Back to Courses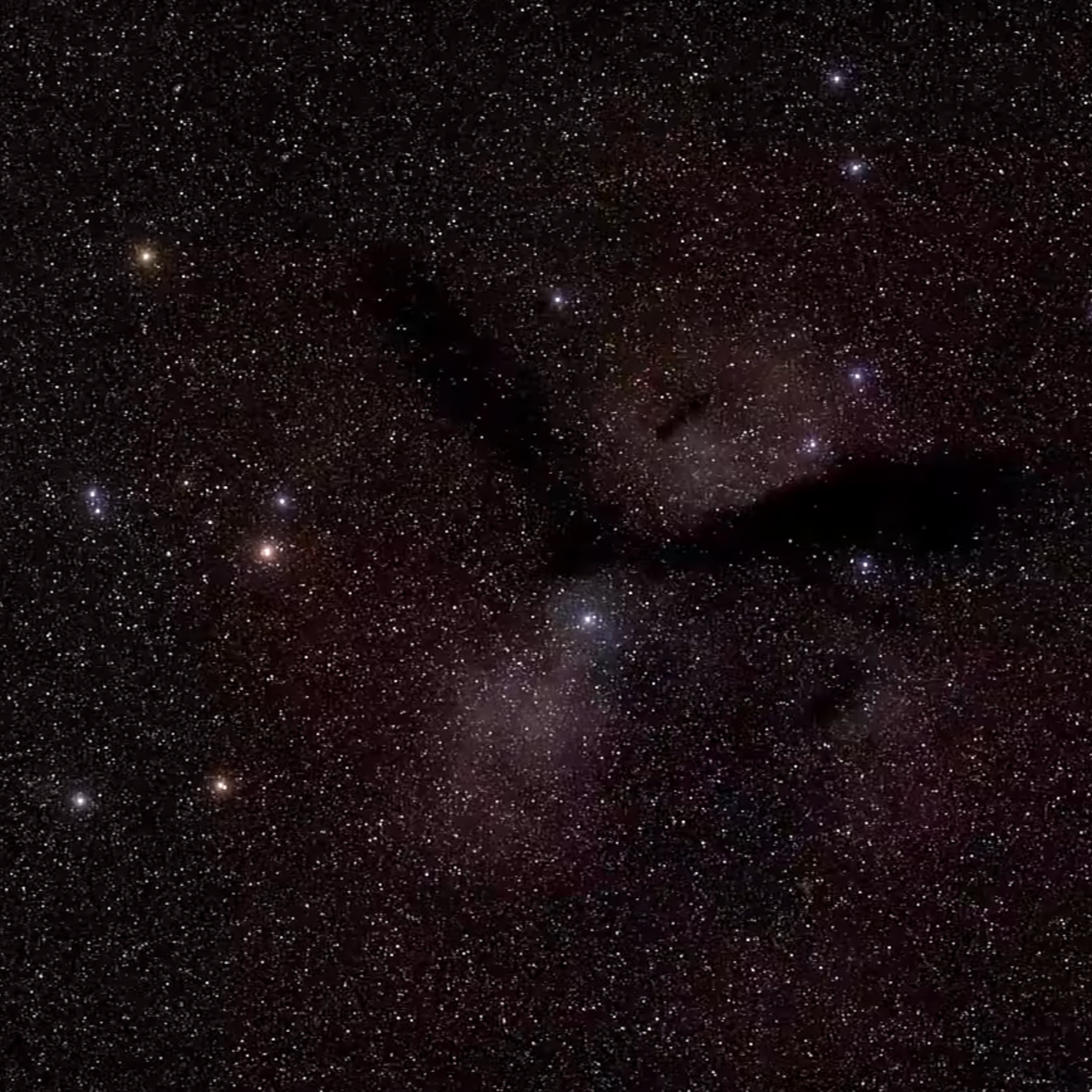
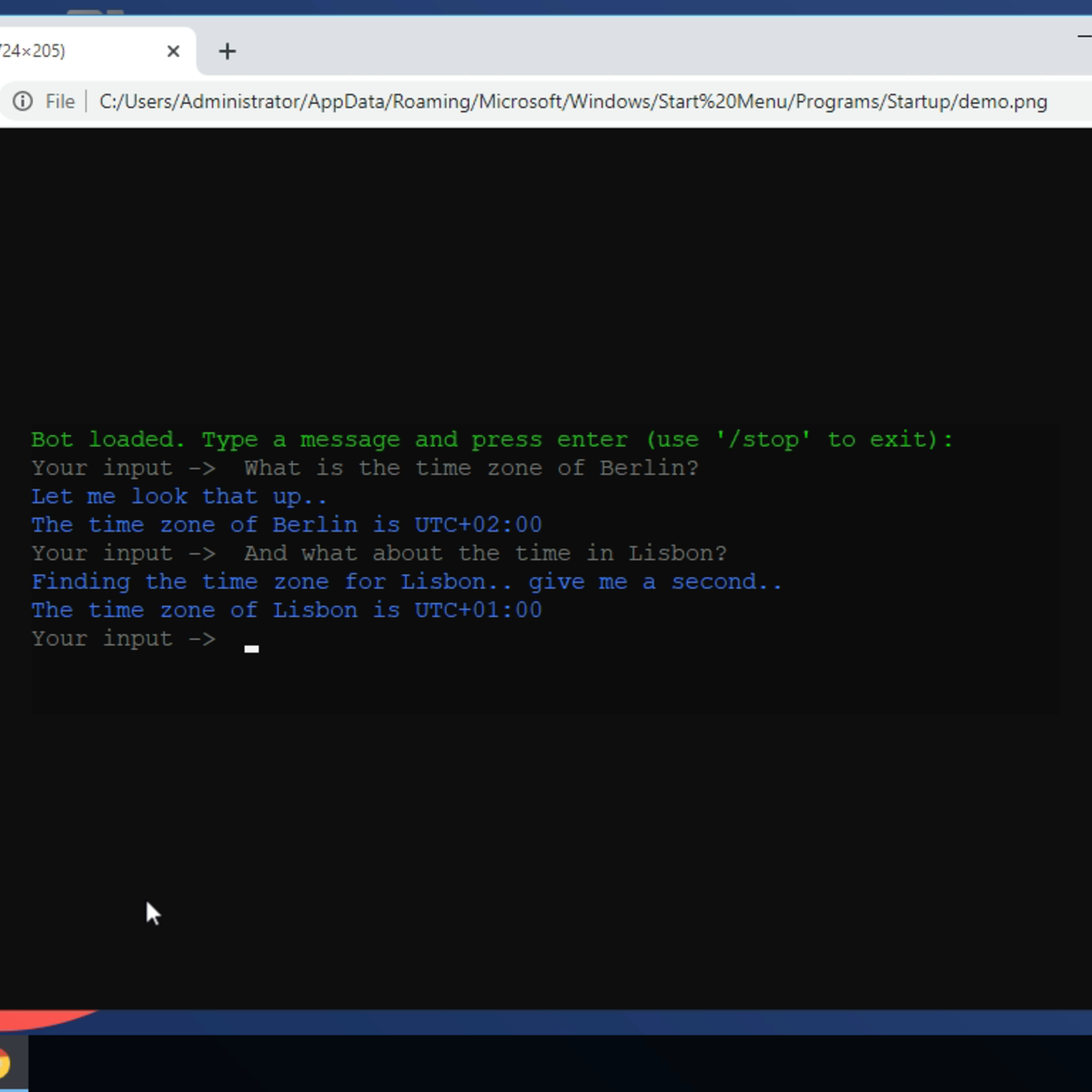
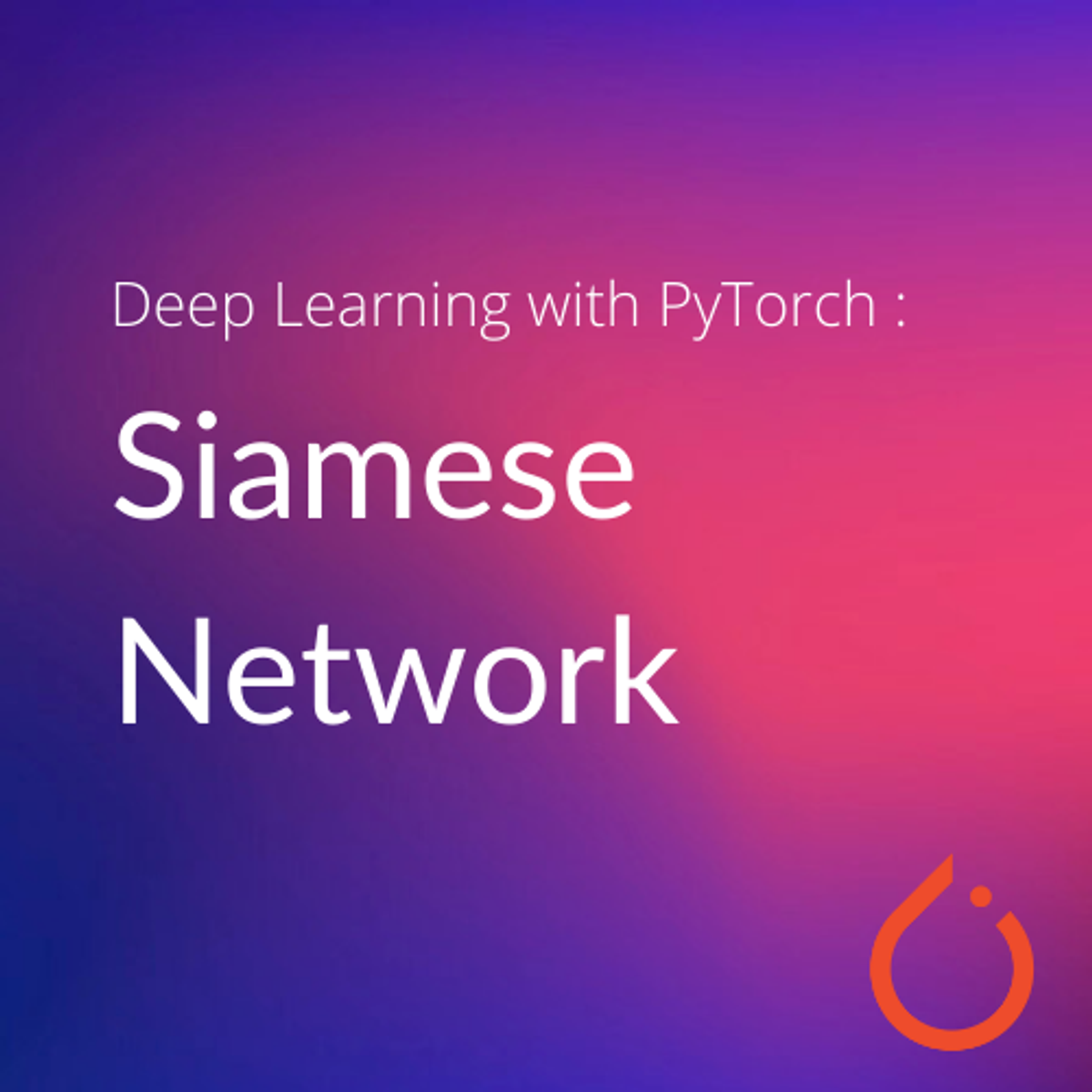
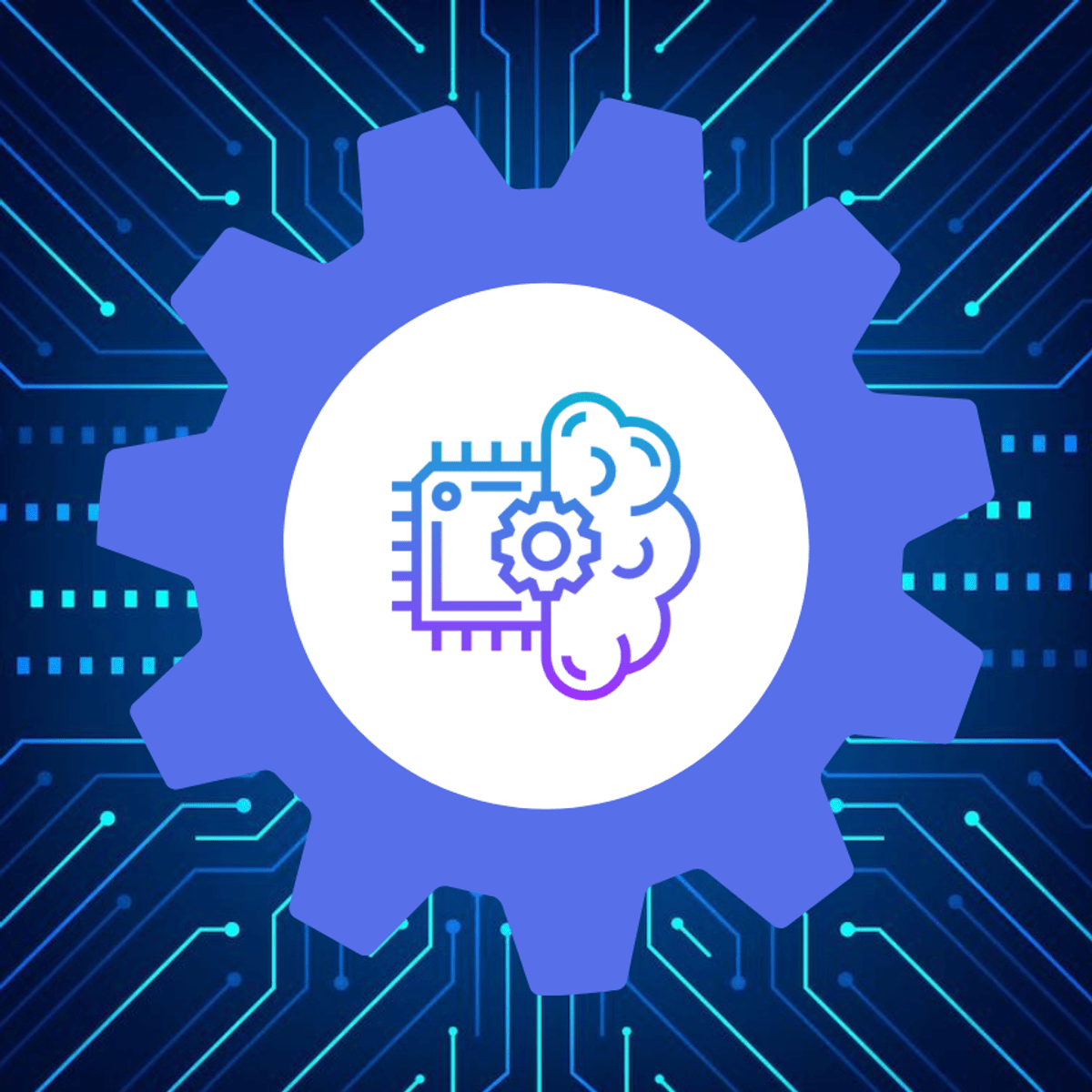
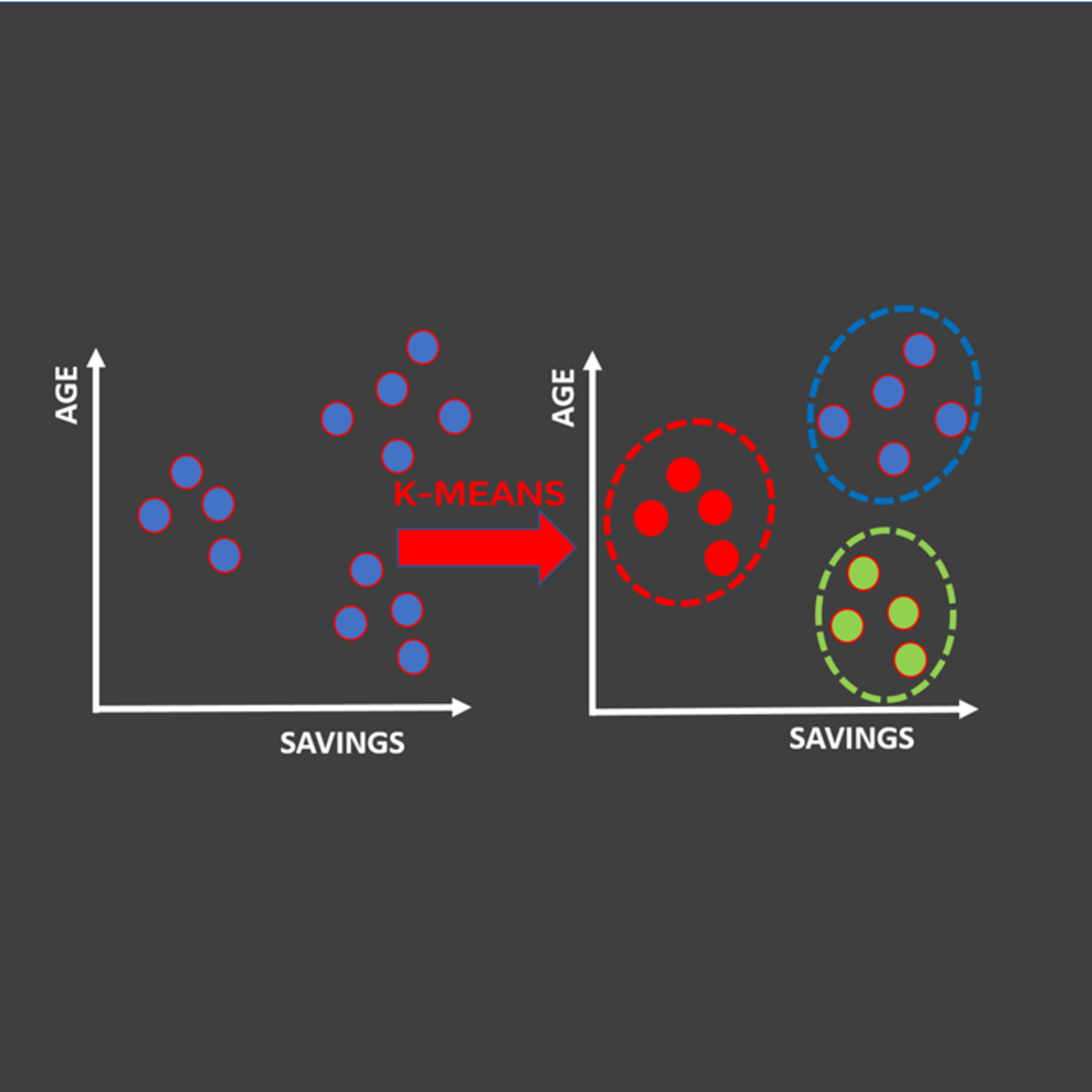
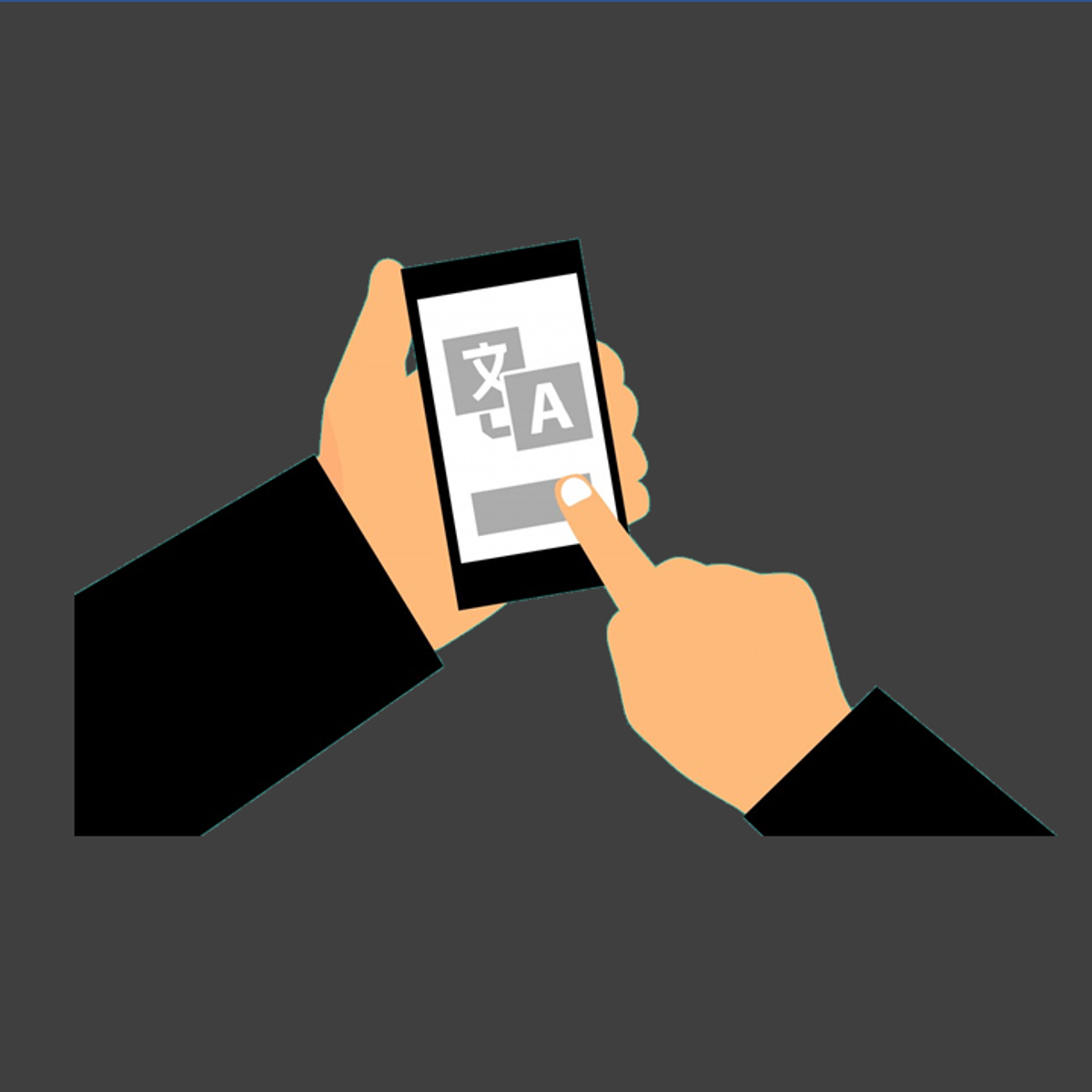
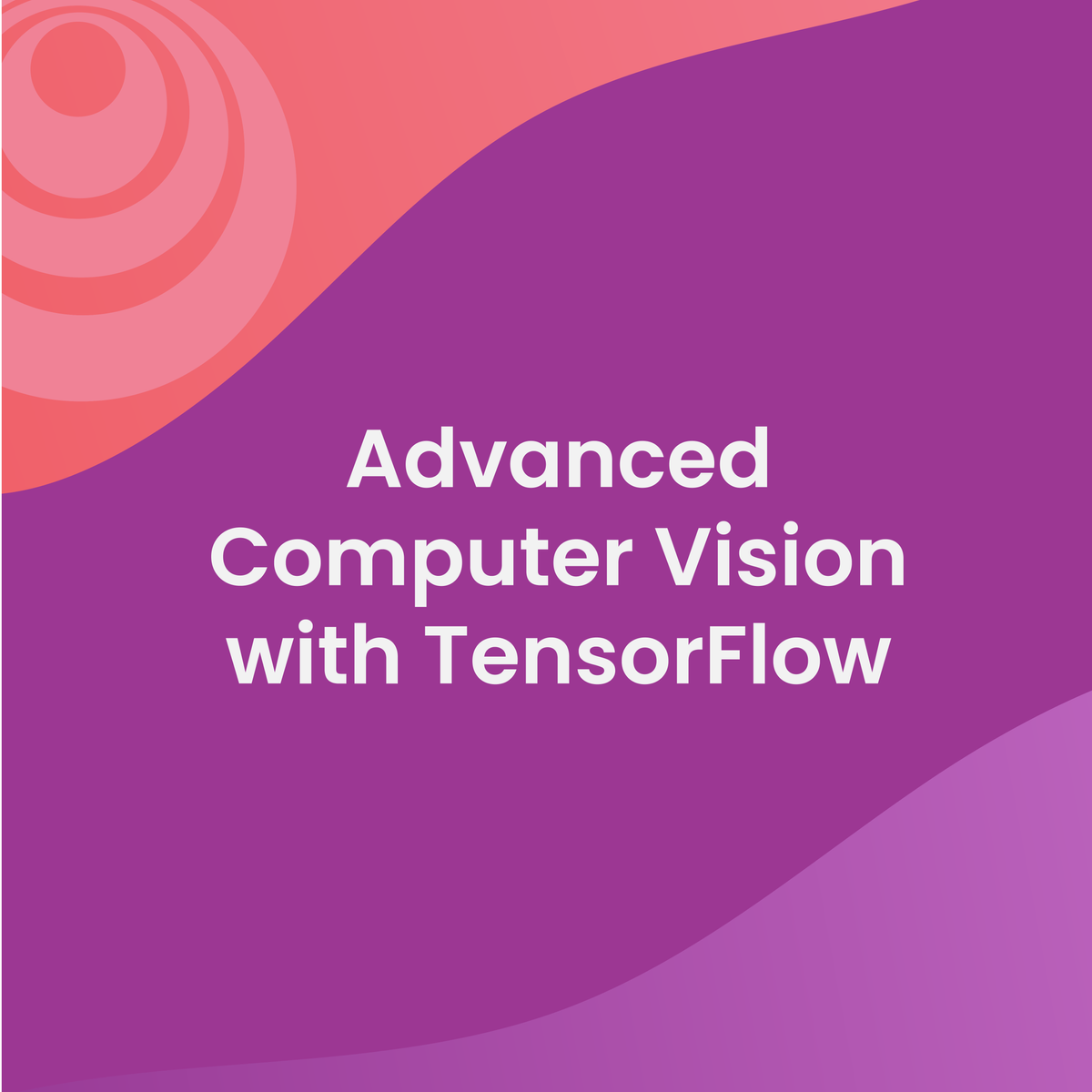
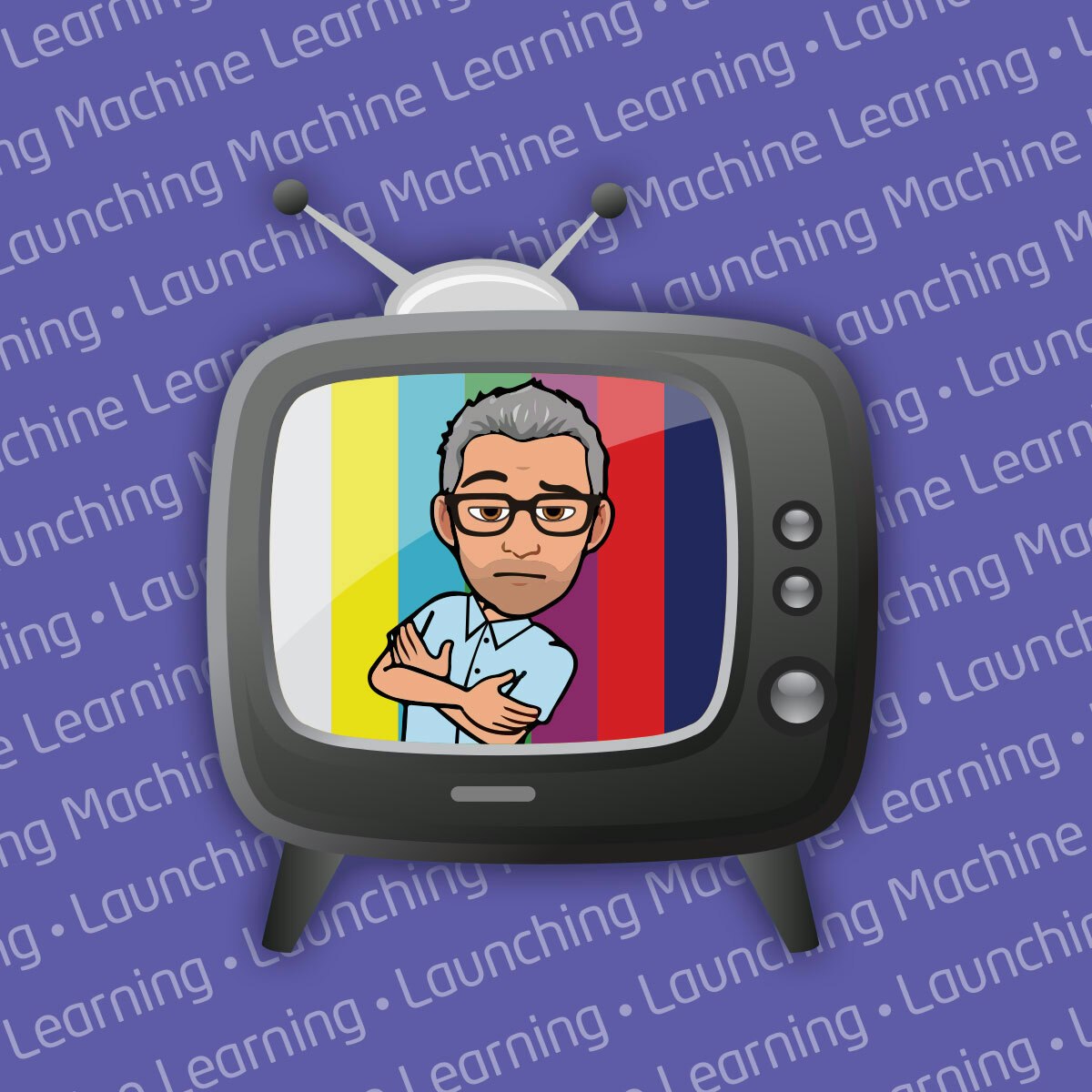
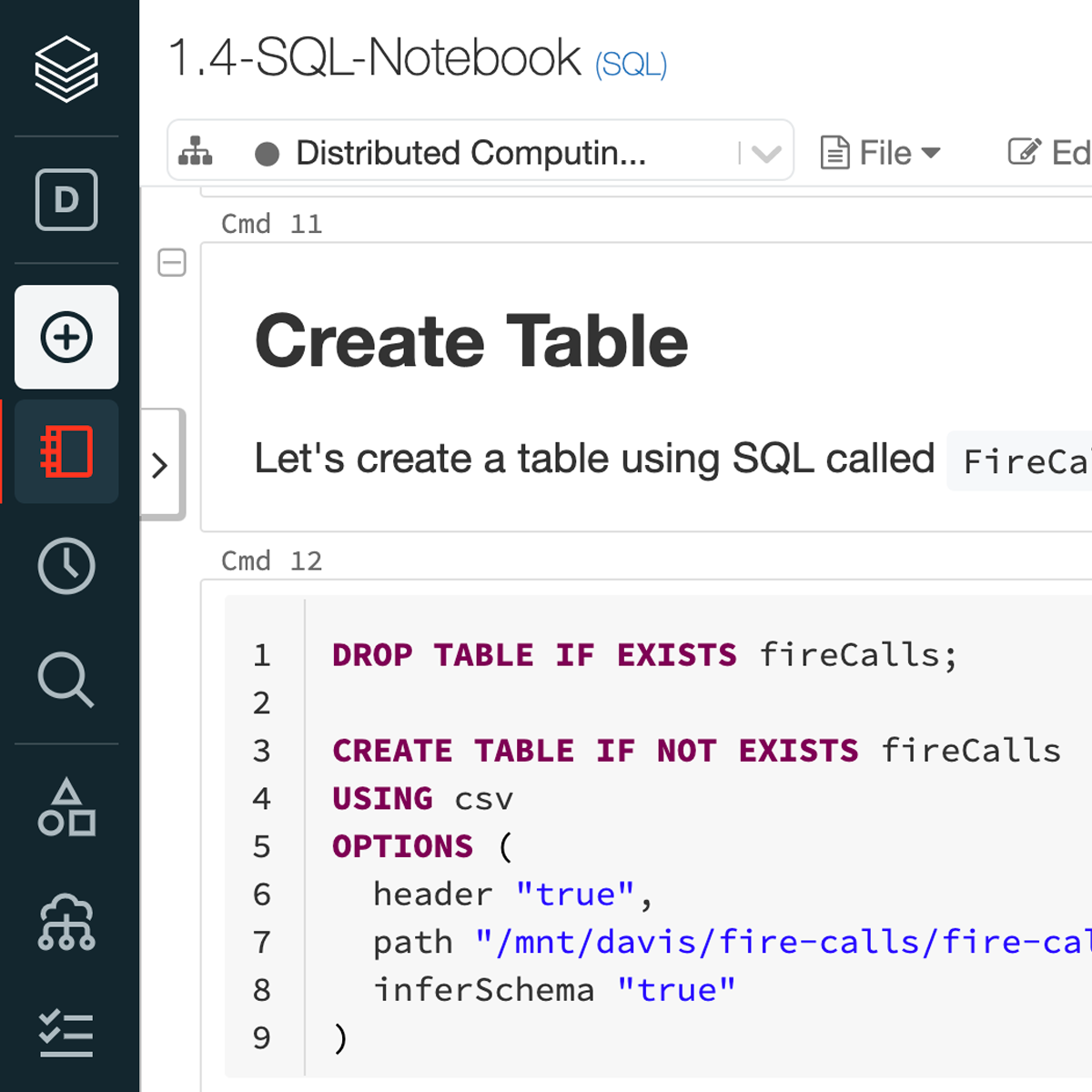
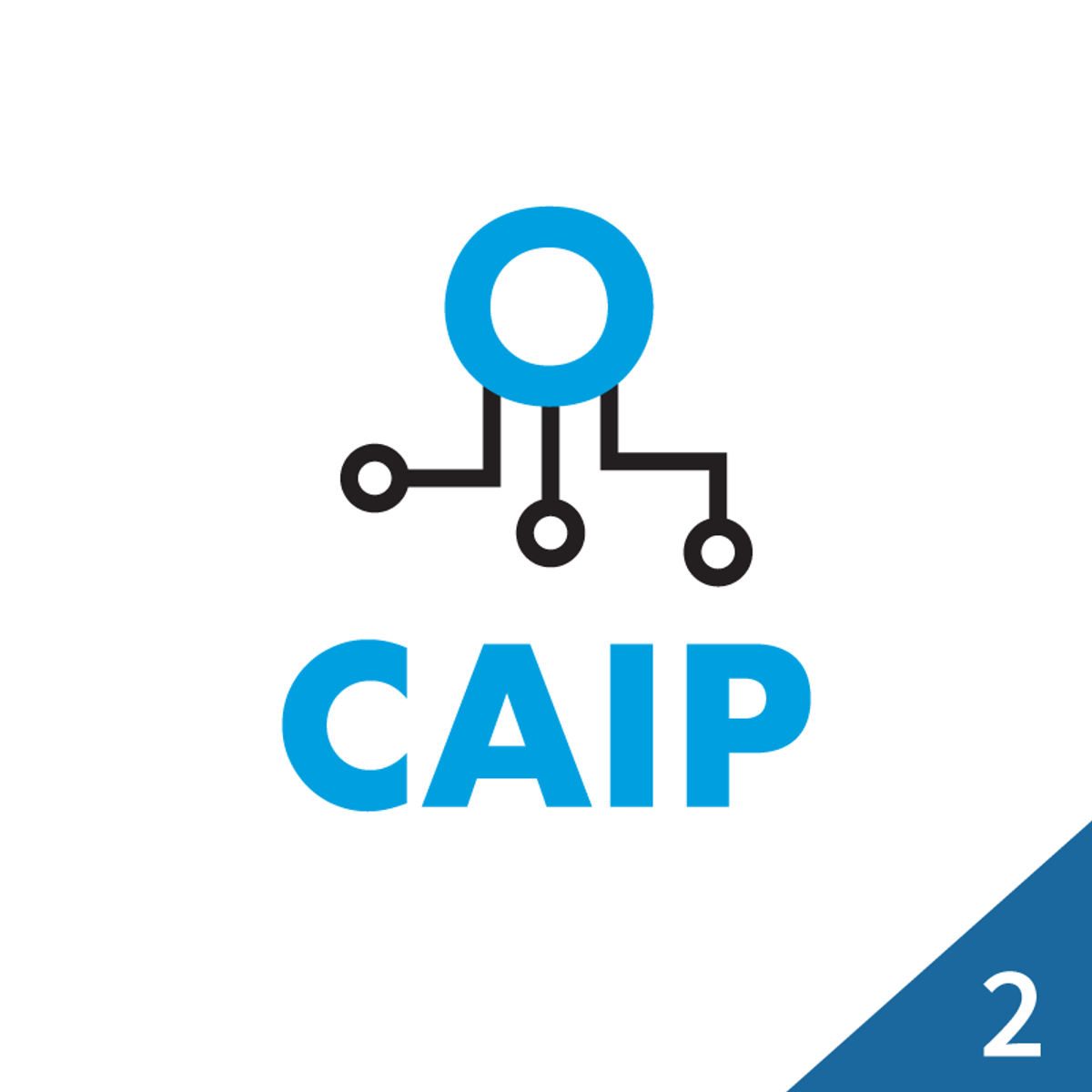
Machine Learning Courses - Page 12
Showing results 111-120 of 485
