Back to Courses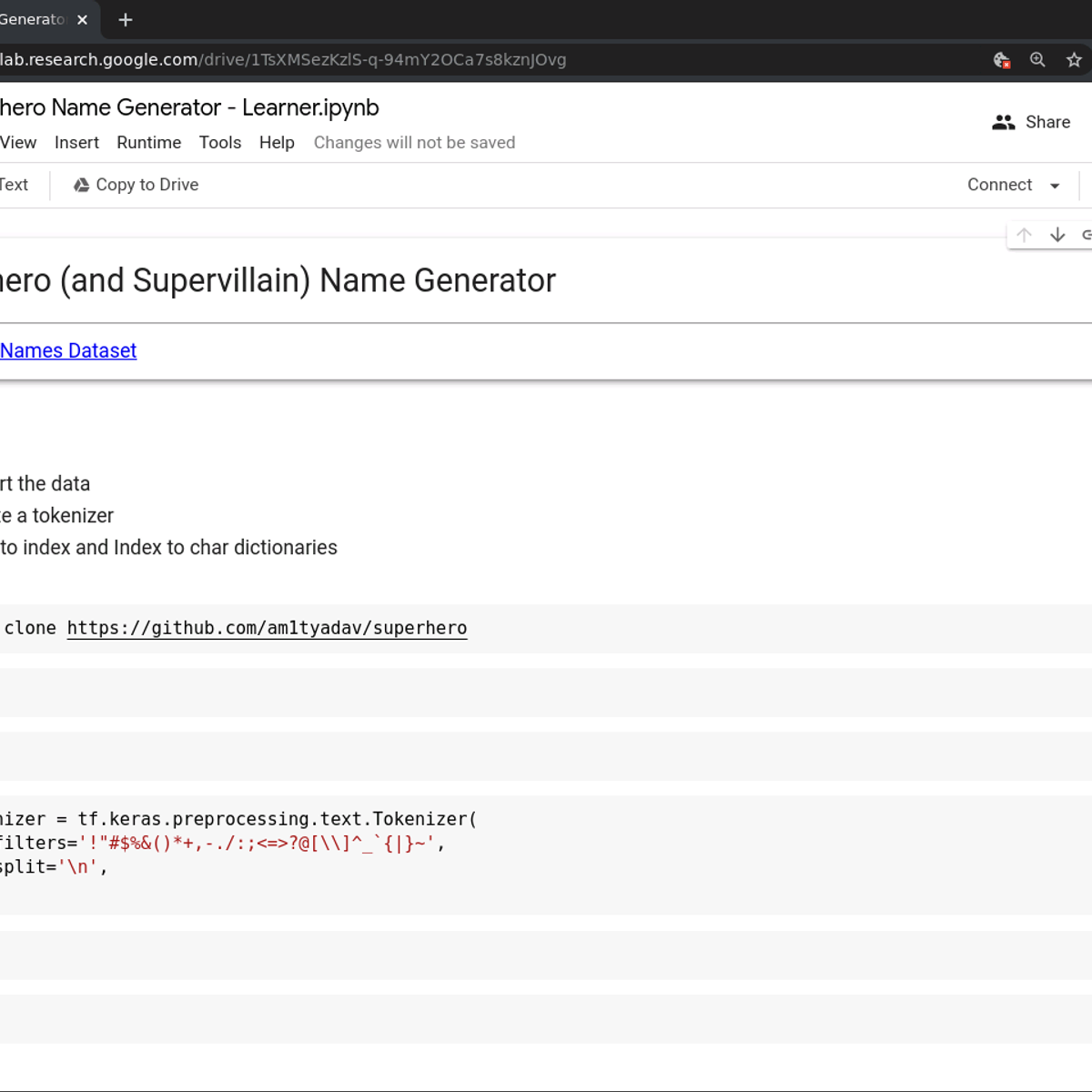
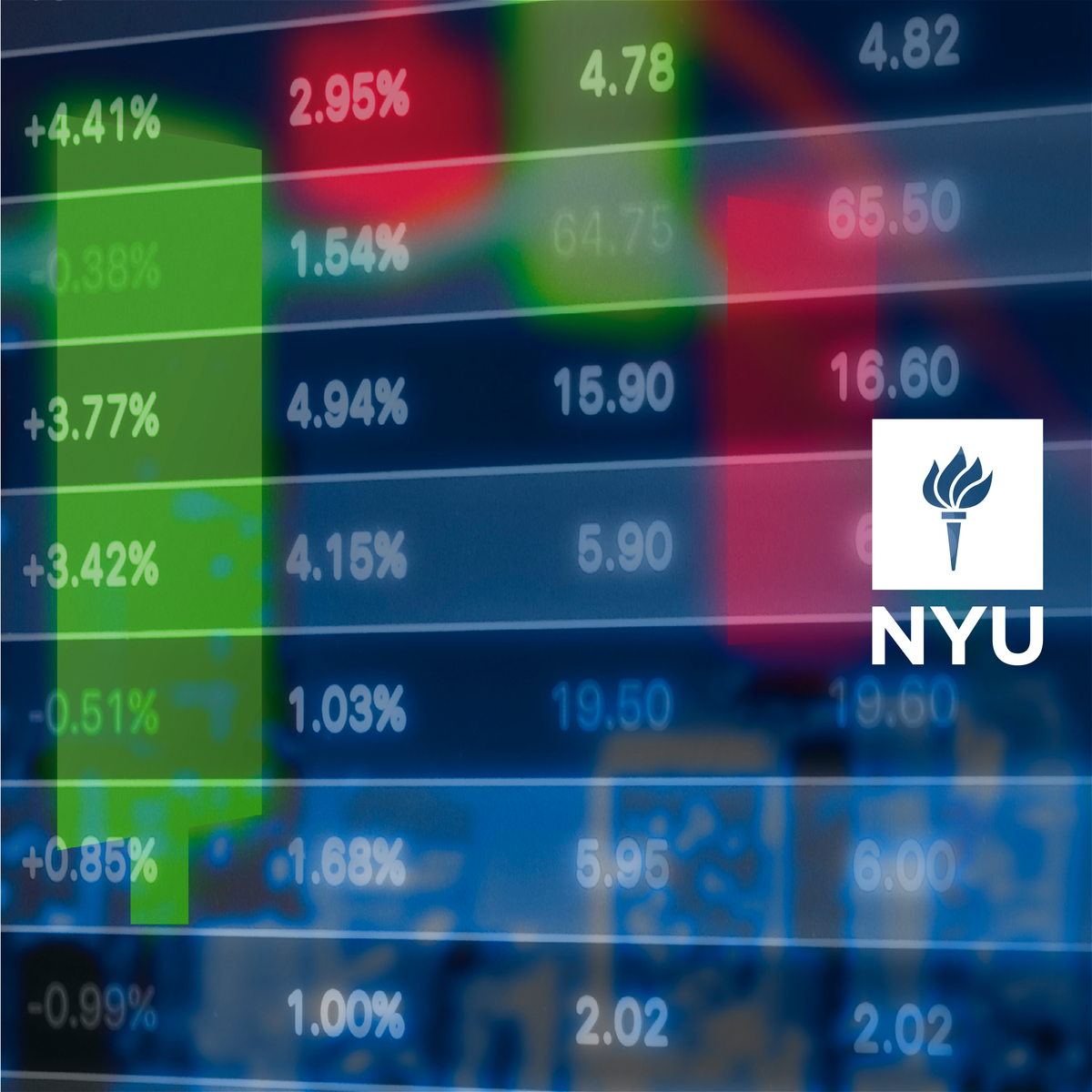
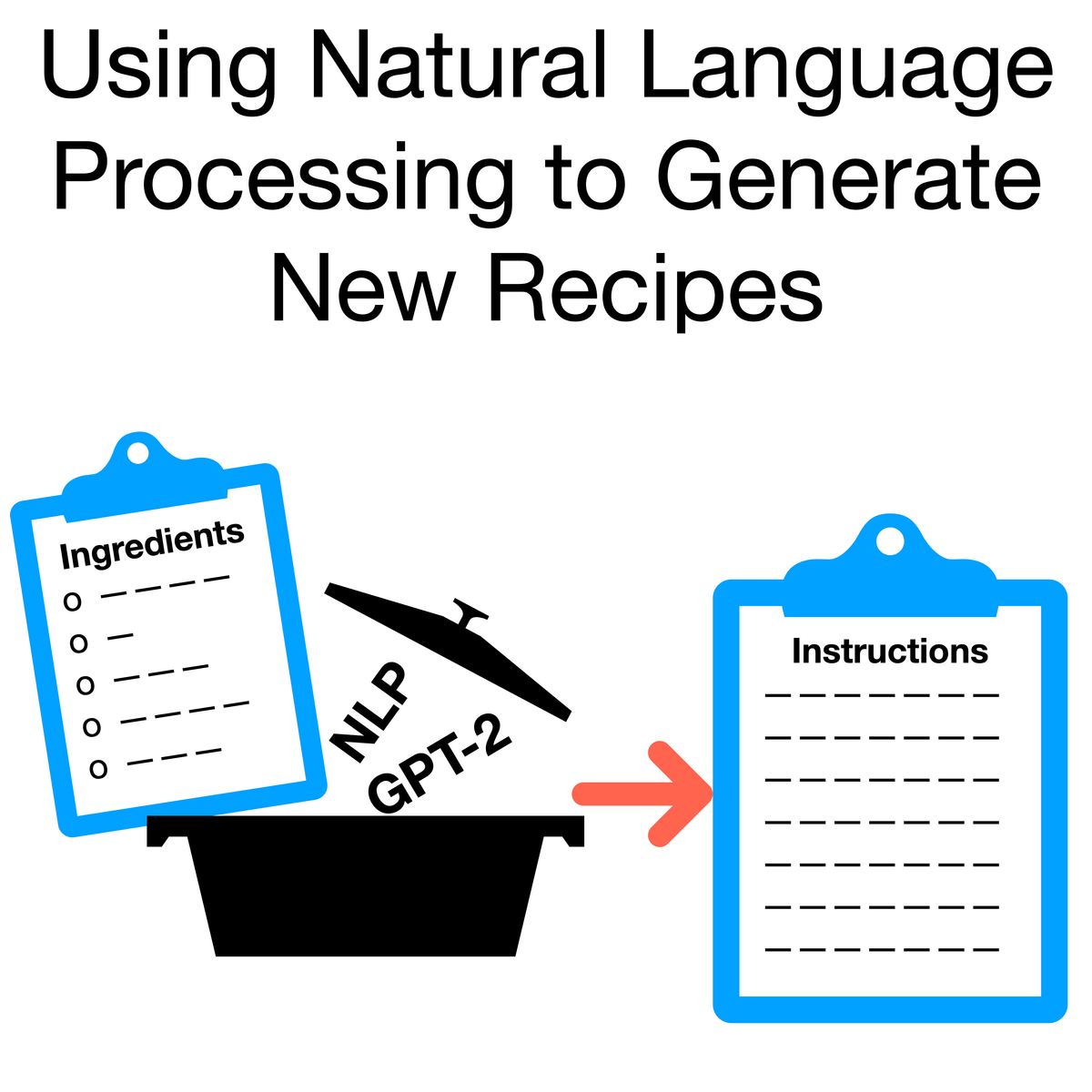
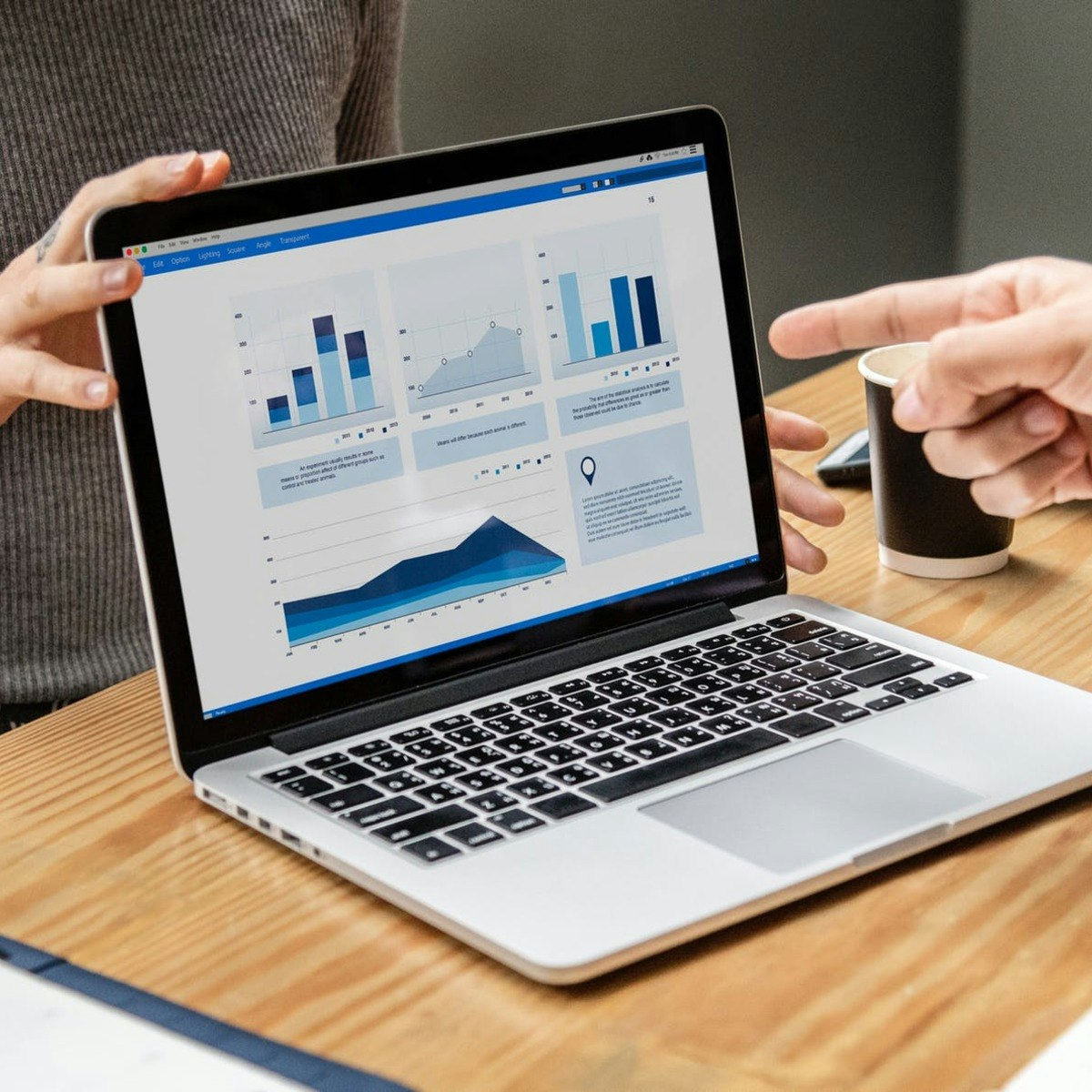
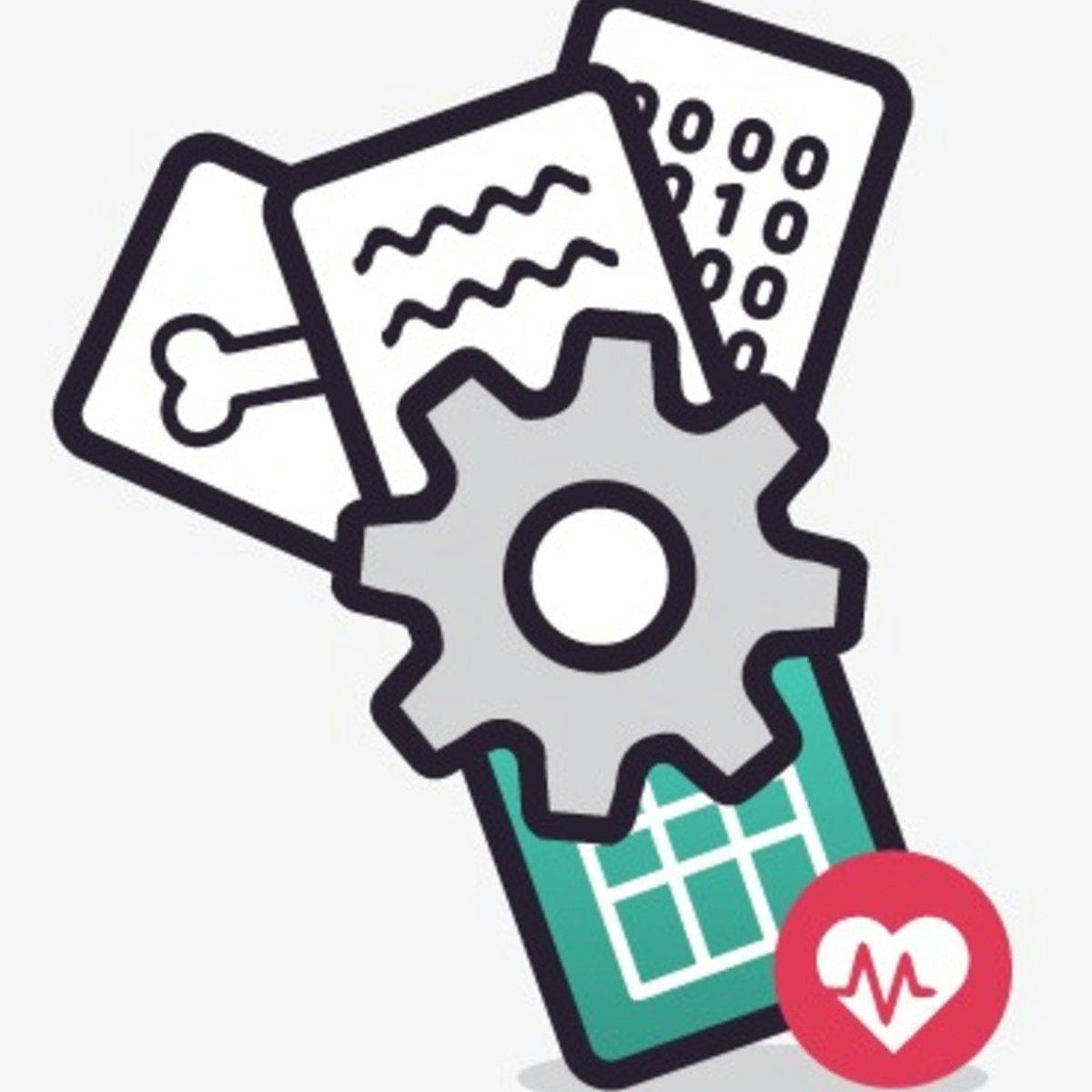
