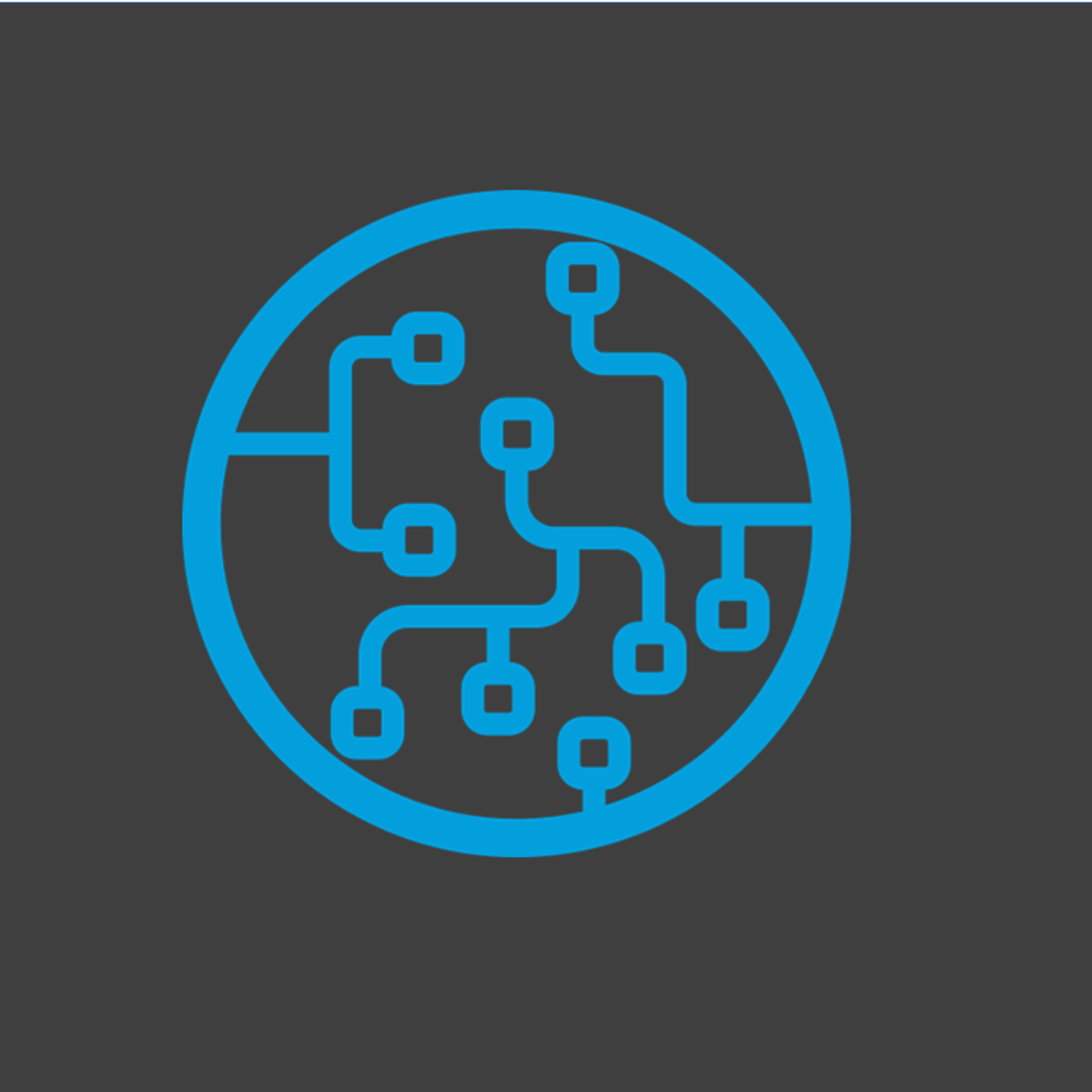
Machine Learning Courses - Page 39
Showing results 381-390 of 485
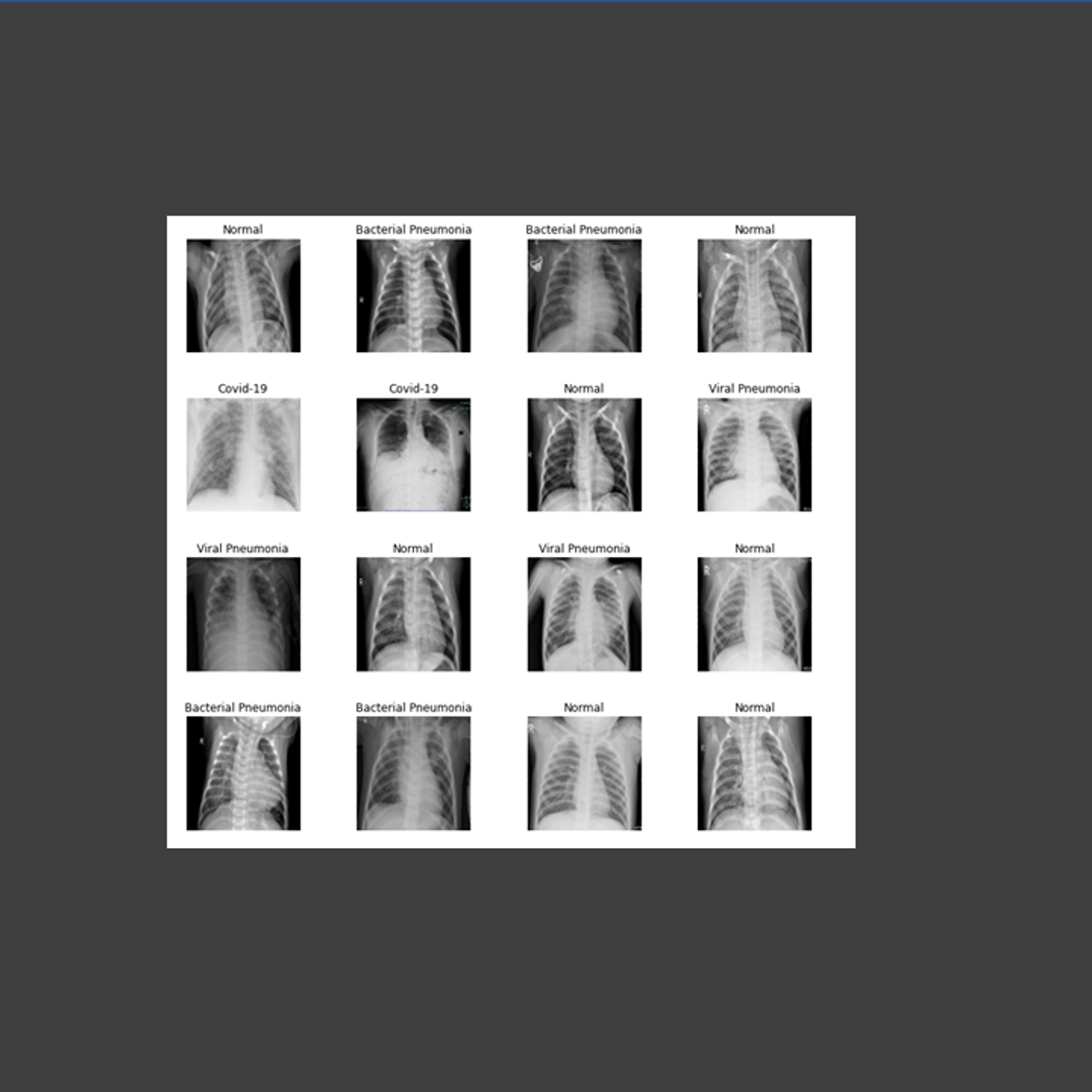
AI-Powered Chest Disease Detection and Classification
Hello everyone and welcome to this hands-on guided project on Artificial intelligence (AI)-powered chest disease detection and classification. AI has been revolutionizing healthcare and medicine in many areas such as: (1) Medical imagery, (2) Drug research, and (3) Genome development. Deep learning has been proven to be superior in detecting and classifying disease using imagery data.