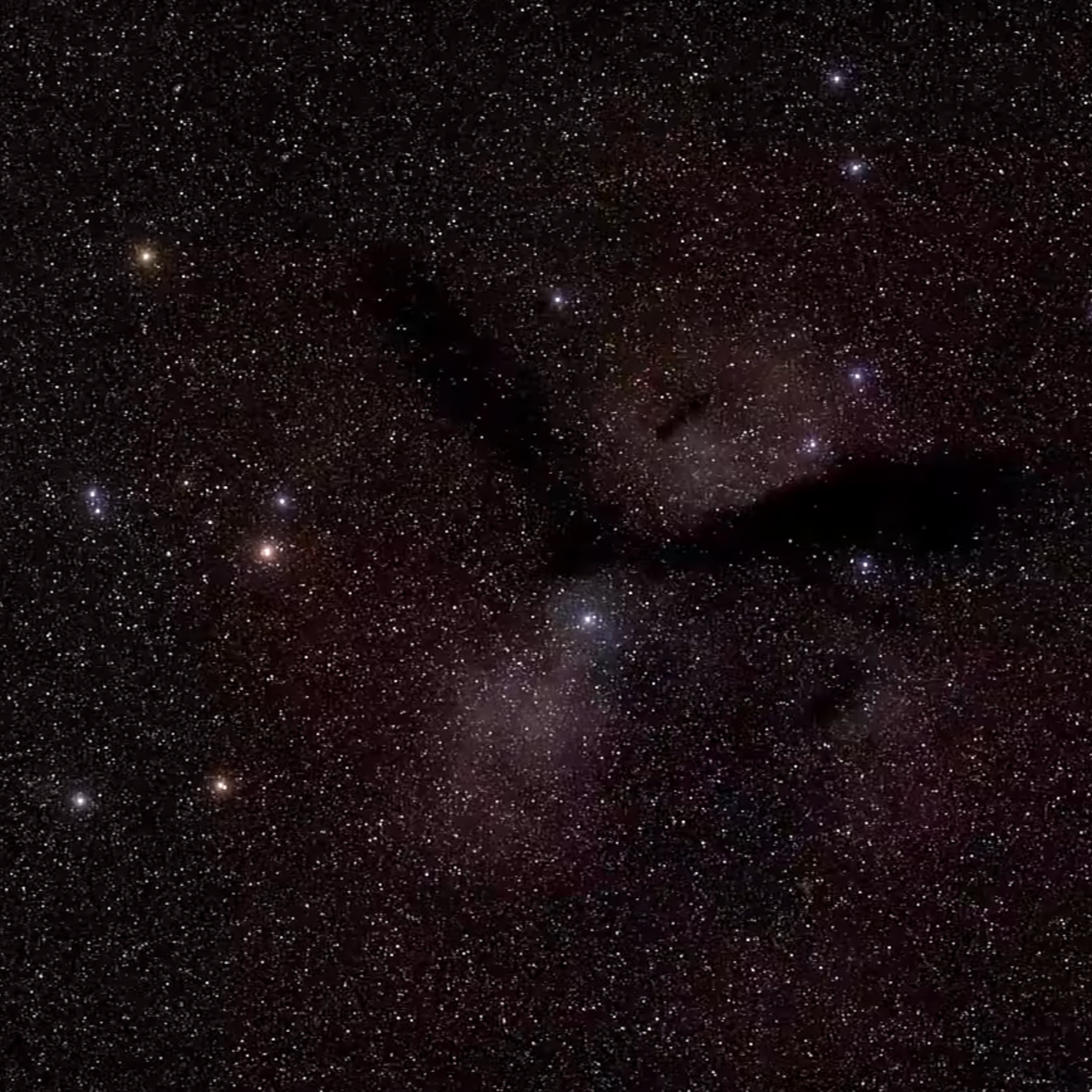
Classify Radio Signals from Space using Keras
In this 1-hour long project-based course, you will learn the basics of using Keras with TensorFlow as its backend and use it to solve an image classification problem. The data we are going to use consists of 2D spectrograms of deep space radio signals collected by the Allen Telescope Array at the SETI Institute. We will treat the spectrograms as images to train an image classification model to classify the signals into one of four classes. By the end of the project, you will have built and trained a convolutional neural network from scratch using Keras to classify signals from space.
This course runs on Coursera's hands-on project platform called Rhyme. On Rhyme, you do projects in a hands-on manner in your browser. You will get instant access to pre-configured cloud desktops containing all of the software and data you need for the project. Everything is already set up directly in your internet browser so you can just focus on learning. For this project, you’ll get instant access to a cloud desktop with Python, Jupyter, and Tensorflow pre-installed.
Notes:
- You will be able to access the cloud desktop 5 times. However, you will be able to access instructions videos as many times as you want.
- This course works best for learners who are based in the North America region. We’re currently working on providing the same experience in other regions.
