Back to Courses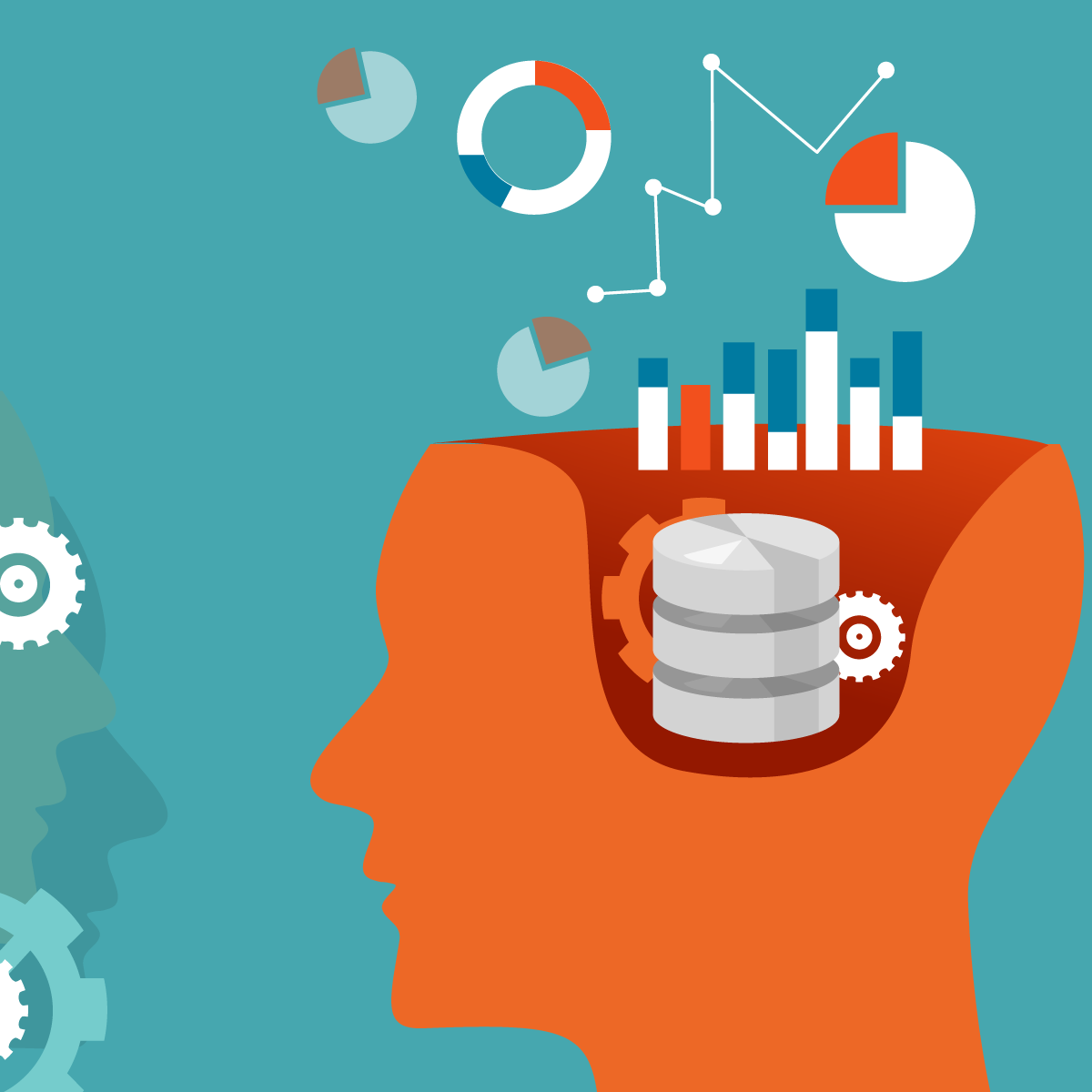
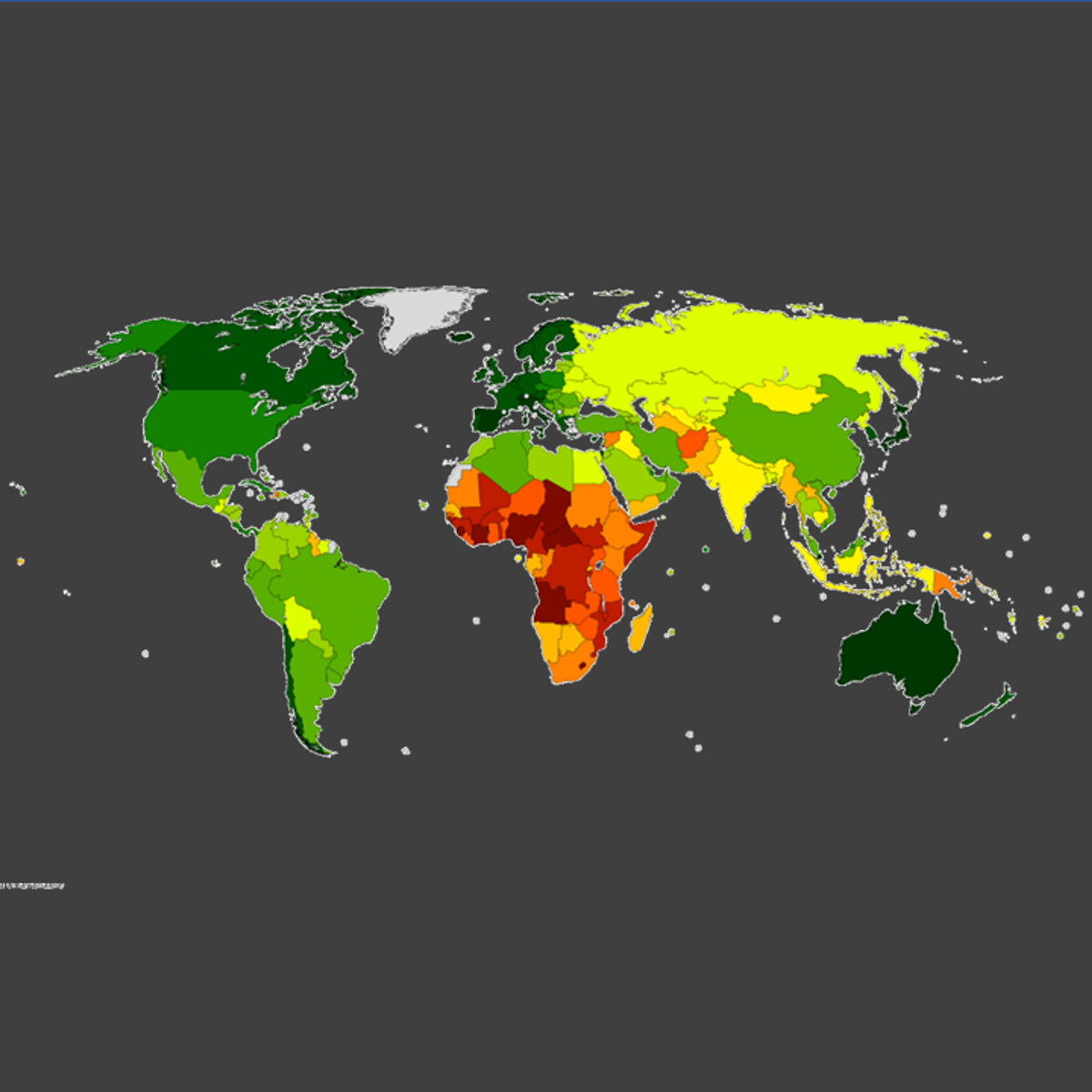
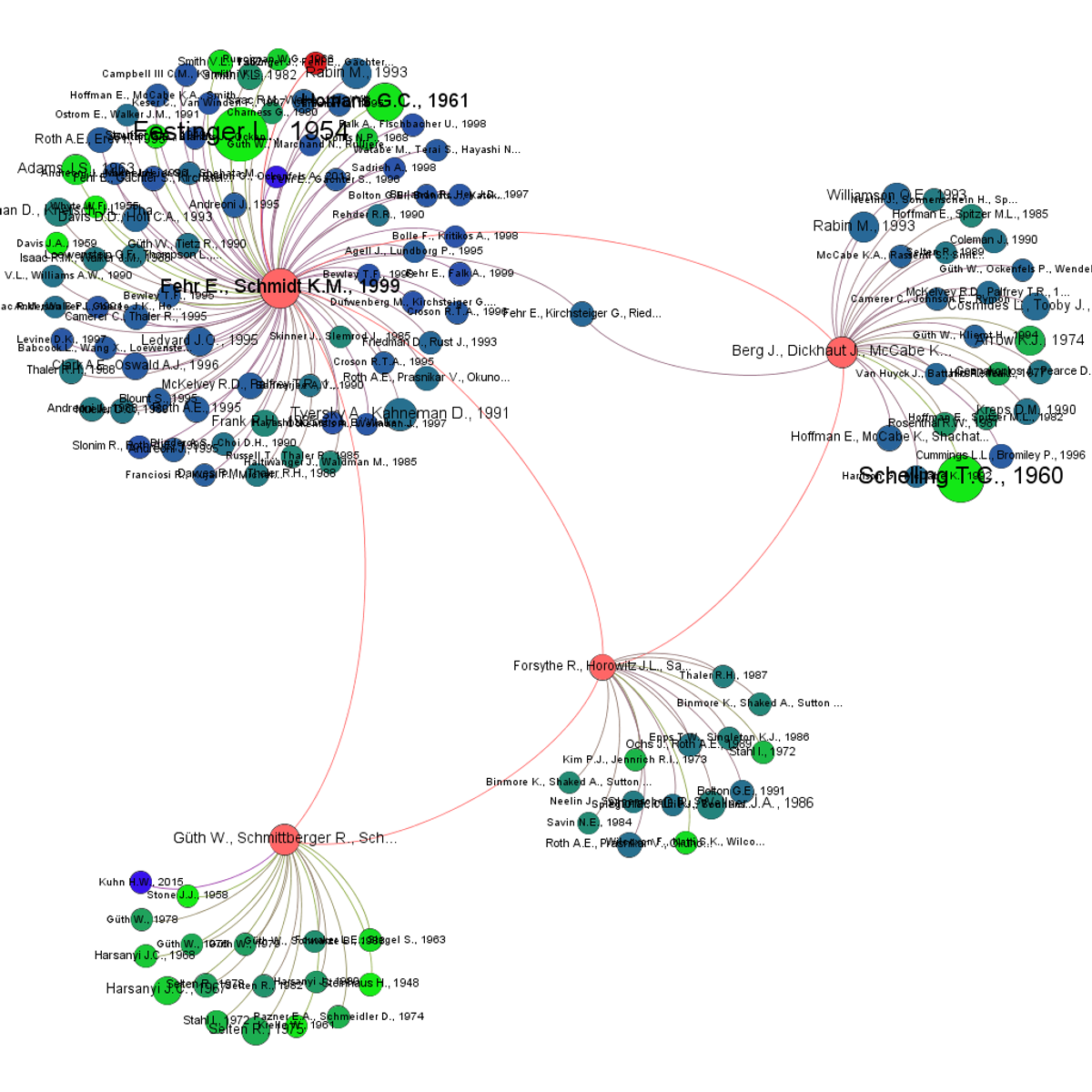
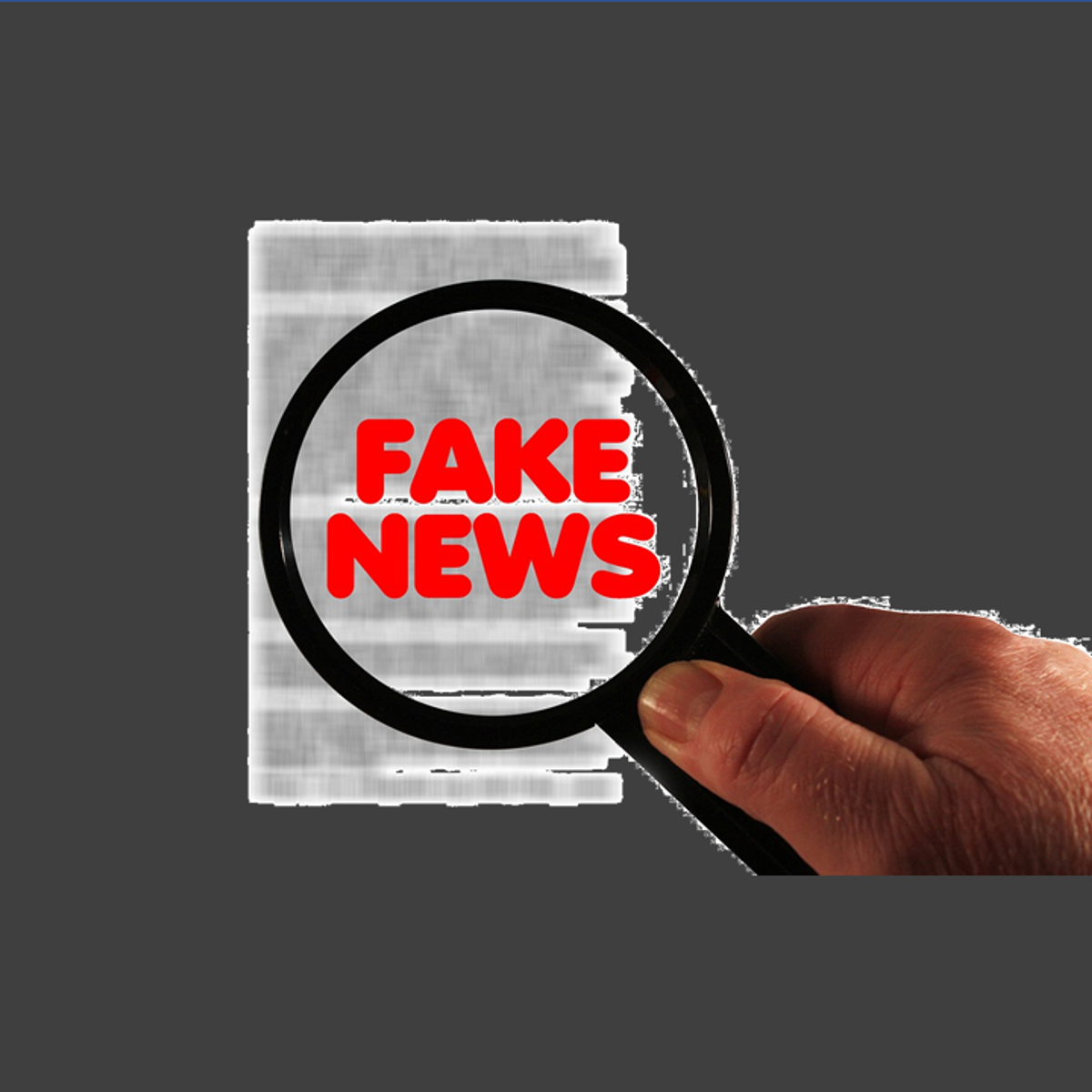
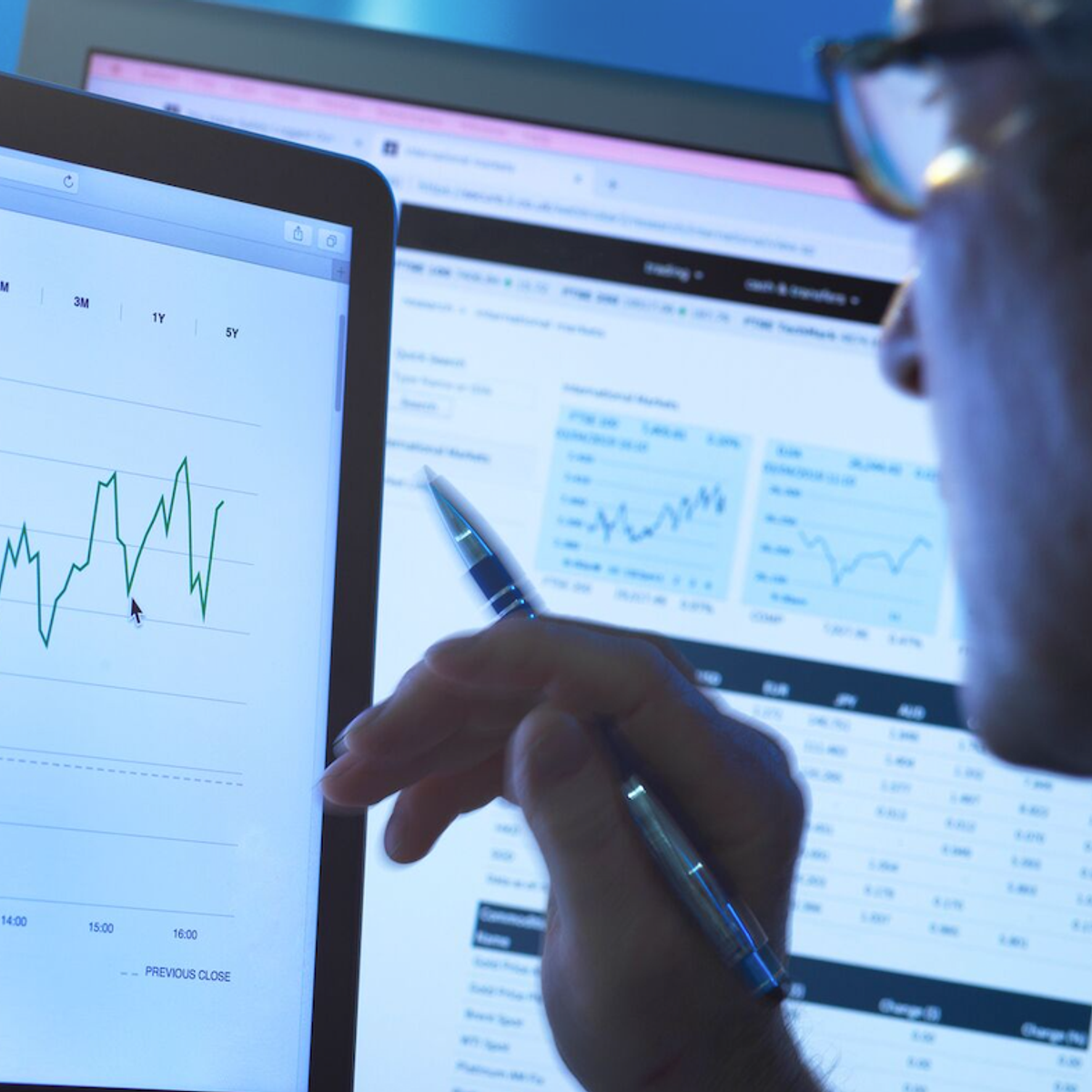