In this case study, we will automate the process of detecting and classifying chest disease from X-Ray images to reduce the cost and time of detection. This guided project is practical and directly applicable to the healthcare industry. You can add this project to your portfolio of projects which is essential for your next job interview.
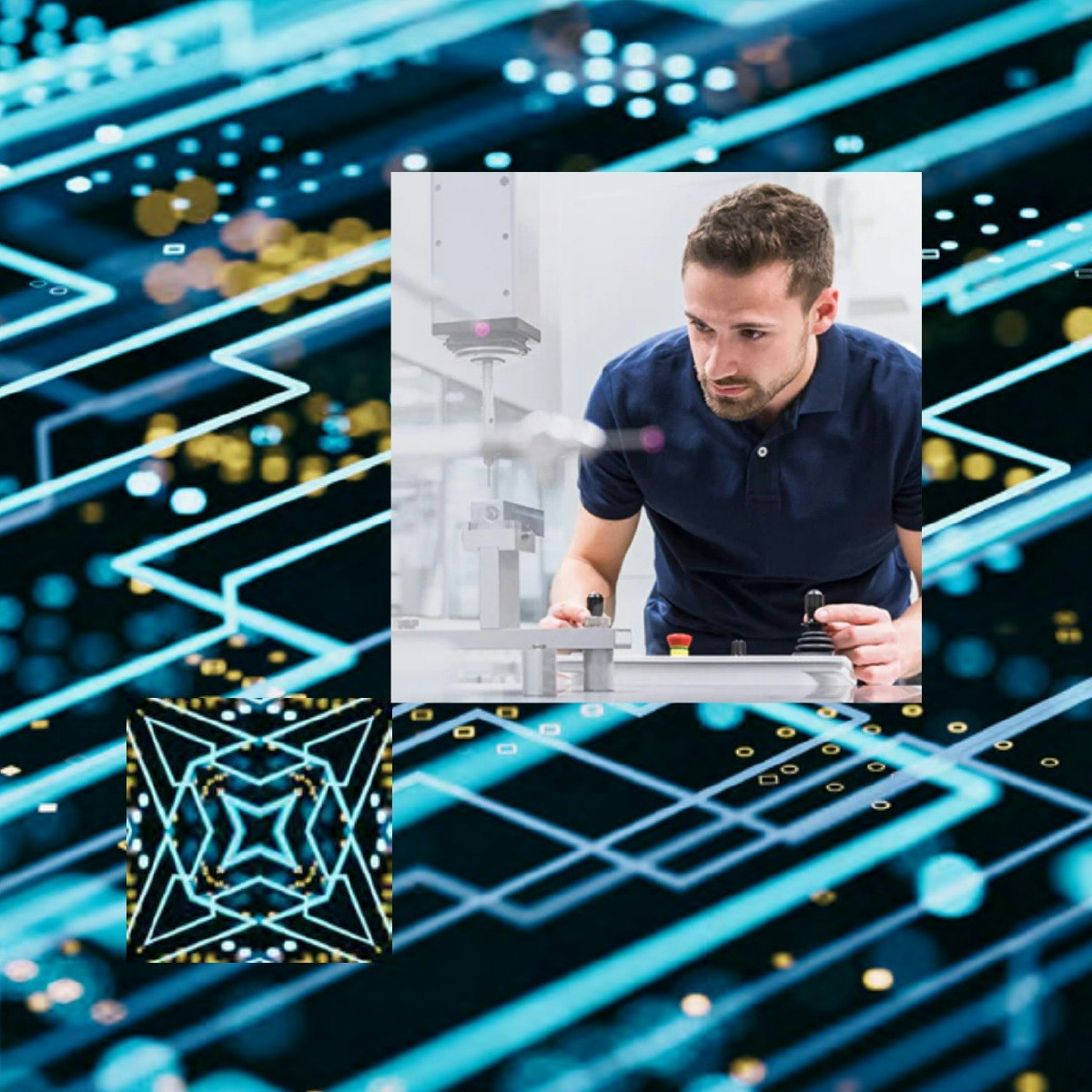
Building Autonomous AI
Practice makes perfect. It’s true for people learning to master a new skill, and it’s also true for your AI brain. Just as you need the right environment to practice, get feedback and try again, so does your AI brain.
