Back to Courses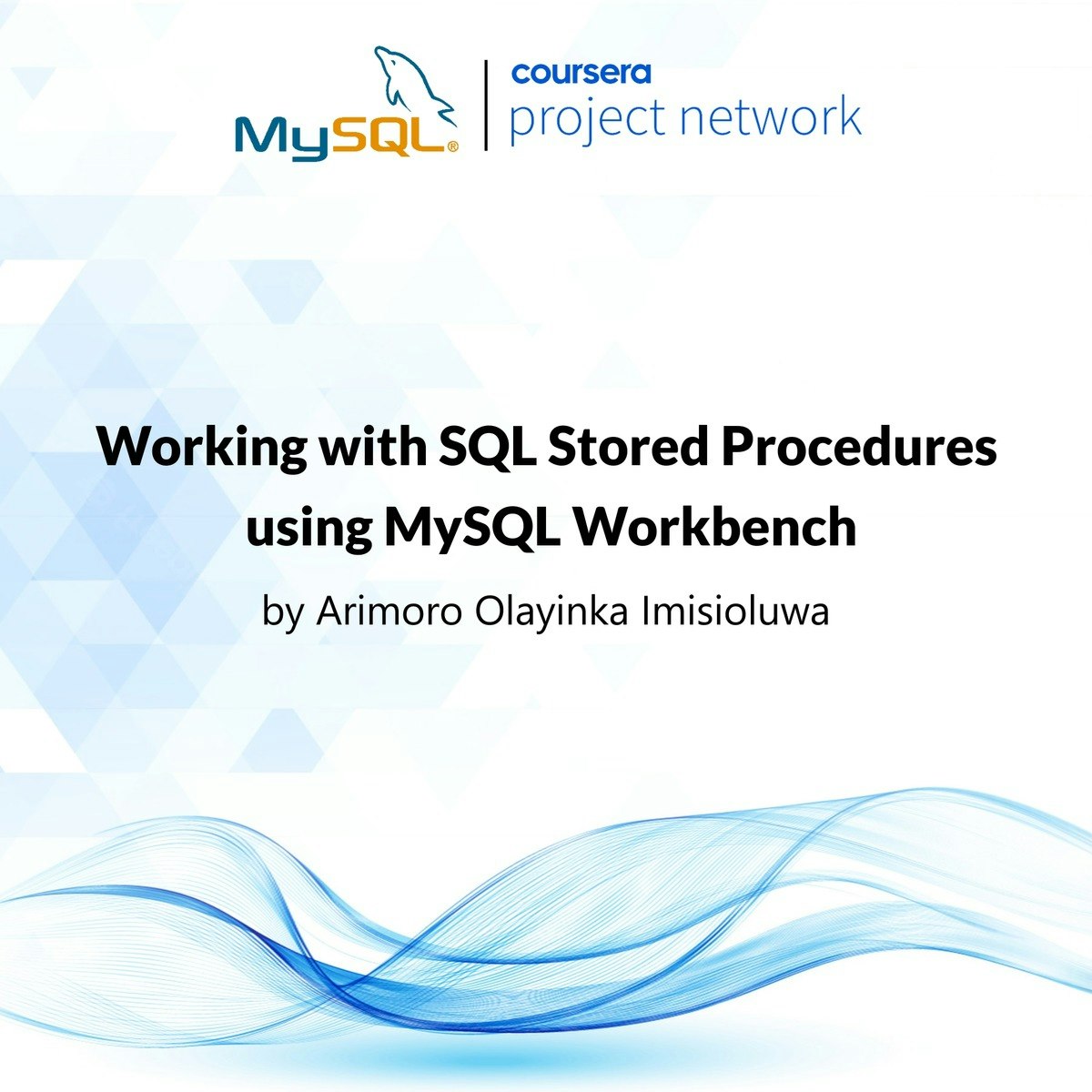
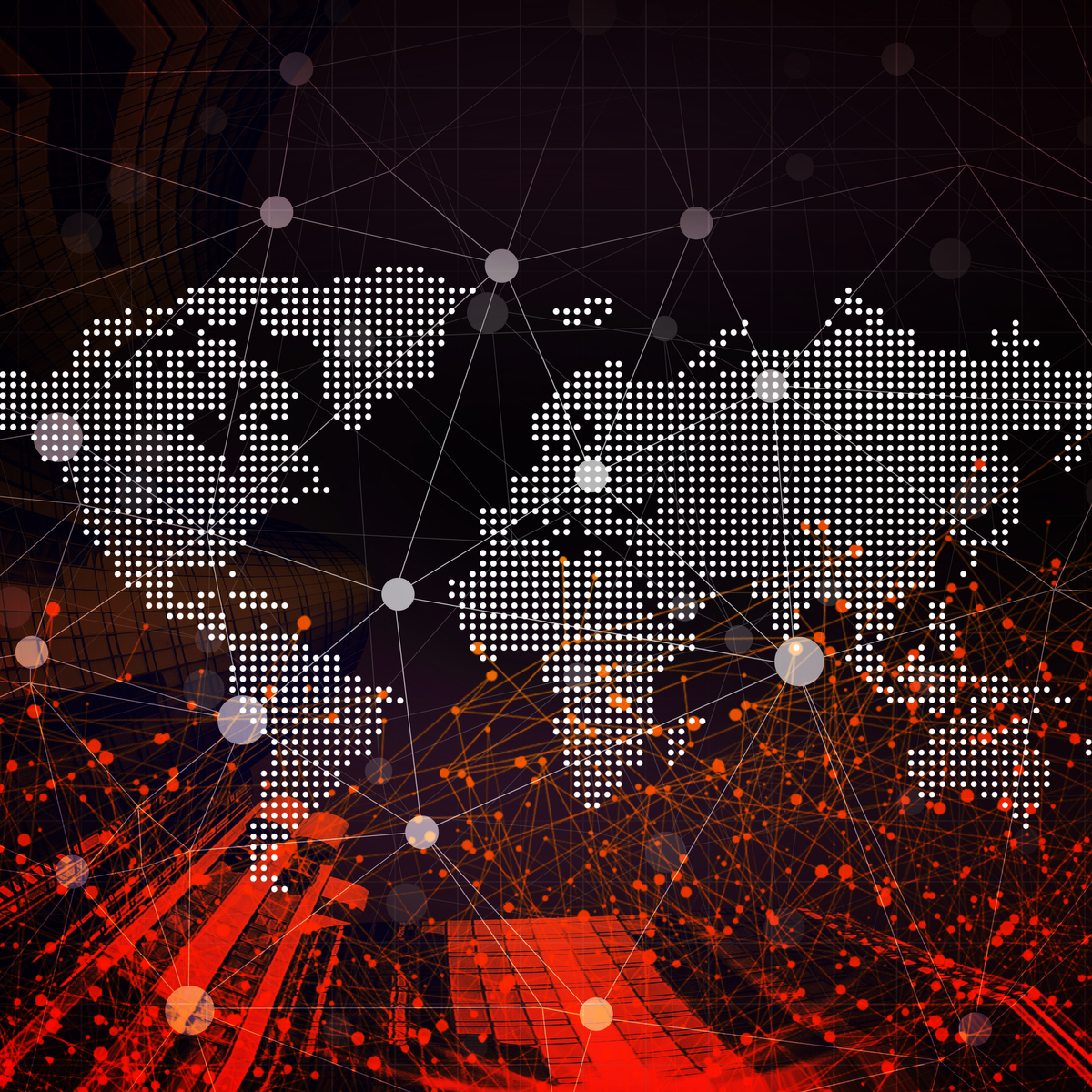
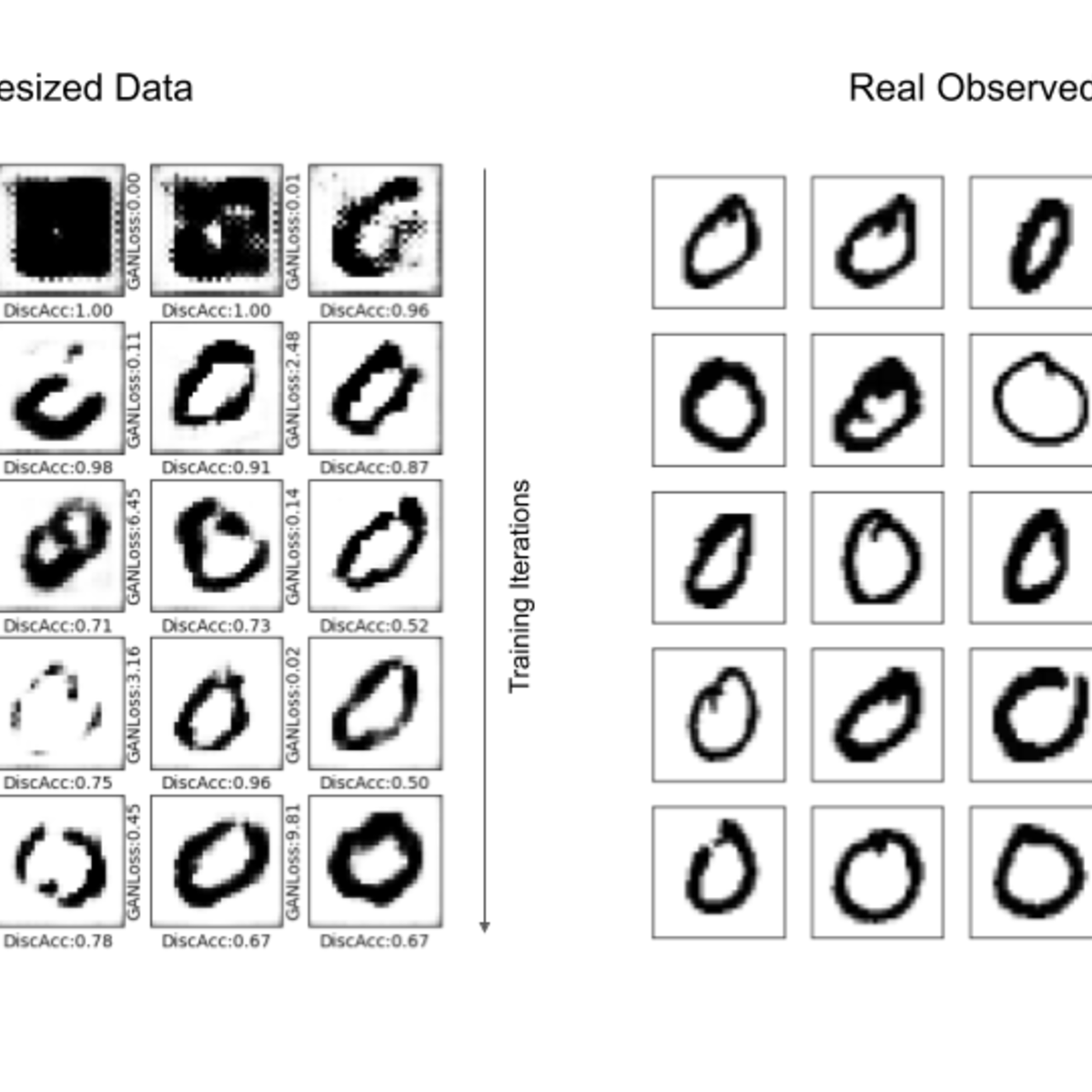
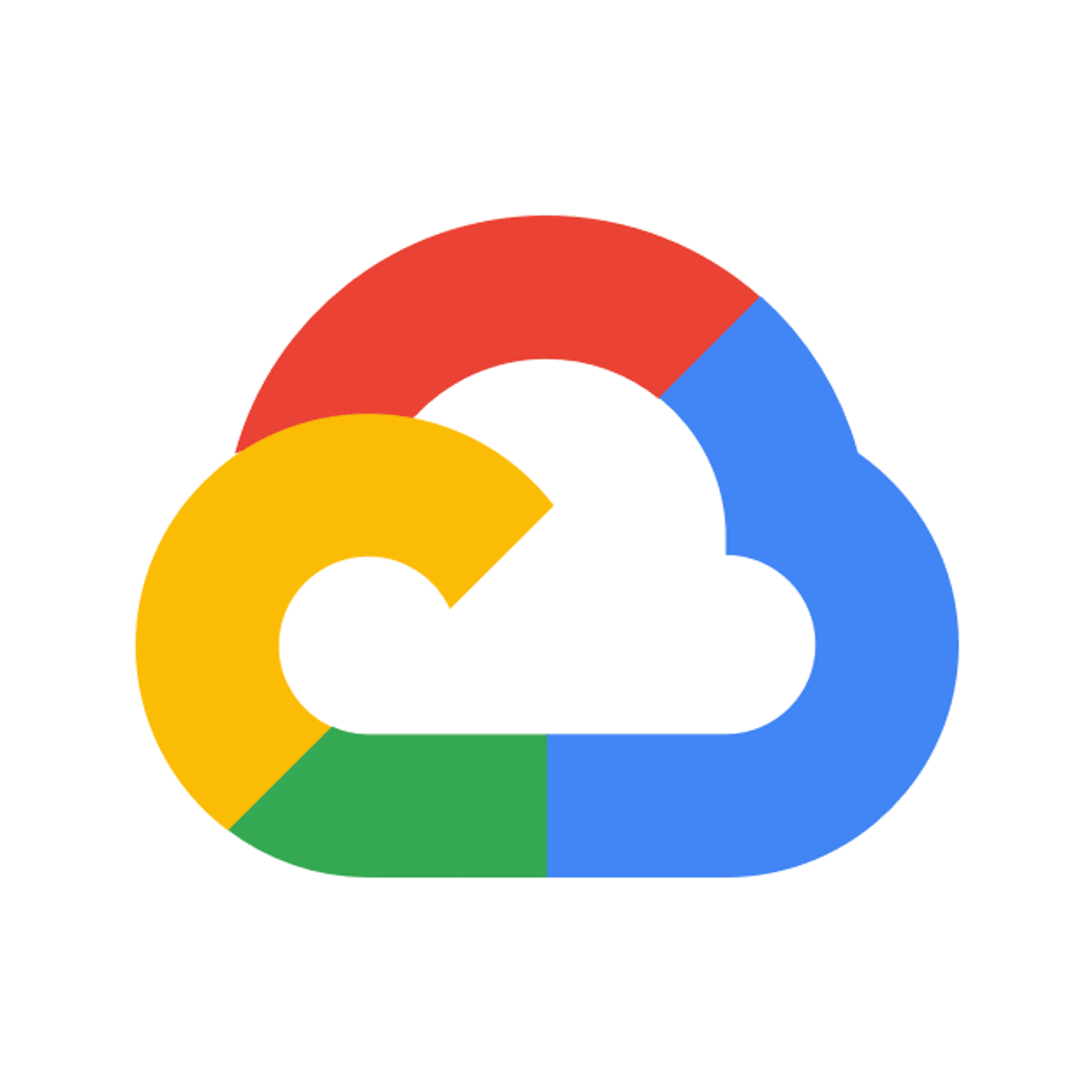
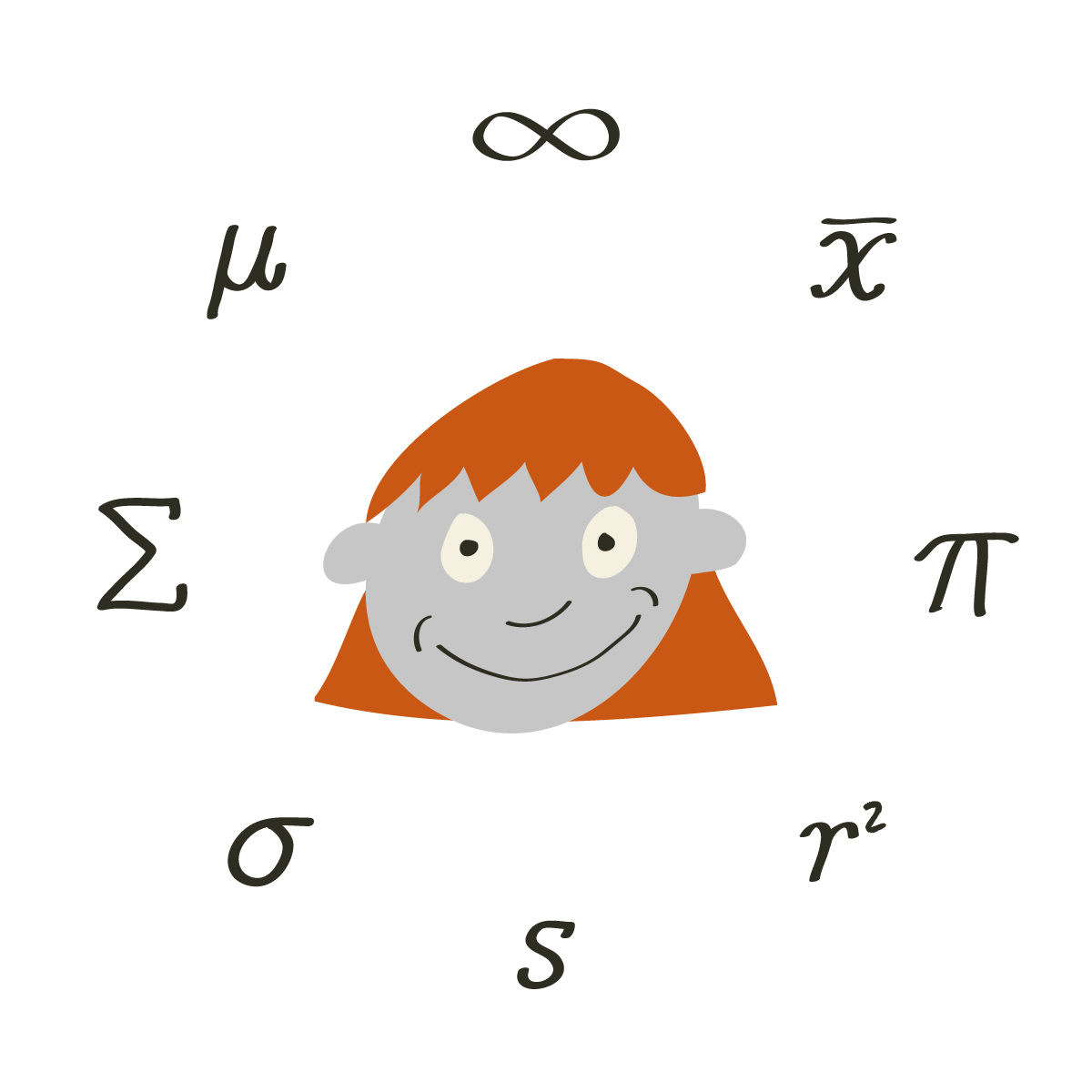
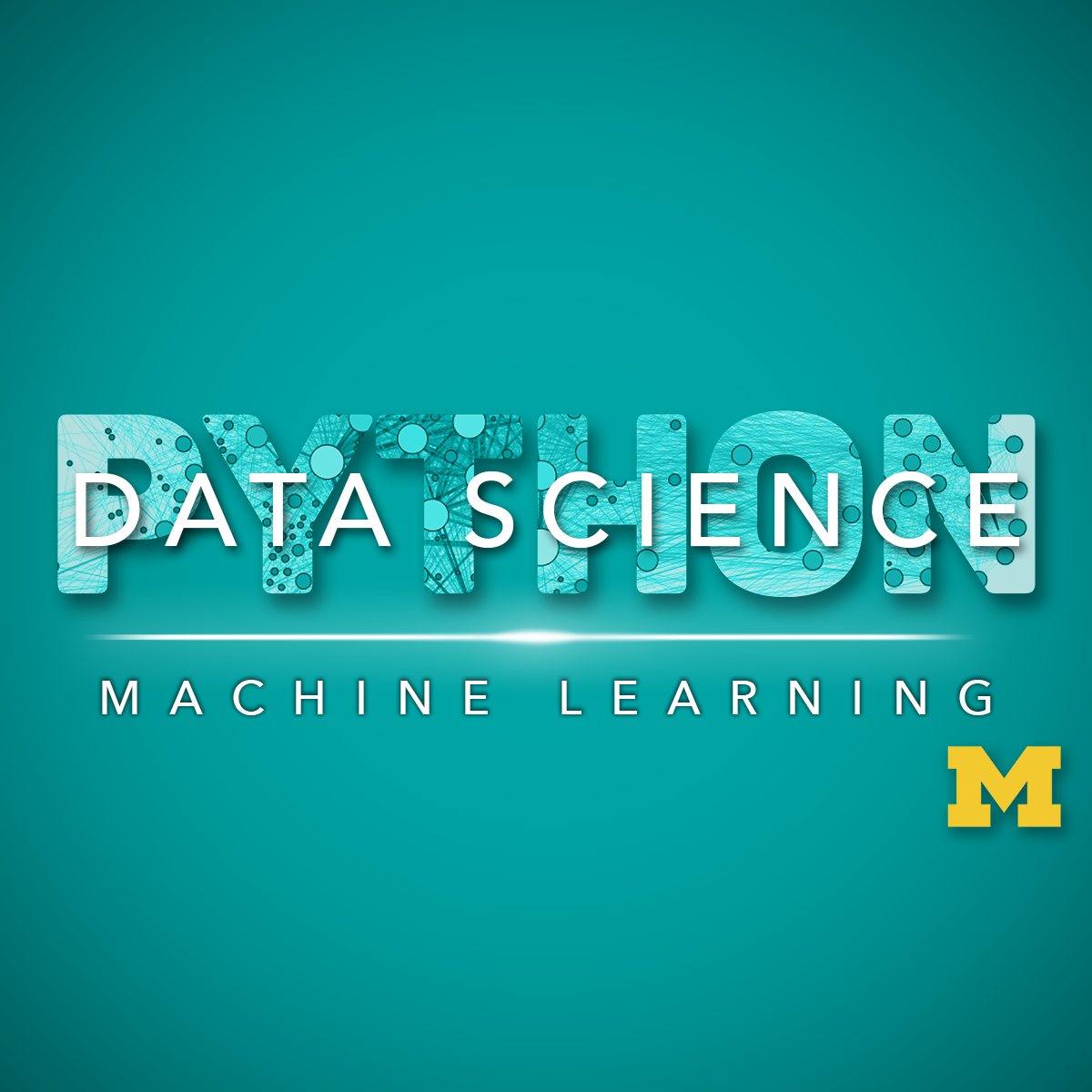