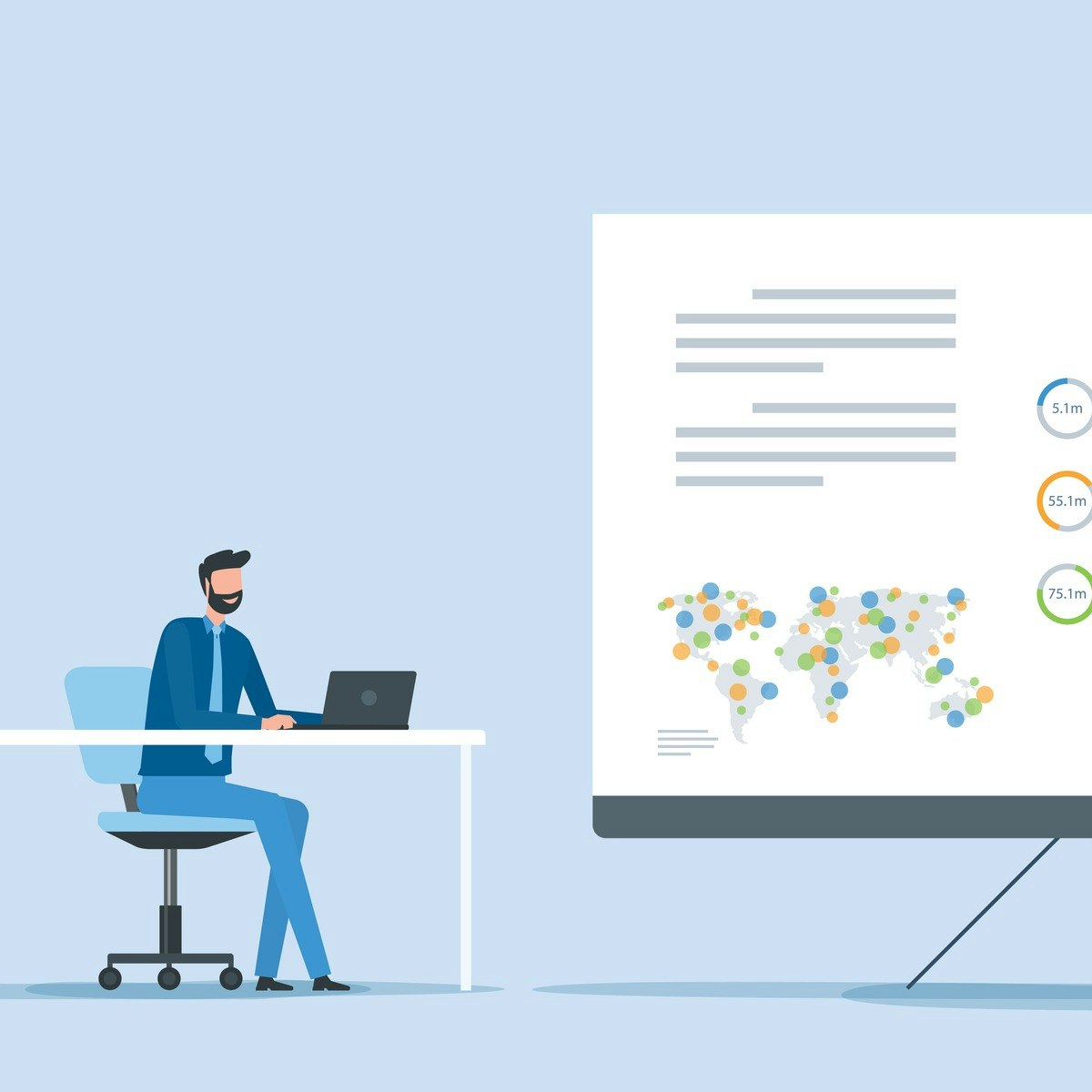
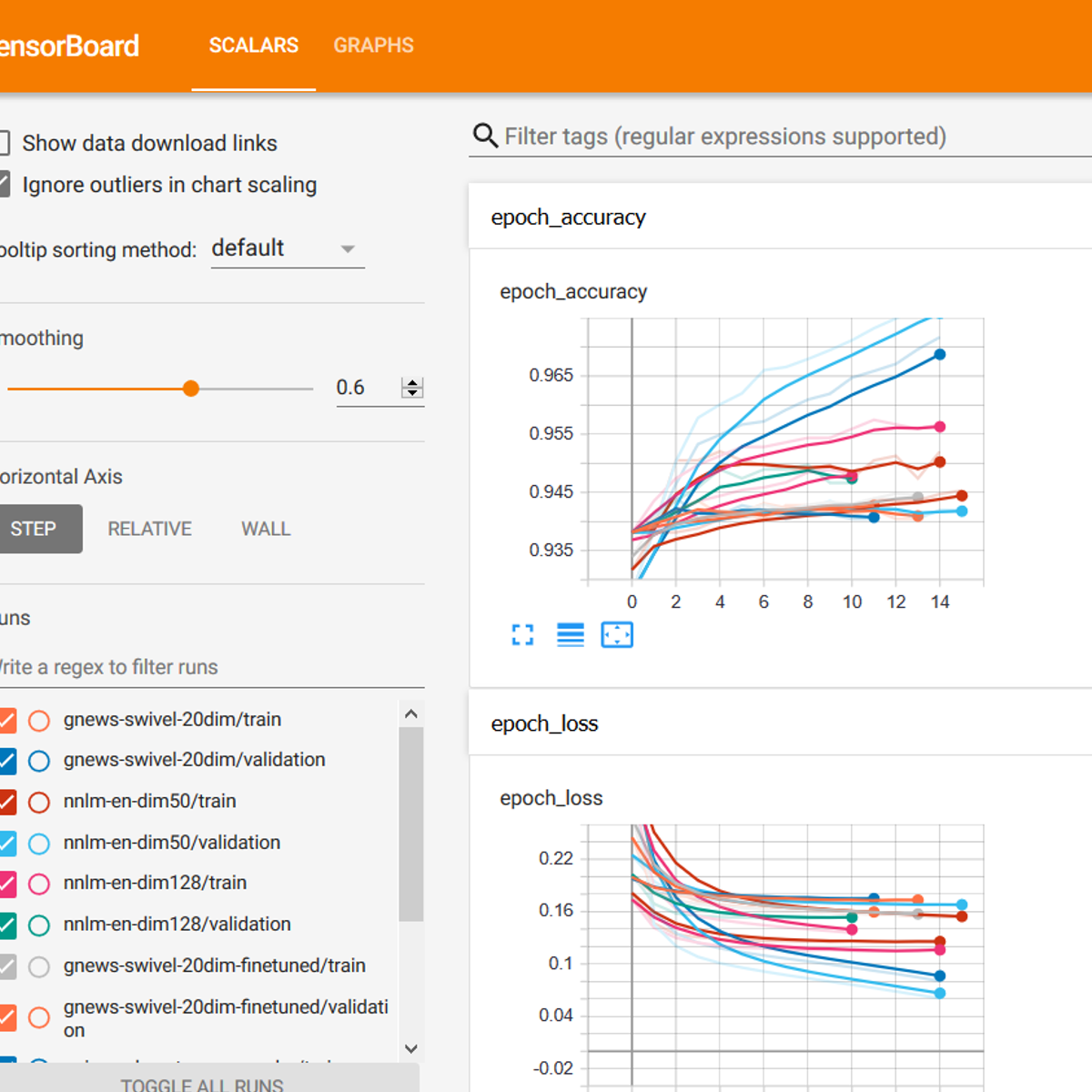
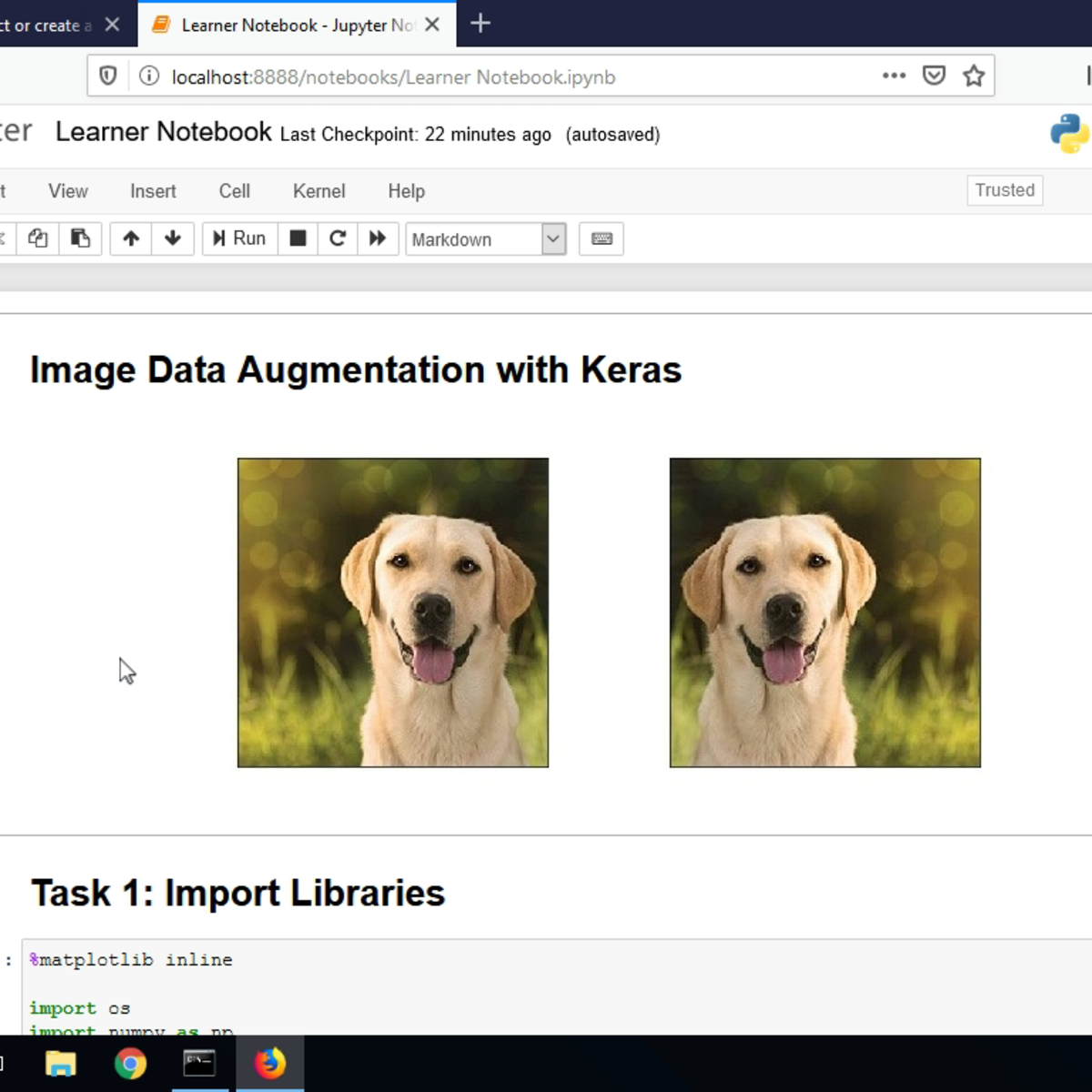
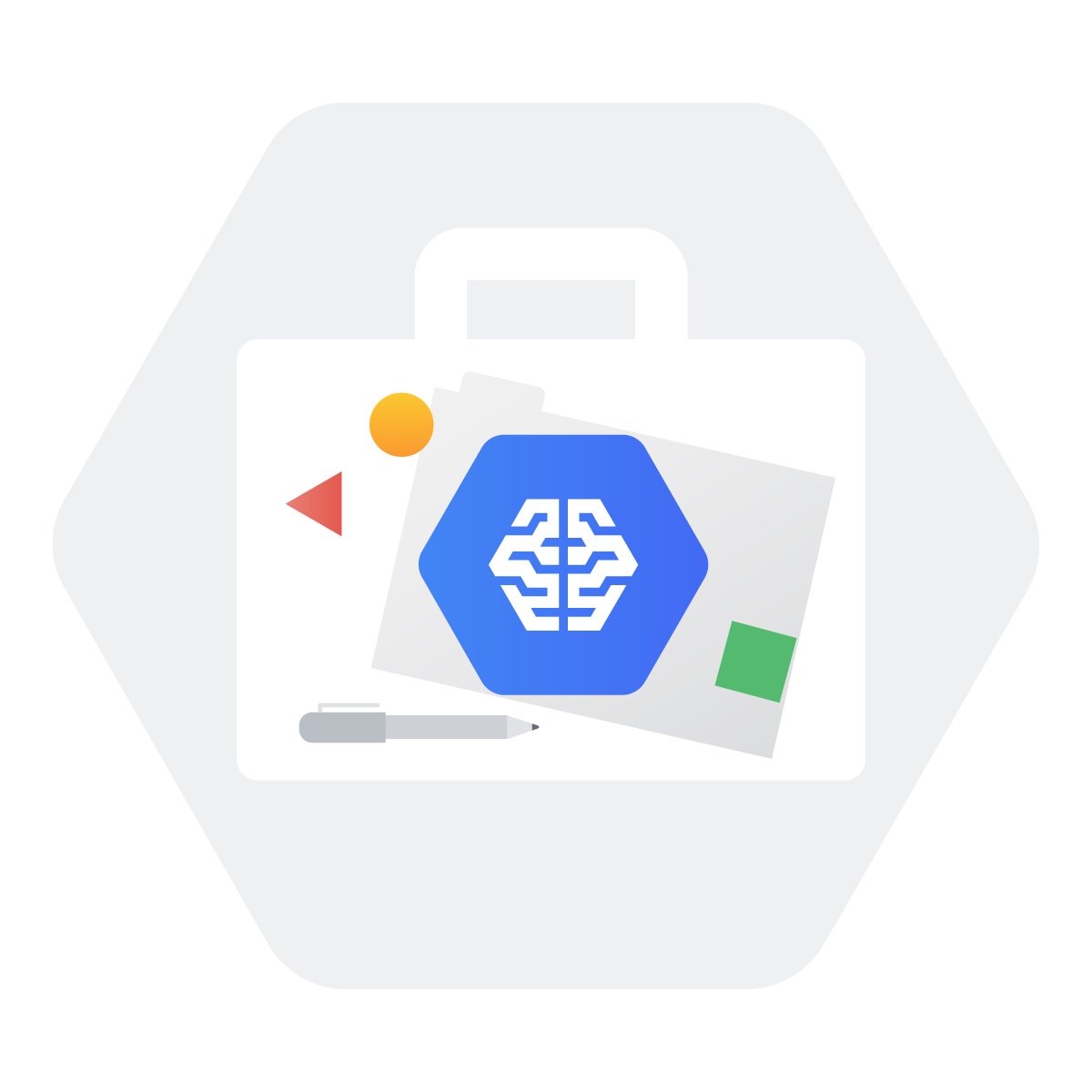
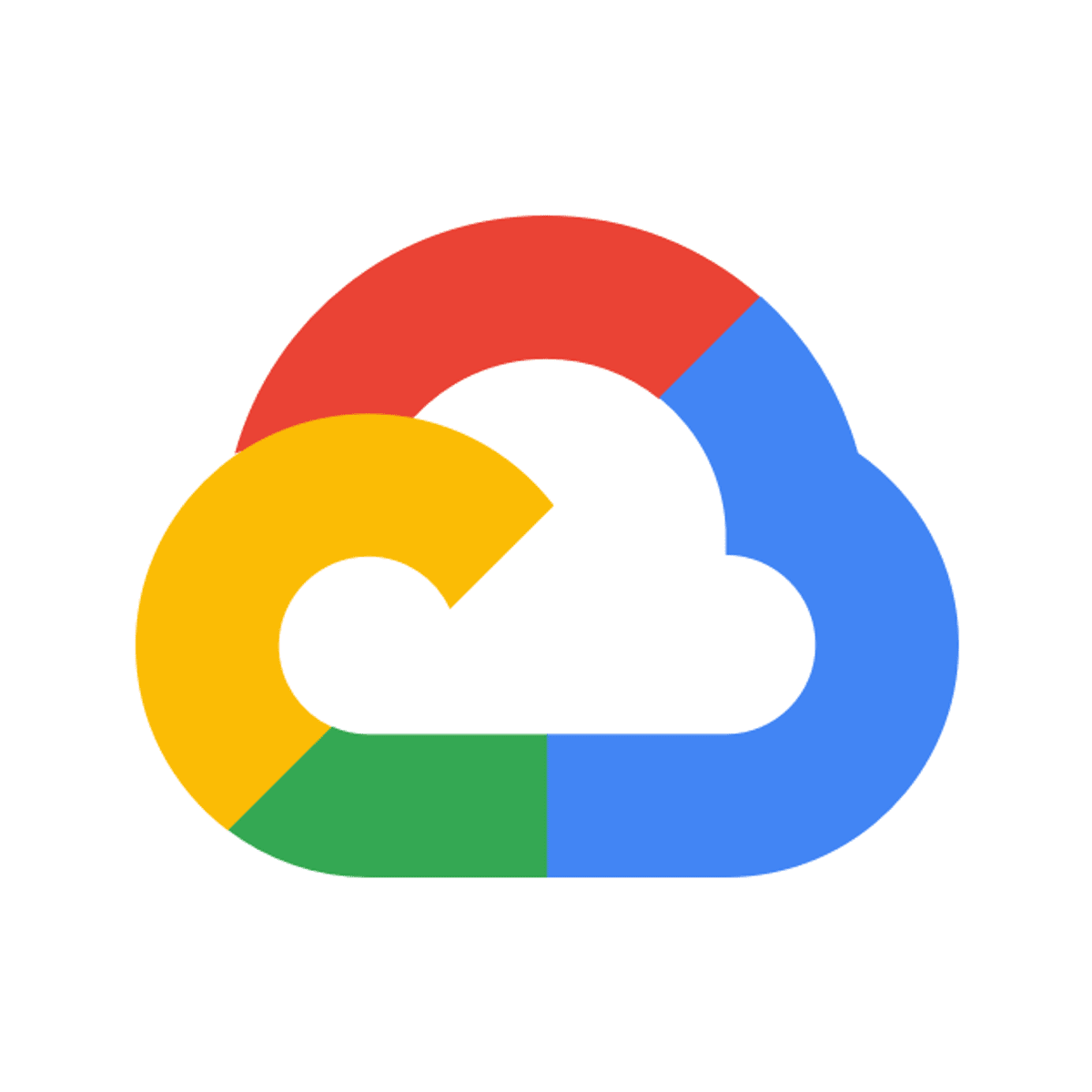
Data Science Courses - Page 12