In this course, you’ll solve industrial engineering problems inspired by real problems your instructors have worked on in industry. You’ll learn how to build, test and deploy an AI brain using Microsoft Bonsai, a cloud-based, low-code platform. We’ll walk through the entire Bonsai platform from setup to deployment. Along the way, you’ll use Bonsai to conduct machine teaching experimentation to train a brain and assess its progress. Because you’ll be teaching the brain a relatively complex task, you’ll run multiple simulations until you’re satisfied with the results. You’ll then prep the brain for graduation into the real world — deploying it into a machinery control system or other live environment.
At the end of this course, you’ll be able to:
• Build an autonomous AI that combines reinforcement learning with machine learning, expert rules and other methods that you’ve used in the first two courses of the specialization
• Establish requirements for a simulated environment for your brain to practice a task
• Validate and assess your brain’s performance of a task and make improvements to your brain design
• Evaluate whether a simulator is a good practice environment
• Deploy a brain on a real piece of hardware
This course requires an Azure subscription.
This course is part of a specialization called Autonomous AI for Industry, which will launch in early 2023.
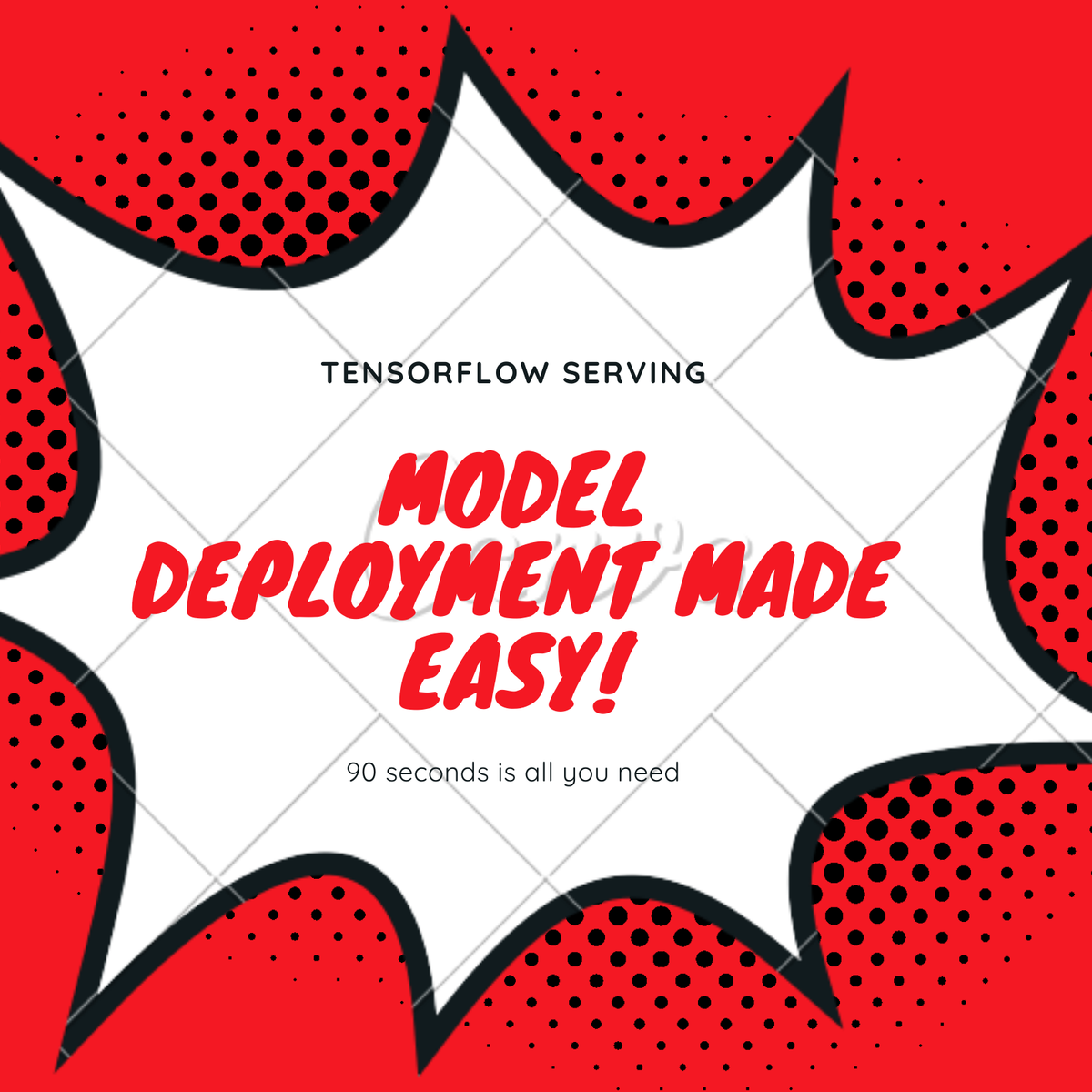
TensorFlow Serving with Docker for Model Deployment
This is a hands-on, guided project on deploying deep learning models using TensorFlow Serving with Docker. In this 1.5 hour long project, you will train and export TensorFlow models for text classification, learn how to deploy models with TF Serving and Docker in 90 seconds, and build simple gRPC and REST-based clients in Python for model inference.