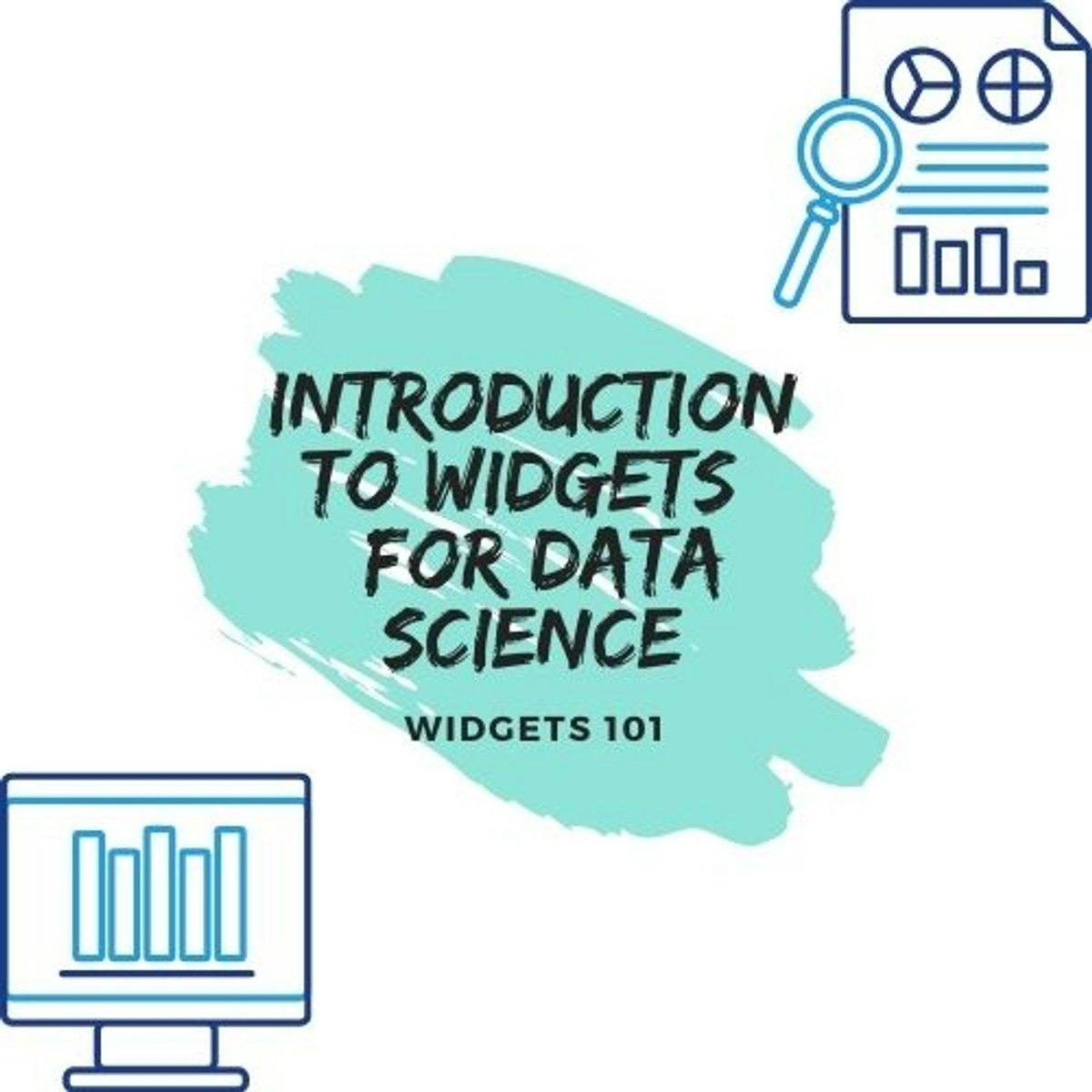
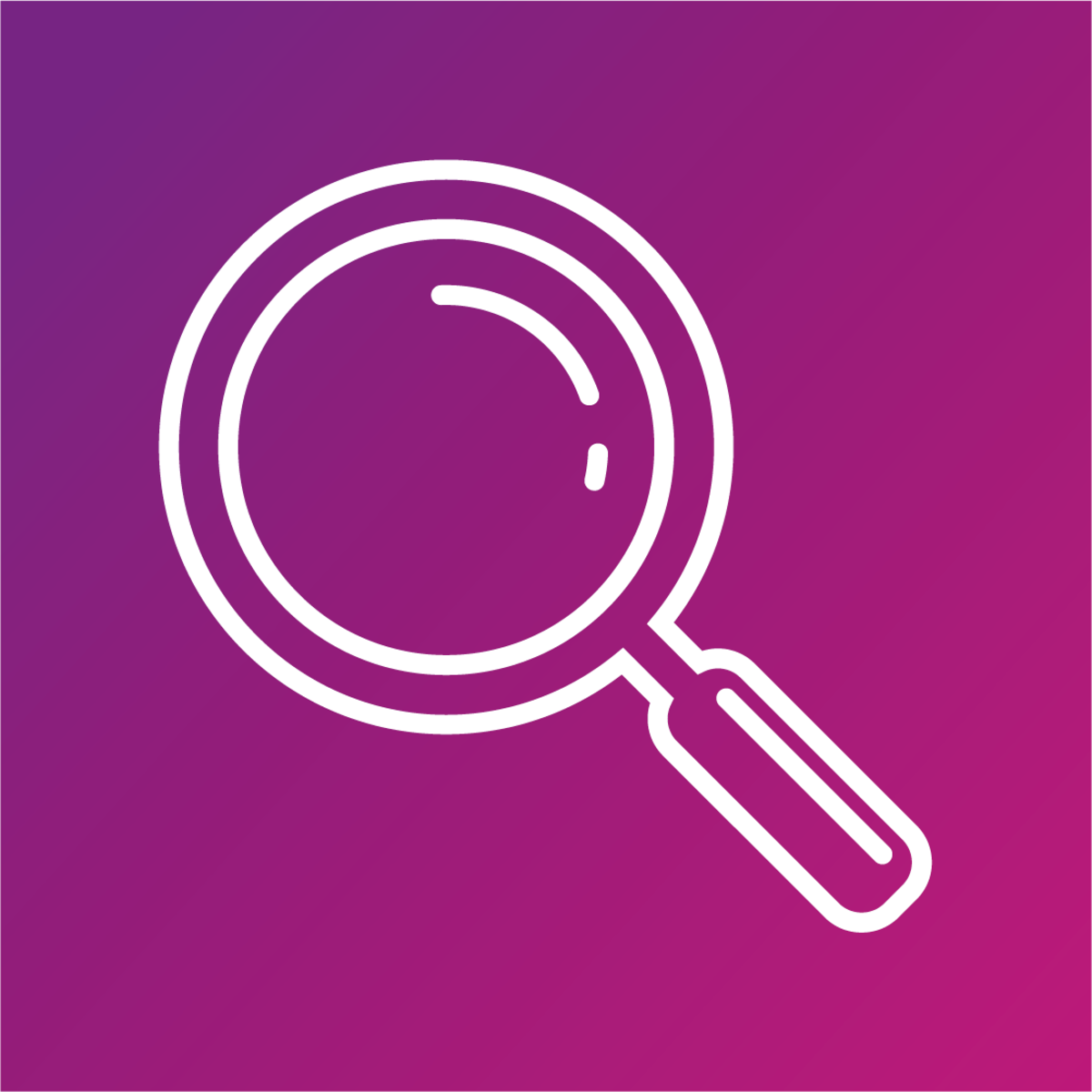
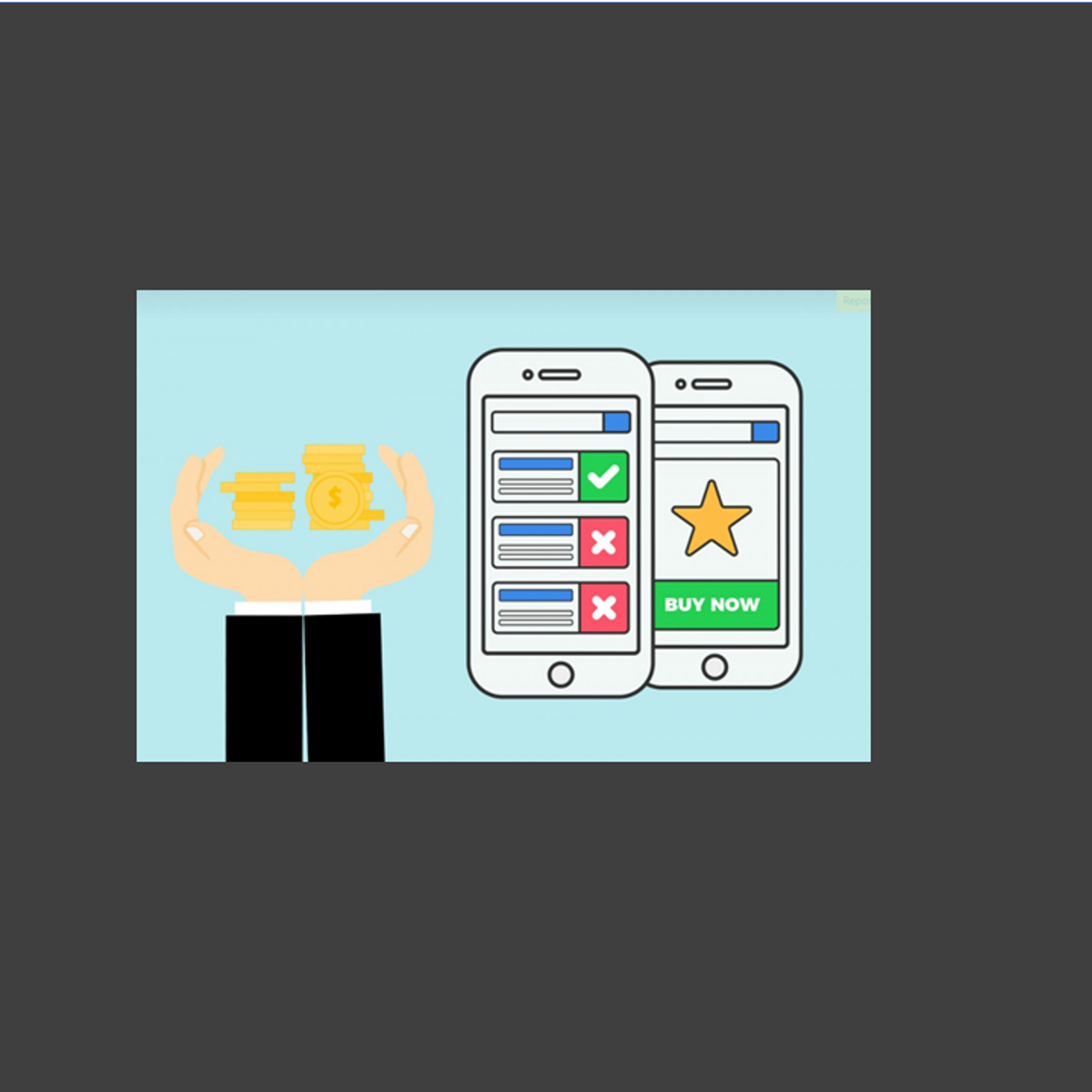
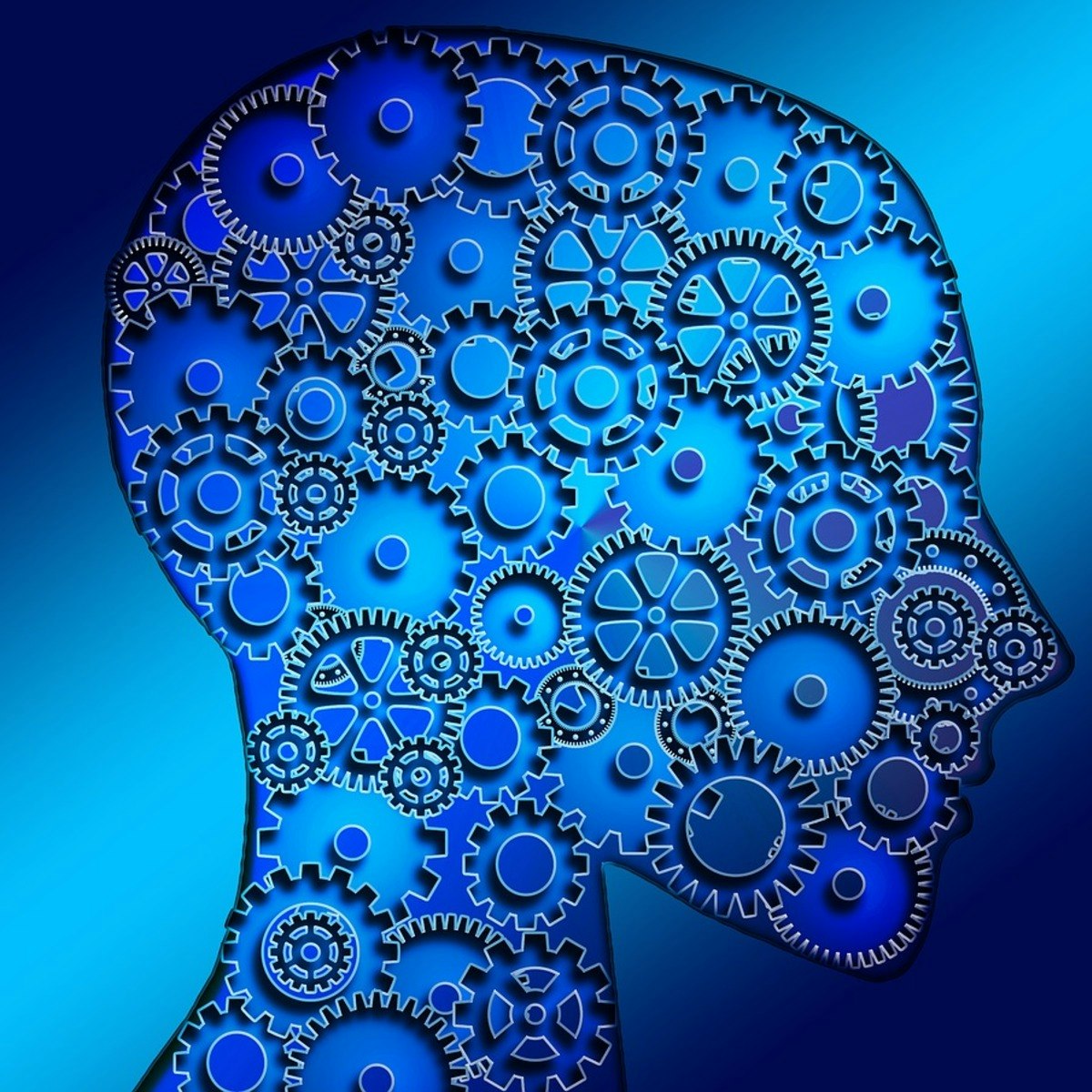
Data Science Courses - Page 101
Showing results 1001-1010 of 1407