Showing results 111-120 of 1407
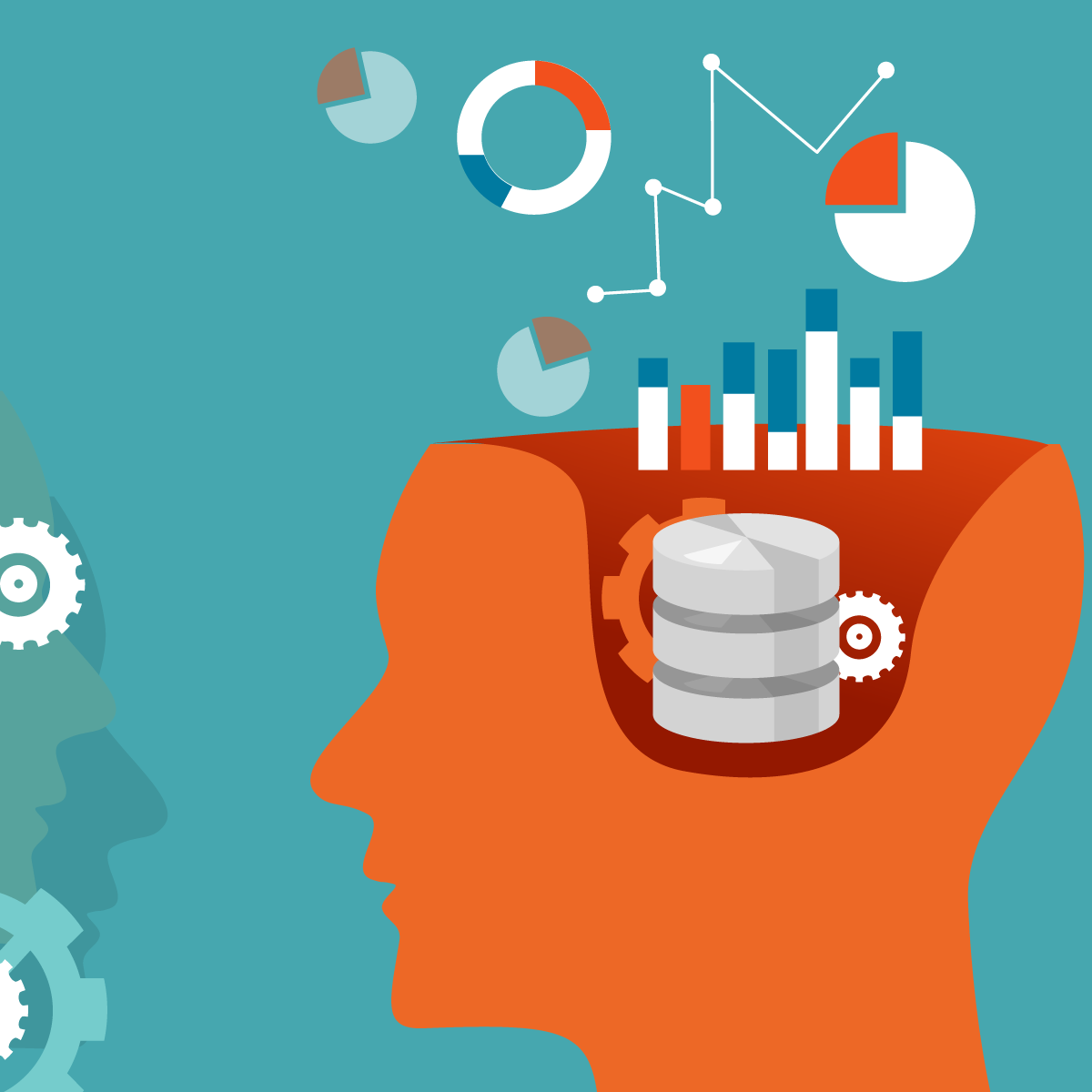
Data Engineering and Machine Learning using Spark
Organizations need skilled, forward-thinking Big Data practitioners who can apply their business and technical skills to unstructured data such as tweets, posts, pictures, audio files, videos, sensor data, and satellite imagery and more to identify behaviors and preferences of prospects, clients, competitors, and others.