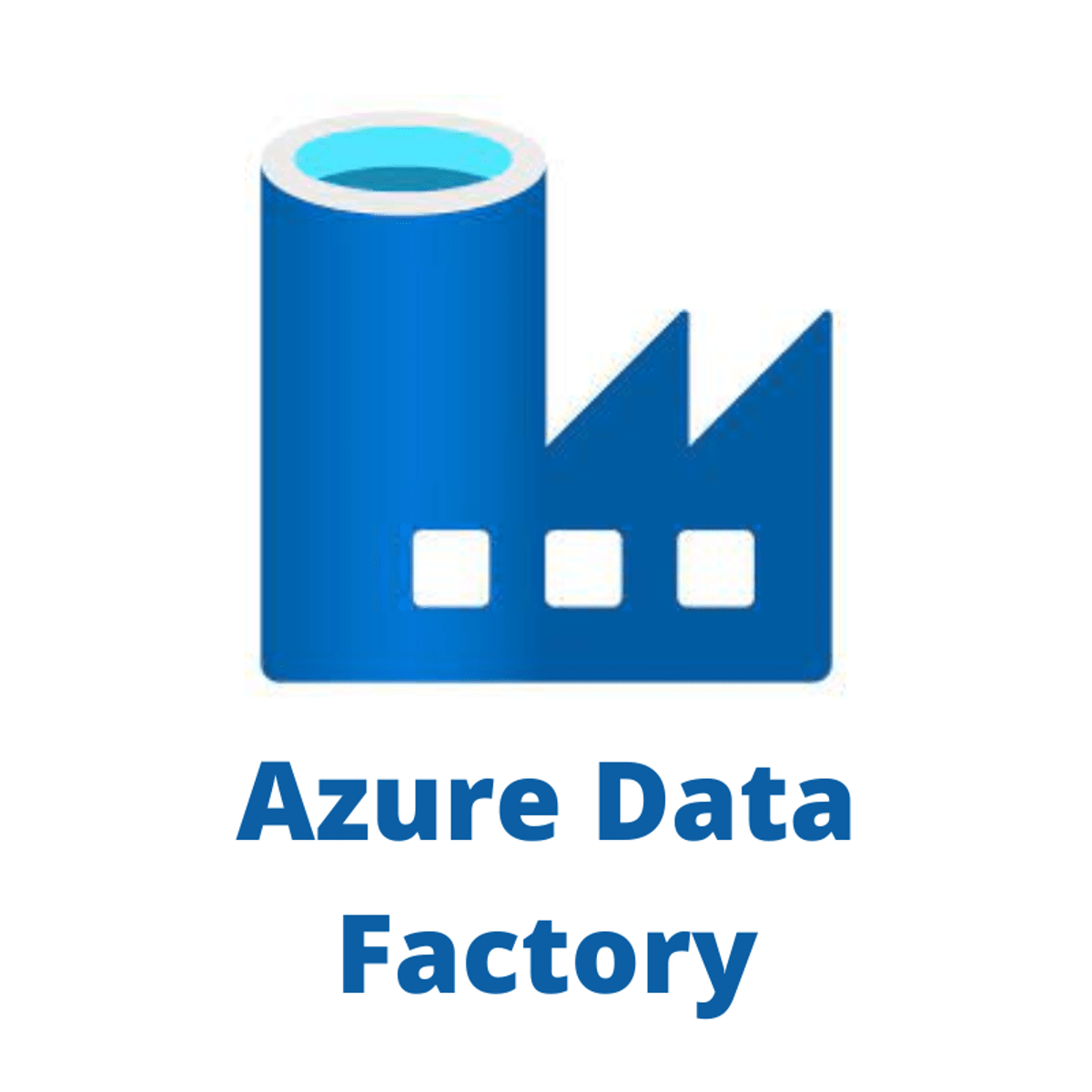
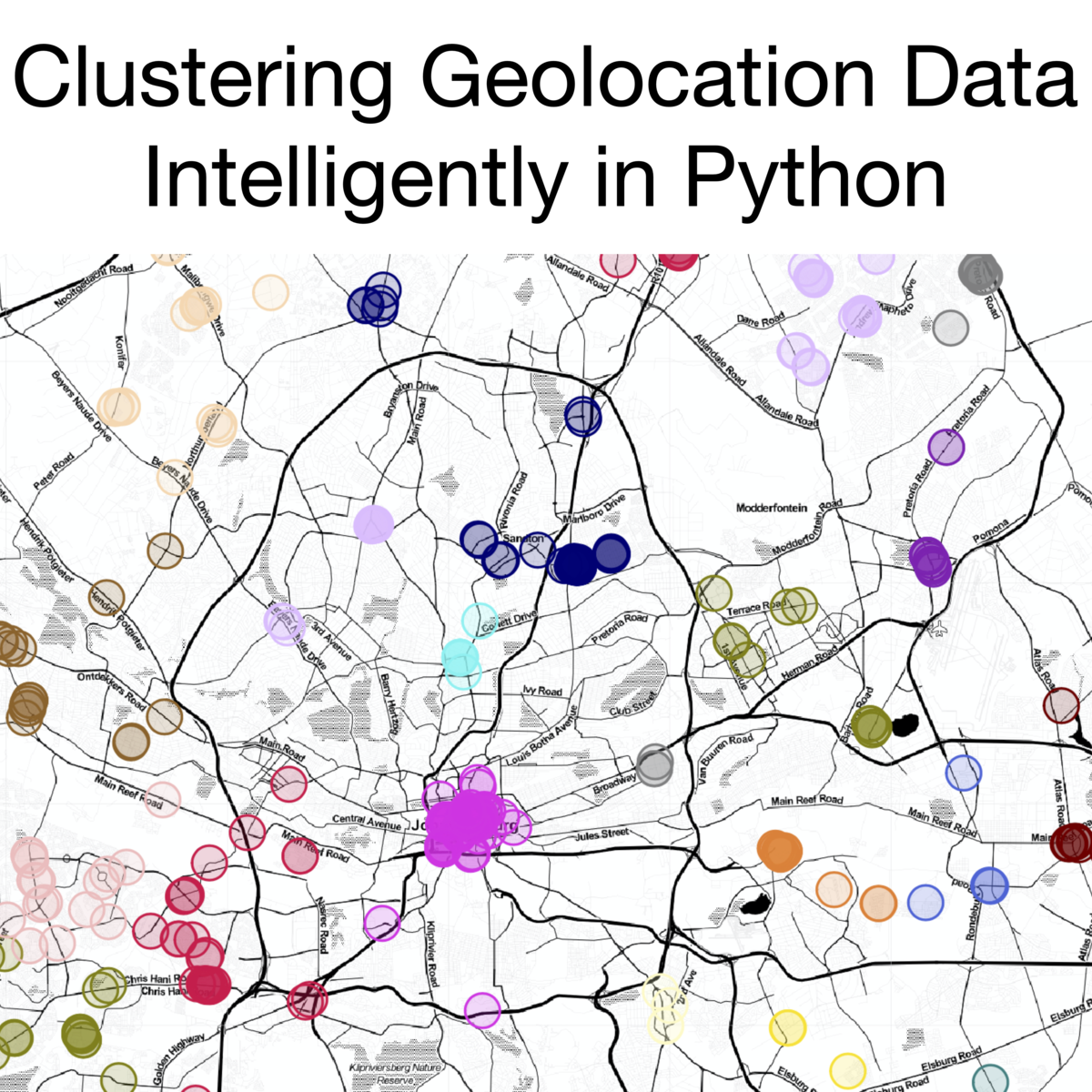
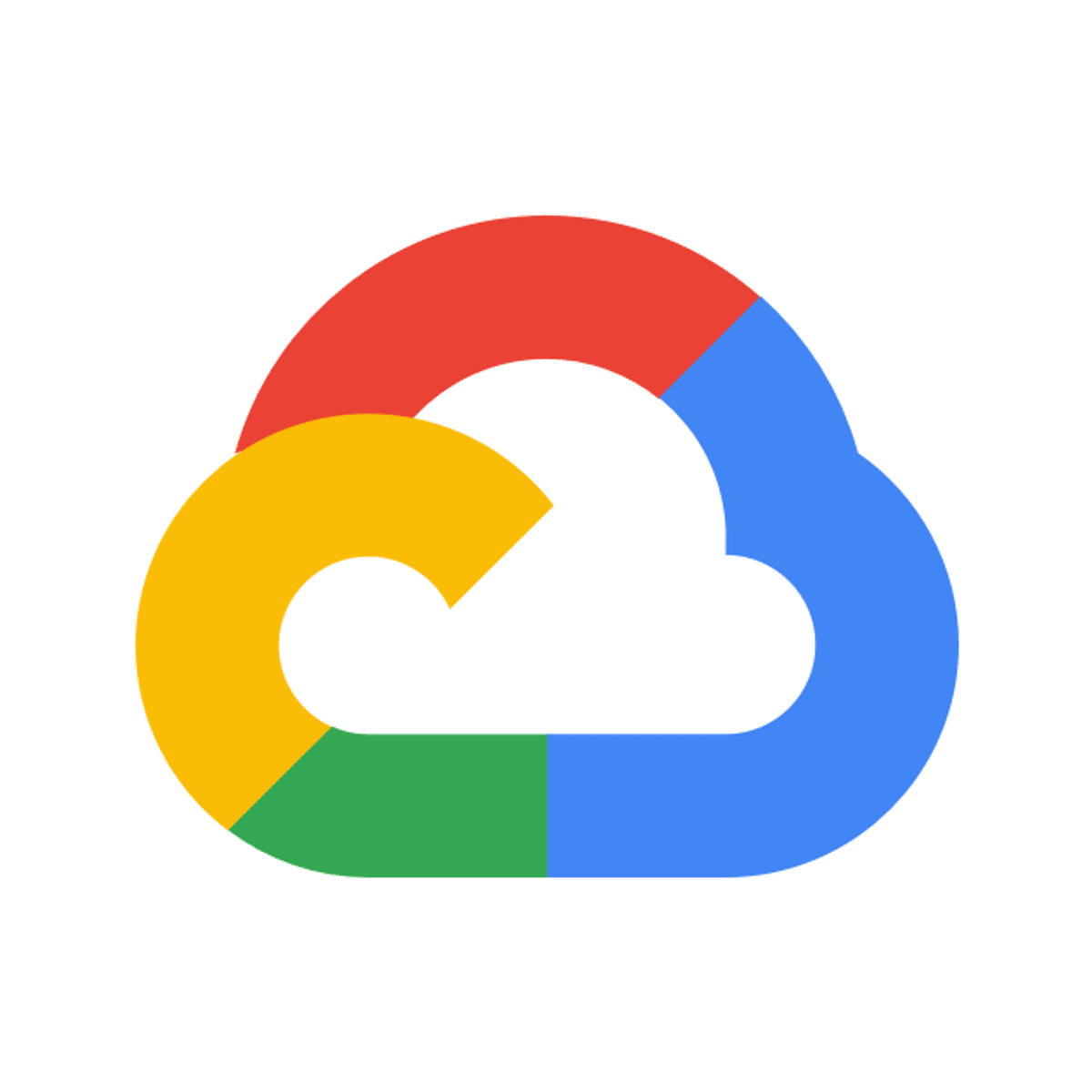
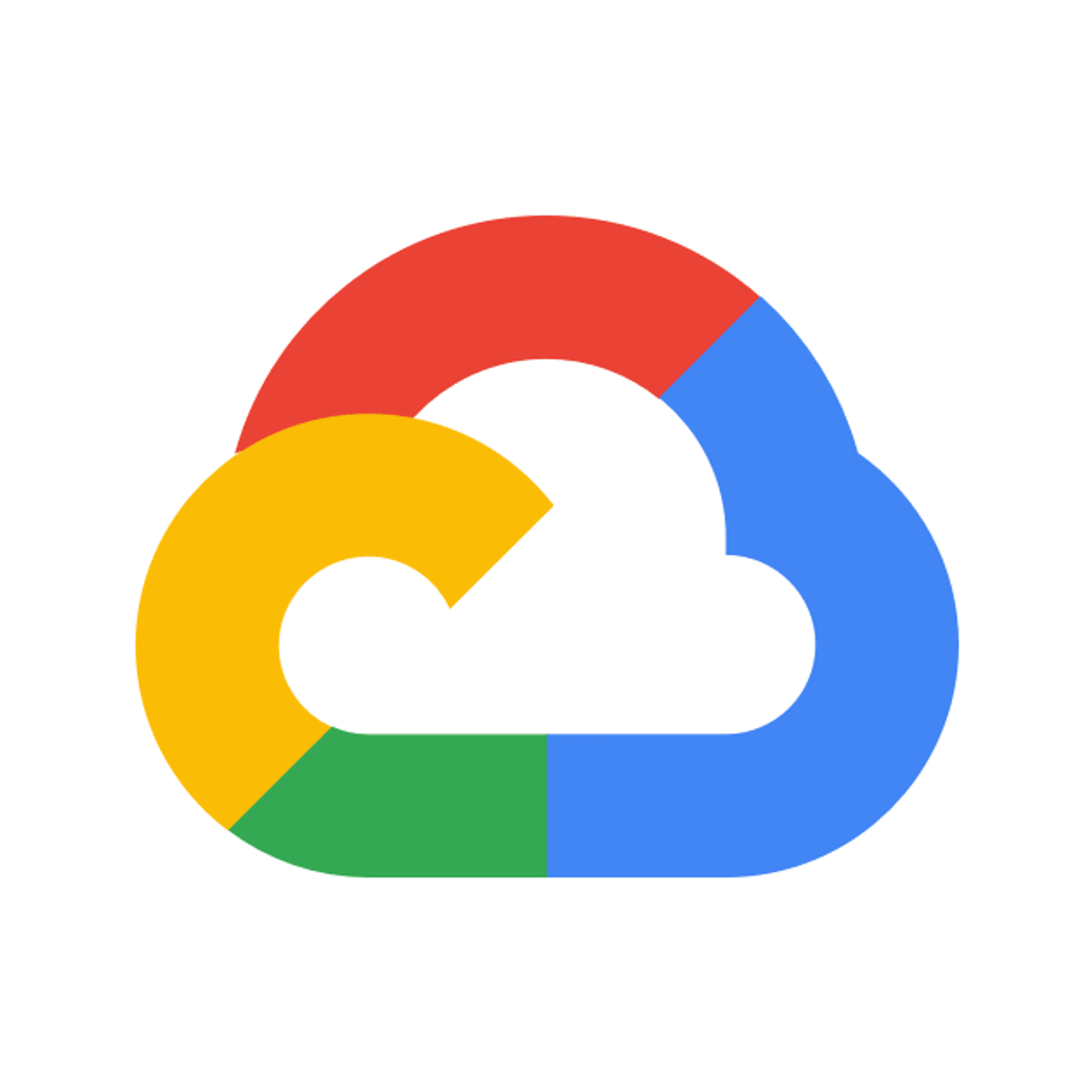
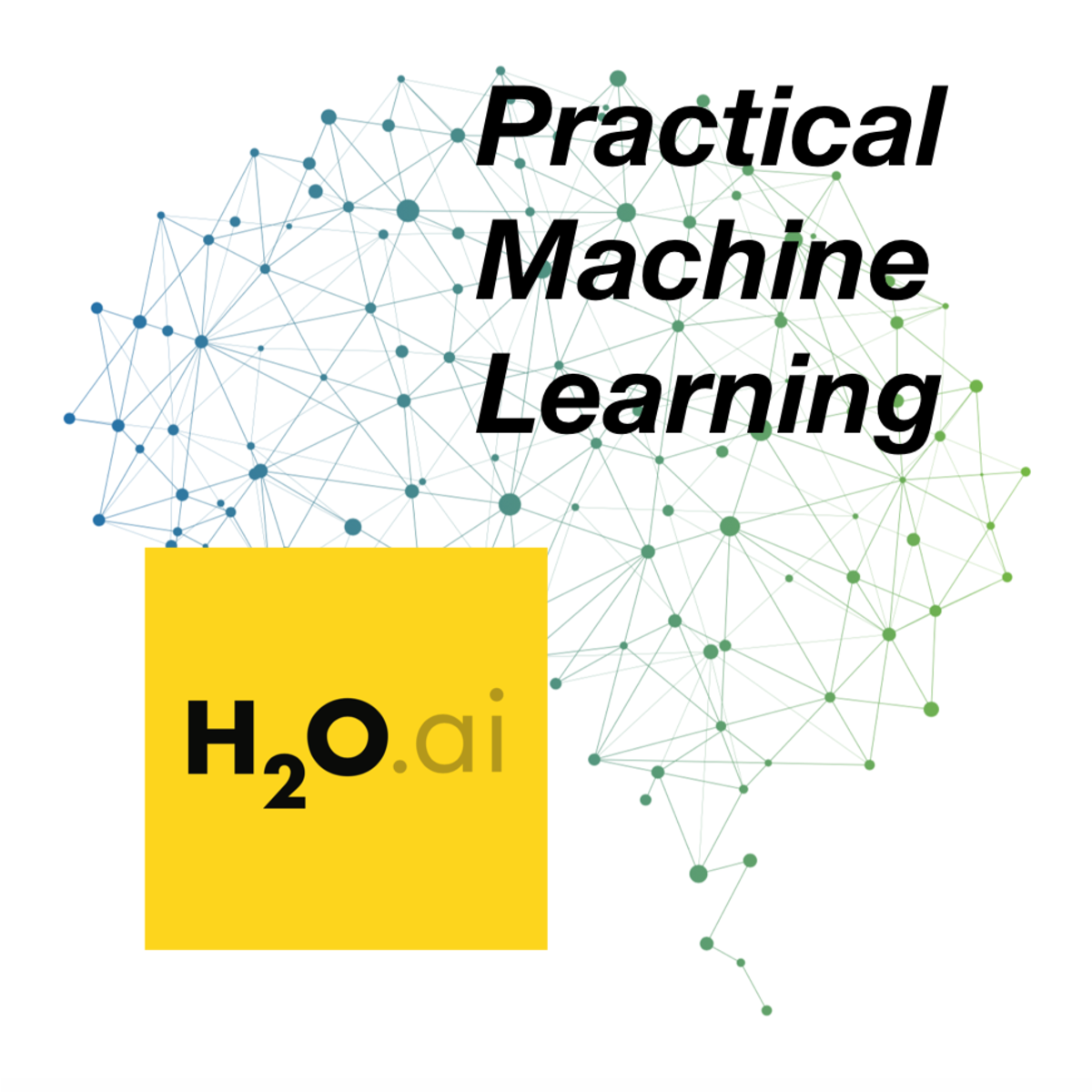
Data Science Courses - Page 97