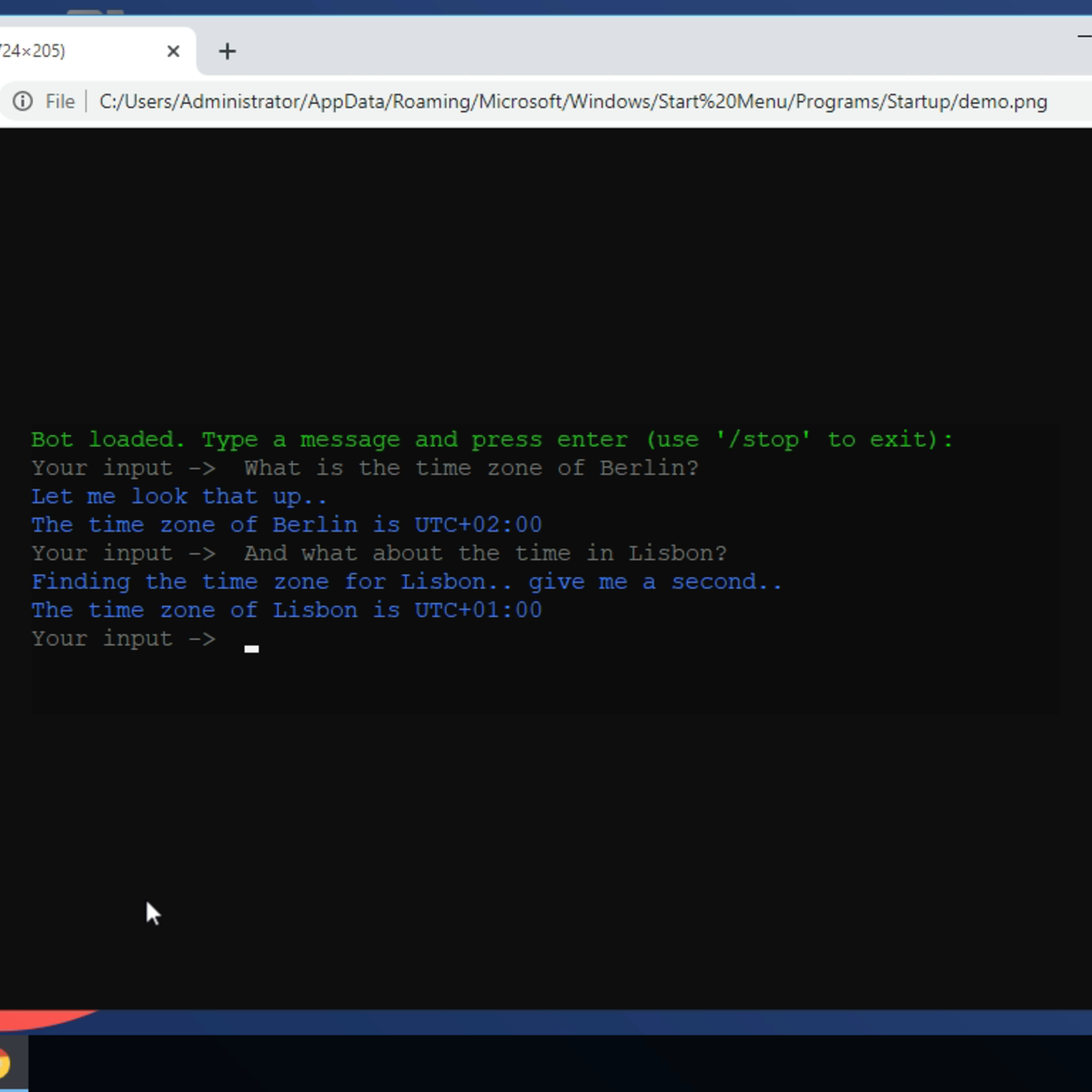
Create Your First Chatbot with Rasa and Python
In this 2 hour long project-based course, you will learn to create chatbots with Rasa and Python. Rasa is a framework for developing AI powered, industrial grade chatbots. It’s incredibly powerful, and is used by developers worldwide to create chatbots and contextual assistants. In this project, we are going to understand some of the most important basic aspects of the Rasa framework and chatbot development. Once you’re done with this project, you will be able to create simple AI powered chatbots on your own.
This project is ideal for programmers who want to get started with chatbot development. You don't need any machine learning or prior chatbot development experience. However, you should be familiar with Python programming.
Note: This course works best for learners who are based in the North America region. We’re currently working on providing the same experience in other regions.
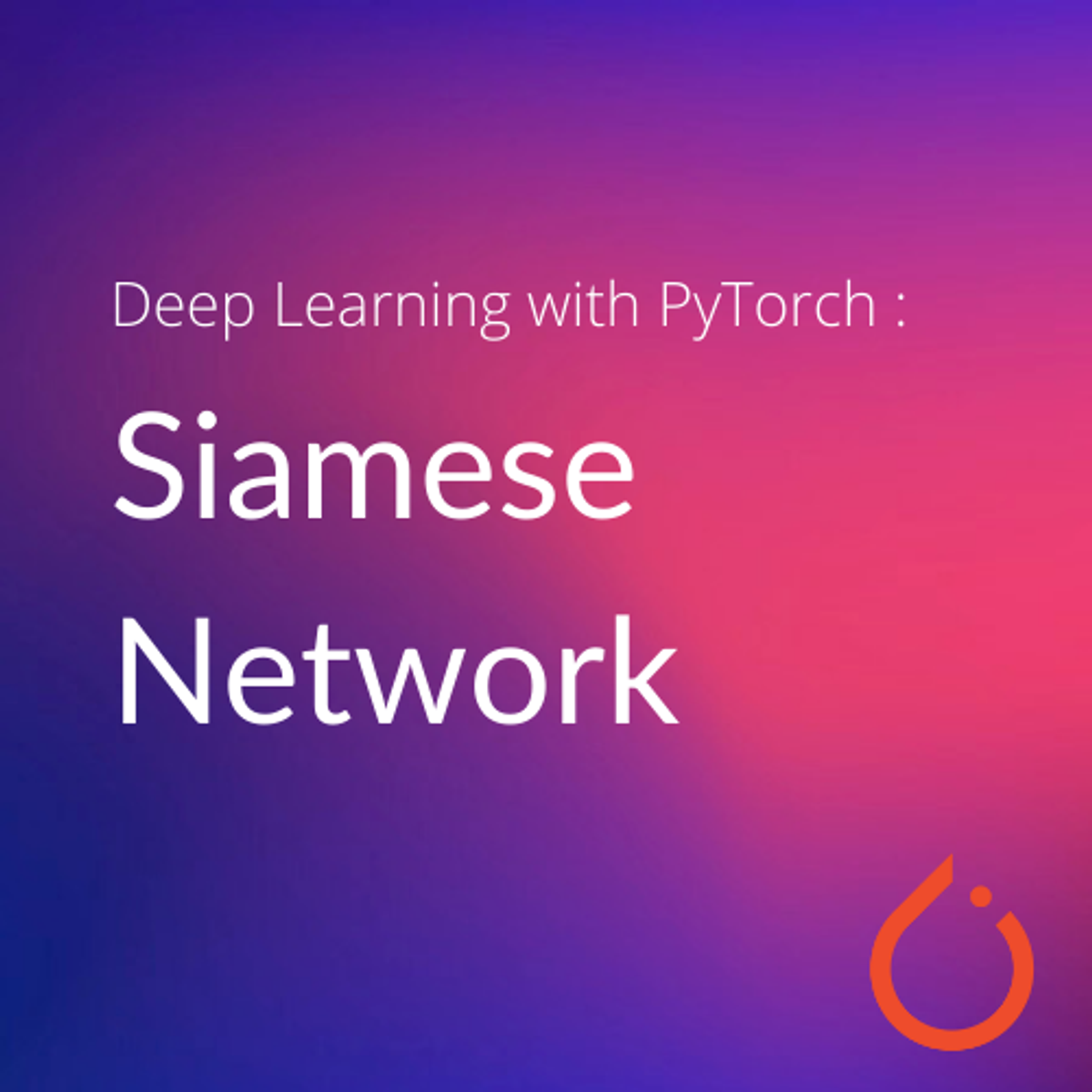
Deep Learning with PyTorch : Siamese Network
In this 2-hour long guided-project course, you will learn how to implement a Siamese Network, you will train the network with the Triplet loss function. You will create Anchor, Positive and Negative image dataset, which will be the inputs of triplet loss function, through which the network will learn feature embeddings. Siamese Network have plethora of applications such as face recognition, signature checking, person re-identification, etc. In this project, you will train a simple Siamese Network for person re-identification.
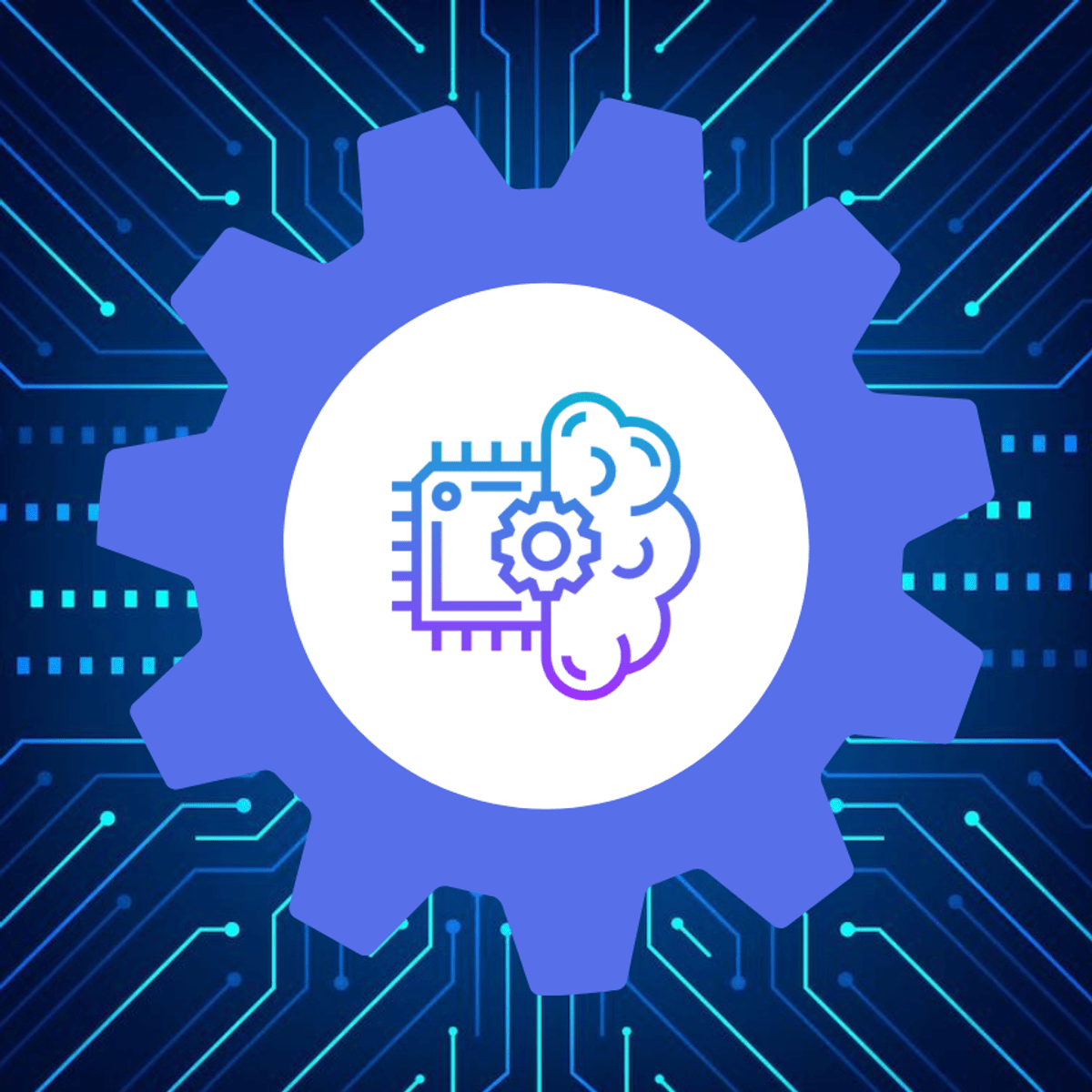
Analyze Data in Azure ML Studio
Did you know that you can use Azure Machine Learning to help you analyze data?