In this short course you'll gain practical skills when you learn how to work with Apache Spark for Data Engineering and Machine Learning (ML) applications. You will work hands-on with Spark MLlib, Spark Structured Streaming, and more to perform extract, transform and load (ETL) tasks as well as Regression, Classification, and Clustering.
The course culminates in a project where you will apply your Spark skills to an ETL for ML workflow use-case.
NOTE: This course requires that you have foundational skills for working with Apache Spark and Jupyter Notebooks. The Introduction to Big Data with Spark and Hadoop course from IBM will equip you with these skills and it is recommended that you have completed that course or similar prior to starting this one.
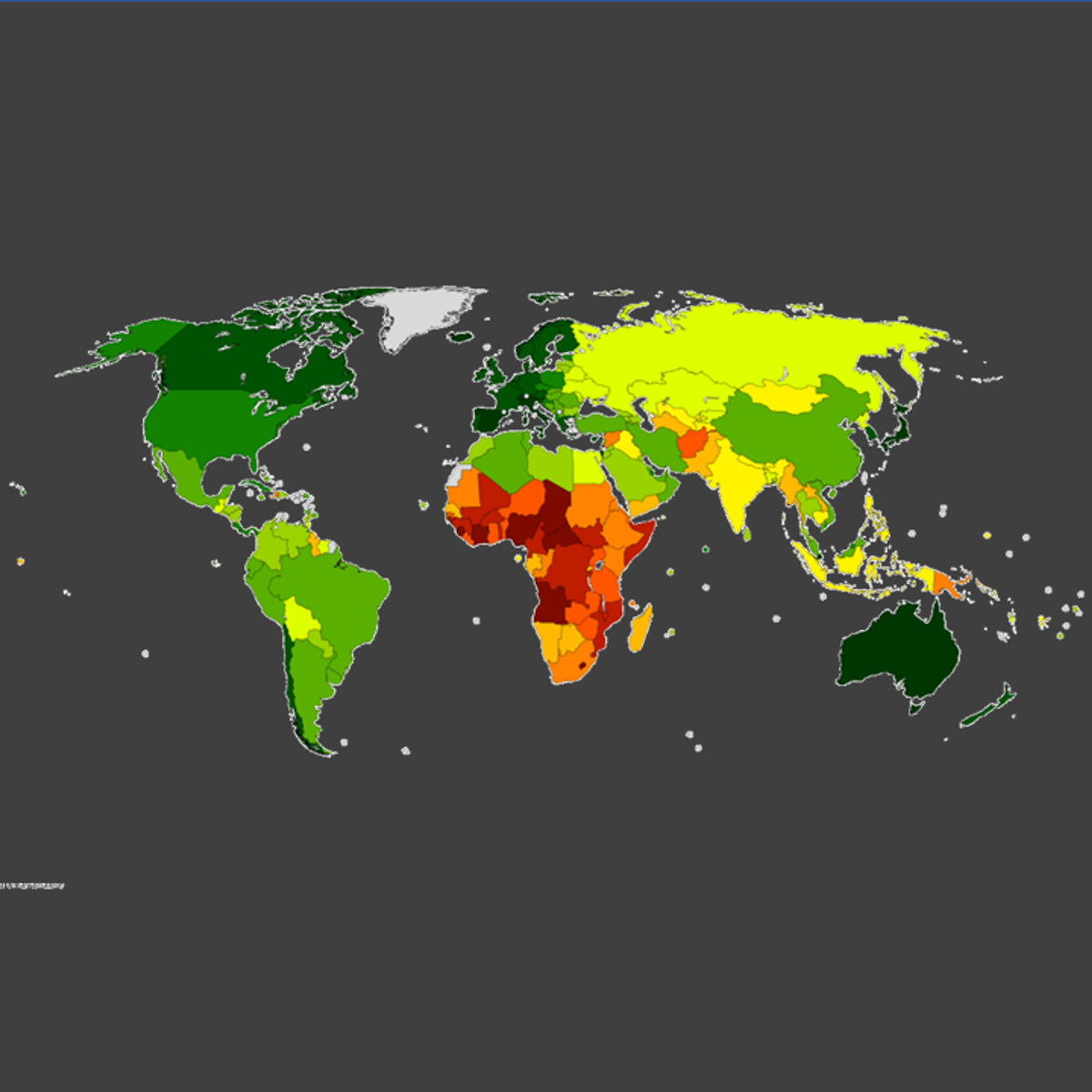
Life Expectancy Prediction Using Machine Learning
In this hands-on project, we will train a Linear Regression model to predict life expectancy. The dataset was initially obtained from the World Health Organization (WHO) and United Nations Websites. Data contains features such as year, status, life expectancy, adult mortality, infant deaths, percentage of expenditure, and alcohol consumption.
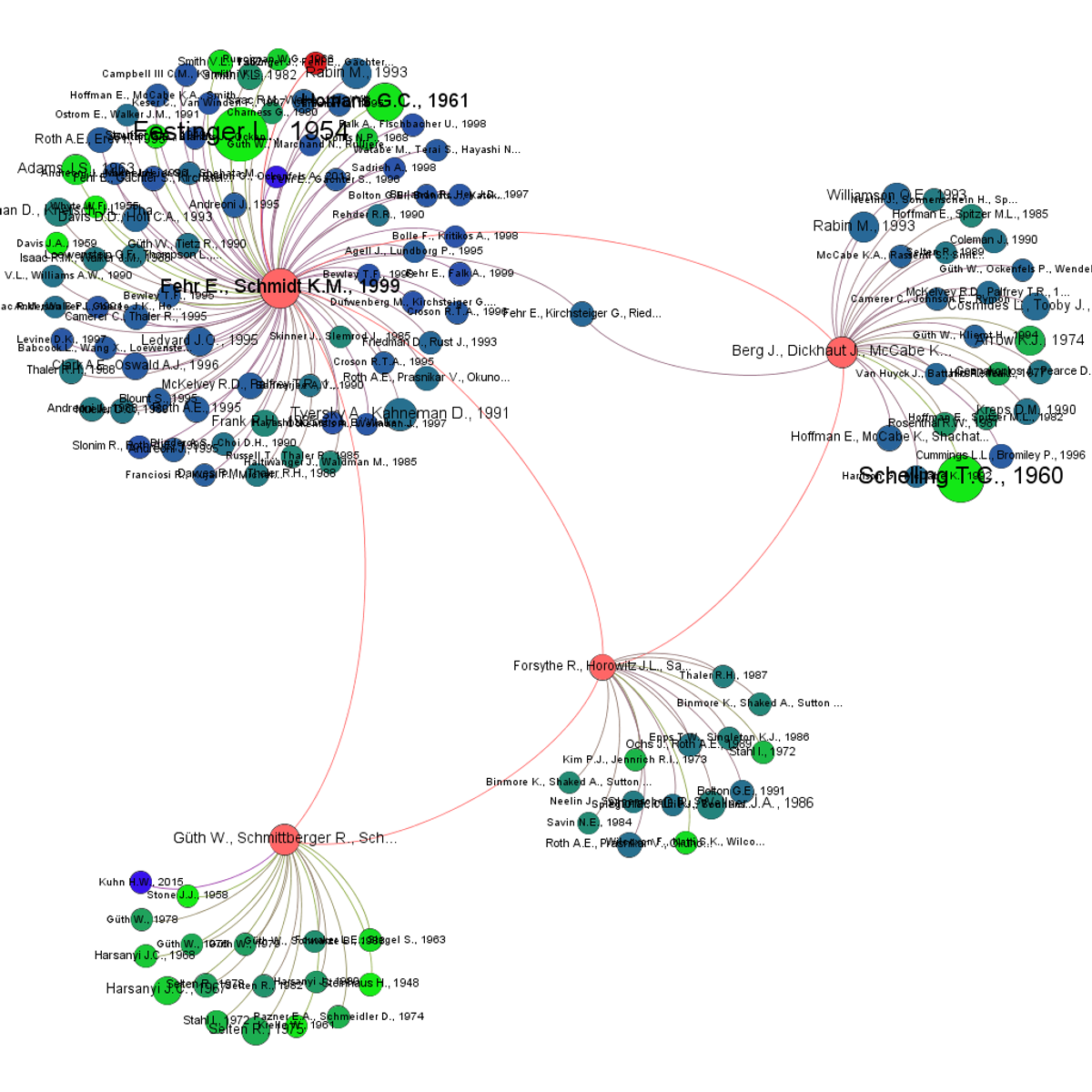
Citation Analysis for Bibliometric Study
In this 2 hour long project, you will learn to search and extract relevant research articles and their linked references efficiently from a journal database to conduct a bibliometric literature review. Then with these extracted data, you will learn to create a citation network. The visualization tool Gephi will be used in this project for citation network analysis. You will also learn, how to modify the network to present more information visually about the extracted citation data.
Note: This course works best for learners who are based in the North America region. We’re currently working on providing the same experience in other regions.