Showing results 961-970 of 1407
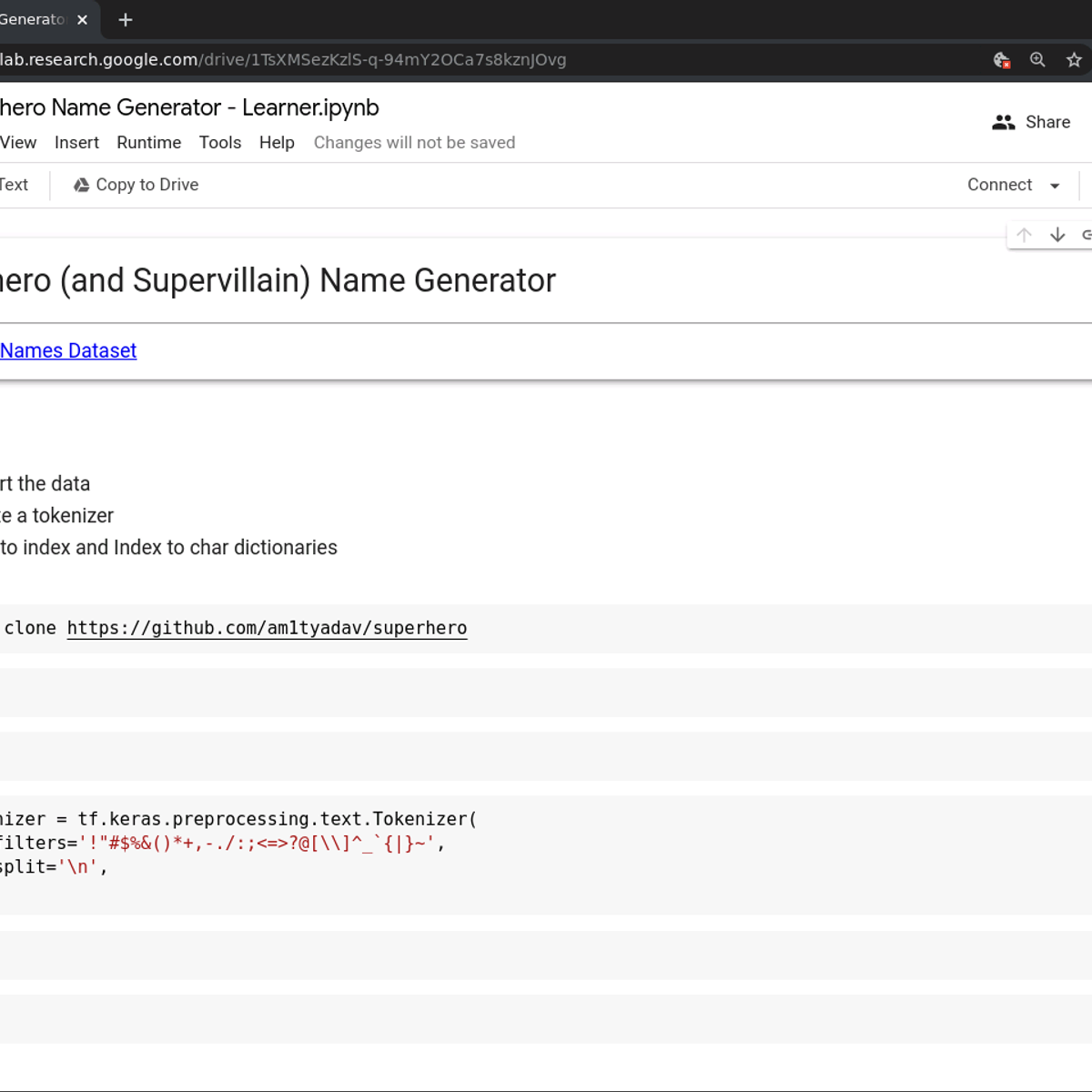
Create a Superhero Name Generator with TensorFlow
In this guided project, we are going to create a neural network and train it on a small dataset of superhero names to learn to generate similar names. The dataset has over 9000 names of superheroes, supervillains and other fictional characters from a number of different comic books, TV shows and movies.