In this 1-hour project-based course, you will learn how to display descriptive statistics of a dataset, measure relationships between variables and visualize relationships between variables. To achieve this, we will use one example diabetes data. We will calculate its descriptive statistics and correlations, train a machine learning model and calculate its feature importance to see how features affect the label and visualize categorical data, as well as relationships between variables, in Jupyter notebook.
In order to be successful in this project, you will need knowledge of Python language and experience with machine learning in Python. Also, Azure subscription is required (free trial is an option for those who don’t have it), as well as Azure Machine Learning resource and a compute instance within. Instructional links will be provided to guide you through creation, if needed, in the first task.
If you are ready to learn how to analyze data, this is a course for you! Let’s get started!
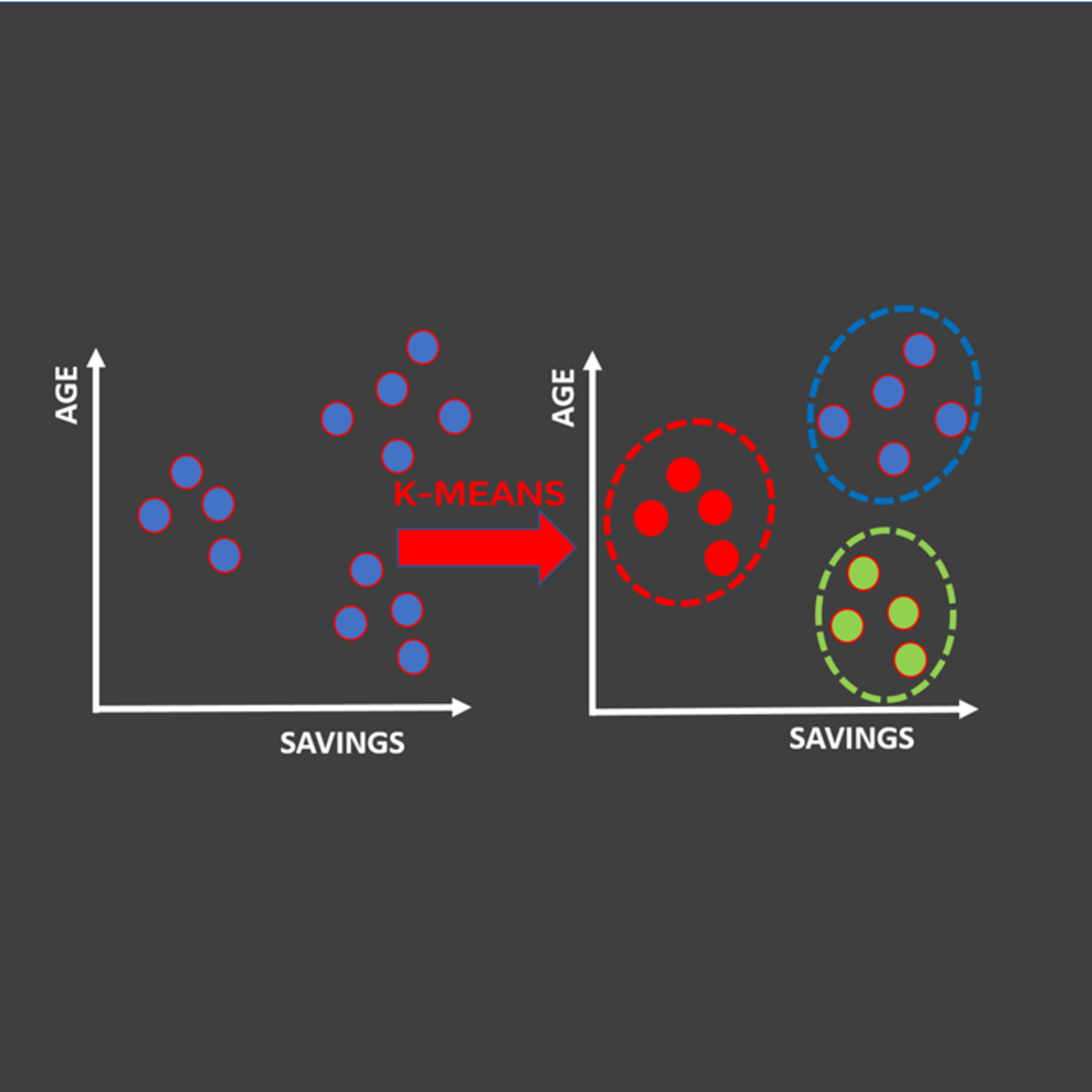
Unsupervised Machine Learning for Customer Market Segmentation
In this hands-on guided project, we will train unsupervised machine learning algorithms to perform customer market segmentation. Market segmentation is crucial for marketers since it enables them to launch targeted ad marketing campaigns that are tailored to customer's specific needs.
Note: This course works best for learners who are based in the North America region. We’re currently working on providing the same experience in other regions.
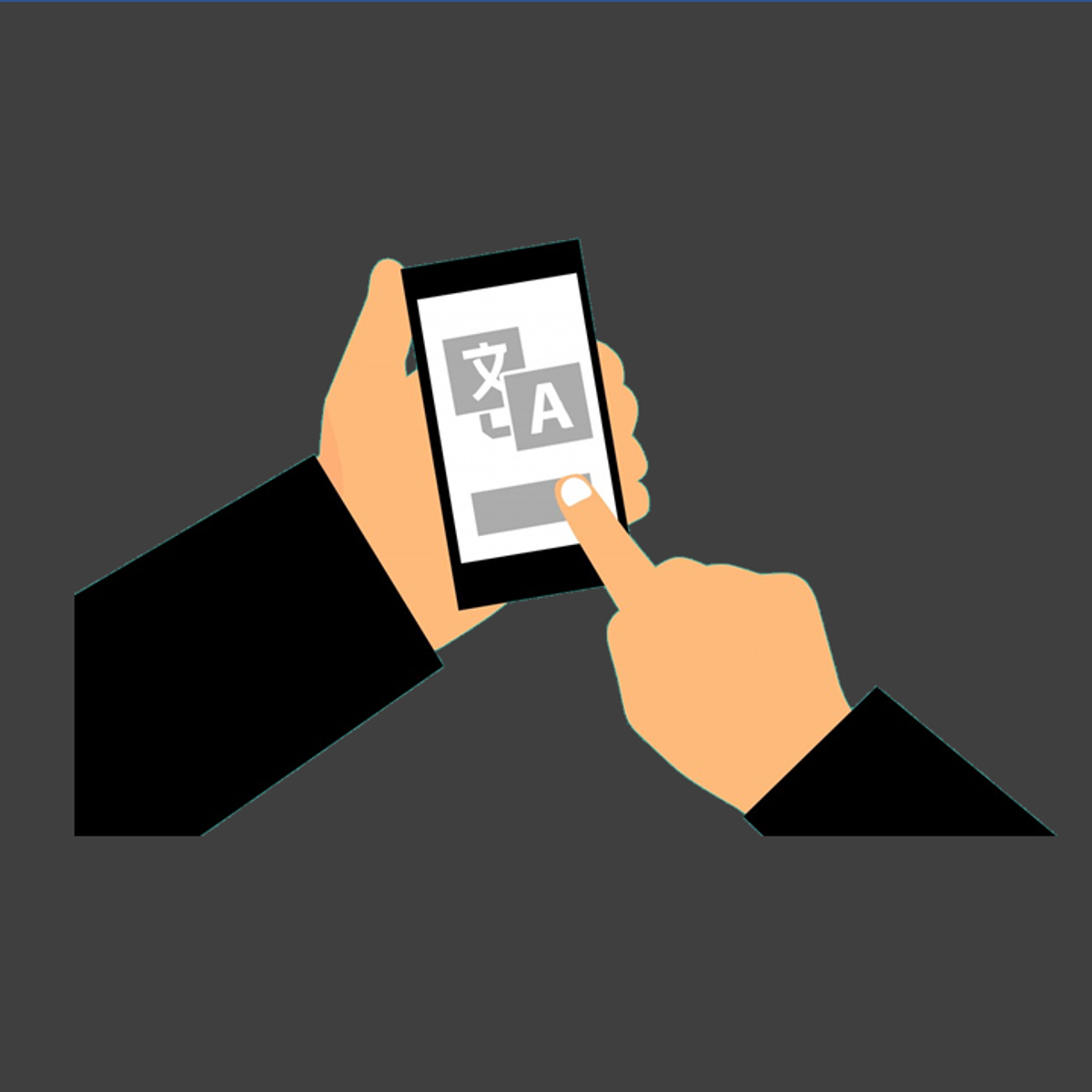
English/French Translator: Long Short Term Memory Networks
In this hands-on project, we will train a Long Short Term (LSTM) Network to perform English to French Translation. This project could be practically used by travelers or people who are settling into a new country.
Note: This course works best for learners who are based in the North America region. We’re currently working on providing the same experience in other regions.