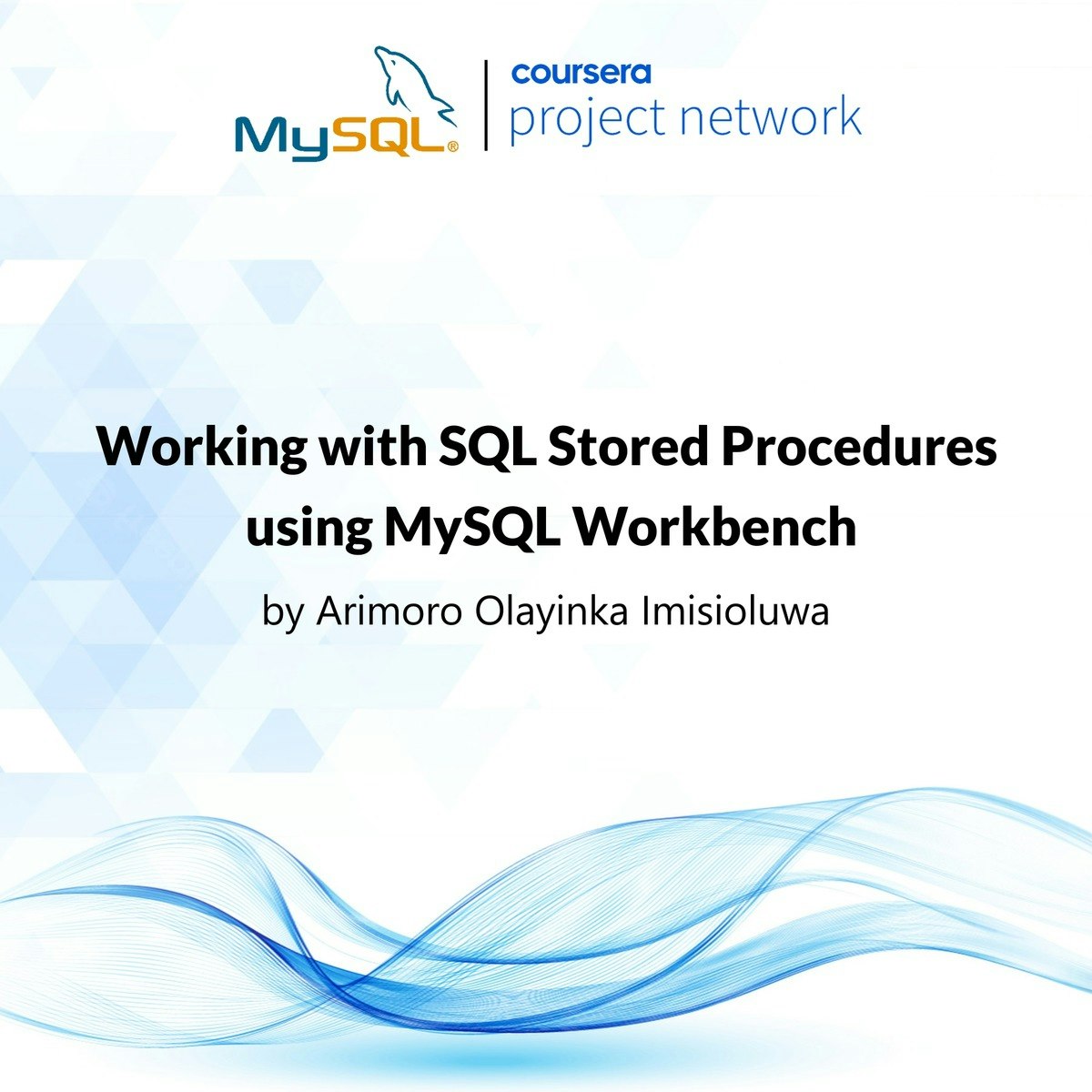
Working with SQL Stored Procedures using MySQL Workbench
Have you thought about creating a query that can be called several times to perform a routine task? Stored procedures offer this with a great advantage of efficiency. This project-based course, "Working with SQL Stored Procedures using MySQL Workbench" is intended for intermediate SQL users with some related experiences with SQL and who are willing to advance their knowledge and skills.