Text generation is a common natural language processing task. We will create a character level language model that will predict the next character for a given input sequence. In order to get a new predicted superhero name, we will need to give our model a seed input - this can be a single character or a sequence of characters, and the model will then generate the next character that it predicts should after the input sequence. This character is then added to the seed input to create a new input, which is then used again to generate the next character, and so on.
You will need prior programming experience in Python. Some experience with TensorFlow is recommended. This is a practical, hands on guided project for learners who already have theoretical understanding of Neural Networks, Recurrent Neural Networks, and optimization algorithms like gradient descent but want to understand how to use the TensorFlow to start performing natural language processing tasks like text classification or text generation.
Note: This course works best for learners who are based in the North America region. We’re currently working on providing the same experience in other regions.
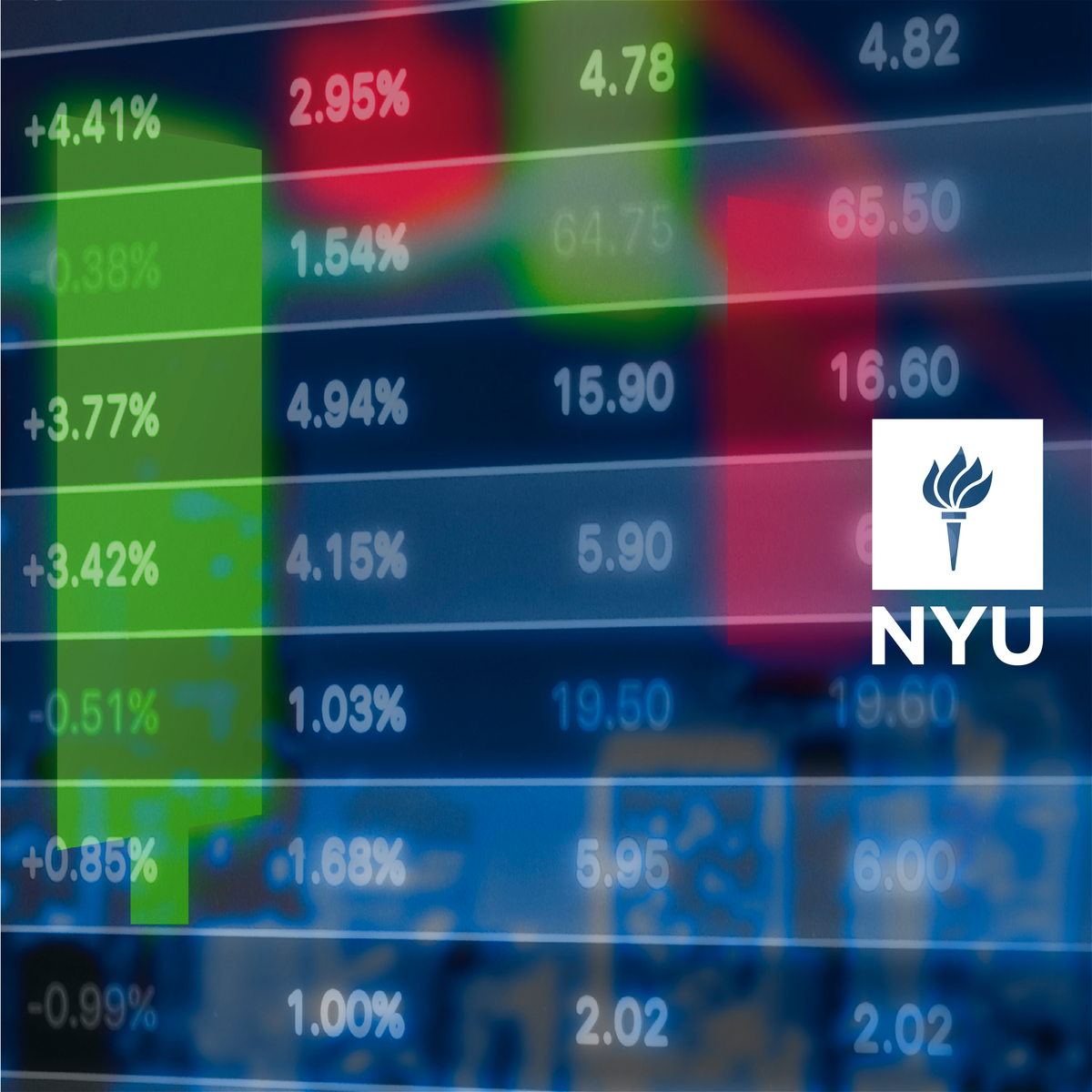
Fundamentals of Machine Learning in Finance
The course aims at helping students to be able to solve practical ML-amenable problems that they may encounter in real life that include: (1) understanding where the problem one faces lands on a general landscape of available ML methods, (2) understanding which particular ML approach(es) would be most appropriate for resolving the problem, and (3) ability to successfully implement a solution, and assess its performance.
A learner with some or no previous knowledge of Machine Learning (ML) will get to know main algorithms of Supervised and Unsupervised Learning, and Reinforcement Learning, and will be able to use ML open source Python packages to design, test, and implement ML algorithms in Finance.
Fundamentals of Machine Learning in Finance will provide more at-depth view of supervised, unsupervised, and reinforcement learning, and end up in a project on using unsupervised learning for implementing a simple portfolio trading strategy.
The course is designed for three categories of students:
Practitioners working at financial institutions such as banks, asset management firms or hedge funds
Individuals interested in applications of ML for personal day trading
Current full-time students pursuing a degree in Finance, Statistics, Computer Science, Mathematics, Physics, Engineering or other related disciplines who want to learn about practical applications of ML in Finance
Experience with Python (including numpy, pandas, and IPython/Jupyter notebooks), linear algebra, basic probability theory and basic calculus is necessary to complete assignments in this course.
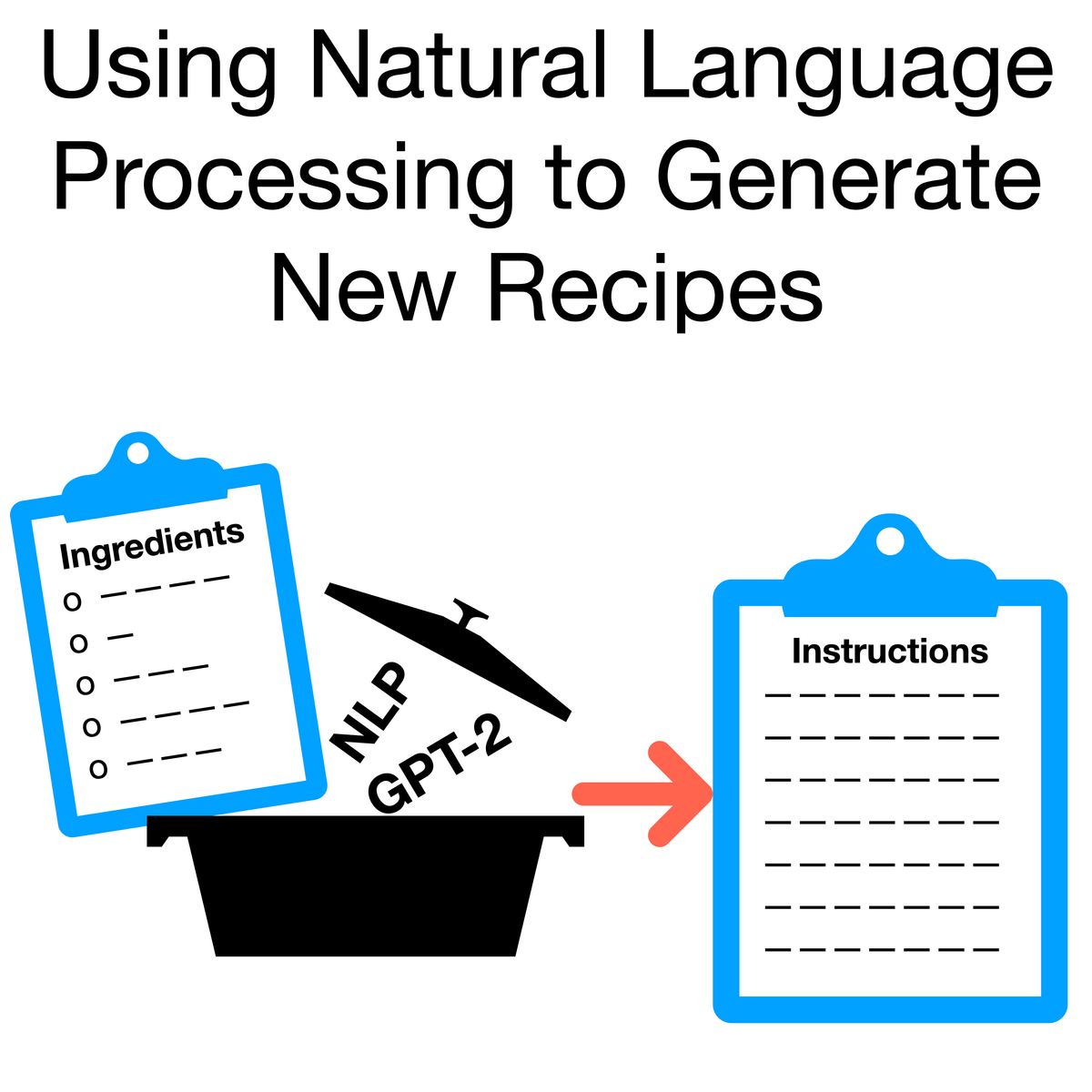
Generating New Recipes using GPT-2
In this 2 hour long project, you will learn how to preprocess a text dataset comprising recipes, and split it into a training and validation set. You will learn how to use the HuggingFace library to fine-tune a deep, generative model, and specifically how to train such a model on Google Colab. Finally, you will learn how to use GPT-2 effectively to create realistic and unique recipes from lists of ingredients based on the aforementioned dataset. This project aims to teach you how to fine-tune a large-scale model, and the sheer magnitude of resources it takes for these models to learn. You will also learn about knowledge distillation and its efficacy in use cases such as this one.