With the worldwide adoption of machine learning and AI by organizations, it is becoming increasingly important for data scientists and machine learning engineers to know how to deploy models to production. While DevOps groups are fantastic at scaling applications, they are not the experts in ML ecosystems such as TensorFlow and PyTorch. This guided project gives learners a solid, real-world foundation of pushing your TensorFlow models from development to production in no time!
Prerequisites:
In order to successfully complete this project, you should be familiar with Python, and have prior experience with building models with Keras or TensorFlow.
Note: This course works best for learners who are based in the North America region. We’re currently working on providing the same experience in other regions.
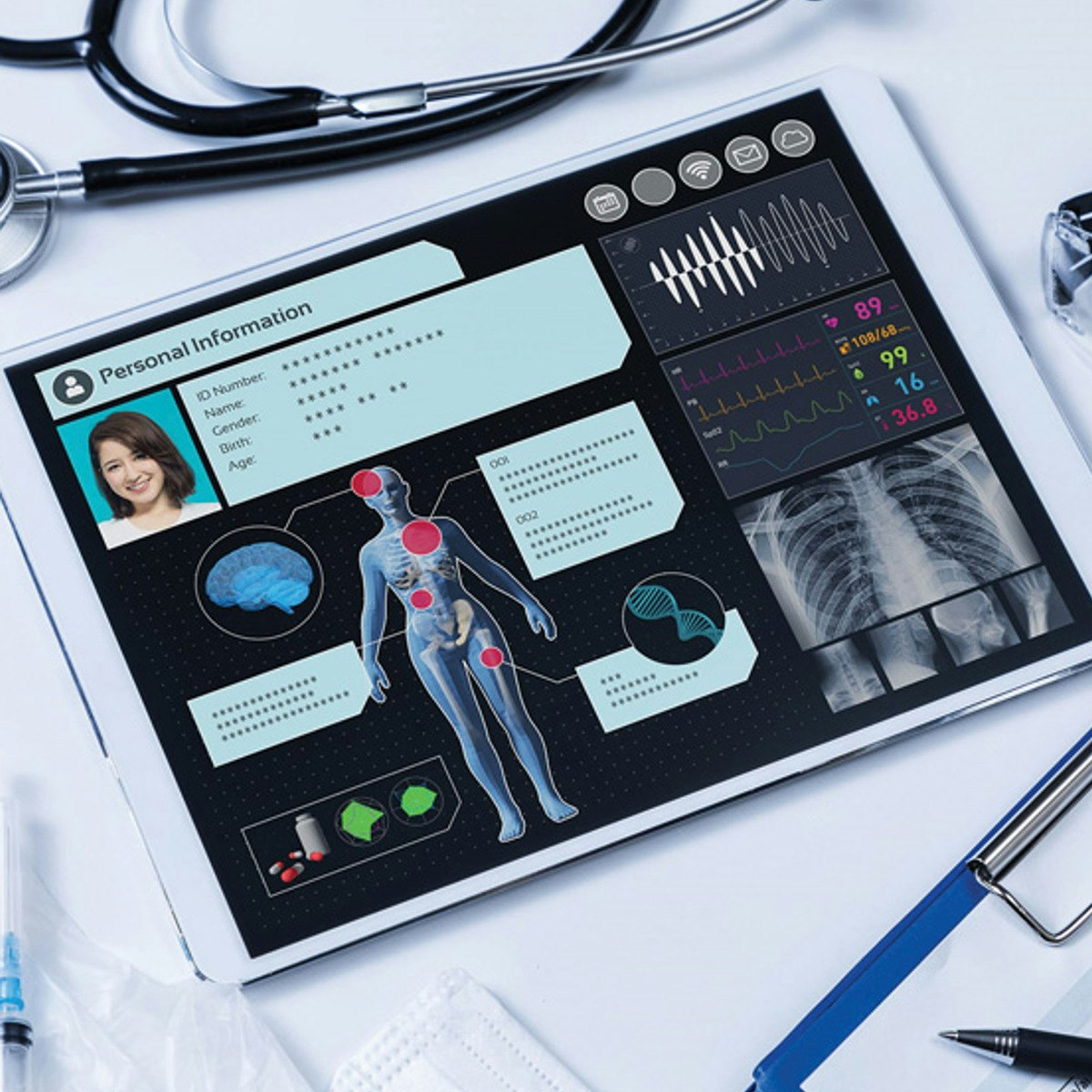
Data mining of Clinical Databases - CDSS 1
This course will introduce MIMIC-III, which is the largest publicly Electronic Health Record (EHR) database available to benchmark machine learning algorithms. In particular, you will learn about the design of this relational database, what tools are available to query, extract and visualise descriptive analytics.
The schema and International Classification of Diseases coding is important to understand how to map research questions to data and how to extract key clinical outcomes in order to develop clinically useful machine learning algorithms.