In this 2-hour project-based course, you will learn how to create stored procedures for different tasks including stored procedures with one input parameter, multiple input parameters, and an output parameter(s). This course is structured in a systematic way and very practical, where you get an option to practice as you progress.
This project-based course is an intermediate-level course in SQL. Therefore, to get the most out of this project, it is essential to understand using SQL. Specifically, you should be able to write SQL JOIN statements and work with aggregate functions. If you are not familiar with these concepts, it will be helpful to complete my previous project titled "Performing Data Aggregation using SQL Aggregate Functions" and “Mastering SQL Joins.”
However, if you are comfortable with these SQL concepts, please join me on this wonderful ride! Let’s get our hands dirty!
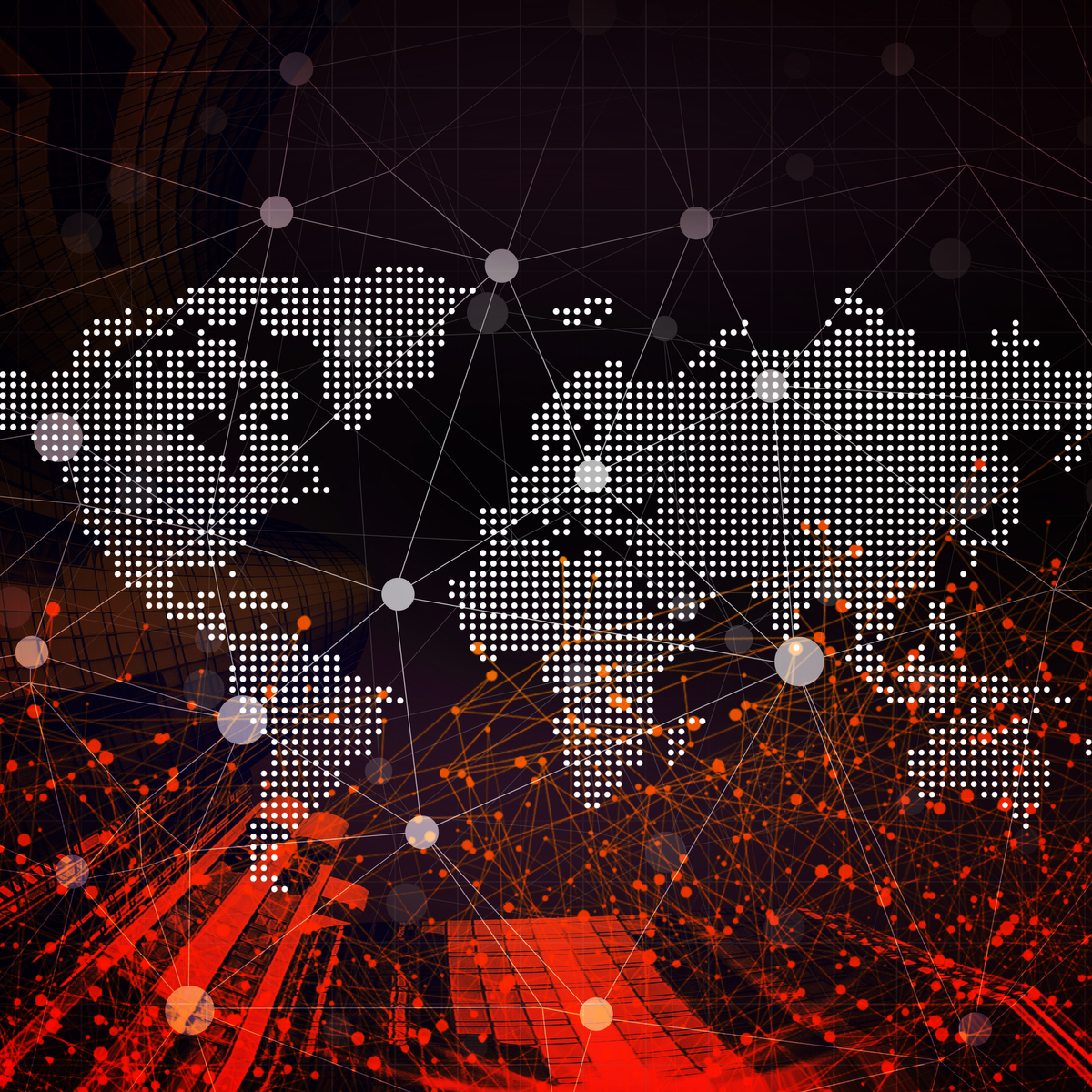
Geospatial Big Data Visualization with Kepler GL
In this 1-hour long project-based course, you will learn how to easily create beautiful data visualization with Kepler and effectively design different geospatial data visualizations.
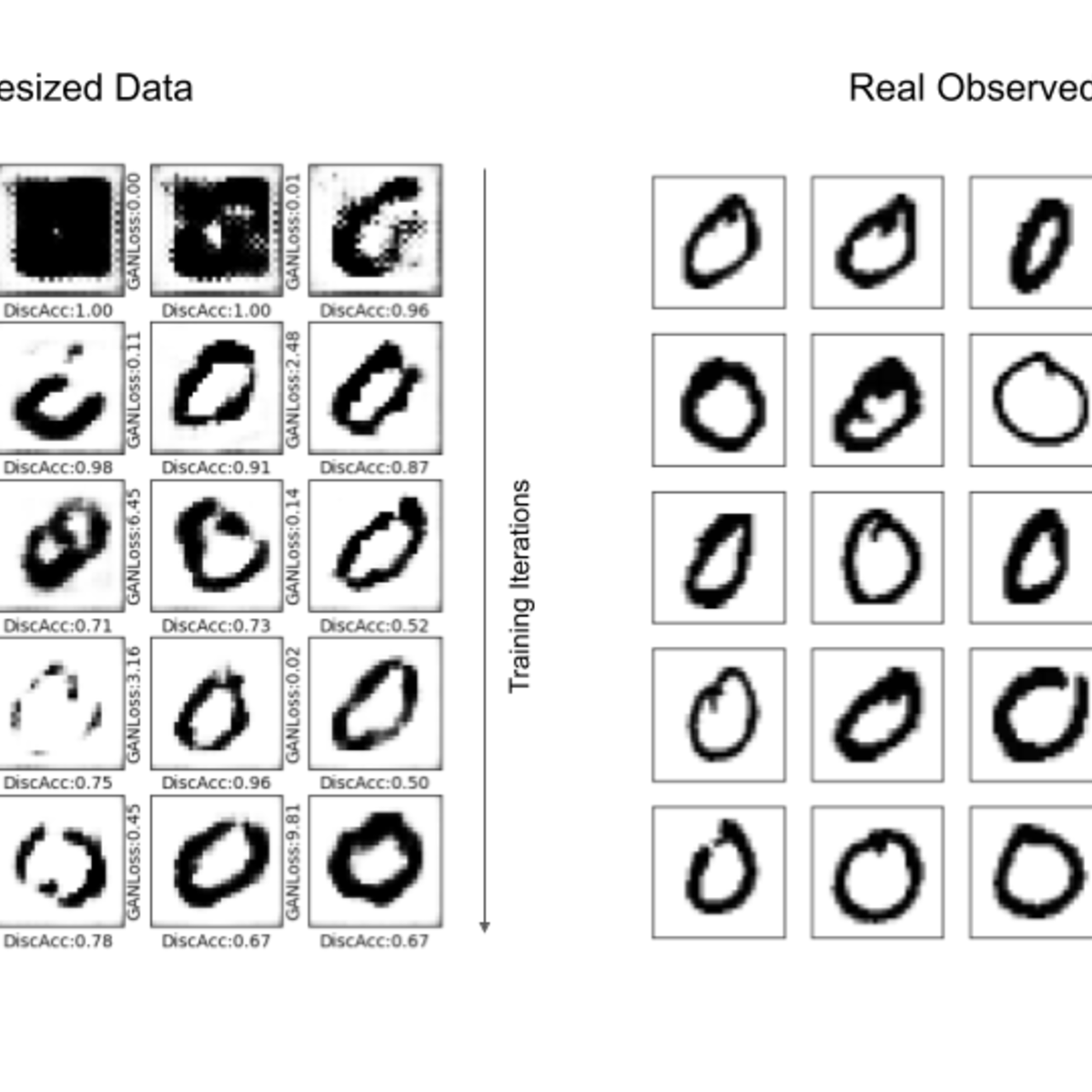
Understanding Deepfakes with Keras
In this 2-hour long project-based course, you will learn to implement DCGAN or Deep Convolutional Generative Adversarial Network, and you will train the network to generate realistic looking synthesized images. The term Deepfake is typically associated with synthetic data generated by Neural Networks which is similar to real-world, observed data - often with synthesized images, videos or audio. Through this hands-on project, we will go through the details of how such a network is structured, trained, and will ultimately generate synthetic images similar to hand-written digit 0 from the MNIST dataset.
Since this is a practical, project-based course, you will need to have a theoretical understanding of Neural Networks, Convolutional Neural Networks, and optimization algorithms like Gradient Descent. We will focus on the practical aspect of implementing and training DCGAN, but not too much on the theoretical aspect. You will also need some prior experience with Python programming.
This course runs on Coursera's hands-on project platform called Rhyme. On Rhyme, you do projects in a hands-on manner in your browser. You will get instant access to pre-configured cloud desktops containing all of the software and data you need for the project. Everything is already set up directly in your internet browser so you can just focus on learning. For this project, you’ll get instant access to a cloud desktop with Python, Jupyter, and Tensorflow pre-installed.
Notes:
- You will be able to access the cloud desktop 5 times. However, you will be able to access instructions videos as many times as you want.
- This course works best for learners who are based in the North America region. We’re currently working on providing the same experience in other regions.