Note: This course works best for learners who are based in the North America region. We’re currently working on providing the same experience in other regions.
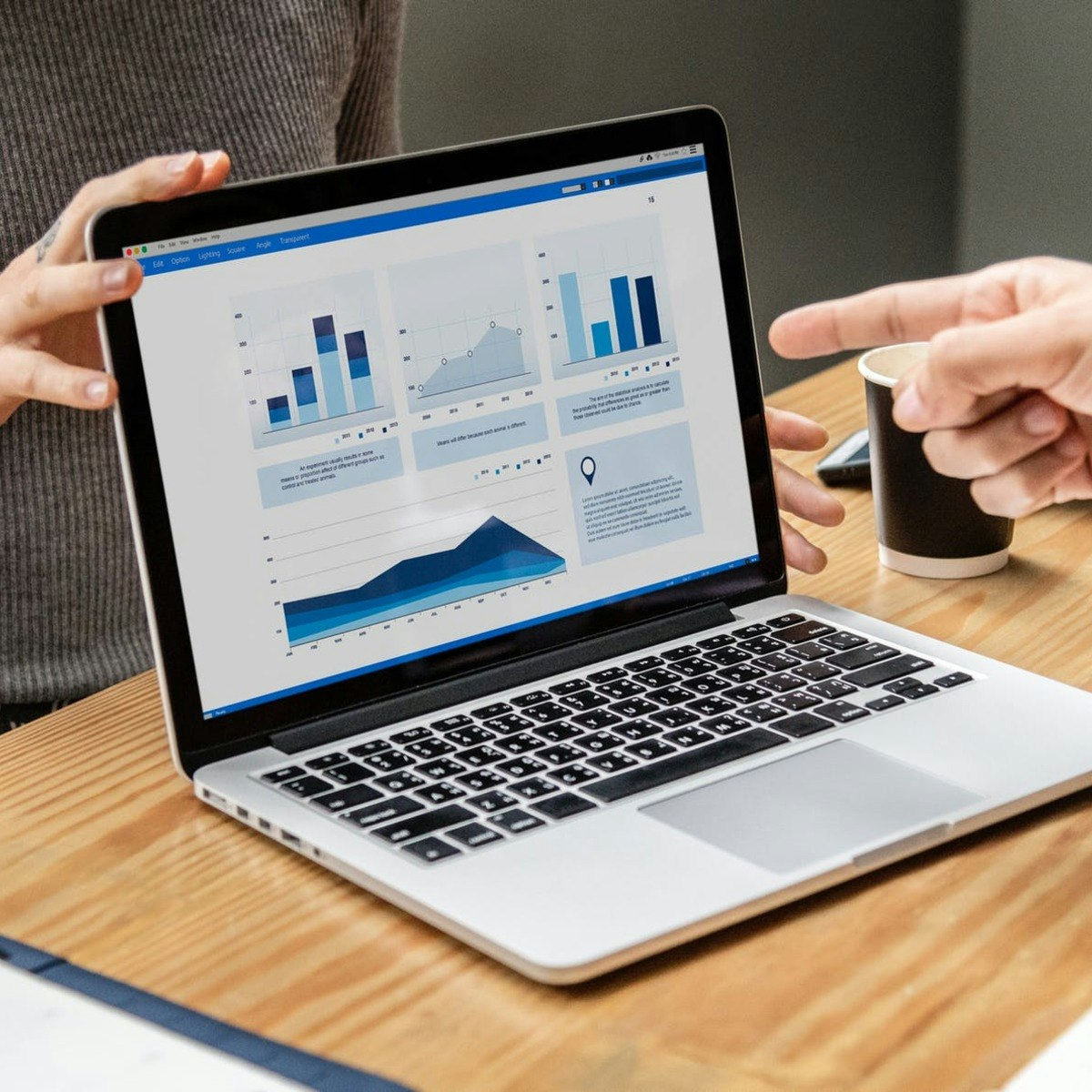
Basic Data Processing and Visualization
This is the first course in the four-course specialization Python Data Products for Predictive Analytics, introducing the basics of reading and manipulating datasets in Python. In this course, you will learn what a data product is and go through several Python libraries to perform data retrieval, processing, and visualization.
This course will introduce you to the field of data science and prepare you for the next three courses in the Specialization: Design Thinking and Predictive Analytics for Data Products, Meaningful Predictive Modeling, and Deploying Machine Learning Models. At each step in the specialization, you will gain hands-on experience in data manipulation and building your skills, eventually culminating in a capstone project encompassing all the concepts taught in the specialization.
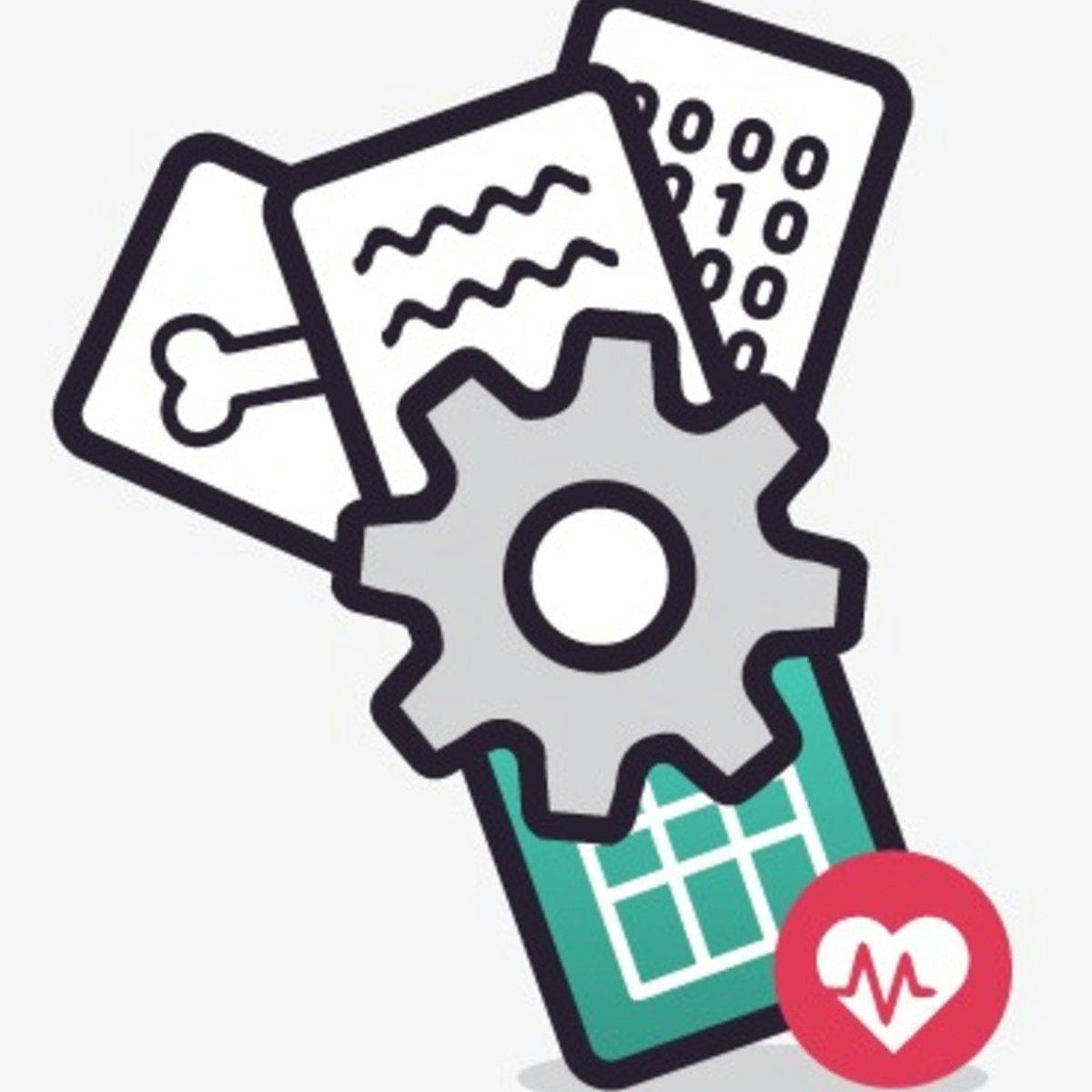
Foundations of mining non-structured medical data
The goal of this course is to understand the foundations of Big Data and the data that is being generated in the health domain and how the use of technology would help to integrate and exploit all those data to extract meaningful information that can be later used in different sectors of the health domain from physicians to management, from patients to caregivers, etc.
The course offers a high-level perspective of the importance of the medical context within the European context, the types of data that are managed in the health (clinical) context, the challenges to be addressed in the mining of unstructured medical data (text and image) as well as the opportunities from the analytical point of view with an introduction to the basics of data analytics field.