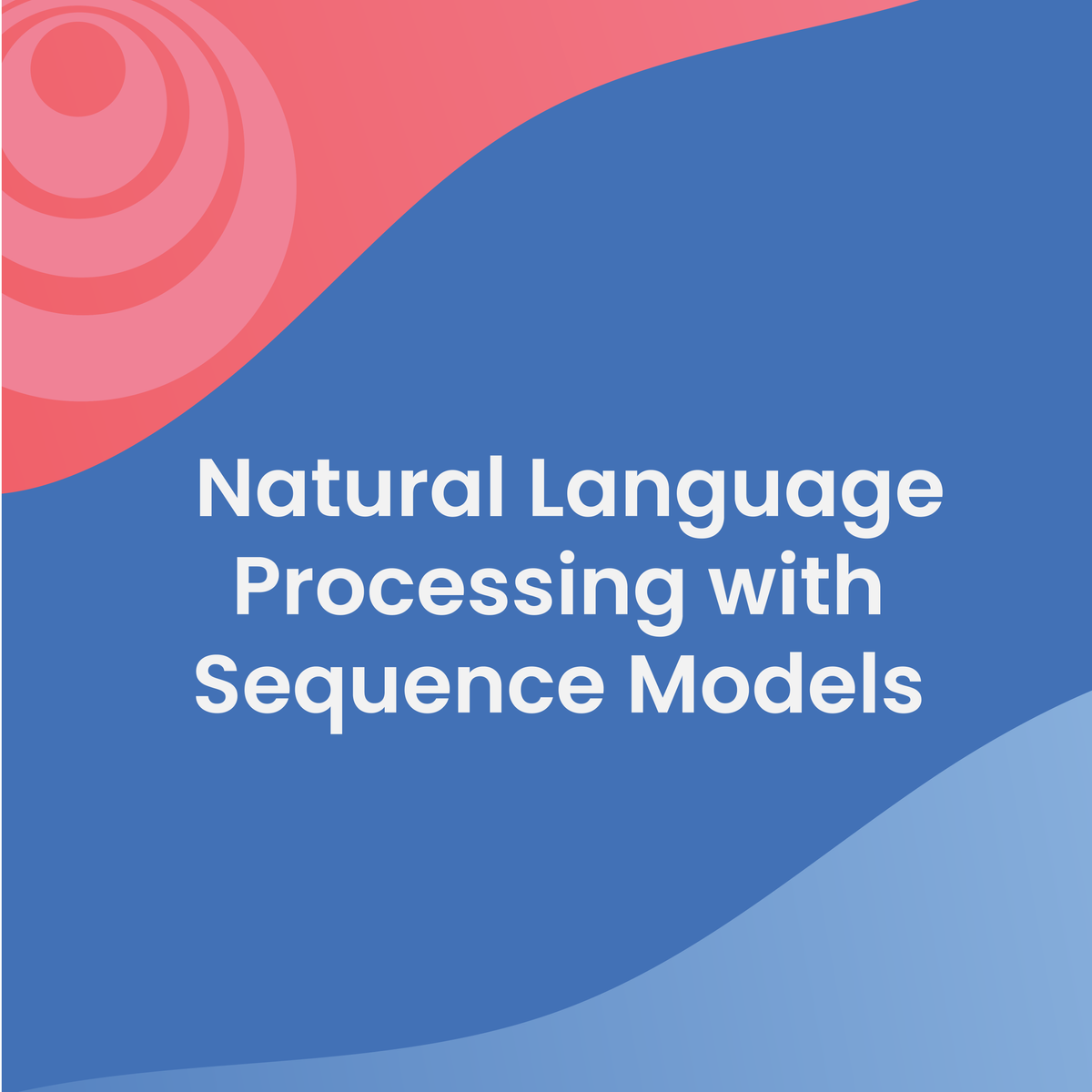
Natural Language Processing with Sequence Models
In Course 3 of the Natural Language Processing Specialization, you will:
a) Train a neural network with GLoVe word embeddings to perform sentiment analysis of tweets,
b) Generate synthetic Shakespeare text using a Gated Recurrent Unit (GRU) language model,
c) Train a recurrent neural network to perform named entity recognition (NER) using LSTMs with linear layers, and
d) Use so-called ‘Siamese’ LSTM models to compare questions in a corpus and identify those that are worded differently but have the same meaning.
By the end of this Specialization, you will have designed NLP applications that perform question-answering and sentiment analysis, created tools to translate languages and summarize text, and even built a chatbot!
This Specialization is designed and taught by two experts in NLP, machine learning, and deep learning. Younes Bensouda Mourri is an Instructor of AI at Stanford University who also helped build the Deep Learning Specialization. Łukasz Kaiser is a Staff Research Scientist at Google Brain and the co-author of Tensorflow, the Tensor2Tensor and Trax libraries, and the Transformer paper.
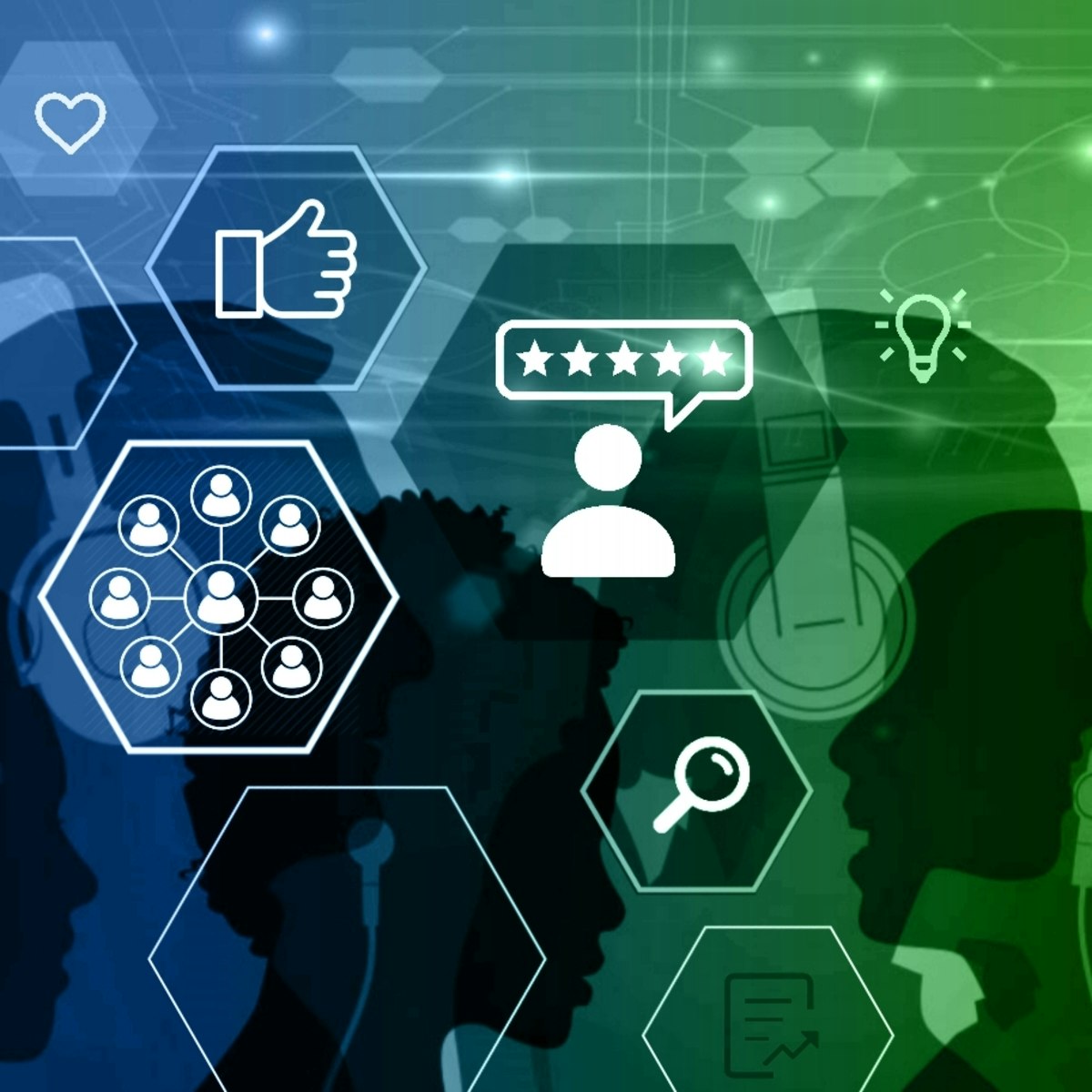
Advanced Recommender Systems