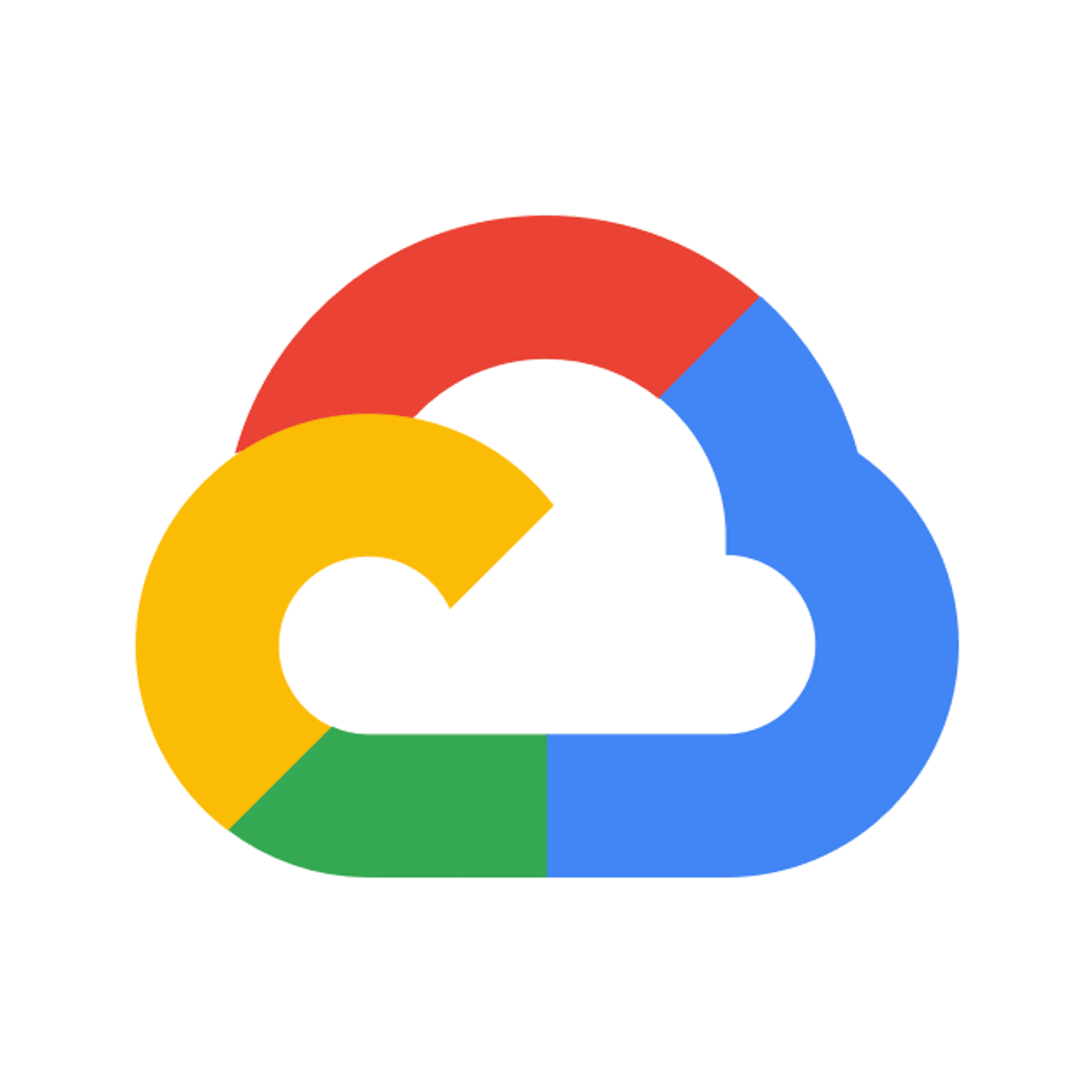
Datastream MySQL to BigQuery
This is a self-paced lab that takes place in the Google Cloud console. Learn to migrate MySQL Databases to BigQuery using Datastream and Dataflow.
Datastream is a serverless and easy-to-use Change Data Capture (CDC) and replication service that allows you to synchronize data across heterogeneous databases, storage systems, and applications reliably and with minimal latency. In this lab you'll learn how to replicate data from your OLTP workloads into BigQuery, in real time.
You will begin by deploying MySQL on Cloud SQL and import a dataset using the gcloud command line. Then, in the Cloud Console UI, you will create and start a Datastream stream and a Dataflow job for replication. The replication uses a Dataflow template to enable continuous replication of data, along with Cloud Storage and Pub/Sub for buffering data.
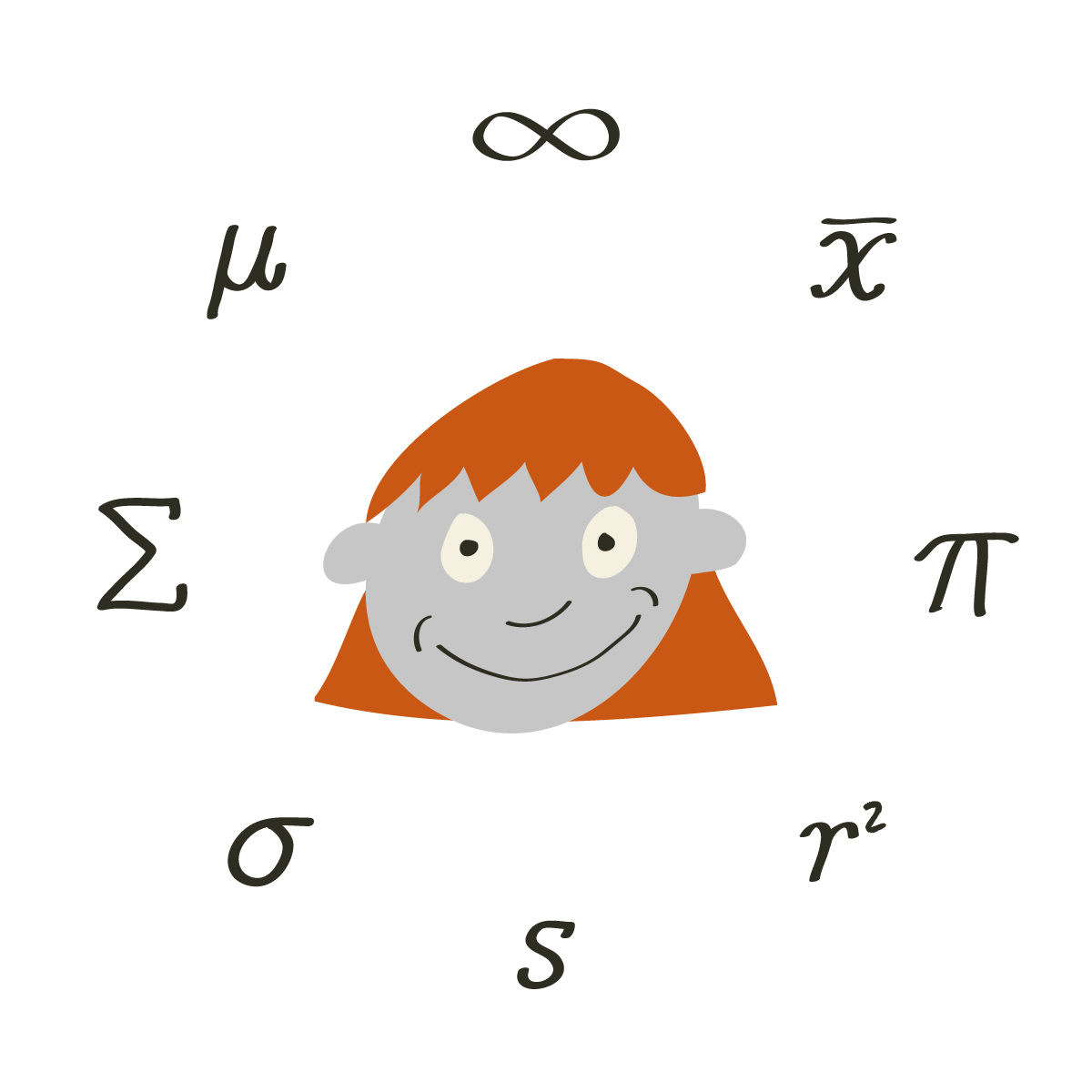
Basic Statistics
Understanding statistics is essential to understand research in the social and behavioral sciences. In this course you will learn the basics of statistics; not just how to calculate them, but also how to evaluate them. This course will also prepare you for the next course in the specialization - the course Inferential Statistics.
In the first part of the course we will discuss methods of descriptive statistics. You will learn what cases and variables are and how you can compute measures of central tendency (mean, median and mode) and dispersion (standard deviation and variance). Next, we discuss how to assess relationships between variables, and we introduce the concepts correlation and regression.
The second part of the course is concerned with the basics of probability: calculating probabilities, probability distributions and sampling distributions. You need to know about these things in order to understand how inferential statistics work.
The third part of the course consists of an introduction to methods of inferential statistics - methods that help us decide whether the patterns we see in our data are strong enough to draw conclusions about the underlying population we are interested in. We will discuss confidence intervals and significance tests.
You will not only learn about all these statistical concepts, you will also be trained to calculate and generate these statistics yourself using freely available statistical software.