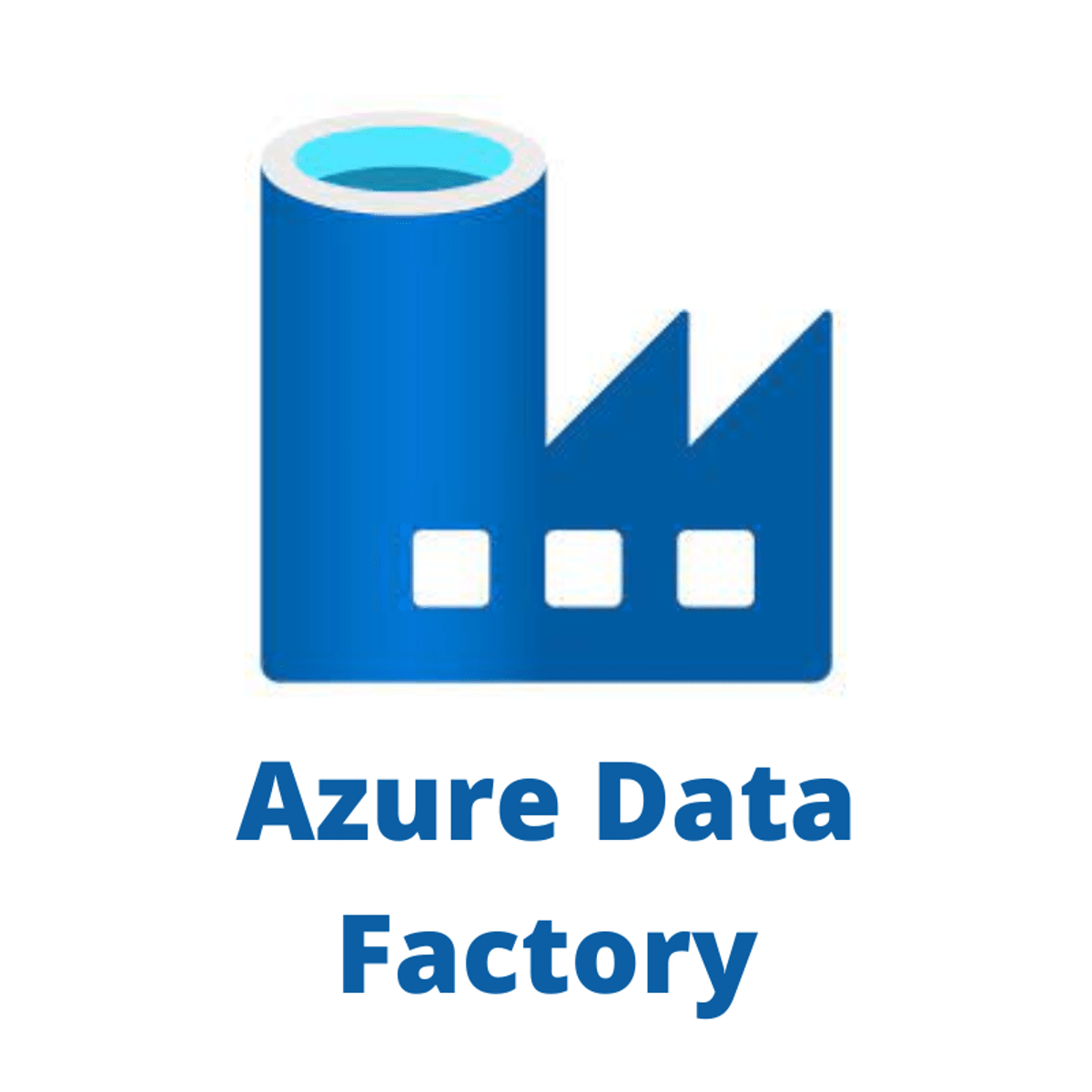
Create Mapping Data Flows in Azure Data Factory
In this 1 hour long project-based course, we’ll learn to create a mapping data flow on the azure data factory. First, we’ll learn to create an azure data factory on the Azure portal. Then we’ll learn to create an azure storage account so that we could store the source data on the blob containers. We’ll learn to configure the source and the sink transformation. We’ll learn to work with basic data flow transformations such as select, filters, sort, joins , derived columns, and conditional split transformations. We’ll learn to create a simple mapping data flow in the azure data factory. We’ll also learn to create and combine multiple streams of data on mapping data flows. Finally, we’ll also learn to store the transformed data to the destination.
You must have an Azure account prior.
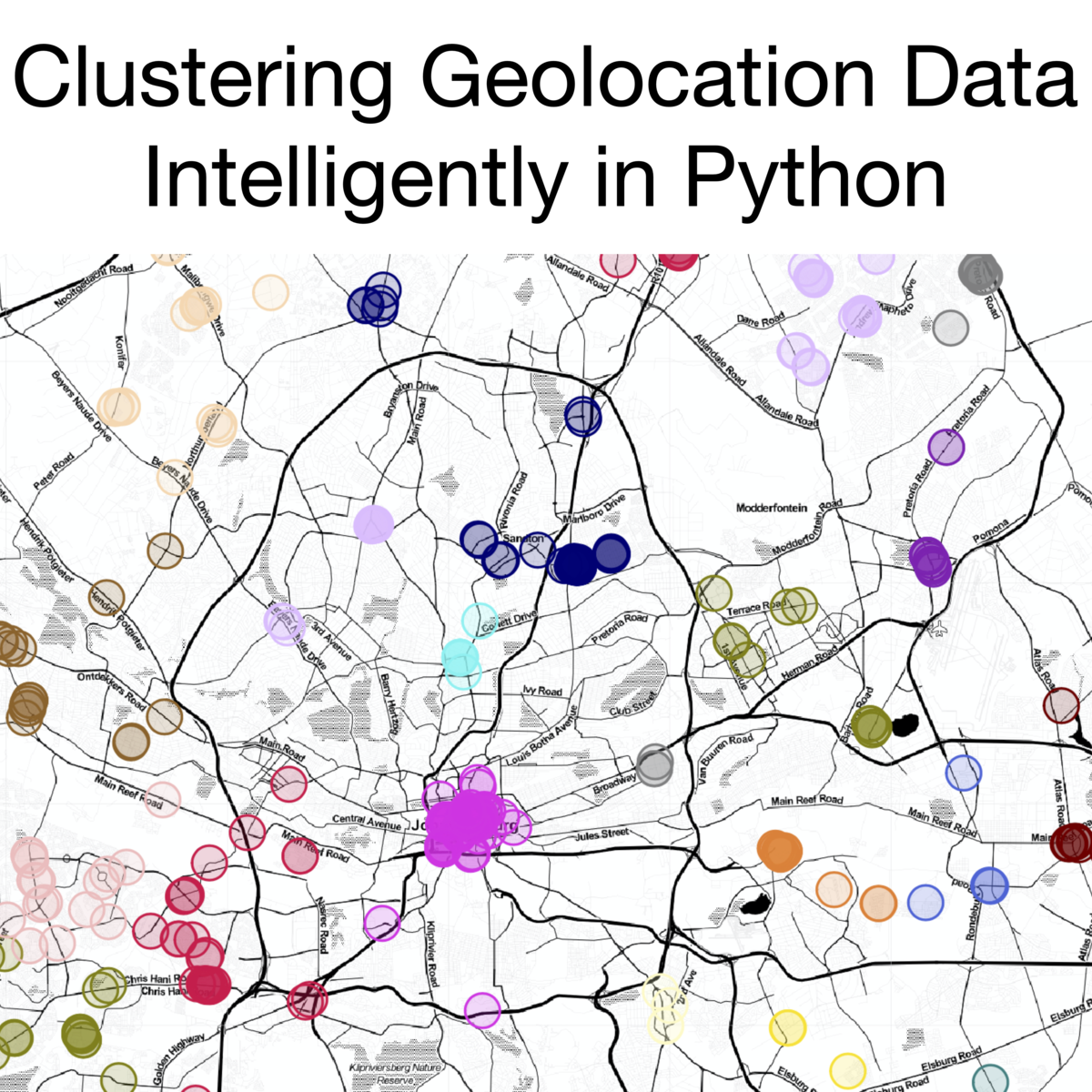
Clustering Geolocation Data Intelligently in Python
In this 1.5-hour long project, you will learn how to clean and preprocess geolocation data for clustering. You will learn how to export this data into an interactive file that can be better understood for the data. You will learn how to cluster initially with a K-Means approach, before using a more complicated density-based algorithm, DBSCAN. We will discuss how to evaluate these models, and offer improvements to DBSCAN with the introduction of HDBSCAN.
Note: This course works best for learners who are based in the North America region. We’re currently working on providing the same experience in other regions.
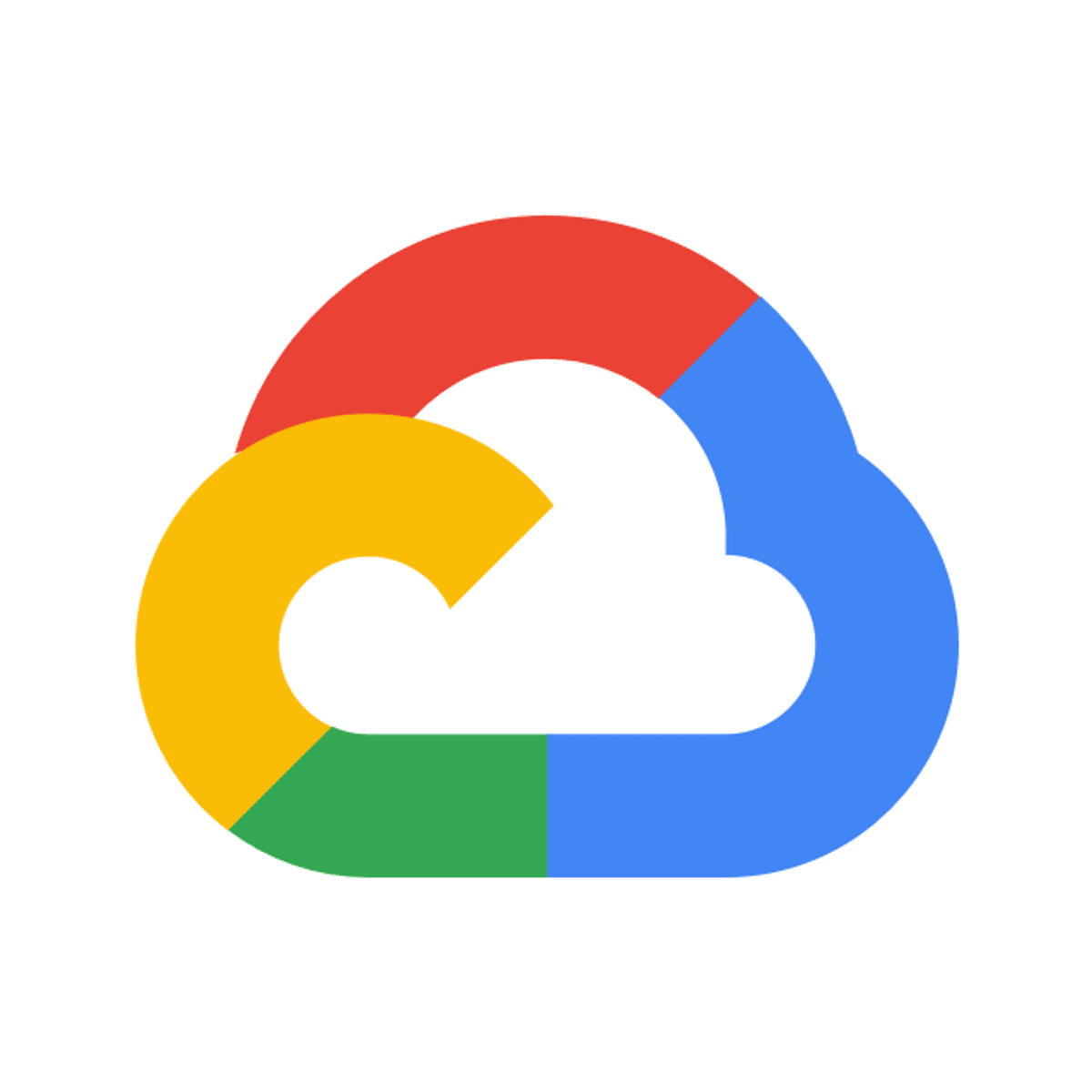
Answering Complex Questions Using Native Derived Tables with LookML
This is a Google Cloud Self-Paced Lab. In this lab you will use native derived tables to answer complex questions with LookML.