In this course, you will see how to use advanced machine learning techniques to build more sophisticated recommender systems. Machine Learning is able to provide recommendations and make better predictions, by taking advantage of historical opinions from users and building up the model automatically, without the need for you to think about all the details of the model.
At the end of this course, you will learn how to manage hybrid information and how to combine different filtering techniques, taking the best from each approach. You will know how to use factorization machines and represent the input data accordingly. You will be able to design more sophisticated recommender systems, which can solve the cross-domain recommendation problem. You will also learn how to identify new trends and challenges in providing recommendations in a range of innovative application contexts.
This course leverages two important EIT Digital Overarching Learning Outcomes (OLOs), related to your creativity and innovation skills. In trying to design a new recommender system you need to think beyond boundaries and try to figure out how you can improve the quality of the outcomes. You should also be able to use knowledge, ideas and technology to create new or significantly improved recommendation tools to support choice-making processes and solve real-life problems in complex and innovative scenarios.
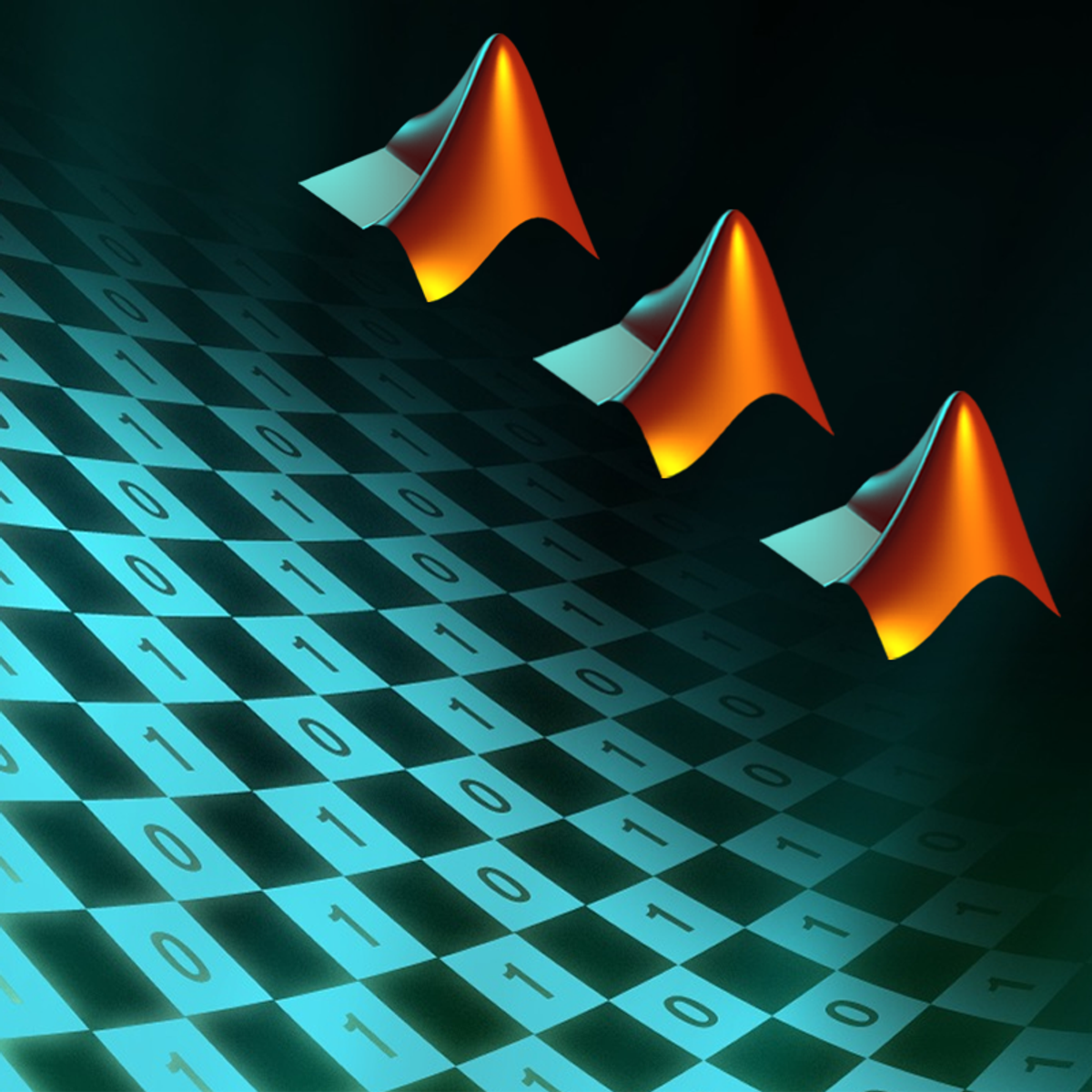
Introduction to Data, Signal, and Image Analysis with MATLAB
Welcome to Introduction to Data, Signal, and Image Analysis with MATLAB!