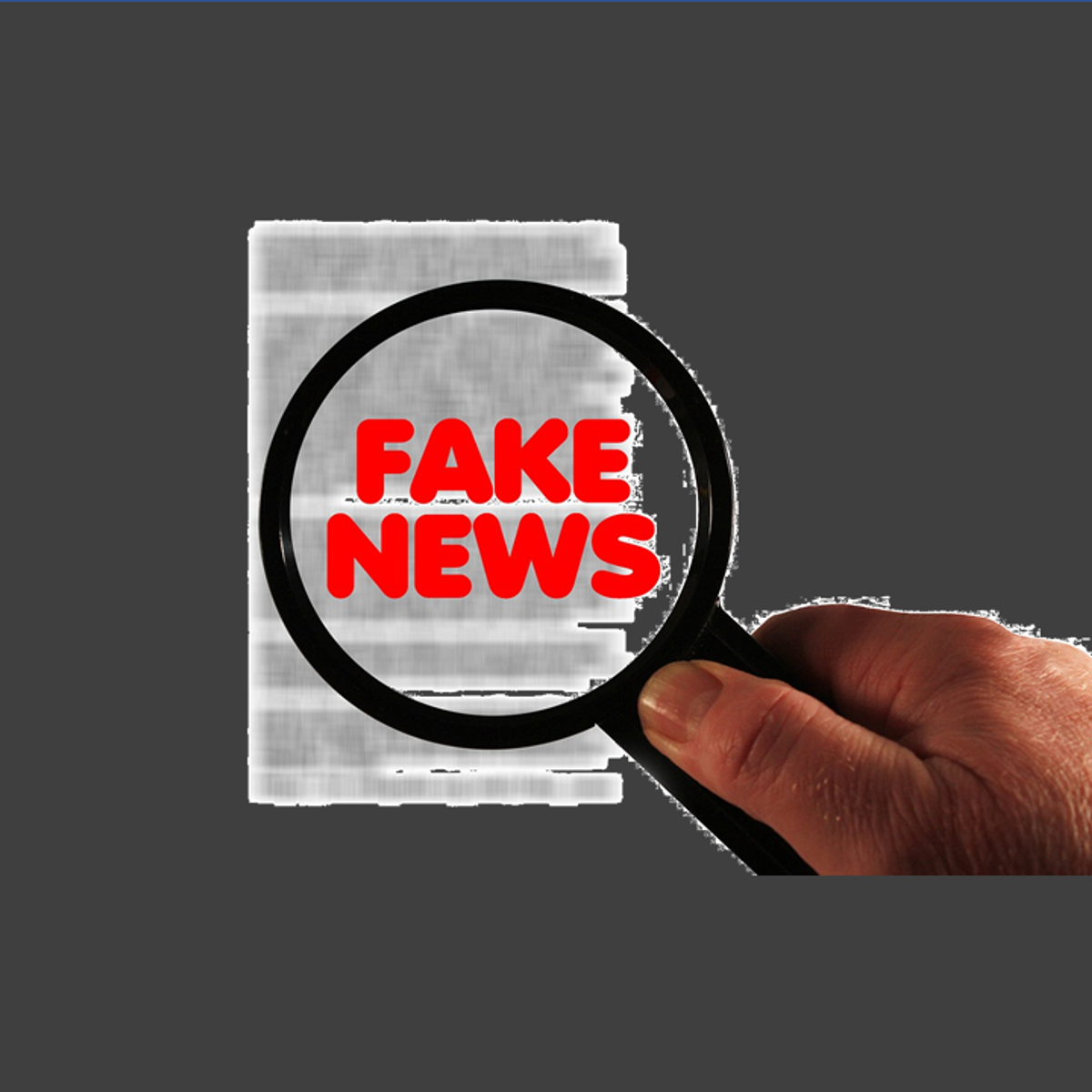
Fake News Detection with Machine Learning
In this hands-on project, we will train a Bidirectional Neural Network and LSTM based deep learning model to detect fake news from a given news corpus. This project could be practically used by any media company to automatically predict whether the circulating news is fake or not. The process could be done automatically without having humans manually review thousands of news related articles.
Note: This course works best for learners who are based in the North America region. We’re currently working on providing the same experience in other regions.
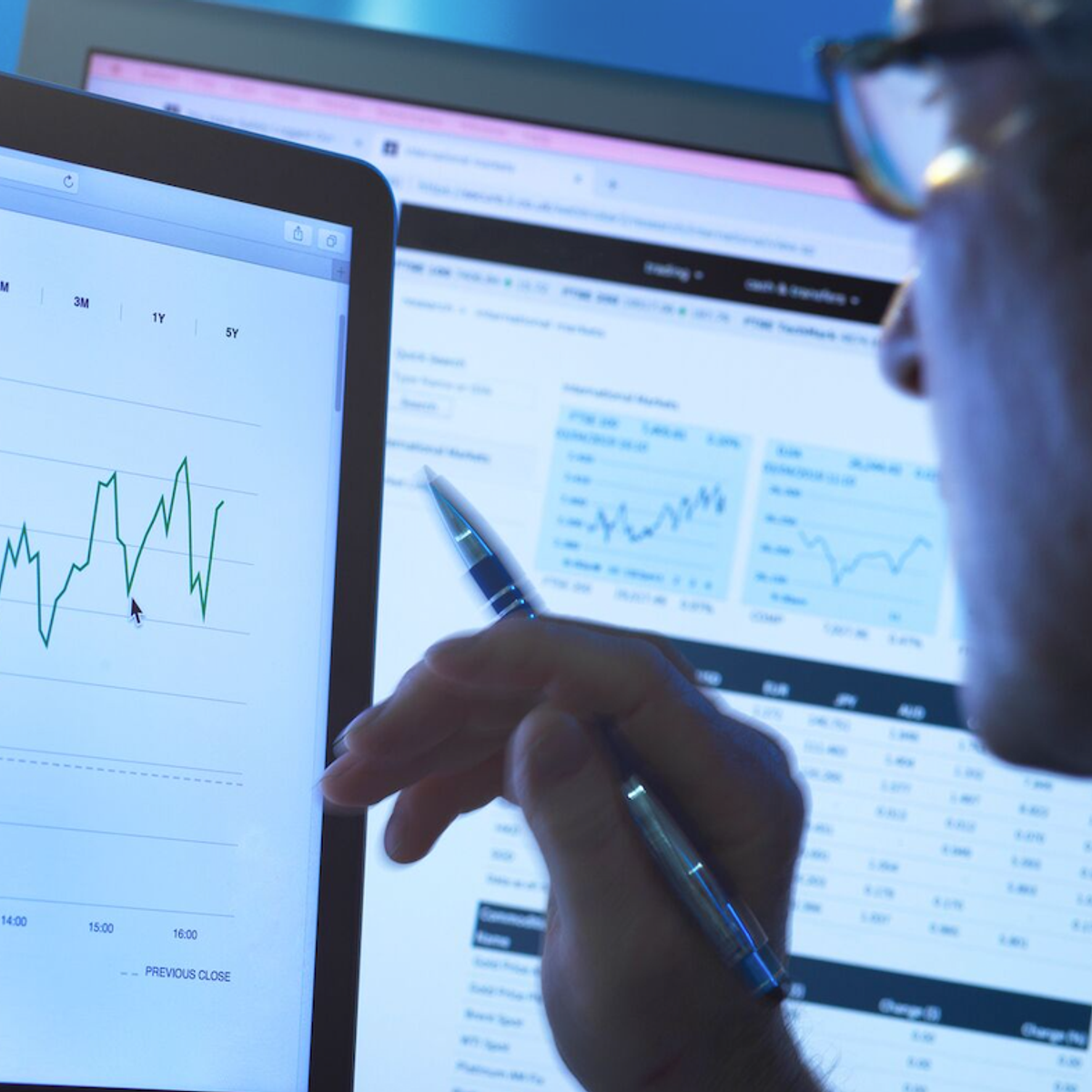
Applied Data Science for Data Analysts
In this course, you will develop your data science skills while solving real-world problems. You'll work through the data science process to and use unsupervised learning to explore data, engineer and select meaningful features, and solve complex supervised learning problems using tree-based models. You will also learn to apply hyperparameter tuning and cross-validation strategies to improve model performance.
NOTE: This is the third and final course in the Data Science with Databricks for Data Analysts Coursera specialization. To be successful in this course we highly recommend taking the first two courses in that specialization prior to taking this course. These courses are: Apache Spark for Data Analysts and Data Science Fundamentals for Data Analysts.