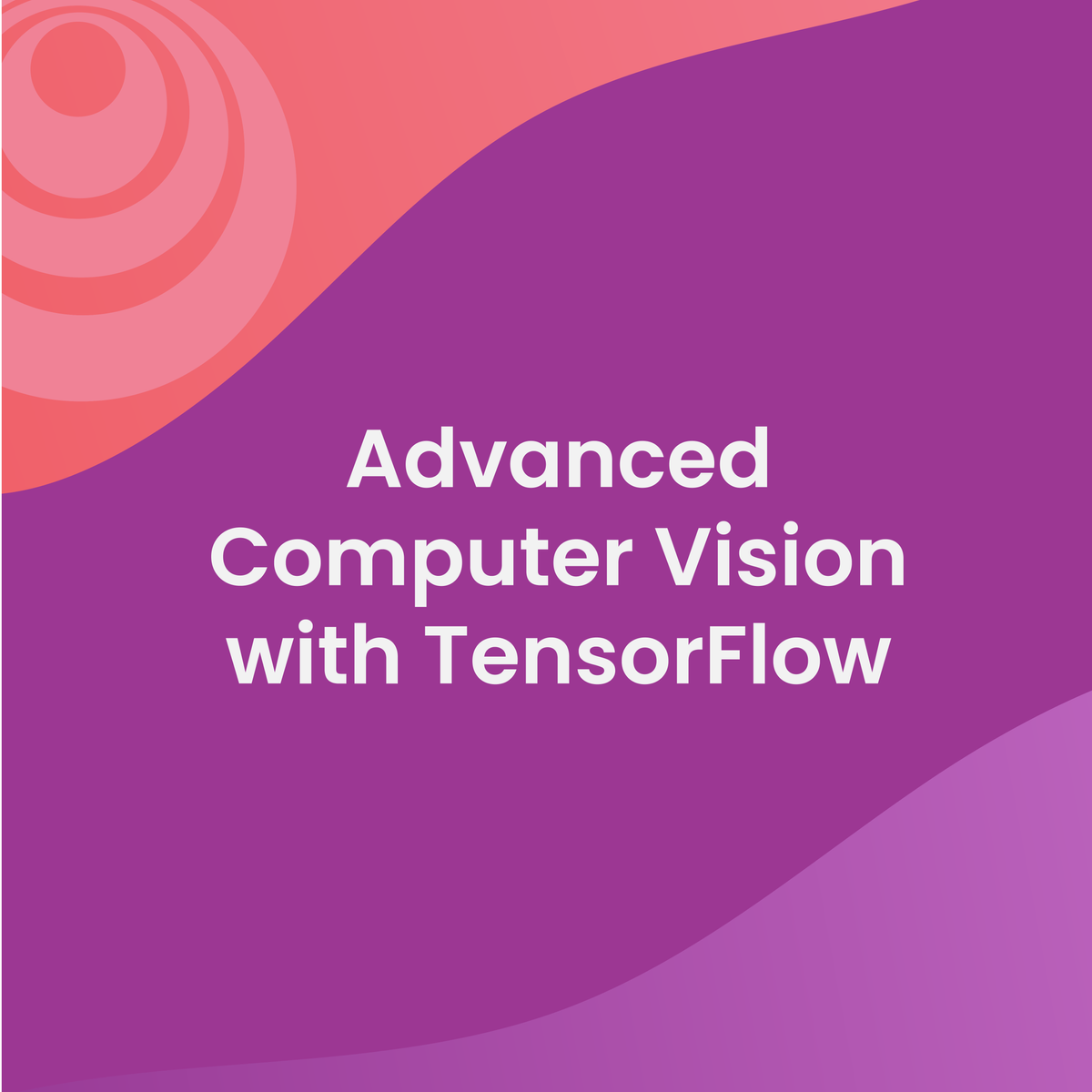
Advanced Computer Vision with TensorFlow
In this course, you will:
a) Explore image classification, image segmentation, object localization, and object detection. Apply transfer learning to object localization and detection.
b) Apply object detection models such as regional-CNN and ResNet-50, customize existing models, and build your own models to detect, localize, and label your own rubber duck images.
c) Implement image segmentation using variations of the fully convolutional network (FCN) including U-Net and d) Mask-RCNN to identify and detect numbers, pets, zombies, and more.
d) Identify which parts of an image are being used by your model to make its predictions using class activation maps and saliency maps and apply these ML interpretation methods to inspect and improve the design of a famous network, AlexNet.
The DeepLearning.AI TensorFlow: Advanced Techniques Specialization introduces the features of TensorFlow that provide learners with more control over their model architecture and tools that help them create and train advanced ML models.
This Specialization is for early and mid-career software and machine learning engineers with a foundational understanding of TensorFlow who are looking to expand their knowledge and skill set by learning advanced TensorFlow features to build powerful models.
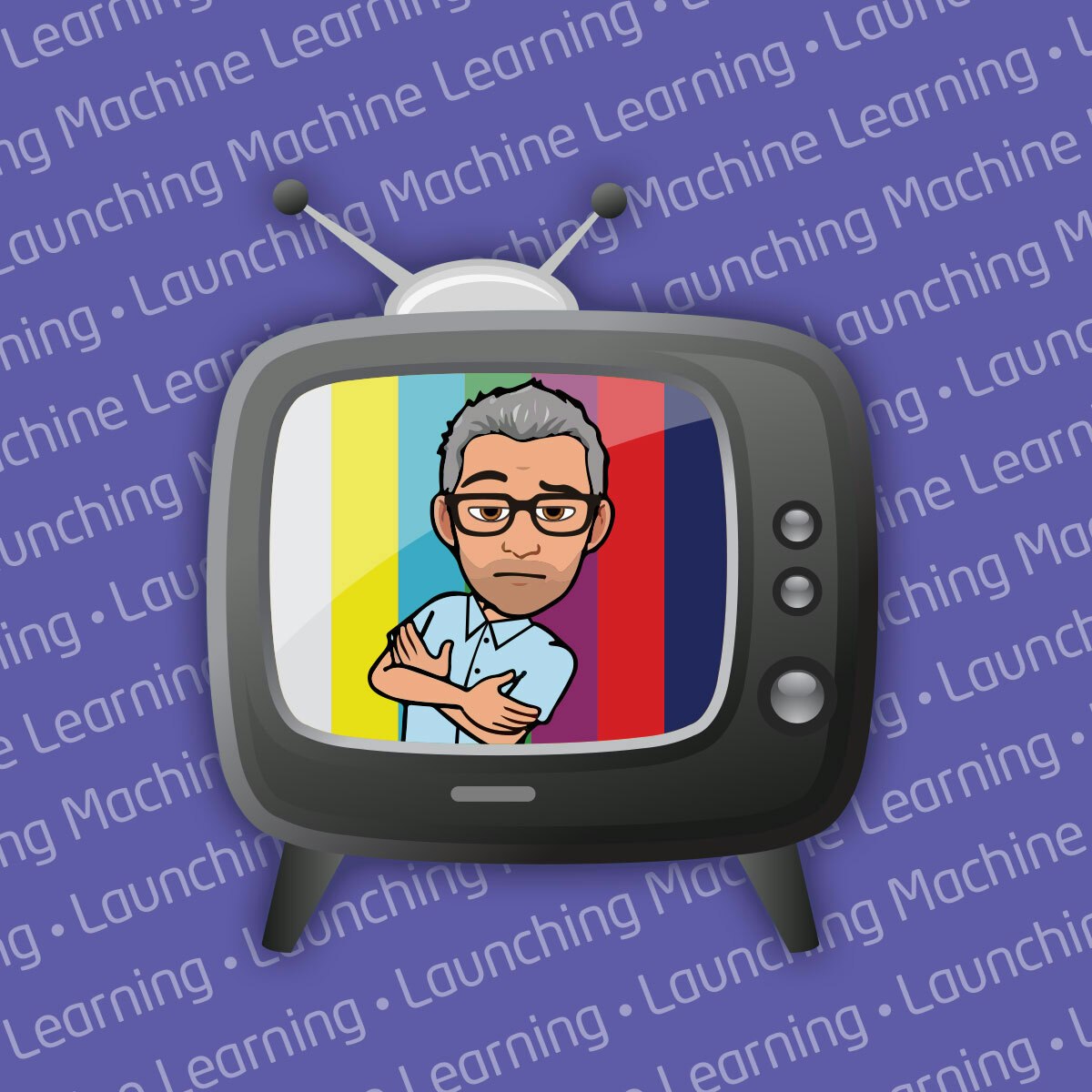
Launching Machine Learning: Delivering Operational Success with Gold Standard ML Leadership
Machine learning runs the world. It generates predictions for each individual customer, employee, voter, and suspect, and these predictions drive millions of business decisions more effectively, determining whom to call, mail, approve, test, diagnose, warn, investigate, incarcerate, set up on a date, or medicate.
But, to make this work, you've got to bridge what is a prevalent gap between business leadership and technical know-how. Launching machine learning is as much a management endeavor as a technical one. Its success relies on a very particular business leadership practice. This means that two different species must cooperate in harmony: the business leader and the quant.
This course will guide you to lead or participate in the end-to-end implementation of machine learning (aka predictive analytics). Unlike most machine learning courses, it prepares you to avoid the most common management mistake that derails machine learning projects: jumping straight into the number crunching before establishing and planning for a path to operational deployment.
Whether you'll participate on the business or tech side of a machine learning project, this course delivers essential, pertinent know-how. You'll learn the business-level fundamentals needed to ensure the core technology works within - and successfully produces value for - business operations. If you're more a quant than a business leader, you'll find this is a rare opportunity to ramp up on the business side, since technical ML trainings don't usually go there. But know this: The soft skills are often the hard ones.
After this course, you will be able to:
- Apply ML: Identify the opportunities where machine learning can improve marketing, sales, financial credit scoring, insurance, fraud detection, and much more.
- Plan ML: Determine the way in which machine learning will be operationally integrated and deployed, and the staffing and data requirements to get there.
- Greenlight ML: Forecast the effectiveness of a machine learning project and then internally sell it, gaining buy-in from your colleagues.
- Lead ML: Manage a machine learning project, from the generation of predictive models to their launch.
- Prep data for ML: Oversee the data preparation, which is directly informed by business priorities.
- Evaluate ML: Report on the performance of predictive models in business terms, such as profit and ROI.
- Regulate ML: Manage ethical pitfalls, such as when predictive models reveal sensitive information about individuals, including whether they're pregnant, will quit their job, or may be arrested - aka AI ethics.
NO HANDS-ON AND NO HEAVY MATH. Rather than a hands-on training, this course serves both business leaders and burgeoning data scientists alike by contextualizing the core technology, guiding you on the end-to-end process required to successfully deploy a predictive model so that it delivers a business impact. There are no exercises involving coding or the use of machine learning software.
WHO IT'S FOR. This concentrated entry-level program is for anyone who wishes to participate in the commercial deployment of machine learning, no matter whether you'll do so in the role of enterprise leader or quant. This includes business professionals and decision makers of all kinds, such as executives, directors, line of business managers, and consultants - as well as data scientists.