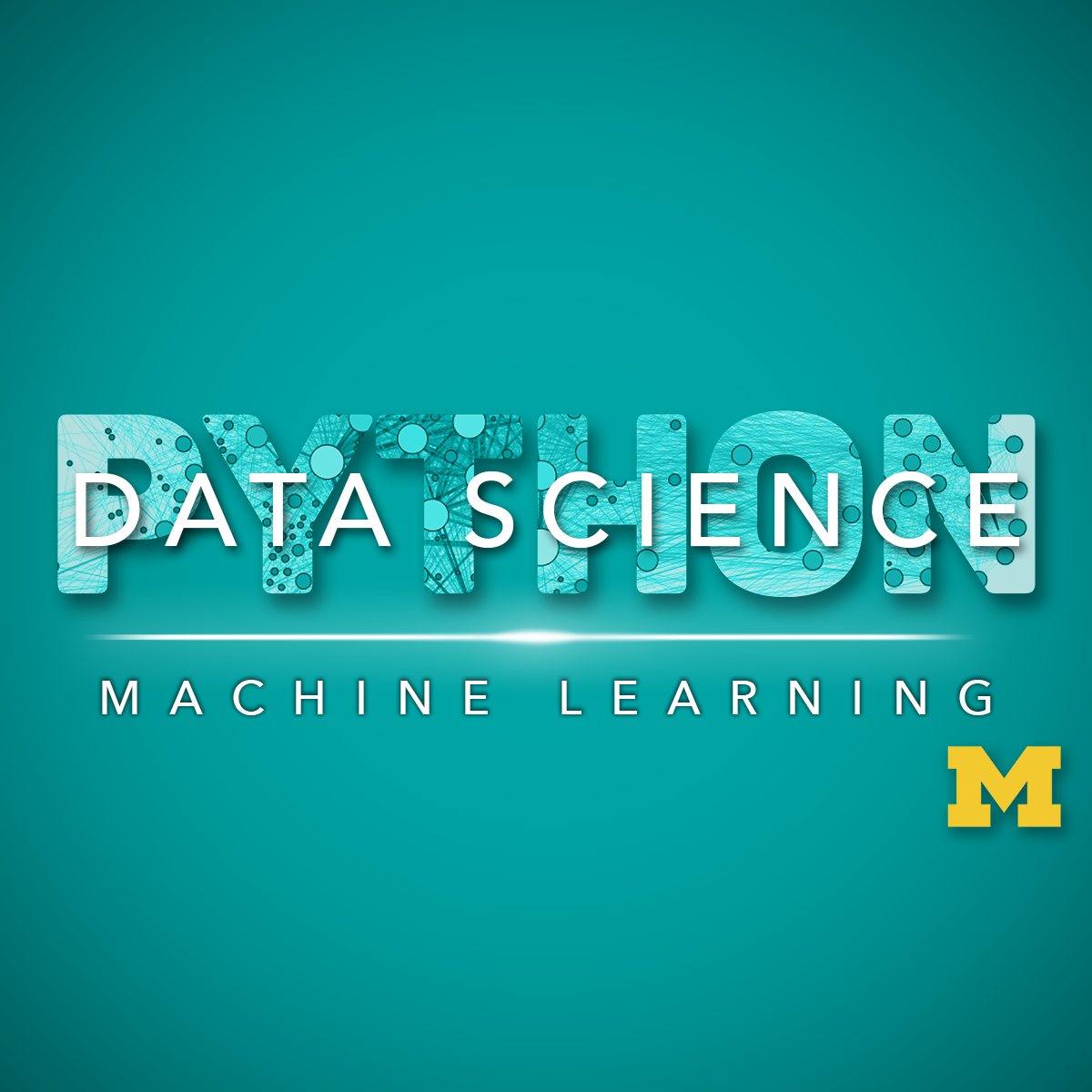
Applied Machine Learning in Python
This course will introduce the learner to applied machine learning, focusing more on the techniques and methods than on the statistics behind these methods. The course will start with a discussion of how machine learning is different than descriptive statistics, and introduce the scikit learn toolkit through a tutorial. The issue of dimensionality of data will be discussed, and the task of clustering data, as well as evaluating those clusters, will be tackled. Supervised approaches for creating predictive models will be described, and learners will be able to apply the scikit learn predictive modelling methods while understanding process issues related to data generalizability (e.g. cross validation, overfitting). The course will end with a look at more advanced techniques, such as building ensembles, and practical limitations of predictive models. By the end of this course, students will be able to identify the difference between a supervised (classification) and unsupervised (clustering) technique, identify which technique they need to apply for a particular dataset and need, engineer features to meet that need, and write python code to carry out an analysis.
This course should be taken after Introduction to Data Science in Python and Applied Plotting, Charting & Data Representation in Python and before Applied Text Mining in Python and Applied Social Analysis in Python.
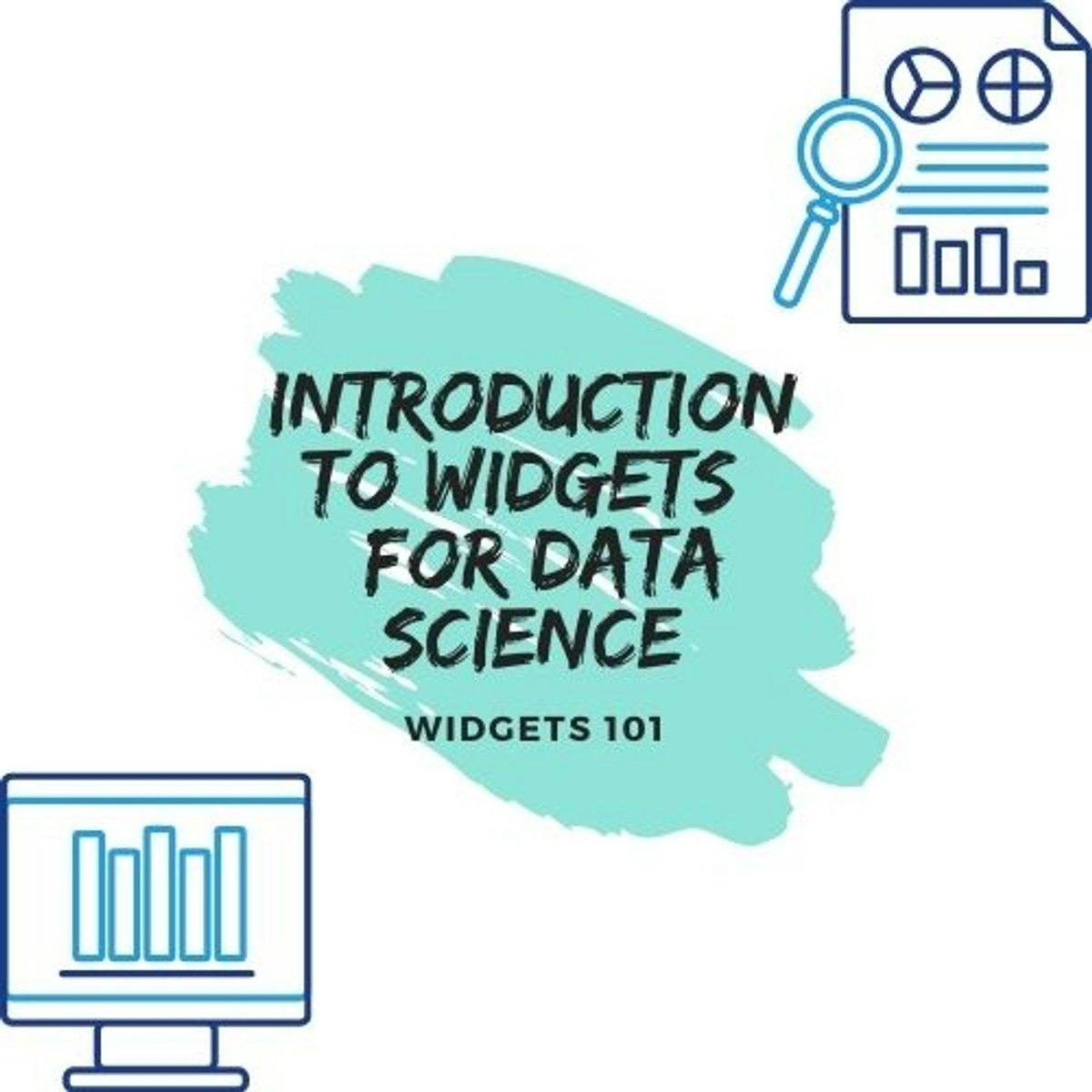
Introduction to Widgets for Data Science
In this 2-hour long project-based course, you will learn what are widgets, how they can used for data science work, types of widgets, linking multiple widgets, basic dashboards of widgets and creating child widgets.
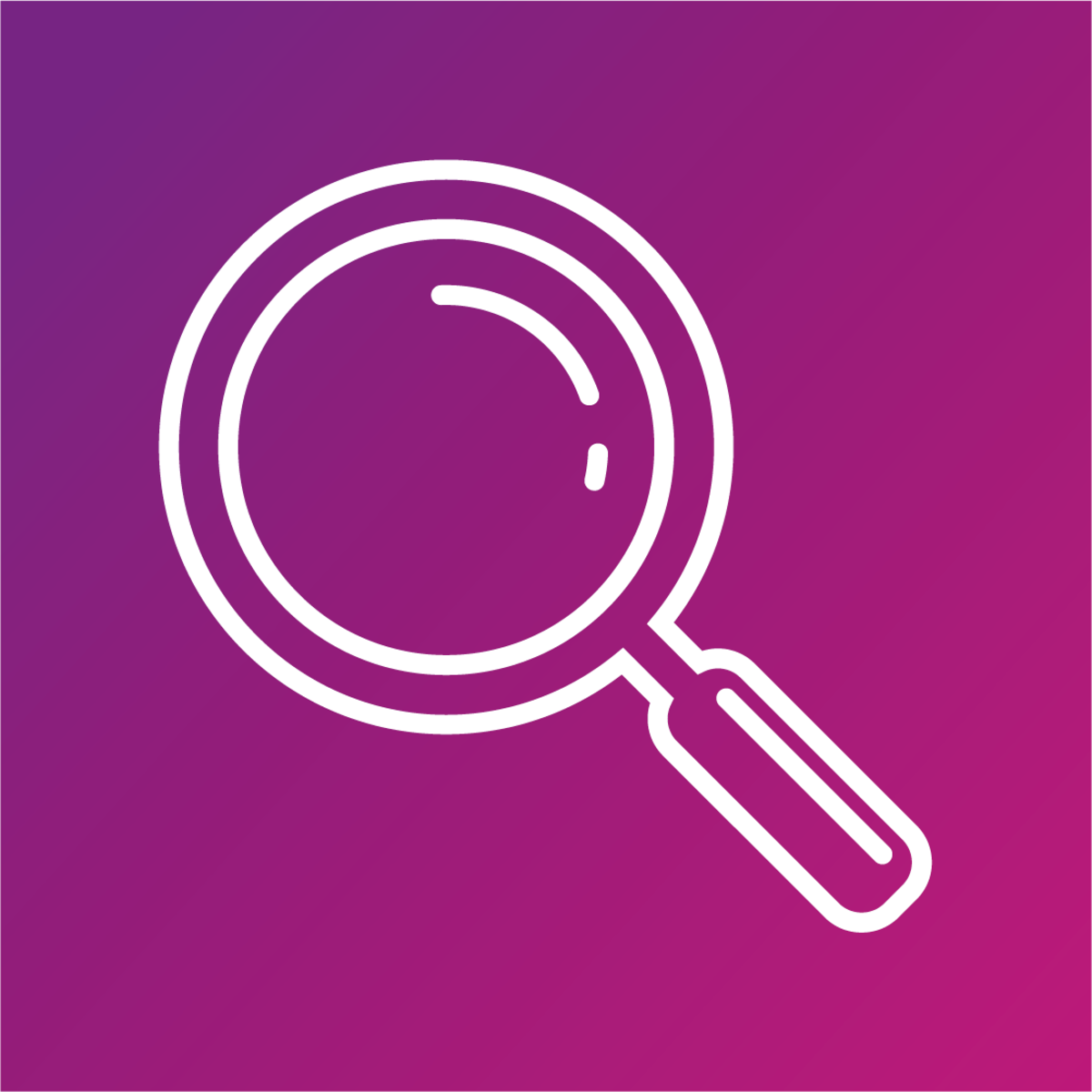
Validity and Bias in Epidemiology
Epidemiological studies can provide valuable insights about the frequency of a disease, its potential causes and the effectiveness of available treatments. Selecting an appropriate study design can take you a long way when trying to answer such a question. However, this is by no means enough. A study can yield biased results for many different reasons. This course offers an introduction to some of these factors and provides guidance on how to deal with bias in epidemiological research. In this course you will learn about the main types of bias and what effect they might have on your study findings. You will then focus on the concept of confounding and you will explore various methods to identify and control for confounding in different study designs. In the last module of this course we will discuss the phenomenon of effect modification, which is key to understanding and interpreting study results. We will finish the course with a broader discussion of causality in epidemiology and we will highlight how you can utilise all the tools that you have learnt to decide whether your findings indicate a true association and if this can be considered causal.