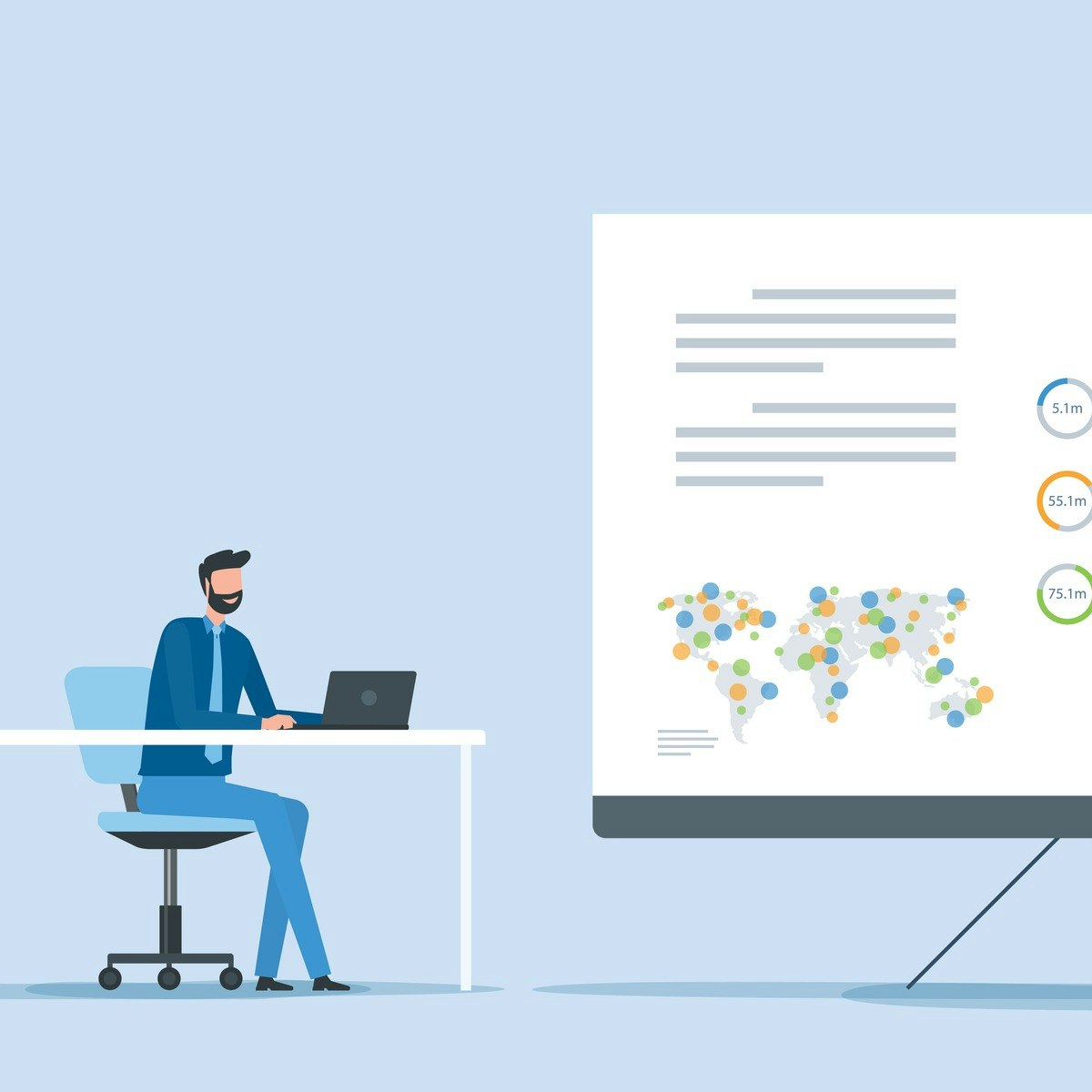
Politics and Ethics of Data Analytics in the Public Sector
Deepen your understanding of the power and politics of data in the public sector, including how values — in addition to data and evidence — are always part of public sector decision-making. In this course, you will explore common ethical challenges associated with data, data analytics, and randomized controlled trials in the public sector. You will also navigate and understand the ethical issues related to data systems and data analysis by understanding frameworks, codes of ethics, and professional guidelines. Using two technical case studies, you will understand common ethical issues, including participation bias in populations and how slicing analysis is used to identify bias in predictive machine learning models. This course also serves as a capstone experience for the Data Analytics in the Public Sector with R Specialization, where you will conduct an applied policy options analysis using authentic data from a real-world case study. In this capstone exercise, you will review data as part of policy options analysis, create a visualization of the results, and make a recommendation.
All coursework is completed in RStudio in Coursera without the need to install additional software.
This is the fourth and final course within the Data Analytics in the Public Sector with R Specialization. The series is ideal for current or early-career professionals working in the public sector looking to gain skills in analyzing public data effectively. It is also ideal for current data analytics professionals or students looking to enter the public sector.
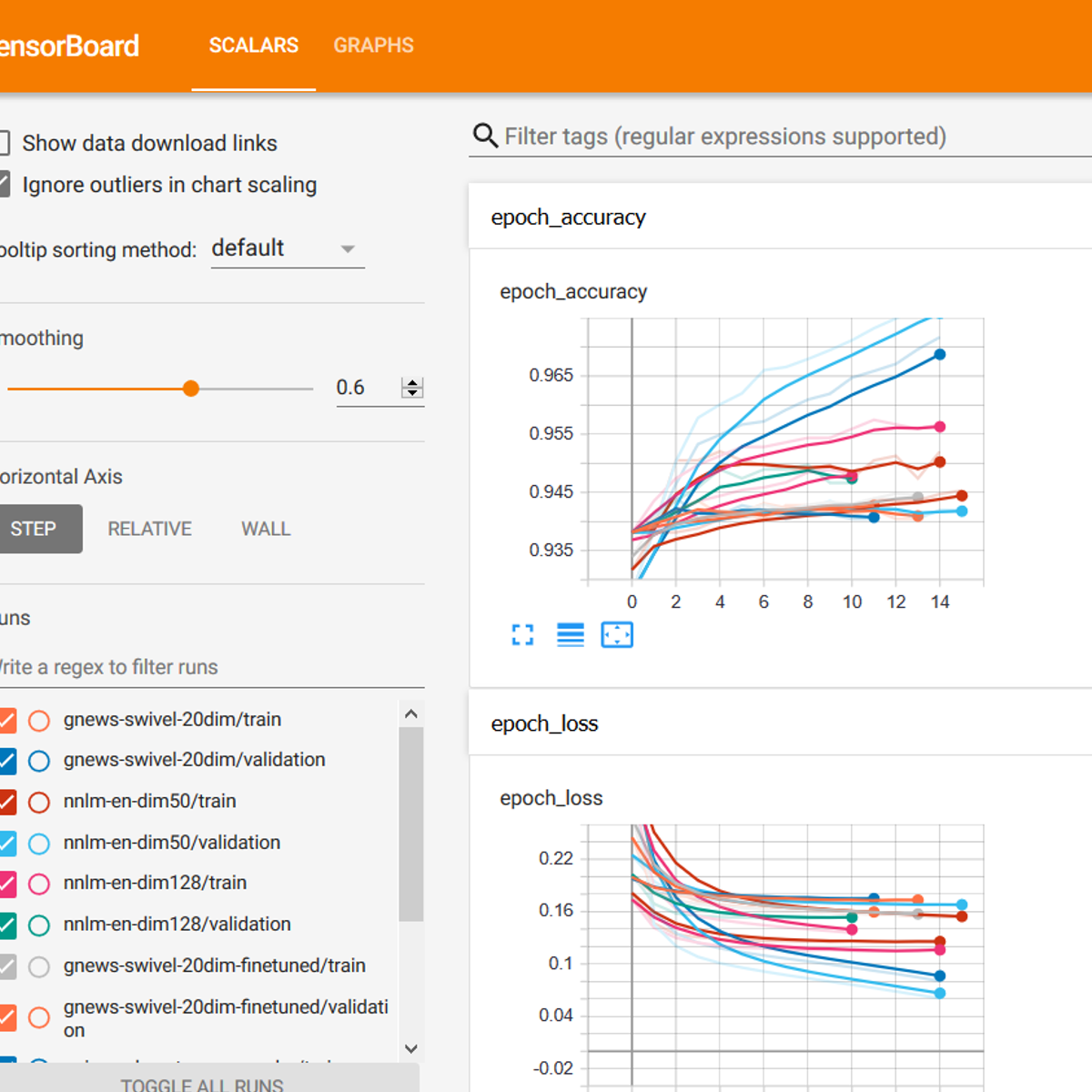
Transfer Learning for NLP with TensorFlow Hub
This is a hands-on project on transfer learning for natural language processing with TensorFlow and TF Hub. By the time you complete this project, you will be able to use pre-trained NLP text embedding models from TensorFlow Hub, perform transfer learning to fine-tune models on real-world data, build and evaluate multiple models for text classification with TensorFlow, and visualize model performance metrics with Tensorboard.
Prerequisites:
In order to successfully complete this project, you should be competent in the Python programming language, be familiar with deep learning for Natural Language Processing (NLP), and have trained models with TensorFlow or and its Keras API.
Note: This course works best for learners who are based in the North America region. We’re currently working on providing the same experience in other regions.
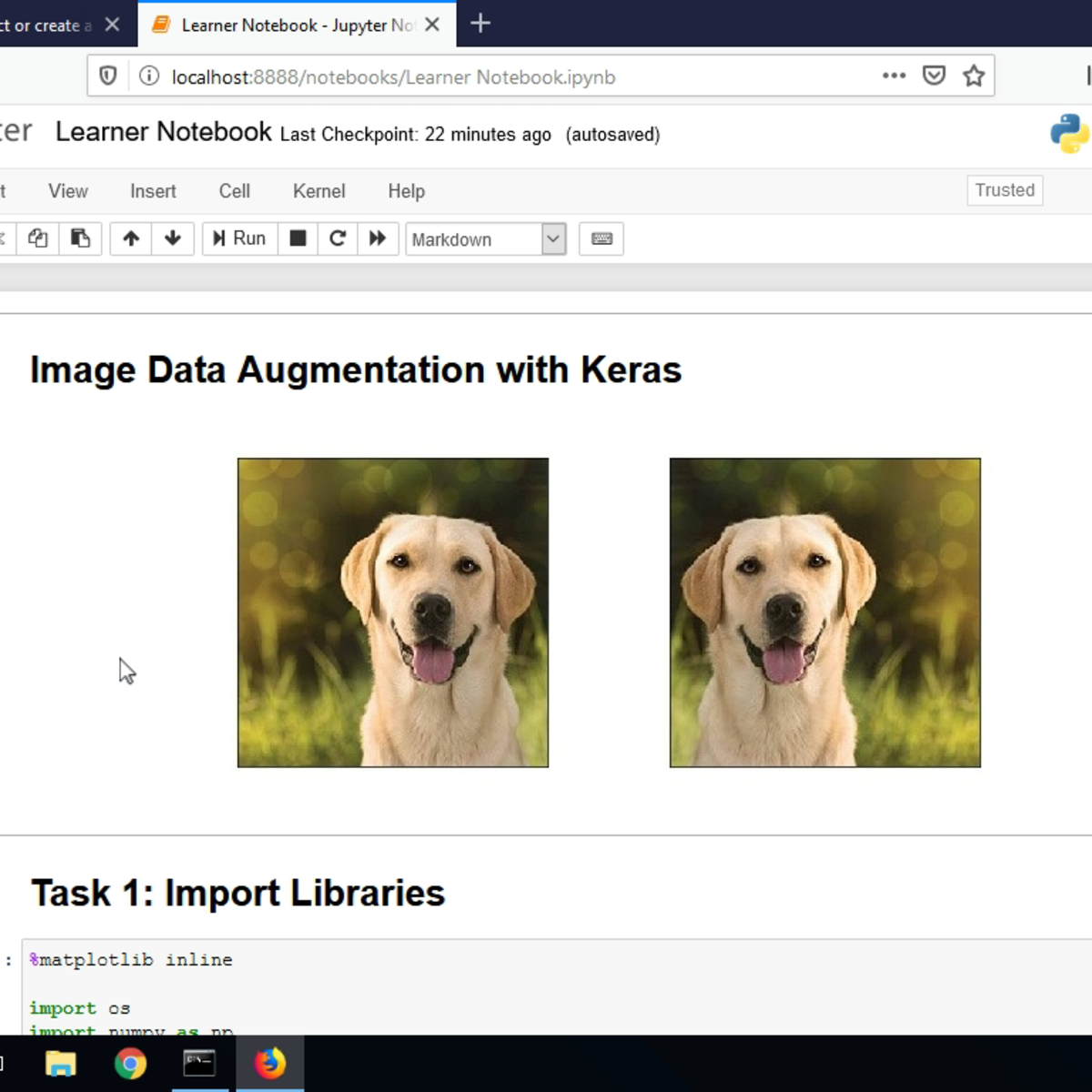
Image Data Augmentation with Keras
In this 1.5-hour long project-based course, you will learn how to apply image data augmentation in Keras. We are going to focus on using the ImageDataGenerator class from Keras’ image preprocessing package, and will take a look at a variety of options available in this class for data augmentation and data normalization.