LIKE A UNIVERSITY COURSE. This course is also a good fit for college students, or for those planning for or currently enrolled in an MBA program. The breadth and depth of the overall three-course specialization is equivalent to one full-semester MBA or graduate-level course.
IN-DEPTH YET ACCESSIBLE. Brought to you by industry leader Eric Siegel - a winner of teaching awards when he was a professor at Columbia University - this curriculum stands out as one of the most thorough, engaging, and surprisingly accessible on the subject of machine learning.
VENDOR-NEUTRAL. This specialization includes illuminating software demos of machine learning in action using SAS products. However, the curriculum is vendor-neutral and universally-applicable. The contents and learning objectives apply, regardless of which machine learning software tools you end up choosing to work with.
PREREQUISITES. Before this course, learners should take the first of this specialization's three courses, "The Power of Machine Learning: Boost Business, Accumulate Clicks, Fight Fraud, and Deny Deadbeats."
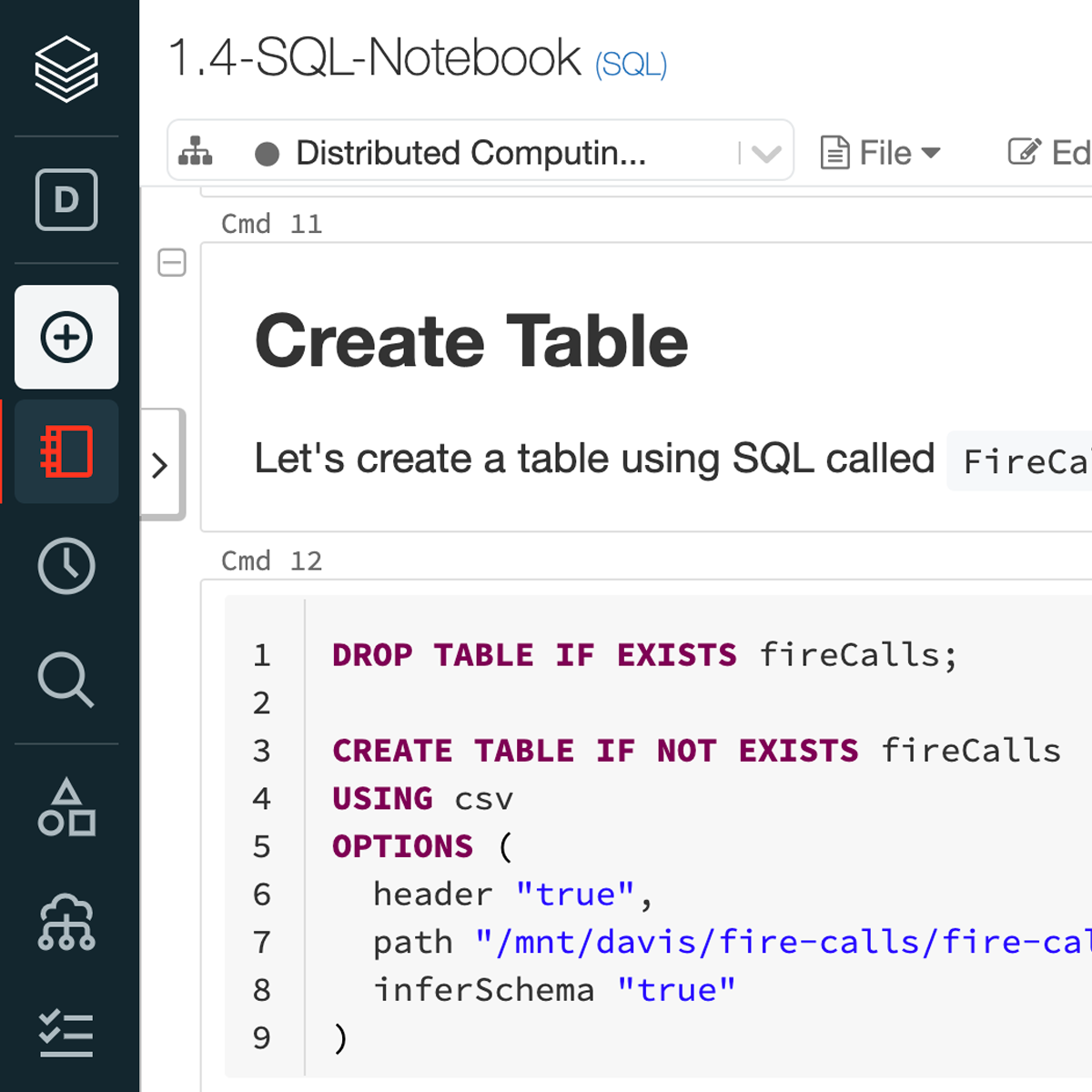
Distributed Computing with Spark SQL
This course is all about big data. It’s for students with SQL experience that want to take the next step on their data journey by learning distributed computing using Apache Spark. Students will gain a thorough understanding of this open-source standard for working with large datasets. Students will gain an understanding of the fundamentals of data analysis using SQL on Spark, setting the foundation for how to combine data with advanced analytics at scale and in production environments. The four modules build on one another and by the end of the course you will understand: the Spark architecture, queries within Spark, common ways to optimize Spark SQL, and how to build reliable data pipelines.
The first module introduces Spark and the Databricks environment including how Spark distributes computation and Spark SQL. Module 2 covers the core concepts of Spark such as storage vs. compute, caching, partitions, and troubleshooting performance issues via the Spark UI. It also covers new features in Apache Spark 3.x such as Adaptive Query Execution. The third module focuses on Engineering Data Pipelines including connecting to databases, schemas and data types, file formats, and writing reliable data. The final module covers data lakes, data warehouses, and lakehouses. Students build production grade data pipelines by combining Spark with the open-source project Delta Lake. By the end of this course, students will hone their SQL and distributed computing skills to become more adept at advanced analysis and to set the stage for transitioning to more advanced analytics as Data Scientists.
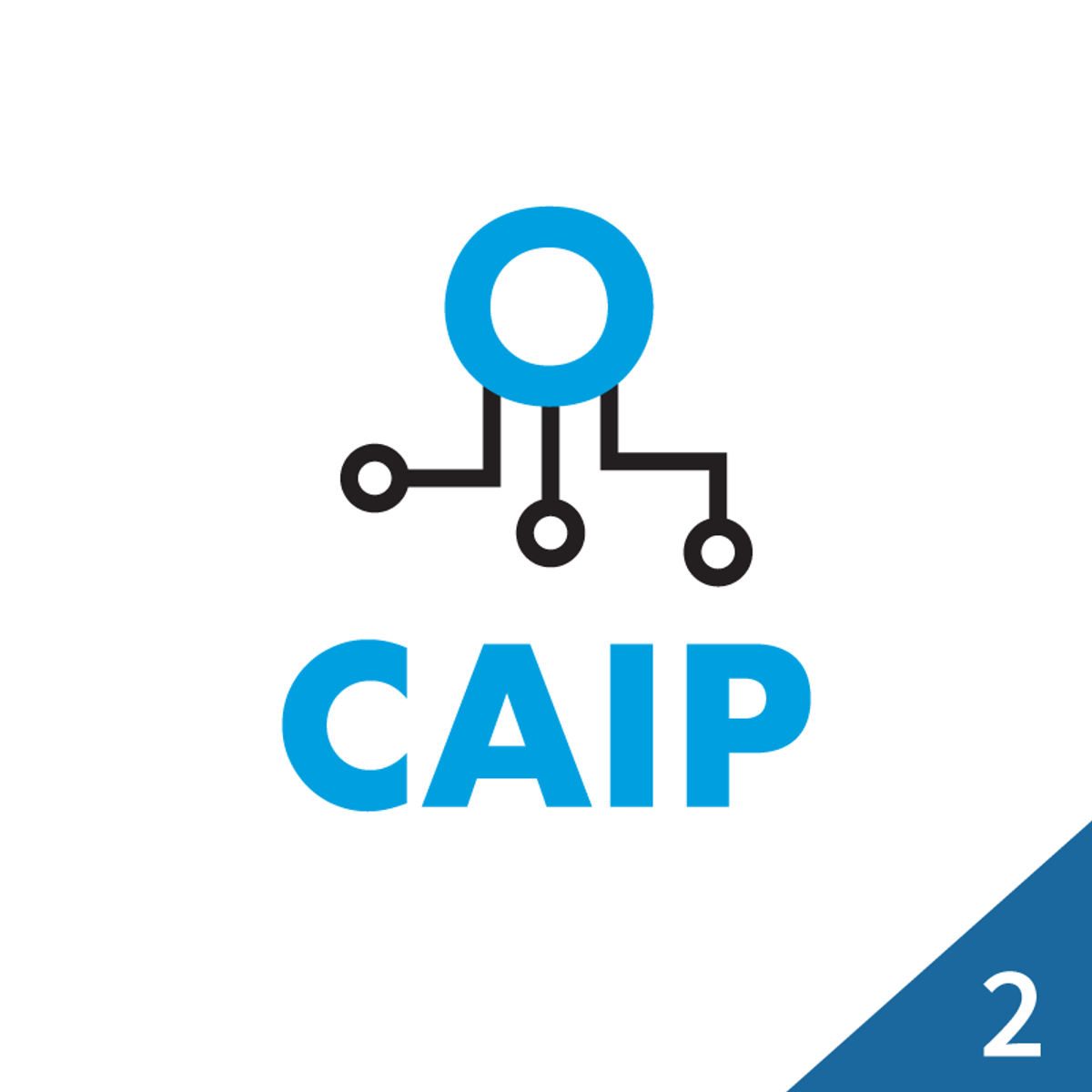
Follow a Machine Learning Workflow
Machine learning is not just a single task or even a small group of tasks; it is an entire process, one that practitioners must follow from beginning to end. It is this process—also called a workflow—that enables the organization to get the most useful results out of their machine learning technologies. No matter what form the final product or service takes, leveraging the workflow is key to the success of the business's AI solution.
This second course within the Certified Artificial Intelligence Practitioner (CAIP) professional certificate explores each step along the machine learning workflow, from problem formulation all the way to model presentation and deployment. The overall workflow was introduced in the previous course, but now you'll take a deeper dive into each of the important tasks that make up the workflow, including two of the most hands-on tasks: data analysis and model training. You'll also learn about how machine learning tasks can be automated, ensuring that the workflow can recur as needed, like most important business processes.
Ultimately, this course provides a practical framework upon which you'll build many more machine learning models in the remaining courses.
Popular Internships and Jobs by Categories
Browse
© 2024 BoostGrad | All rights reserved