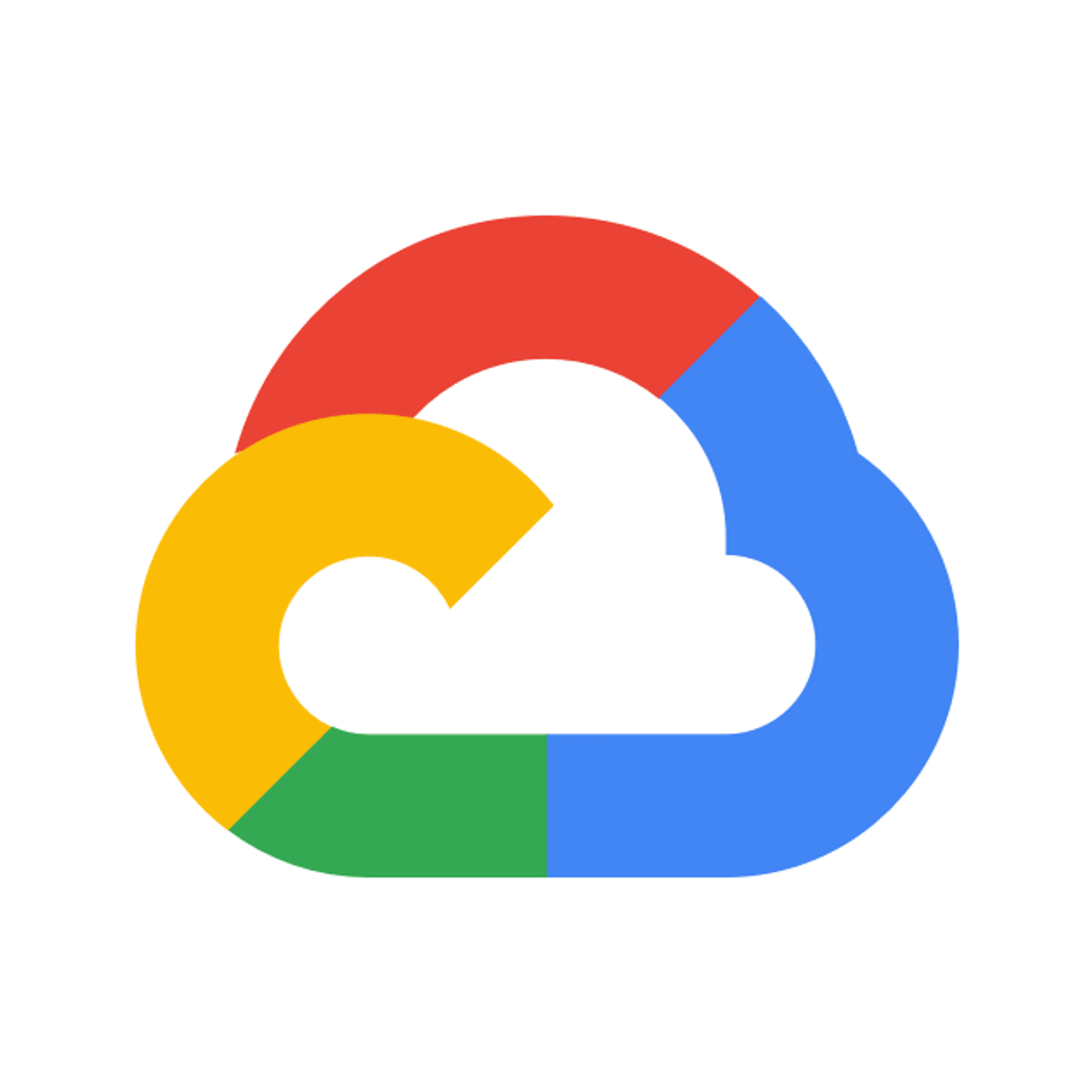
APIs Explorer: Cloud Storage
This is a self-paced lab that takes place in the Google Cloud console. In this lab, you will use the APIs Explorer tool to create Cloud Storage buckets, upload data to the bucket, and remove content from buckets.
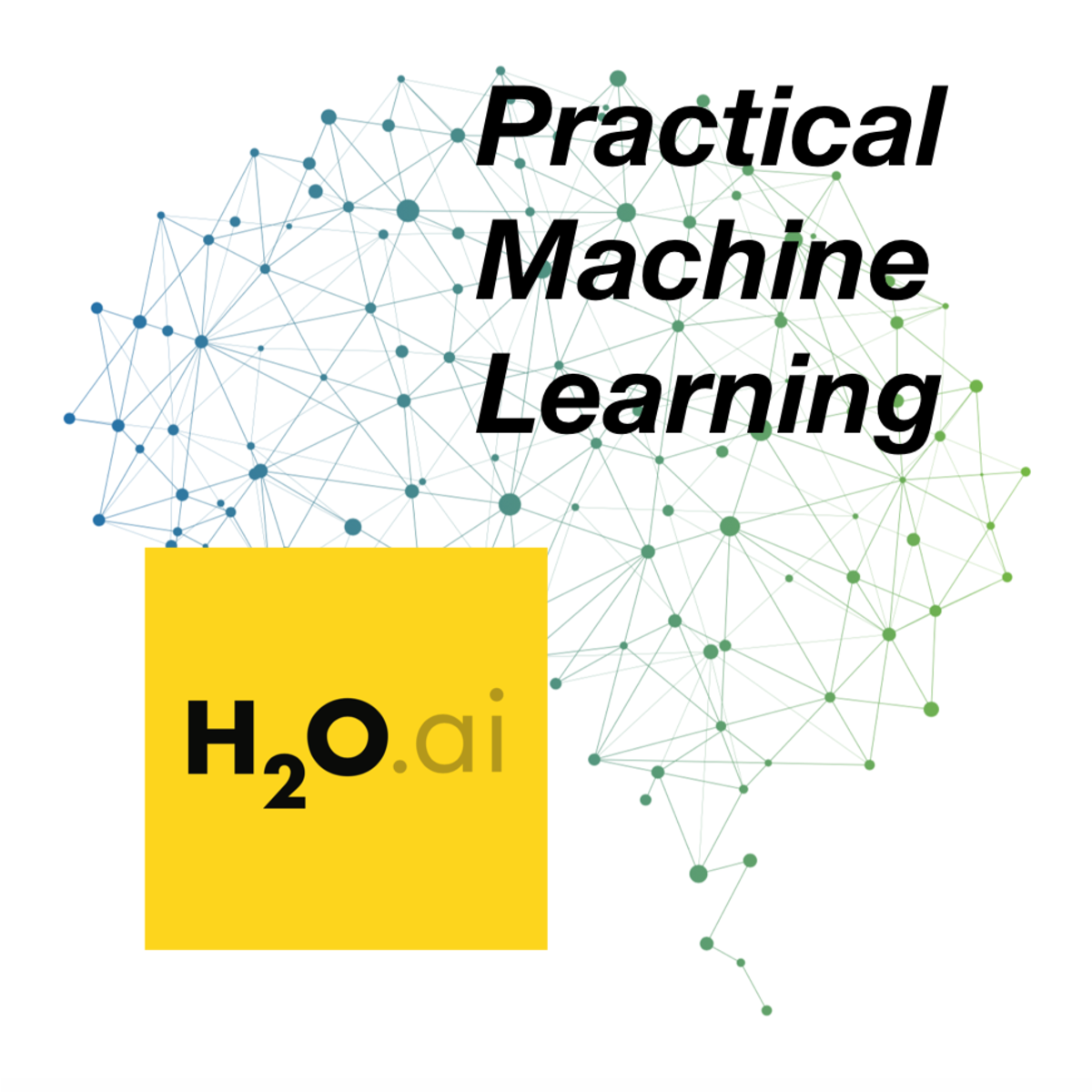
Practical Machine Learning on H2O
In this course, we will learn all the core techniques needed to make effective use of H2O. Even if you have no prior experience of machine learning, even if your math is weak, by the end of this course you will be able to make machine learning models using a variety of algorithms. We will be using linear models, random forest, GBMs and of course deep learning, as well as some unsupervised learning algorithms. You will also be able to evaluate your models and choose the best model to suit not just your data but the other business restraints you may be under.
Popular Internships and Jobs by Categories
Find Jobs & Internships
Browse
© 2024 BoostGrad | All rights reserved