MATLAB is an extremely versatile programming language for data, signal, and image analysis tasks. This course provides an introduction on how to use MATLAB for data, signal, and image analysis. After completing the course, learners will understand how machine learning methods can be used in MATLAB for data classification and prediction; how to perform data visualization, including data visualization for high dimensional datasets; how to perform image processing and analysis methods, including image filtering and image segmentation; and how to perform common signal analysis tasks, including filter design and frequency analysis.
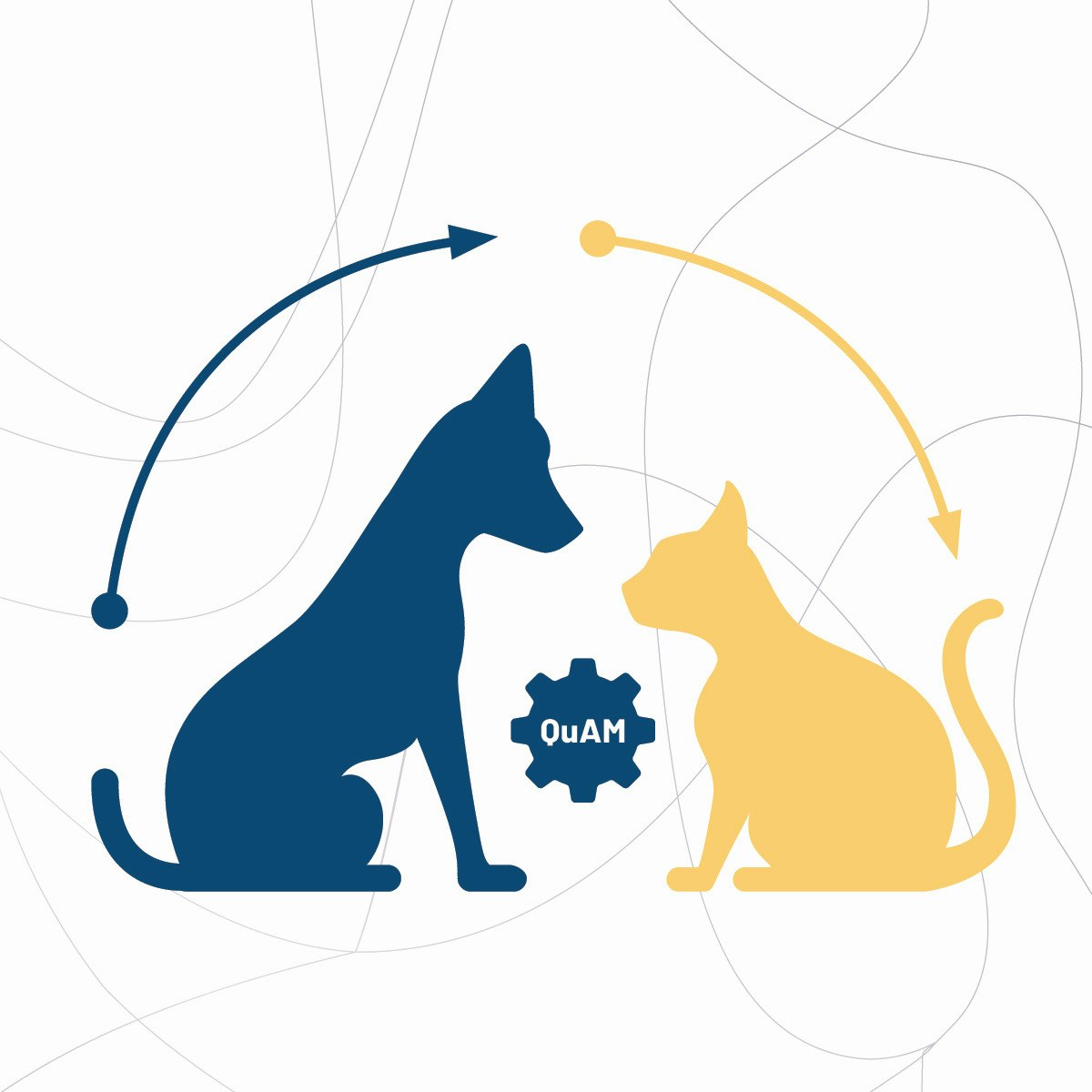
Machine Learning Algorithms: Supervised Learning Tip to Tail
This course takes you from understanding the fundamentals of a machine learning project. Learners will understand and implement supervised learning techniques on real case studies to analyze business case scenarios where decision trees, k-nearest neighbours and support vector machines are optimally used. Learners will also gain skills to contrast the practical consequences of different data preparation steps and describe common production issues in applied ML.
To be successful, you should have at least beginner-level background in Python programming (e.g., be able to read and code trace existing code, be comfortable with conditionals, loops, variables, lists, dictionaries and arrays). You should have a basic understanding of linear algebra (vector notation) and statistics (probability distributions and mean/median/mode).
This is the second course of the Applied Machine Learning Specialization brought to you by Coursera and the Alberta Machine Intelligence Institute.