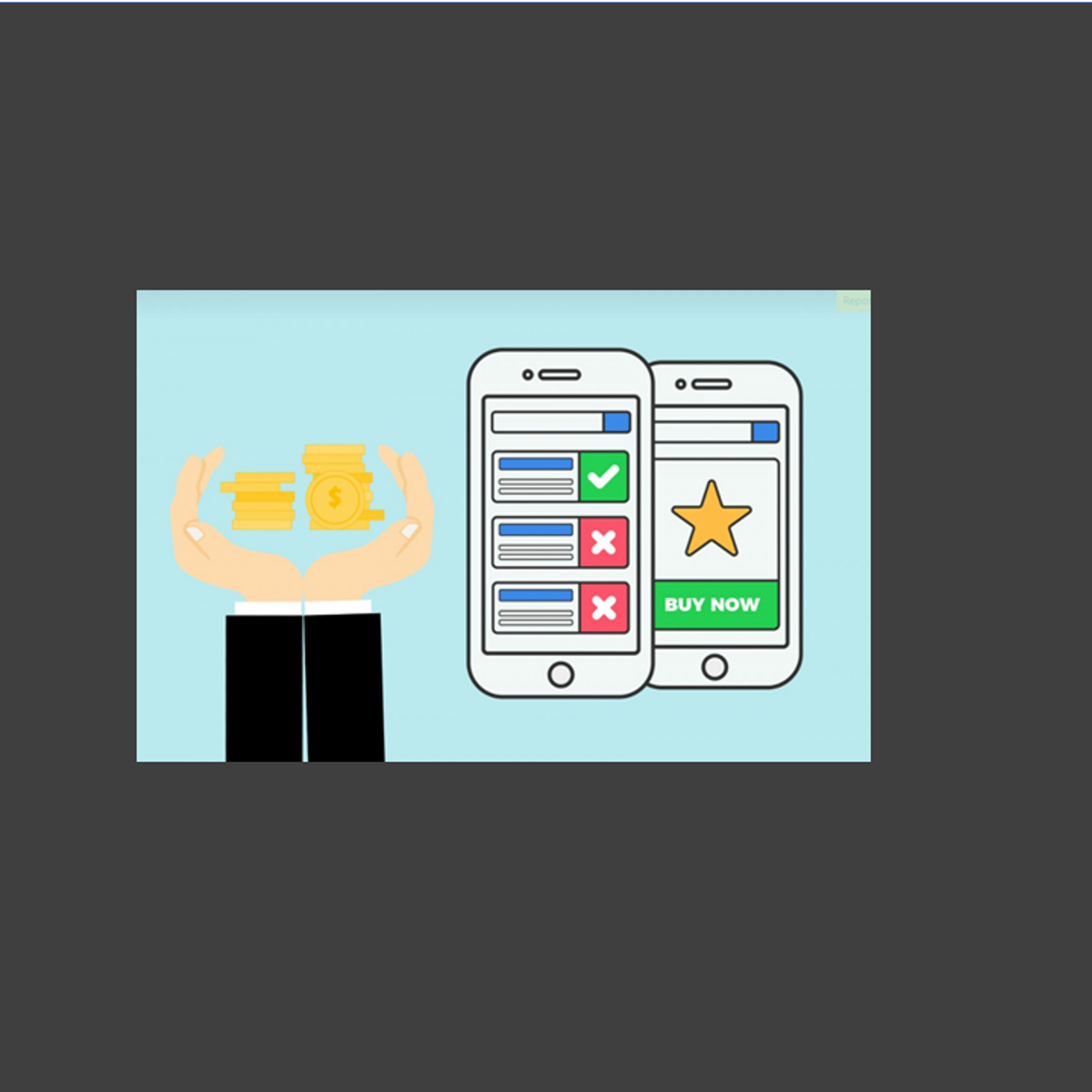
Predict Ad Clicks Using Logistic Regression and XG-Boost
In this project, we will predict Ads clicks using logistic regression and XG-boost algorithms. In this project, we will assume that you have been hired as a consultant to a start-up that is running a targeted marketing ad campaign on Facebook. The company wants to analyze customer behavior by predicting which customer clicks on the advertisement.
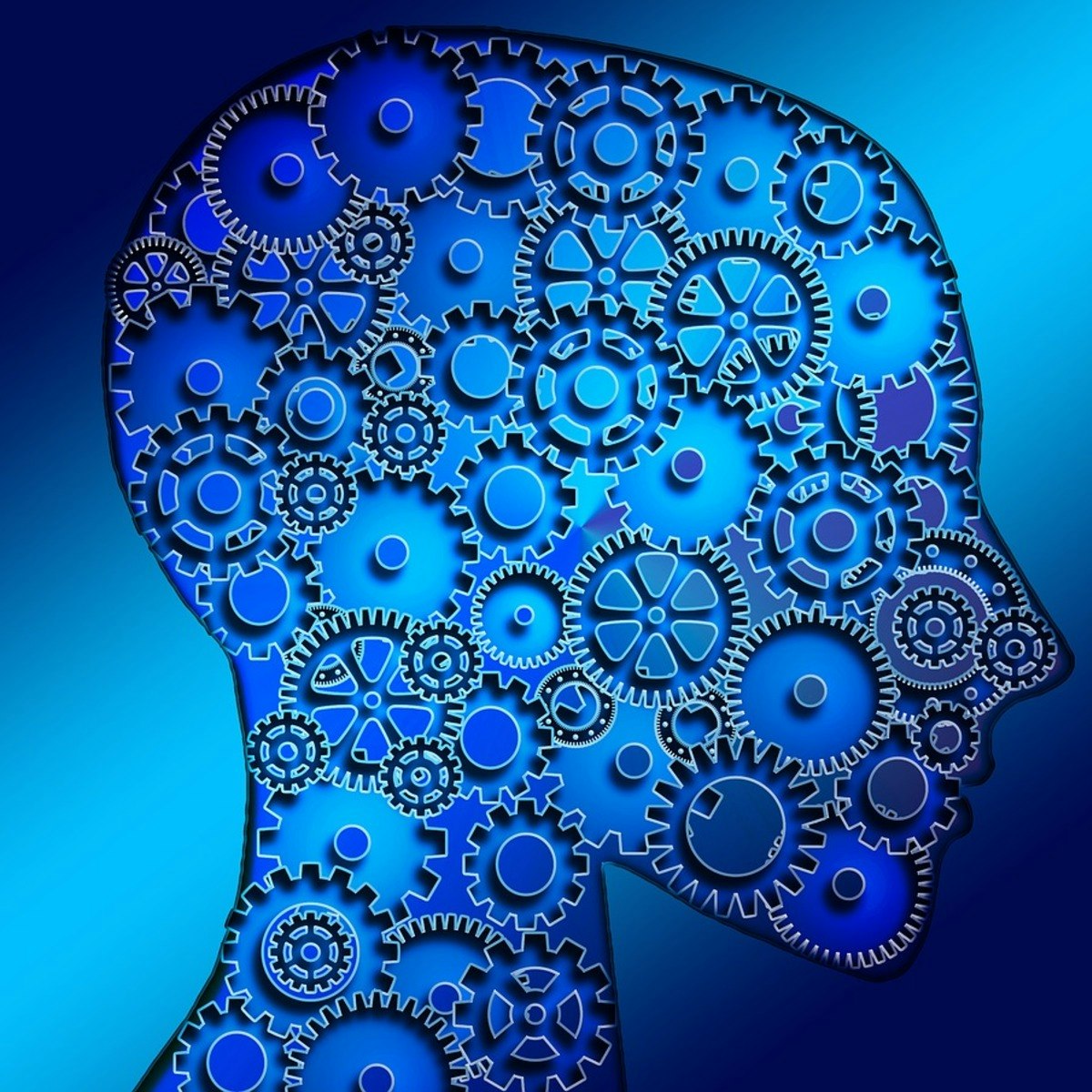
Machine Learning: Clustering & Retrieval
Case Studies: Finding Similar Documents
A reader is interested in a specific news article and you want to find similar articles to recommend. What is the right notion of similarity? Moreover, what if there are millions of other documents? Each time you want to a retrieve a new document, do you need to search through all other documents? How do you group similar documents together? How do you discover new, emerging topics that the documents cover?
In this third case study, finding similar documents, you will examine similarity-based algorithms for retrieval. In this course, you will also examine structured representations for describing the documents in the corpus, including clustering and mixed membership models, such as latent Dirichlet allocation (LDA). You will implement expectation maximization (EM) to learn the document clusterings, and see how to scale the methods using MapReduce.
Learning Outcomes: By the end of this course, you will be able to:
-Create a document retrieval system using k-nearest neighbors.
-Identify various similarity metrics for text data.
-Reduce computations in k-nearest neighbor search by using KD-trees.
-Produce approximate nearest neighbors using locality sensitive hashing.
-Compare and contrast supervised and unsupervised learning tasks.
-Cluster documents by topic using k-means.
-Describe how to parallelize k-means using MapReduce.
-Examine probabilistic clustering approaches using mixtures models.
-Fit a mixture of Gaussian model using expectation maximization (EM).
-Perform mixed membership modeling using latent Dirichlet allocation (LDA).
-Describe the steps of a Gibbs sampler and how to use its output to draw inferences.
-Compare and contrast initialization techniques for non-convex optimization objectives.
-Implement these techniques in Python.