Since this is a practical, project-based course, you will need to prior experience with Python programming, convolutional neural networks, and Keras with a TensorFlow backend.
Data augmentation is a technique used to create more examples, artificially, from an existing dataset. This is useful if your dataset is small and you want to increase the number of examples. Data augmentation can often solve over-fitting so that your model generalizes well after training. For images, a variety of augmentation can be applied to increase the number of examples.
Note: This course works best for learners who are based in the North America region. We’re currently working on providing the same experience in other regions.
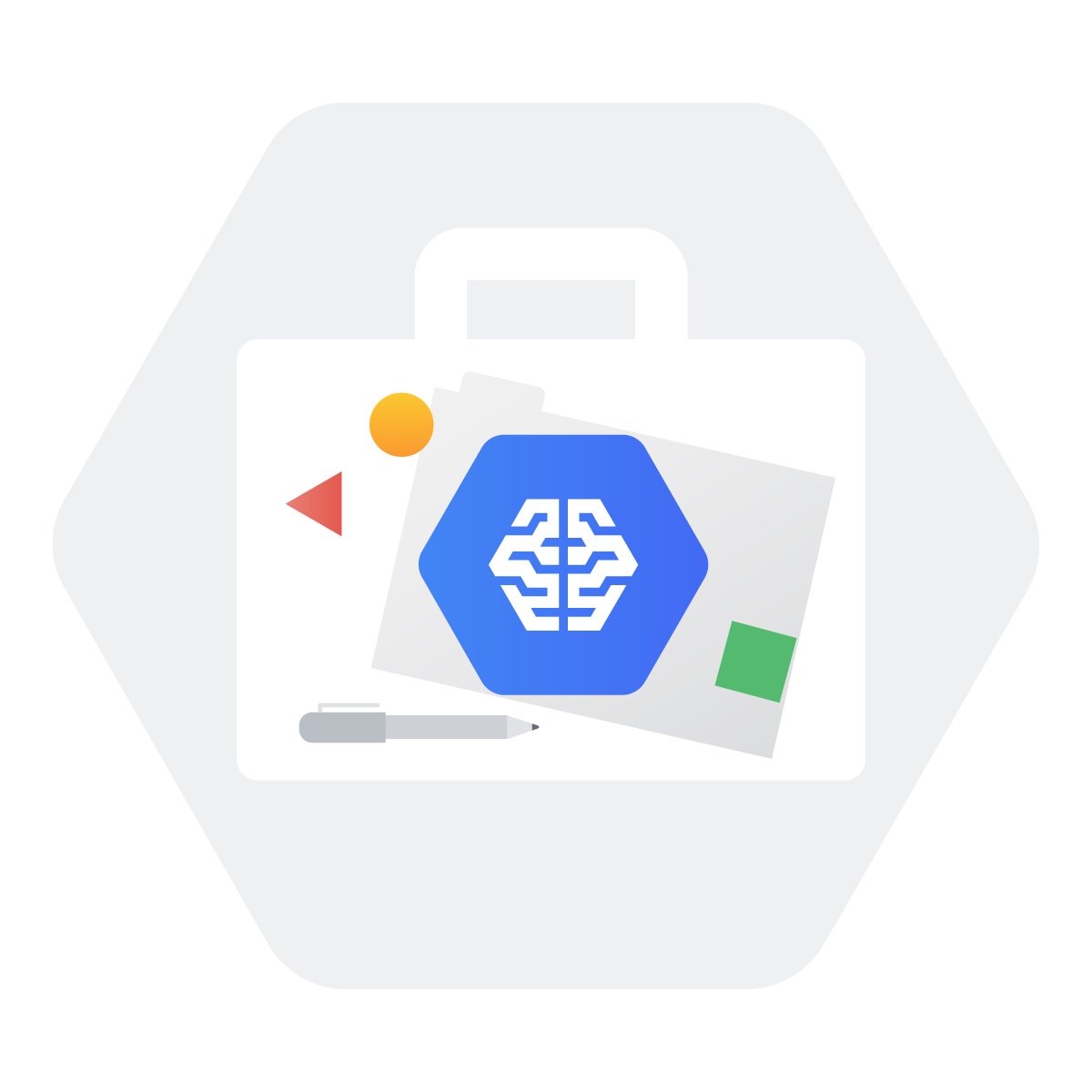
Managing Machine Learning Projects with Google Cloud
Business professionals in non-technical roles have a unique opportunity to lead or influence machine learning projects. If you have questions about machine learning and want to understand how to use it, without the technical jargon, this course is for you. Learn how to translate business problems into machine learning use cases and vet them for feasibility and impact. Find out how you can discover unexpected use cases, recognize the phases of an ML project and considerations within each, and gain confidence to propose a custom ML use case to your team or leadership or translate the requirements to a technical team.
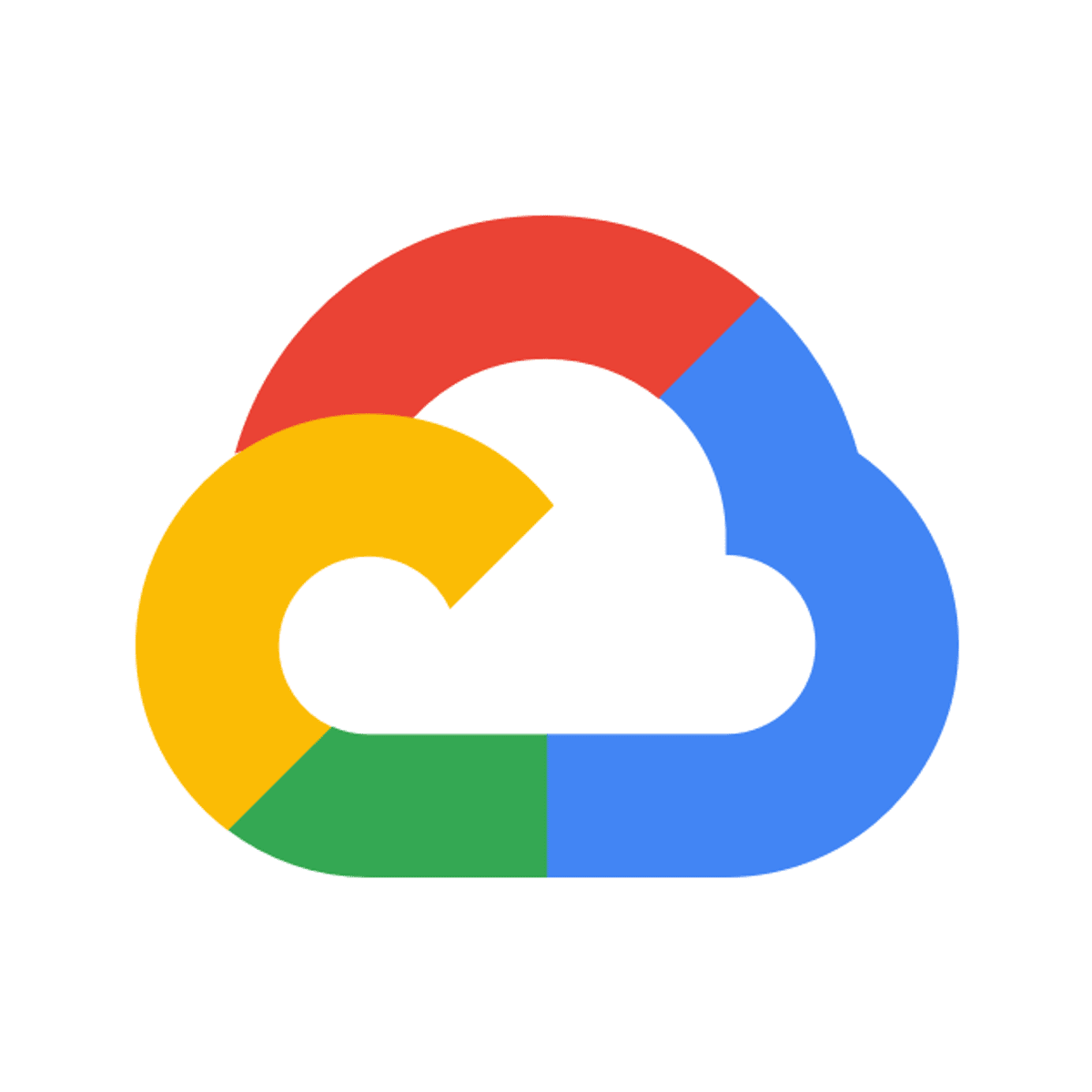
Using Cloud Trace on Kubernetes Engine
This is a self-paced lab that takes place in the Google Cloud console. This lab deployings a Kubernetes Engine cluster, then a simple web application fronted by a load balancer is deployed to the cluster. The web app publishes messages provided by the user to a Cloud Pub/Sub topic. You will see the correlated telemetry data from HTTP requests to the app will be available in the Cloud Trace Console.
Popular Internships and Jobs by Categories
Find Jobs & Internships
Browse
© 2024 BoostGrad | All rights reserved