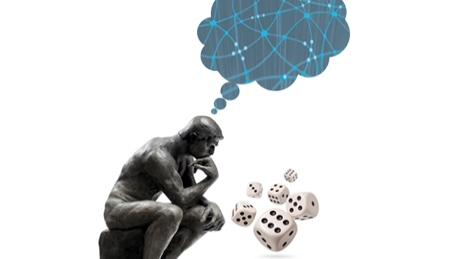
Probabilistic Graphical Models 1: Representation
Probabilistic graphical models (PGMs) are a rich framework for encoding probability distributions over complex domains: joint (multivariate) distributions over large numbers of random variables that interact with each other. These representations sit at the intersection of statistics and computer science, relying on concepts from probability theory, graph algorithms, machine learning, and more. They are the basis for the state-of-the-art methods in a wide variety of applications, such as medical diagnosis, image understanding, speech recognition, natural language processing, and many, many more. They are also a foundational tool in formulating many machine learning problems.
This course is the first in a sequence of three. It describes the two basic PGM representations: Bayesian Networks, which rely on a directed graph; and Markov networks, which use an undirected graph. The course discusses both the theoretical properties of these representations as well as their use in practice. The (highly recommended) honors track contains several hands-on assignments on how to represent some real-world problems. The course also presents some important extensions beyond the basic PGM representation, which allow more complex models to be encoded compactly.
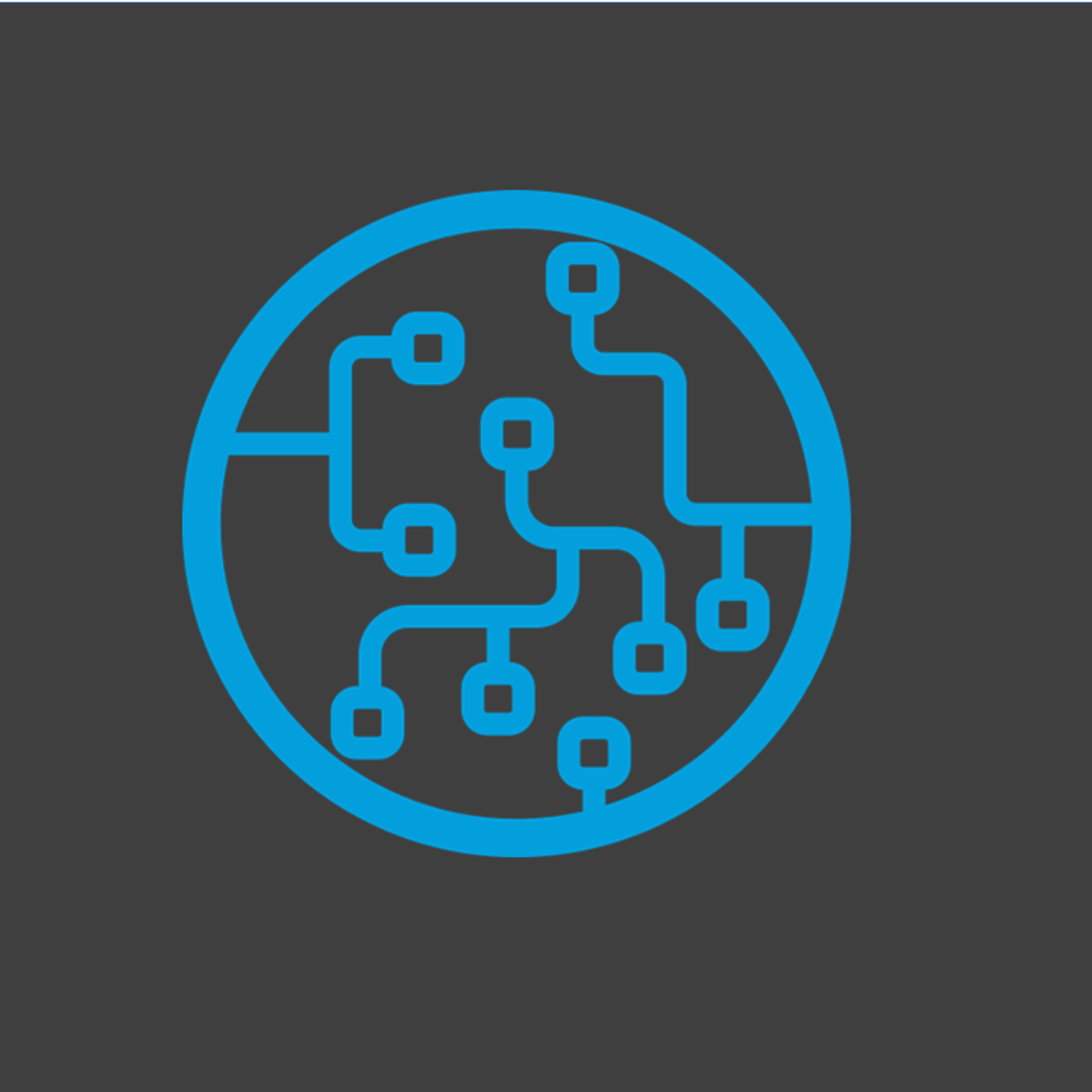
Mining Quality Prediction Using Machine & Deep Learning
In this 1.5-hour long project-based course, you will be able to:
- Understand the theory and intuition behind Simple and Multiple Linear Regression.
- Import Key python libraries, datasets and perform data visualization
- Perform exploratory data analysis and standardize the training and testing data.
- Train and Evaluate different regression models using Sci-kit Learn library.
- Build and train an Artificial Neural Network to perform regression.
- Understand the difference between various regression models KPIs such as MSE, RMSE, MAE, R2, and adjusted R2.
- Assess the performance of regression models and visualize the performance of the best model using various KPIs.
Popular Internships and Jobs by Categories
Browse
© 2024 BoostGrad | All rights reserved